- Center of Data Economics & Network Study, ICOI, Shenzhen University, Shenzhen, China
In the era of globalization, industries of critical metals are organized through the global supply chain. However, the global supply chains have been disrupted since 2020 by the outbreak of COVID-19 and a series of geopolitical crises. To better address the supply chain challenges of critical metals, a review is needed about the sources, propagation, and responses of the supply chain risks. Firstly, this review provides an overview about the research progress in identifying the risk sources and assessing the risks and then proposes a new supply chain framework, categorizing relevant risk factors into upstream risks, middle-stream risks, downstream risks, and general risks, for risk analysis of critical metals. Secondly, this review offers a comprehensive understanding about how the risks propagate horizontally and vertically. Finally, responses such as supply diversification, stockpiling, material substitution, recycling and circular economy strategy, price volatility hedging, and supply chain traceability are reviewed. This survey features the supply chain perspective, overviews on network-based studies, and affirms the urgency and need for further studies on supply chain risks and resilience, which may contribute to a smooth clean energy transition.
Introduction
Critical metals usually refer to rare metals, rare disperse elements, and rare earth elements that play irreplaceable and significant roles in new energy industries—from solar photovoltaic and wind turbines to electric vehicles and battery storage (Zhai et al., 2019; IEA, 2021a). Gallium, tungsten, rare earths, bismuth, antimony, magnesium, germanium, vanadium, molybdenum, indium, tin, silver, lithium, niobium, beryllium, nickel, cobalt, chromium, platinum, and copper are recognized as the critical metals for low-carbon technologies (Wang et al., 2021a; Zuo et al., 2021). With the massive and increasing deployment of low-carbon technologies for a net zero emission society, demands for these metals have been increasing significantly and the world is moving from fuel-intensive systems to more material-intensive systems (IEA, 2021b). Against this background, it is important to review the research progress related to these metals.
Nowadays mining, refining, manufacturing, use, and waste management of critical metals are sliced and located across the world, forming various global supply chains of critical metals, as shown in Figure 1. In the supply chain environment, productions are interconnected, and the effective operation of one production stage depends increasingly on the normal operation of the other stages which may be located differently (Ponomarov and Holcomb, 2009). Under this circumstance, disruptions of any stage can be propagated either upstream or downstream. A resilient supply chain of critical metals is therefore of high importance to the global energy transition, which, however, experiences more and more unexpected disruptions.
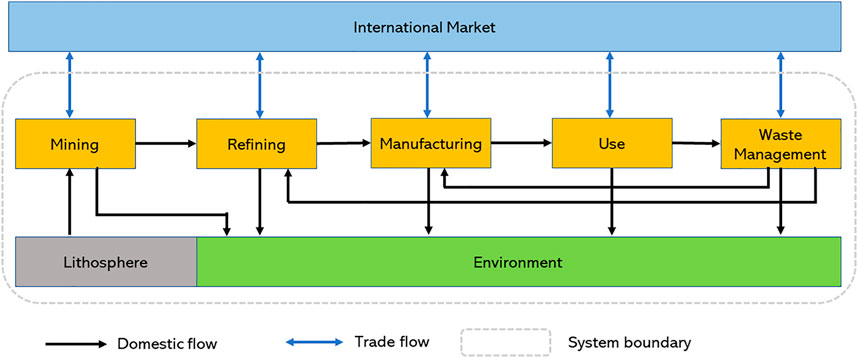
FIGURE 1. Overview of the supply chain of metals. This figure is based on the studies of Muller et al. (2014), Chen et al. (2019), Sun et al. (2019), and Di et al. (2022).
Since 2020, the global pandemic has pushed governments to adopt various lockdown policies, which seriously affect the operations of global supply chains (Guan et al., 2020), which include those of critical metals. It has been reported that South Africa’s lockdown disrupted 75% of the global output of platinum, and Peru’s copper-mining activities ground to a halt due to its anti-pandemic policy (IEA, 2020). Besides the pandemic crisis, the global supply chains of critical metals were hit unexpectedly by the Russia–Ukraine event in 2022. Russia is responsible for 10.69% of global production of nickel and 4.4% of global production of cobalt (USGS, 2021), this geopolitical crisis made the market worry about the supply availability from Russia, and prices of nickel and cobalt have undergone high volatilities accordingly. Crises that occurred in the mining stage of the supply chain have disturbed downstream significantly. It is reported that prices of power lithium-ion batteries and electric vehicles have spiked (IEA, 2022). The pandemic crisis and frequent geopolitical events highlight that maintaining resilient supply chains of critical metals is an urgent issue to be addressed, and its related studies have become the global frontiers and hot topics (Ibn-Mohammed et al., 2021). This review aims to provide an overview on the research progress achieved in identifying and assessing supply chain risks, analyses of the propagation of risks, and responses to supply chain risks of critical metals.
Many reviews have been carried out on critical metals. Achzet and Helbig (2013) and Schrijvers et al. (2020) reviewed the methods to determine raw material criticality. Watari et al. (2020) emphasized the research progresses on the long-term outlook and sustainability of 48 metals. Wang et al. (2021a) did a review on the nexus between low-carbon energy and critical metals. Swain and Mishra (2019) summarized the various processes developed for the separation of rare earths and transition metals from secondary resources. Miao et al. (2022) provided an overview on the critical metal recycling associated with global power lithium-ion batteries. Using CiteSpace, an increasingly applied tool for scientometrics reviews, Zuo et al. (2021) and Wang et al. (2019) analyzed the research clusters, cooperation networks, and burstiness for strategic mineral resource security and resource recycling industry. Nevertheless, we currently lack a picture of the progress on the supply chain risks of critical metals. The absence of such review impedes our comprehensive understanding of supply chain resilience of critical metals, which in turn could possibly mislead the policy-decision makings for clean energy transitions. This review is related to the review by An and Li (2022) who emphasized various interactions in the industrial chains at the macro and micro levels. This review, different from theirs, focuses on the supply chain risk sources, propagation of the risks, and responses to the risks and thus makes a contribution to building a resilient supply chain of critical metals.
The remainder of this review is organized as follows: Section 2 introduces risks identification and assessment; Section 3 presents the propagation of risks in the global supply chain networks of critical metals; Section 4 discusses responses to the supply chain risks, followed by the last section of conclusion.
2 Identifying risk sources and assessing risks
2.1 Supply risks in criticality assessment
In the field of criticality assessments of metals, supply risk is a key component, as shown in Eq. 1 (Graedel et al., 2012; Graedel et al., 2015). Identifying and assessing the supply risks thus become the starting points to assess the criticality (Achzet and Helbig, 2013).
