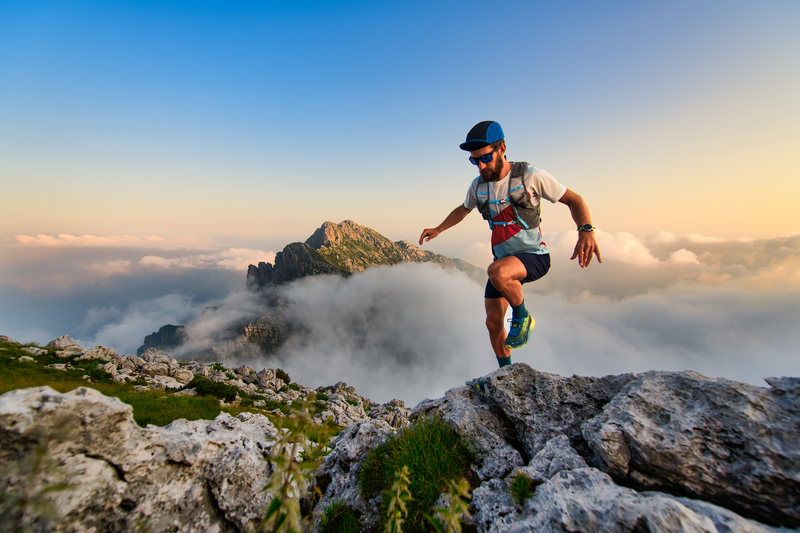
95% of researchers rate our articles as excellent or good
Learn more about the work of our research integrity team to safeguard the quality of each article we publish.
Find out more
EDITORIAL article
Front. Energy Res. , 08 July 2022
Sec. Nuclear Energy
Volume 10 - 2022 | https://doi.org/10.3389/fenrg.2022.965581
This article is part of the Research Topic Artificial Intelligence Applications in Nuclear Energy View all 13 articles
Editorial on the Research Topic
Artificial Intelligence Applications in Nuclear Energy
As a highly complex man-machine-network integration system, the nuclear power plant’s development, construction and operation are still facing many obstacles and risks. Firstly, plant instruments and equipment may fail during operation, which will affect the performance and safety of nuclear power plants. Secondly, although nuclear power plants have been digitalized after decades of development, most of them still adopt traditional and inefficient operation and control methods. Finally, due to the above reasons and stringent control requirements, human operators are under great pressure. In the past decades, artificial intelligence (AI) and machine learning (ML), especially methods related to deep learning, have made great progress and have been widely used in computer vision, automatic control and other fields (Bakator and Radosav, 2018; Singla et al., 2020; Taskiran et al., 2020; Usuga Cadavid et al., 2020). At present, many researchers have begun to apply AI to the field of nuclear energy to overcome the above obstacles and risks. Potential application scenarios include nuclear power software development (Bao et al., 2019; Liu et al., 2019), equipment prognostics and health management (Zhao et al., 2021; Zhong and Ban, 2022), reactor design optimization (Kumar and Tsvetkov, 2015; Turkmen et al., 2021), reactor autonomous control and operation (Wilson, 2019; Lee et al., 2020; Lin et al., 2021), and nuclear safety analysis and accident management (Zeng et al., 2018; Chung, 2021). This topic explores the application of the latest AI technologies in nuclear energy to promote research, sharing and development.
We have collected two papers on AI for nuclear power software development: Dong et al. and Wu et al. Dong’ work proposed a neural network-based data-driven model to predict the bubble departure diameter in subcooled boiling flow. The model is based on mechanistic bubble departure models and takes dimensionless numbers as input, thus demonstrating good generalization capability on a broad range of flow conditions.
We have collected three papers on intelligent prognostics and health management of plant equipment: Fan et al., He et al., and Yao et al. Fan’s work focused on the fatigue detection of glass-to-metal seals in nuclear power plants, with the assistance of the spectrum characterization of fiber Bragg grating (FBG) sensors. The spectral response to non-uniform strain distributions in glass-to-metal are reconstructed precisely based on the transfer matrix model, and the asymmetric deformation induced by fatigue conditions is detected efficiently by the variations of Bragg wavelength shift and full width at half maximum.
We have collected four papers on AI for reactor design optimization: Pevey et al., Zhang et al., Yu et al., and Li et al. Hines’ work proposed a convolutional neural network–based surrogate model optimization of fast neutron source configurations. Their new algorithm produced more viable designs that significantly improved the objective function utilizing the same computational resources compared to the standard multi-objective genetic algorithm NSGA-II.
We have collected three papers on AI for nuclear safety analysis and accident management: Gong et al., Dong et al., and Sallehhudin and Diab. With the assistance of a deep learning model called zLSTM, Gong’s work focused on the multivariate time series prediction for LOCA development. The zLSTM is constructed by introducing an improved gate function Zigmoid within the original LSTM model, allowing the non-linearity, both short and long-term memory, and multiple system parameters to be fully covered for a more accurate LOCA prediction.
As the development of AI technologies has accelerated in recent years, the nuclear industry has begun to look for the potential of AI for code development, real-time intelligent operation and maintenance, reactor design optimization, and safety analysis and accident management. The industry will follow suit if AI shows strong capabilities in research. In AI research, data as a carrier of knowledge plays a dominant role in the performance of AI and ML models. However, data containing valid information is scarce in the nuclear industry. In the coming period, the focus should be on how to make AI effective in practice under small sample, sample imbalance, and strong noise conditions. This may be a long-term challenge, but in the end all the effort will be worthwhile. In the future, with the popular application of AI technologies, the whole chain of the nuclear industry will become more intelligent.
All authors listed have made a substantial, direct, and intellectual contribution to the work and approved it for publication.