In an overview of the raw material supply risks, Achzet and Helbig (2013) summarized that there are 20 indicators to evaluate the supply risks, namely, country concentration, country risk (social and political factors), depletion time, by-product dependency, company concentration in mining corporations, demand growth, recycling potential, substitutability, import dependence, commodity prices, exploration degree, production costs in extraction, stock keeping, market balance, mine/refinery capacity, future market capacity, investment in mining, climate change vulnerability, temporary scarcity, risk of strategic use, and abundance in the Earth’s crust. Besides these conventional risks, trade risk, natural disasters, logistic restrictions, resource competition, and ore concentration are newly added indicators (Klimek et al., 2015; Hao et al., 2018; Schrijvers et al., 2020; Althaf and Babbitt, 2021). Some scholars also propose the thermodynamic rarity dimension of raw materials. That is, if a material is obtained in energy-intensive ways and is scarce in nature, it is thermodynamically rare (Calvo et al., 2018). Relevant studies using these indicators are listed in Supplementary Table S1.
Due to the various economic structures and resource endowments, different economies have adopted differentiated methods to assess supply risks. For example, in the European Commission (EC)’s supply risk assessment, the supply risk is measured as a product of supply concentration, import reliance, governance performance, trade adjusted parameter, and substitution index (European Commission, 2017; Blengini et al., 2020), as expressed by Eq. 2:
where SR denotes the supply risk, HHI is the Herfindahl–Hirschman Index to proxy for country concentration, WGI is the scaled World Governance Index to proxy for country governance, t is the trade adjusted parameter, GS is the global supplier countries mix,
In the supply risk evaluation framework of the United States, the National Research Council identified five supply risk sources, namely, demand growth, thin markets, production concentration, by-product production, and recycling. Based on this research, the United States Department of Energy (DOE) developed a weighted index for evaluating supply risk, in which 40% is given to basic availability of metals, 20% to political, social, and regulatory factors, 20% to producer diversity, 10% to competing technology demand, and 10% to codependence to other markets. The United States framework was later developed by Graedel et al. (2012), which differs medium-term risks from long-term risks.
Low-carbon technologies have been massively deployed in China, and some of them have the largest market size in the world (e.g., electric vehicles). To develop a criticality assessment for the Chinese context, Yan et al. (2021) analyzed three types of supply risks, i.e., sustainability risk, reliance risk, and tolerance risk, in identifying the critical metals for China, as given in Eq. 3:
The illustrated cases show that not all these indicators are utilized in each assessment of the supply risks. The selection of indicators depends on the goal and scope of the relevant research; accordingly, there is no unified assessment of supply risks and the lists on critical metals are varying (Helbig et al., 2021). It is noteworthy, however, that among the 26 used indicators for supply risks, country/company concentration, country risk, depletion time, by-product dependency, and demand growth are the most frequently chosen indicators for evaluating supply risks (Achzet and Helbig, 2013; Schrijvers et al., 2020). It has been pointed out by Watari et al. (2020) that demand-related risks, social and environmental risks induced by the growing demand for metals, spatial divergence, and circular economy strategies should be further studied. They also held that life cycle assessment and the input–output model are the potential tools that better monitor the supply risks, which is closely related to the supply chain concept. Therefore, it has been suggested that a supply chain perspective be adopted for analyzing the supply risks in later criticality assessments.
2.2 From supply risks of single stage to supply chain risks
From the perspective of a supply chain, the supply risks can be categorized into four groups, namely, upstream risks, middle-stream risks, downstream risks, and general risks, as shown in Figure 2. The general risks are the disruptions that could occur at any stage of the supply chain. This perspective could provide a more holistic view about the supply chain risks of critical metals and a better understanding of the existing supply risk studies. Most existing studies on the supply risks of critical metals focus on the upstream risks, and only a few analyze the potential bottlenecks in middle-stream, downstream (Schrijvers et al., 2020), and the whole supply chain.
Shocks in the middle-stream and downstream, however, should be fully acknowledged. For the shocks to the middle-stream, the supply chain of metals is seriously affected due to China’s processing role in the global production network (Dente and Hashimoto, 2020). Disruption to the downstream could be positive or negative. For example, the end-use structure change could significantly affect the supply–demand balance of materials and cause supply risks in the upstream (Zeng and Li, 2015). Recycling from the end-of-life products also deserves attention (Fu et al., 2019; Rasmussen et al., 2019). In addition, evidence shows that there are a few countries that participate in the whole chain and own global influences (Shi et al., 2022). Ignoring the potential disruptions in the key countries impedes the effective management of supply chain risks. Acknowledging these shortcomings, the European Commission exercised a double-stage supply risk assessment for the first time (Blengini et al., 2020), and van den Brink et al. (2020) examined the supply chain risks for cobalt. Yan et al. (2020) developed a supply resilience assessment framework that includes upstream and downstream factors for the Chinese lithium-ion battery industry.
There are four theoretical tools to analyze the supply chain risks. The first is the material flow analysis (MFA), which is widely used in analyzing the material cycles of metals in the anthroposphere (Muller et al., 2014). Since MFA can trace the flow volume along the whole supply chain of metals, the derived results can be used as parameter inputs (especially the trade-related factors) to the supply risk analysis and better monitor the risks. Nuss and Blengini (2018) developed a model combining criticality assessment and MFA for the European Union. Sun et al. (2020) discussed the supply chain risks for manganese. The second is the multiregional input–output (MRIO) model, which can reveal indirect risks through the input–output linkages between sectors across the world. In this context, Nansai et al. (2015) proposed a global mining risk footprint of critical metals, and Nansai et al. (2017) highlighted the role of primary processing in the supply risks of critical metals. The third one is system dynamics proposed and developed by Forrester (1994) and has been adopted for the rare earth supply chain disturbance (Sprecher et al., 2015) and tantalum supply chain risk (Mancheri et al., 2018). The last tool is complex network analysis, which is an effective and widely used tool for analyzing the interactions between the actors and structures of the resultant complex systems (Barabasi and Albert, 1999; Newman, 2003). Klimek et al. (2015) developed network-based indicators for trade risks. Based on the global copper trade network, Li et al. (2021) innovatively introduced betweenness, an indicator for reflecting the position and resource control capability of one country in the trade network, into the supply chain risk analysis.
3 Propagation of risks in global supply chain networks of critical metals
There are several terminologies for the propagation of risks in the academic literature, which include risk diffusion (Basole and Bellamy, 2014), cascading failures, ripple/domino effect (Dolgui et al., 2018), supply chain disruption propagation (Scheibe and Blackhurst, 2018), and risk contagion (Paltalidis et al., 2015). These terms may be applied in different fields. For example, risk diffusion is closely related to the epidemiological models (Zhao et al., 2018); cascading failures and ripple/domino effects are often used by scholars in complex network studies (Ash and Newth, 2007; Xia et al., 2010); and risk contagion is a widely used term related to the systemic risk studies in the financial literature (Gai and Kapadia, 2010; Elliott et al., 2014; Jackson and Pernoud, 2021). Nonetheless, the essence of these terminologies is the same, i.e., referring to the propagation of both endogenous and exogenous risks from one node to the other nodes (Basole and Bellamy, 2014) and generating an amplified impact in the system (Ojha et al., 2018). Failure nodes, propagation links, and amplified impact are three core elements for the definition of the propagation of risks. The risk/shock propagation, therefore, can be defined as the process that failure nodes amplify their impact through various channels. Trade links, input–output linkages/supply–customer relations, and price correlations are the most common propagation channels.
The global supply chains of critical metals are complex systems that contain various economic and financial connections. These connections provide various channels for shock/disruption of one node to propagate to its directly connected nodes, which then propagate their crises to the other connected nodes. This process continues until the disruption effects are absorbed totally by the chain or all the nodes in the supply chain are jeopardized. The propagation process in the global supply chain of critical metals is essentially the same as the virus-spreading phenomenon, which has been widely analyzed by network-based models (Newman, 2002; Pastor-Satorras et al., 2015). Due to trade links and price correlations being the most studied channels, this review mainly summarizes the current status of these two fields.
3.1 Propagation of shocks in single-layer trade network
Systemic trade-related risks have been widely acknowledged (Klimek et al., 2015). The resource trade network consists of nodes and links (An et al., 2014; Zhong et al., 2014). In the context of international trade, for example, if country A imports cobalt minerals from country B, then there is a link between A and B. The trade links thus provide a channel for crisis contagion (Lee et al., 2011). By constructing a trade network of critical metals and applying the network metrics, Wang et al. (2018) and Sun et al. (2022) firstly identified the systemic importance of economies for the graphite trade network and cobalt trade network, respectively. Then they established cascading failure models and analyzed the propagation processes caused by the failures of systemic importance economies. It is found that GDP and export volume affect the scope of the influence (Wang et al., 2018), and indirect links play a key role in the propagation process (Sun et al., 2022). The main contribution of these studies is that they provide a model framework to identify the propagation paths and understand the systemic impacts of epicenter economies. There are many cases where more than two materials are required to produce one good. For example, cobalt, lithium, and nickel are the necessary materials to produce lithium-ion battery and are called joint consumption products (Shammugam et al., 2019). To analyze the shock of technology progress affecting the trade of joint consumption products, Shao et al. (2022) constructed a cobalt–lithium trade network and simulated the structural changes under trade weight preference and trade country preference scenarios.
Besides the disruptions that happened in the upstream, Wu et al. (2021) designed a non-dominated sorting genetic (NSGA-II) algorithm to identify the key countries and trade relations in the stability of the lithium carbonate trade network, which enriches the understanding of potential supply risk propagation paths in the trade network of middle-stream products. As for the risk propagations in the downstream of the supply chain, disruptions spreading caused by node failure and link interruption in the lithium-ion battery trade network (Hu et al., 2021) and pandemic-related disruption contagion in the solar panel trade network (Wang et al., 2021b) have been studied and both are based on single-layer networks. All these studies only focus on the geographic disruption propagations, ignoring the propagation along the whole global supply chain.
3.2 Propagation of disruptions in multiplex trade network
From the perspective of a multiplex network, mining, refining, manufacturing, consumption of end-use products, and waste management of the supply chain are different layers (Shi et al., 2022), as illustrated in Figure 3. In each layer, countries exchange goods through international trade, forming a single-layer network. Single-layer networks connect via global input–output linkages, forming an interdependent multiplex network. In this interdependent multiplex network, the failure of nodes in the mining stage leads to failure of the dependent nodes in middle-stream or downstream layers, which in turn may cause further damage to the first network, leading to cascading failures and possibly catastrophic consequences (Gao et al., 2014). To design resilient supply chains of critical metals, we should understand how vulnerability is affected by such interdependences.
Through investigating the interdependencies between the copper raw materials–trading network and its waste-trading network, Hu et al. (2020) analyzed the direct and indirect impacts of China’s restrictive scrap imports on the global copper multiplex network. It should be noted that their multiplex shocks model only contains two stages of the supply chain and implies research gaps for future studies. With the development of lithium-ion batteries containing high nickel elements, more attention is being paid to the supply chain of nickel. Wang et al. (2022) developed a multilayer network crisis propagation model to simulate the disruption spreading in the global nickel industry chain.
3.3 Price volatility spillover/transmission
Price volatility is also recognized as an important risk, which significantly affects the supply–demand balance, trade dependence structure (Zhao et al., 2022), investment decisions, and other sectors of the economic system (Sun et al., 2018; Sun et al., 2019). Understanding the price volatility spillovers is helpful in hedging the price risks. From the perspective of a single-layer network, price volatility spillovers are transmitted horizontally and occur at different levels, from firms, commodities, markets, sectors, countries, to time, which have been studied intensively. Guo et al. (2019) examined simultaneously the price transmissions across countries and cross products in the middle-stream of the steel industrial chain. An et al. (2020) constructed a network model and analyzed the dynamic volatility spillovers among bulk mineral commodities, such as cobalt, nickel, and copper. Li et al. (2021) analyzed the dynamic joint impacts of gold and oil prices on the copper price. These studies feature the combination of econometric modeling and network analysis. Using a firm’s high-frequency data, Zheng et al. (2021) studied the asymmetric connectedness and dynamic spillovers between renewable energy and rare earth markets in China. Zhou et al. (2022) investigated the spillovers from China’s rare earths stock prices to its trading partners. Considering the substitute effect of clean energy, a series of studies have been conducted to examine the spillover effects of fossil energy prices on clean energy metal prices (Shao and Zhang, 2020; Hammoudeh et al., 2021; Niu, 2021; Shao et al., 2021; Chen et al., 2022). There are also studies examining the price volatility links between the rare earth market and financial market (Reboredo and Ugolini, 2020; Bouri et al., 2021; Song et al., 2021).