The authors declare that the research was conducted in the absence of any commercial or financial relationships that could be construed as a potential conflict of interest.
All claims expressed in this article are solely those of the authors and do not necessarily represent those of their affiliated organizations, or those of the publisher, the editors and the reviewers. Any product that may be evaluated in this article, or claim that may be made by its manufacturer, is not guaranteed or endorsed by the publisher.
Bakator, M., and Radosav, D. (2018). Deep Learning and Medical Diagnosis: A Review of Literature. Multimodal Technol. Interact. 2, 47. doi:10.3390/MTI2030047
Bao, H., Dinh, N. T., Lane, J. W., and Youngblood, R. W. (2019). A Data-Driven Framework for Error Estimation and Mesh-Model Optimization in System-Level Thermal-Hydraulic Simulation. Nucl. Eng. Des. 349, 27–45. doi:10.1016/j.nucengdes.2019.04.023
Chung, K. Y. (2021). A Machine Learning Strategy with Restricted Sliding Windows for Real-Time Assessment of Accident Conditions in Nuclear Power Plants. Nucl. Eng. Des. 378, 111140. doi:10.1016/j.nucengdes.2021.111140
Kumar, A., and Tsvetkov, P. V. (2015). A New Approach to Nuclear Reactor Design Optimization Using Genetic Algorithms and Regression Analysis. Ann. Nucl. Energy 85, 27–35. doi:10.1016/j.anucene.2015.04.028
Lee, D., Arigi, A. M., and Kim, J. (2020). Algorithm for Autonomous Power-Increase Operation Using Deep Reinforcement Learning and a Rule-Based System. IEEE Access 8, 196727–196746. doi:10.1109/ACCESS.2020.3034218
Lin, L., Athe, P., Rouxelin, P., Avramova, M., Gupta, A., Youngblood, R., et al. (2021). Development and Assessment of a Nearly Autonomous Management and Control System for Advanced Reactors. Ann. Nucl. Energy 150, 107861. doi:10.1016/j.anucene.2020.107861
Liu, Y., Sun, X., and Dinh, N. T. (2019). Validation and Uncertainty Quantification of Multiphase-CFD Solvers: A Data-Driven Bayesian Framework Supported by High-Resolution Experiments. Nucl. Eng. Des. 354, 110200. doi:10.1016/j.nucengdes.2019.110200
Singla, N., Kaur, M., and Sofat, S. (2020). Automated Latent Fingerprint Identification System: A Review. Forensic Sci. Int. 309, 110187. doi:10.1016/j.forsciint.2020.110187
Taskiran, M., Kahraman, N., and Erdem, C. E. (2020). Face Recognition: Past, Present and Future (A Review). Digit. Signal Process. 106, 102809. doi:10.1016/J.DSP.2020.102809
Turkmen, M., Chee, G. J. Y., and Huff, K. D. (2021). Machine Learning Application to Single Channel Design of Molten Salt Reactor. Ann. Nucl. Energy 161, 108409. doi:10.1016/j.anucene.2021.108409
Usuga Cadavid, J. P., Lamouri, S., Grabot, B., Pellerin, R., and Fortin, A. (2020). Machine Learning Applied in Production Planning and Control: a State-Of-The-Art in the Era of Industry 4.0. J. Intell. Manuf. 31, 1531–1558. doi:10.1007/s10845-019-01531-7
Wilson, J. (2019). Machine Learning for Nuclear Fission Systems: Preliminary Investigation of an Autonomous Control System for the MGEP. Cambridge, MA: Massachusetts Institute of Technology.
Zeng, Y., Liu, J., Sun, K., and Hu, L.-W. (2018). Machine Learning Based System Performance Prediction Model for Reactor Control. Ann. Nucl. Energy 113, 270–278. doi:10.1016/j.anucene.2017.11.014
Zhao, X., Kim, J., Warns, K., Wang, X., Ramuhalli, P., Cetiner, S., et al. (2021). Prognostics and Health Management in Nuclear Power Plants: An Updated Method-Centric Review with Special Focus on Data-Driven Methods. Front. Energy Res. 9, 696785. doi:10.3389/fenrg.2021.696785
Keywords: artificial intelligence—AI, machine learning—ML, software development, equipment prognostics and health management, reactor design optimization, nuclear safety analysis and accident management
Citation: Zhong X, Wang J, Zhao X, Liu Y and Revankar ST (2022) Editorial: Artificial Intelligence Applications in Nuclear Energy. Front. Energy Res. 10:965581. doi: 10.3389/fenrg.2022.965581
Received: 09 June 2022; Accepted: 20 June 2022;
Published: 08 July 2022.
Edited and reviewed by:
Uwe Schröder, University of Greifswald, GermanyCopyright © 2022 Zhong, Wang, Zhao, Liu and Revankar. This is an open-access article distributed under the terms of the Creative Commons Attribution License (CC BY). The use, distribution or reproduction in other forums is permitted, provided the original author(s) and the copyright owner(s) are credited and that the original publication in this journal is cited, in accordance with accepted academic practice. No use, distribution or reproduction is permitted which does not comply with these terms.
*Correspondence: Xianping Zhong, eGlhbnBpbmcuemhvbmdAcGl0dC5lZHU=; Jun Wang, andhbmc1NjRAd2lzYy5lZHU=
Disclaimer: All claims expressed in this article are solely those of the authors and do not necessarily represent those of their affiliated organizations, or those of the publisher, the editors and the reviewers. Any product that may be evaluated in this article or claim that may be made by its manufacturer is not guaranteed or endorsed by the publisher.
Research integrity at Frontiers
Learn more about the work of our research integrity team to safeguard the quality of each article we publish.