From the perspective of the supply chain, price volatility is transmitted vertically. The vertical transmission can be further categorized into forward vertical transmission, backward vertical transmission, and bidirectional transmission. In a study on the dynamic relationship between primary and scrap prices, Xiarchos and Fletcher (2009) found that scrap prices do not improve the long-run interpretation of primary prices of copper, lead, and zinc, which, however, exist in the short run. In recent years, network-based transmission models are developed to reveal the sources, receptors, hubs, media, paths, and motifs of price spillovers. Related studies have been examined in the steel supply chain (Liu et al., 2019; Qi et al., 2020) and rare earth supply chain (Jia et al., 2021).
4 Responses to supply chain risks
The responses to the supply chain risks are essential to improve supply chain resilience, which is defined as “the capacity to supply enough of a given material to satisfy the demands of society, and to provide suitable alternatives if insufficient supply is available” (Sprecher et al., 2015). On the supply side, the diversity of supply and increasing stockpiling are proposed to improve supply chain resilience of critical metals; on the demand side, improving material efficiency and developing material substitution are advocated (Sprecher et al., 2015; Sprecher et al., 2017; Mancheri et al., 2018). Recycling and circular economy strategies are also widely promoted (Hua et al., 2020; Zeng and Li, 2021).
4.1 Responses on supply side
Supply diversification. More sources of raw materials are helpful in reducing damages caused by disruptions. Using rare earths as an illustrated case, with increasing alternative supplies from Australia and the United States, the HHI of rare earths has decreased from 0.68 in 2017 to 0.43 in 2019, which could potentially decrease to 0.34 (Althaf and Babbitt, 2021). This result highlights that diversifying supply could substantially reduce the risks from supply concentration. Besides expanding sources, increasing domestic mineral extraction could also effectively alleviate the supply risk of raw materials (Yan et al., 2020). Currently, the mostly advocated diversity of supply is about the diversified ores supply. It should be noted, however, that the availability of domestic mining and extraction technologies is the prerequisite for domestic production. In addition, supply diversification comes at the cost of regulatory limits, high social and environmental risks, high economic costs, etc. (Althaf and Babbitt, 2021). Furthermore, from the supply chain perspective, the supply diversification in the upstream is not enough. It is argued that investment in all stages of the chain can realize true resilience, which is unfortunately against the basic law of comparative advantages that drives globalization.
Increasing stockpiling. Stockpiling is a short term and effective tool to hedge supply disruption and price hikes (Sun et al., 2022) and can be applied to any stage of the supply chain. A critical issue of stockpiling is the feedback loop through the price mechanism (Sprecher et al., 2015). When a supply disruption occurs, emergency stockpiling by actual needs and speculation activities will drive up the demand and price, which in turn lead to pessimistic expectations about supply and more stockpiling. On the contrary, releasing inventories will increase the supply and ease the market, which in turn negatively influences the price. How does this price mechanism interact with the stockpiling behavior in the field of critical metals? There are few studies in this regard to date.
Recycling and circular economy strategy. Supply diversification and stockpiling cannot sufficiently mitigate the supply chain's risks. The supply chain is a closed loop, and in the reverse supply chain/circular value chain, old scrap/waste can be redesigned, reused, remanufactured, and recycled/urban-mined, therefore, recycling and circular economy strategy are treated as a sustainable solution to reduce the supply chain risks (Zeng and Li, 2015; Hua et al., 2020; Baars et al., 2021; Zeng and Li, 2021; Miao et al., 2022). It is estimated that there are 0.21–0.52 million tons (Mt) of lithium, 0.10–0.52 Mt of cobalt, and 0.49–2.52 Mt of nickel contained in the global end-of-life batteries (Xu et al., 2020). Using dynamic material flow analysis, Wang and Ge (2020) estimated China’s urban cobalt mines could reach 78,800–186,500 tons. At the city level, the urban mines are estimated to be in the range of 300–500 kilotons from 2015 to 2050 in Hong Kong SAR of China, and the economic potential will be 2 billion US dollar each year (Kuong et al., 2019). Taking full advantage of these recycling potentials requires clear recycling targets, public education, a comprehensive recycling system at the national scale, abundant treatment capacities, mature recycling technologies, recycling-oriented design strategies, cost competitiveness, and effective fiscal incentives (He et al., 2020; Wang and Ge, 2020; Tang et al., 2021; Mao et al., 2022; Shahjalal et al., 2022). However, these are still at a very early stage in the context of the global battery industry (Tang et al., 2021; Mao et al., 2022). Global efforts are called for studying these aspects to promote the economic and sustainable recycling strategies.
Supply chain traceability. Social and environmental risks draw more and more attention, for example, the artisanal mining in the Democratic Republic of the Congo (Nkulu et al., 2018). To reduce related risks, it is suggested to establish a supply chain traceability system (Shi, 2022). Due to the advantages of decentralized control, security, traceability, and auditable time-stamped transactions (Shi and Sun, 2020; Omar et al., 2022), blockchain is proposed as a tool for supply chain traceability in the mineral industry and critical success factor for this type of tracing system are discussed (Hastig and Sodhi, 2020). Despite the benefit of high traceability, blockchain applications are energy intensive, which is against the development trend of sustainability (Jiang et al., 2021; Biswas et al., 2022). This trade-off needs more studies in the future for promoting a traceable supply chain for the metal industry.
4.2 Responses on demand side
Material substitution. Price hikes of critical metals have determining influence on material substitution (Mancheri et al., 2018). With the increasing prices of raw materials, many studies have suggested developing alternative technologies as substitutions to cope with the supply chain disruptions (Olivetti et al., 2017; Alves Dias et al., 2018; IEA, 2021a). For example, the nickel manganese cobalt (NMC) chemistry has evolved from cobalt intensive to less cobalt intensive, and the annual cobalt demand in the medium and low cobalt scenarios are 21.46 and 54.15%, respectively, lower than that in the cobalt intensive chemistry (Shi, 2022). This case shows that material substitution can reduce the supply chain risks to some extent. However, the decrease of cobalt use accompanies the increase of nickel, which in turn drives up nickel supply risks. In addition, due to the expected massive deployment of low-carbon technologies, supply strains are still there even with material substitution (Gourley et al., 2020).
Hedging price volatility. Buyers and sellers can use long-term contracts to negotiate a stable price for a specific period and stabilize profits/costs (Mancheri et al., 2018). Besides the long-term contracts, the market players are suggested to make full use of modern financial tools, such as futures and options. Establishing long-term cooperation between suppliers and customers along the supply chains and adopting integrated operation strategies are also effective ways to hedge price volatility.
5 Conclusion
To better address the supply chain challenges of critical metals in the era of frequent disturbance, this article reviews the studies on the sources, propagation, and responses of supply chain risks of critical metals. Based on the literature review on these three aspects, the following issues are proposed to be further studied in the future.
Firstly, to better identify and assess the risks in the criticality assessment of metals, there are more studies to be conducted in a new holistic framework of supply chain risks. This new framework should not only focus on the specific risks in the upstream, middle-stream, and downstream but also on general risks along the whole supply chain. Besides this conceptual framework, building a comprehensive database is required for this type of risk assessment. As for the potential direction of empirical studies, scholars could perform a holistic risk assessment for a particular metal, cobalt, for example, or they could carry out a holistic assessment for joint-metals, cobalt–lithium–nickel, for instance, or do comparison studies of risk assessments and thus better understand the criticality of the corresponding metals. The material flow analysis, multiregional input–output model, systemic dynamics, and complex network theory are potential tools to address these issues.
Secondly, the propagation of trade-related risks and price volatility are intensively examined, and we have a comprehensive understanding of how the risks propagate in a single-layer network. Nonetheless, there is still a lack of enough understanding about the supply chain vulnerability caused by the interdependences between different stages of the supply chain. In addition, the vertical transmissions of price risk, which include forward vertical transmission, backward transmission, and bidirectional transmission, should be further explored. Multiplex network could be a useful tool for these explorations. Interdisciplinary studies that combine the multiplex network, econometric modeling, multiregional analysis, etc., also deserve a try since they may provide flexible and comprehensive solutions to these issues in the future.
Thirdly, to respond effectively to the supply chain challenges, there are short-term measures like stockpiling and price hedging and long-term approaches like recycling and circular strategy. In the future, more studies should be done to investigate supply chain diversification rather than diversification of one stage. In addition, more quantitative models those include the price factor, recycling, and technological breakthrough are worthy of attention due to rapid clean energy transition and the nature of metal scarcity. As for the recycling of critical metals, studies on the cost of recycling and its influencing factors are few but deserve academic investigations. Supply chain traceability supported by blockchain has also become an emerging frontier topic. It should be noted that there are trade-offs in every response approach, which deserves more studies in the future.
Author contributions
XS: conceptualization, methodology, formal analysis, writing—original draft and revision.
Funding
I’m thankful for the financial support from the National Natural Science Foundation of China (Grants No. 41901246).
Acknowledgments
The author is thankful to Prof. Xiangyun Gao at China University of Geosciences (Beijing) for his opinions and suggestions on the topics reviewed, to FZ for his handling of the article, and to the reviewers for their constructive comments that improved this article substantially.
Conflict of interest
The author declares that the research was conducted in the absence of any commercial or financial relationships that could be construed as a potential conflict of interest.
Publisher’s note
All claims expressed in this article are solely those of the authors and do not necessarily represent those of their affiliated organizations, or those of the publisher, the editors and the reviewers. Any product that may be evaluated in this article, or claim that may be made by its manufacturer, is not guaranteed or endorsed by the publisher.
Supplementary material
The Supplementary Material for this article can be found online at: https://www.frontiersin.org/articles/10.3389/fenrg.2022.957884/full#supplementary-material
References
Achzet, B., and Helbig, C. (2013). How to evaluate raw material supply risks—an overview. Resour. Policy 38 (4), 435–447. doi:10.1016/j.resourpol.2013.06.003
Althaf, S., and Babbitt, C. W. (2021). Disruption risks to material supply chains in the electronics sector. Resour. Conserv. Recycl. 167, 105248. doi:10.1016/j.resconrec.2020.105248
Alves Dias, P., Blagoeva, D., Pavel, C., and Arvanitidis, N. (2018). Cobalt: Demand-Supply balances in the transition to electric mobility. Luxembourg: EUR-Scientific and Technical Research Reports Publications Office of the European Union European Commission, Joint Research Centre. doi:10.2760/97710
An, H., and Li, H. (2022). Theory and research advances in whole industrial chain of strategic mineral resources (in Chinese). Resour. Industries 24 (1), 8–14. doi:10.13776/j.cnki.resourcesindustries.20211221.007
An, H., Zhong, W., Chen, Y., Li, H., and Gao, X. (2014). Features and evolution of international crude oil trade relationships: a trading-based network analysis. Energy 74, 254–259. doi:10.1016/j.energy.2014.06.095
An, S., Gao, X., An, H., Liu, S., Sun, Q., and Jia, N. (2020). Dynamic volatility spillovers among bulk mineral commodities: a network method. Resour. Policy 66, 101613. doi:10.1016/j.resourpol.2020.101613
Ash, J., and Newth, D. (2007). Optimizing complex networks for resilience against cascading failure. Phys. A Stat. Mech. Appl. 380, 673–683. doi:10.1016/j.physa.2006.12.058
Baars, J., Domenech, T., Bleischwitz, R., Melin, H. E., and Heidrich, O. (2021). Circular economy strategies for electric vehicle batteries reduce reliance on raw materials. Nat. Sustain. 4 (1), 71–79. doi:10.1038/s41893-020-00607-0
Barabasi, A. L., and Albert, R. (1999). Emergence of scaling in random networks. Science 286 (5439), 509–512. doi:10.1126/science.286.5439.509
Basole, R. C., and Bellamy, M. A. (2014). Supply network structure, visibility, and risk diffusion: a computational approach. Decis. Sci. 45 (4), 753–789. doi:10.1111/deci.12099
Biswas, D., Jalali, H., Ansaripoor, A. H., and Giovanni, P. D. (2022). Traceability vs. Sustainability in supply chains: the implications of blockchain. Eur. J. Oper. Res. doi:10.1016/j.ejor.2022.05.034
Blengini, G., Latunussa, C., Eynard, U., Matos, C., Georgitzikis, K., Pavel, C., et al. (2020). Study on the EU’s list of critical raw materials (2020). Luxembourg: Publications Office of the European Union. doi:10.2873/92480
Bouri, E., Kanjilal, K., Ghosh, S., Roubaud, D., and Saeed, T. (2021). Rare earth and allied sectors in stock markets: extreme dependence of return and volatility. Appl. Econ. 53 (49), 5710–5730. doi:10.1080/00036846.2021.1927971
Calvo, G., Valero, A., and Valero, A. (2018). Thermodynamic approach to evaluate the criticality of raw materials and its application through a material flow analysis in Europe. J. Ind. Ecol. 22 (4), 839–852. doi:10.1111/jiec.12624
Chen, J., Liang, Z., Ding, Q., and Liu, Z. (2022). Extreme spillovers among fossil energy, clean energy, and metals markets: evidence from A quantile-based analysis. Energy Econ. 107, 105880. doi:10.1016/j.eneco.2022.105880
Chen, Z., Zhang, L., and Xu, Z. (2019). Tracking and quantifying the cobalt flows in mainland China during 1994–2016: insights into use, trade and prospective demand. Sci. Total Environ. 672, 752–762. doi:10.1016/j.scitotenv.2019.02.411
Dente, S., and Hashimoto, S. (2020). COVID-19: a pandemic with positive and negative outcomes on resource and waste flows and stocks. Resour. Conserv. Recycl. 161, 104979. doi:10.1016/j.resconrec.2020.104979
Di, J., Wen, Z., Jiang, M., and Miatto, A. (2022). Patterns and features of embodied environmental flow networks in the international trade of metal resources: a study of aluminum. Resour. Policy 77, 102767. doi:10.1016/j.resourpol.2022.102767
Dolgui, A., Ivanov, D., and Sokolov, B. (2018). Ripple effect in the supply chain: an analysis and recent literature. Int. J. Prod. Res. 56 (1-2), 414–430. doi:10.1080/00207543.2017.1387680
Elliott, M., Golub, B., and Jackson, M. (2014). Financial networks and contagion. Am. Econ. Rev. 104 (10), 3115–3153. doi:10.1257/aer.104.10.3115
European Commission (2017). Methodology for establishing the EU list of critical raw materials: Guidelines. Luxembourg: Publications Office. Available at: https://data.europa.eu/doi/10.2873/769526 (Accessed 05 23, 2022).
Forrester, J. W. (1994). System dynamics, systems thinking, and soft OR. Syst. Dyn. Rev. 10 (2-3), 245–256. doi:10.1002/sdr.4260100211
Fu, X., Polli, A., and Olivetti, E. (2019). High-resolution Insight into materials criticality: quantifying risk for by-product metals from primary production. J. Ind. Ecol. 23 (2), 452–465. doi:10.1111/jiec.12757
Gai, P., and Kapadia, S. (2010). Contagion in financial networks. Proc. R. Soc. A 466 (2120), 2401–2423. doi:10.1098/rspa.2009.0410
Gao, J., Li, D., and Havlin, S. (2014). From A single network to A network of networks. Natl. Sci. Rev. 1 (3), 346–356. doi:10.1093/nsr/nwu020
Gourley, S., Or, T., and Chen, Z. (2020). Breaking free from cobalt reliance in lithium-ion batteries. iScience 23 (9), 101505. doi:10.1016/j.isci.2020.101505
Graedel, T., Barr, R., Chandler, C., Chase, T., Choi, J., Christoffersen, L., et al. (2012). Methodology of metal criticality determination. Environ. Sci. Technol. 46 (2), 1063–1070. doi:10.1021/es203534z
Graedel, T., Harper, E., Nassar, N., Nuss, P., and Reck, B. (2015). Criticality of metals and metalloids. Proc. Natl. Acad. Sci. U. S. A. 112 (14), 4257–4262. doi:10.1073/pnas.1500415112
Guan, D., Wang, D., Hallegatte, S., Davis, S., Huo, J., Li, S., et al. (2020). Global supply-chain effects of covid-19 control measures. Nat. Hum. Behav. 4 (6), 577–587. doi:10.1038/s41562-020-0896-8
Guo, S., Li, H., An, H., Sun, Q., Hao, X., Liu, Y., et al. (2019). Steel product prices transmission activities in the midstream industrial chain and global markets. Resour. Policy 60, 56–71. doi:10.1016/j.resourpol.2018.11.014
Hammoudeh, S., Mokni, K., Ben-Salha, O., and Ajmi, A. (2021). Distributional predictability between oil prices and renewable energy stocks: is there a role for the covid-19 pandemic? Energy Econ. 103, 105512. doi:10.1016/j.eneco.2021.105512
Hao, X., An, H., Sun, X., and Zhong, W. (2018). The import competition relationship and intensity in the international iron ore trade: from network perspective. Resour. Policy 57, 45–54. doi:10.1016/j.resourpol.2018.01.005
Hastig, G., and Sodhi, M. (2020). Blockchain for supply chain traceability: business requirements and critical success factors. Prod. Oper. Manag. 29 (4), 935–954. doi:10.1111/poms.13147
He, P., Feng, H., Hu, G., Hewage, K., Achari, G., Wang, C., et al. (2020). Life cycle cost analysis for recycling high-tech minerals from waste mobile phones in China. J. Clean. Prod. 251, 119498. doi:10.1016/j.jclepro.2019.119498
Helbig, C., Schrijvers, D., and Hool, A. (2021). Selecting and prioritizing material resources by criticality assessments. One Earth 4 (3), 339–345. doi:10.1016/j.oneear.2021.02.006
Hu, X., Wang, C., Lim, M., and Chen, W. (2020). Characteristics of the global copper raw materials and scrap trade systems and the policy impacts of China's import ban. Ecol. Econ. 172, 106626. doi:10.1016/j.ecolecon.2020.106626
Hu, X., Wang, C., Zhu, X., Yao, C., and Ghadimi, P. (2021). Trade structure and risk transmission in the international automotive Li-ion batteries trade. Resour. Conserv. Recycl. 170, 105591. doi:10.1016/j.resconrec.2021.105591
Hua, Y., Zhou, S., Huang, Y., Liu, X., Ling, H., Zhou, X., et al. (2020). Sustainable value chain of retired lithium-ion batteries for electric vehicles. J. Power Sources 478, 228753. doi:10.1016/j.jpowsour.2020.228753
Ibn-Mohammed, T., Mustapha, K., Godsell, J., Adamu, Z., Babatunde, K., Akintade, D., et al. (2021). A critical analysis of the impacts of covid-19 on the global economy and ecosystems and opportunities for circular economy strategies. Resour. Conserv. Recycl. 164, 105169. doi:10.1016/j.resconrec.2020.105169
IEA (2020). Clean energy progress after the covid-19 crisis will need reliable supplies of critical minerals. Paris, France. Available at: https://www.iea.org/articles/clean-energy-progress-after-the-covid-19-crisis-will-need-reliable-supplies-of-critical-minerals (Accessed May 23, 2022).
IEA (2021a). The role of critical minerals in clean energy transitions. Paris, France. Available at: https://www.iea.org/reports/the-role-of-critical-minerals-in-clean-energy-transitions (Accessed May 22, 2022).
IEA (2021b). World energy outlook. Paris, France. Available at: https://www.iea.org/reports/world-energy-outlook-2021 (Accessed May 22, 2022).
IEA (2022). Critical minerals threaten a decades-long trend of cost declines for clean energy technologies. Paris, France. Available at: https://www.iea.org/commentaries/critical-minerals-threaten-a-decades-long-trend-of-cost-declines-for-clean-energy-technologies (Accessed May 22, 2022).
Jackson, M., and Pernoud, A. (2021). Systemic risk in financial networks: a survey. Annu. Rev. Econ. 13, 171–202. doi:10.1146/annurev-economics-083120-111540
Jia, Y., Ding, C., and Dong, Z. (2021). Transmission mechanism of stock price fluctuation in the rare earth industry chain. Sustainability 13, 12913. doi:10.3390/su132212913
Jiang, S., Li, Y., Lu, Q., Hong, Y., Guan, D., Xiong, Y., et al. (2021). Policy assessments for the carbon emission flows and sustainability of bitcoin blockchain operation in China. Nat. Commun. 12 (1), 1938. doi:10.1038/s41467-021-22256-3
Klimek, P., Obersteiner, M., and Thurner, S. (2015). Systemic trade risk of critical resources. Sci. Adv. 1 (10), e1500522. doi:10.1126/sciadv.1500522
Kuong, I., Li, J., Zhang, J., and Zeng, X. (2019). Estimating the evolution of urban mining resources in Hong Kong, up to the year 2050. Environ. Sci. Technol. 53 (3), 1394–1403. doi:10.1021/acs.est.8b04063
Lee, K., Yang, J., Kim, G., Lee, J., Goh, K., and Kim, I. (2011). Impact of the topology of global macroeconomic network on the spreading of economic crises. PLoS One 6 (3), e18443. doi:10.1371/journal.pone.0018443
Li, Y., Gao, X., An, S., Zheng, H., and Wu, T. (2021). Network approach to the dynamic transformation characteristics of the joint impacts of gold and oil on copper. Resour. Policy 70, 101967. doi:10.1016/j.resourpol.2020.101967
Liu, Y., Li, H., Guan, J., Liu, X., and Qi, Y. (2019). The role of the world's major steel markets in price spillover networks: an analysis based on complex network motifs. J. Econ. Interact. Coord. 14 (4), 697–720. doi:10.1007/s11403-019-00261-6
Mancheri, N., Sprecher, B., Deetman, S., Young, S., Bleischwitz, R., Dong, L., et al. (2018). Resilience in the tantalum supply chain. Resour. Conserv. Recycl. 129, 56–69. doi:10.1016/j.resconrec.2017.10.018
Mao, J., Ye, C., Zhang, S., Xie, F., Zeng, R., Davey, K., et al. (2022). Toward practical lithium-ion battery recycling: adding value, tackling circularity and recycling-oriented design. Energy Environ. Sci. 15, 2732–2752. doi:10.1039/D2EE00162D
Miao, Y., Liu, L., Zhang, Y., Tan, Q., and Li, J. (2022). An overview of global power lithium-ion batteries and associated critical metal recycling. J. Hazard. Mat. 425, 127900. doi:10.1016/j.jhazmat.2021.127900
Muller, E., Hilty, L. M., Widmer, R., Schluep, M., and Faulstich, M. (2014). Modeling metal stocks and flows: a review of dynamic material flow analysis methods. Environ. Sci. Technol. 48 (4), 2102–2113. doi:10.1021/es403506a
Nansai, K., Nakajima, K., Kagawa, S., Kondo, Y., Shigetomi, Y., Suh, S., et al. (2015). Global mining risk footprint of critical metals necessary for low-carbon technologies: the case of neodymium, cobalt, and platinum in Japan. Environ. Sci. Technol. 49 (4), 2022–2031. doi:10.1021/es504255r
Nansai, K., Nakajima, K., Suh, S., Kagawa, S., Kondo, Y., Takayanagi, W., et al. (2017). The role of primary processing in the supply risks of critical metals. Econ. Syst. Res. 29 (3), 335–356. doi:10.1080/09535314.2017.1295923
Newman, M. (2002). Spread of epidemic disease on networks. Phys. Rev. E 66 (1), 016128. doi:10.1103/PhysRevE.66.016128
Newman, M. (2003). The structure and function of complex networks. SIAM Rev. Soc. Ind. Appl. Math. 45 (2), 167–256. doi:10.1137/S003614450342480
Niu, H. (2021). Correlations between crude oil and stocks prices of renewable energy and technology companies: a multiscale time-dependent analysis. Energy 221, 119800. doi:10.1016/j.energy.2021.119800
Nkulu, C., Casas, L., Haufroid, V., De Putter, T., Saenen, N., Kayembe-Kitenge, T., et al. (2018). Sustainability of artisanal mining of cobalt in DR Congo. Nat. Sustain. 1 (9), 495–504. doi:10.1038/s41893-018-0139-4
Nuss, P., and Blengini, G. (2018). Towards better monitoring of technology critical elements in Europe: coupling of natural and anthropogenic cycles. Sci. Total Environ. 613-614, 569–578. doi:10.1016/j.scitotenv.2017.09.117
Ojha, R., Ghadge, A., Tiwari, M. K., and Bititci, U. S. (2018). Bayesian network modelling for supply chain risk propagation. Int. J. Prod. Res. 56 (17), 5795–5819. doi:10.1080/00207543.2018.1467059
Olivetti, E., Ceder, G., Gaustad, G., and Fu, X. (2017). Lithium-ion battery supply chain considerations: analysis of potential bottlenecks in critical metals. Joule 1 (2), 229–243. doi:10.1016/j.joule.2017.08.019
Omar, I., Debe, M., Jayaraman, R., Salah, K., Omar, M., Arshad, J., et al. (2022). Blockchain-based supply chain traceability for COVID-19 personal protective equipment. Comput. Ind. Eng. 167, 107995. doi:10.1016/j.cie.2022.107995
Paltalidis, N., Gounopoulos, D., Kizys, R., and Koutelidakis, Y. (2015). Transmission channels of systemic risk and contagion in the European financial network. J. Bank. Finance 61, S36–S52. doi:10.1016/j.jbankfin.2015.03.021
Pastor-Satorras, R., Castellano, C., Van Mieghem, P., and Vespignani, A. (2015). Epidemic processes in complex networks. Rev. Mod. Phys. 87 (3), 925–979. doi:10.1103/RevModPhys.87.925
Ponomarov, S., and Holcomb, M. (2009). Understanding the concept of supply chain resilience. Int. J. Logist. Manag. 20 (1), 124–143. doi:10.1108/09574090910954873
Qi, Y., Li, H., Liu, Y., Feng, S., Li, Y., and Guo, S. (2020). Granger causality transmission mechanism of steel product prices under multiple scales—the industrial chain perspective. Resour. Policy 67, 101674. doi:10.1016/j.resourpol.2020.101674
Rasmussen, K., Wenzel, H., Bangs, C., Petavratzi, E., and Liu, G. (2019). Platinum demand and potential bottlenecks in the global green transition: a dynamic material flow analysis. Environ. Sci. Technol. 53 (19), 11541–11551. doi:10.1021/acs.est.9b01912
Reboredo, J., and Ugolini, A. (2020). Price spillovers between rare earth stocks and financial markets. Resour. Policy 66, 101647. doi:10.1016/j.resourpol.2020.101647
Scheibe, K., and Blackhurst, J. (2018). Supply chain disruption propagation: a systemic risk and normal accident theory perspective. Int. J. Prod. Res. 56 (1-2), 43–59. doi:10.1080/00207543.2017.1355123
Schrijvers, D., Hool, A., Blengini, G., Chen, W.-Q., Dewulf, J., Eggert, R., et al. (2020). A review of methods and data to determine raw material criticality. Resour. Conserv. Recycl. 155, 104617. doi:10.1016/j.resconrec.2019.104617
Shahjalal, M., Roy, P., Shams, T., Fly, A., Chowdhury, J., Ahmed, M., et al. (2022). A review on second-life of Li-ion batteries: prospects, challenges, and issues. Energy 241, 122881. doi:10.1016/j.energy.2021.122881
Shammugam, S., Rathgeber, A., and Schlegl, T. (2019). Causality between metal prices: is joint consumption a more important determinant than joint production of main and by-product metals? Resour. Policy 61, 49–66. doi:10.1016/j.resourpol.2019.01.010
Shao, L., Kou, W., and Zhang, H. (2022). The evolution of the global cobalt and lithium trade pattern and the impacts of the low-cobalt technology of lithium batteries based on multiplex network. Resour. Policy 76, 102550. doi:10.1016/j.resourpol.2022.102550
Shao, L., Zhang, H., Chen, J., and Zhu, X. (2021). Effect of oil price uncertainty on clean energy metal stocks in China: evidence from a nonparametric causality-in-quantiles approach. Int. Rev. Econ. Financ. 73, 407–419. doi:10.1016/j.iref.2021.01.009
Shao, L., and Zhang, H. (2020). The impact of oil price on the clean energy metal prices: a multi-scale perspective. Resour. Policy 68, 101730. doi:10.1016/j.resourpol.2020.101730
Shi, Q. (2022). Cobalt demand for automotive electrification in China: scenario analysis based on the bass model. Front. Energy Res. 10, 903465. doi:10.3389/fenrg.2022.903465
Shi, Q., and Sun, X. (2020). A scientometric review of digital currency and electronic payment research: a network perspective. Complexity 2020, 8876017. doi:10.1155/2020/8876017
Shi, Q., Sun, X., Xu, M., and Wang, M. (2022). The multiplex network structure of global cobalt industry chain. Resour. Policy 76, 102555. doi:10.1016/j.resourpol.2022.102555
Song, Y., Bouri, E., Ghosh, S., and Kanjilal, K. (2021). Rare earth and financial markets: dynamics of return and volatility connectedness around the covid-19 outbreak. Resour. Policy 74, 102379. doi:10.1016/j.resourpol.2021.102379
Sprecher, B., Daigo, I., Murakami, S., Kleijn, R., Vos, M., Kramer, G., et al. (2015). Framework for resilience in material supply chains, with a case study from the 2010 rare earth crisis. Environ. Sci. Technol. 49 (11), 6740–6750. doi:10.1021/acs.est.5b00206
Sprecher, B., Daigo, I., Spekkink, W., Vos, M., Kleijn, R., Murakami, S., et al. (2017). Novel indicators for the quantification of resilience in critical material supply chains, with a 2010 rare earth crisis case study. Environ. Sci. Technol. 51 (7), 3860–3870. doi:10.1021/acs.est.6b05751
Sun, Q., An, H., Gao, X., Guo, S., Wang, Z., Liu, S., et al. (2019b). Effects of crude oil shocks on the PPI system based on variance decomposition network analysis. Energy 189, 116378. doi:10.1016/j.energy.2019.116378
Sun, Q., Gao, X., Wen, S., Chen, Z., and Hao, X. (2018). The transmission of fluctuation among price indices based on granger causality network. Phys. A Stat. Mech. its Appl. 506, 36–49. doi:10.1016/j.physa.2018.04.055
Sun, X., Hao, H., Liu, Z., and Zhao, F. (2020). Insights into the global flow pattern of manganese. Resour. Policy 65, 101578. doi:10.1016/j.resourpol.2019.101578
Sun, X., Hao, H., Liu, Z., Zhao, F., and Song, J. (2019a). Tracing global cobalt flow: 1995–2015. Resour. Conserv. Recycl. 149, 45–55. doi:10.1016/j.resconrec.2019.05.009
Sun, X., Shi, Q., and Hao, X. (2022). Supply crisis propagation in the global cobalt trade network. Resour. Conserv. Recycl. 179, 106035. doi:10.1016/j.resconrec.2021.106035
Swain, N., and Mishra, S. (2019). A review on the recovery and separation of rare earths and transition metals from secondary resources. J. Clean. Prod. 220, 884–898. doi:10.1016/j.jclepro.2019.02.094
Tang, C., Sprecher, B., Tukker, A., and Mogollón, J. M. (2021). The impact of climate policy implementation on lithium, cobalt and nickel demand: the case of the Dutch automotive sector up to 2040. Resour. Policy 74, 102351. doi:10.1016/j.resourpol.2021.102351
van den Brink, S., Kleijn, R., Sprecher, B., and Tukker, A. (2020). Identifying supply risks by mapping the cobalt supply chain. Resour. Conserv. Recycl. 156, 104743. doi:10.1016/j.resconrec.2020.104743
Wang, C., Huang, X., Hu, X., Zhao, L., Liu, C., Ghadimi, P., et al. (2021b). Trade characteristics, competition patterns and covid-19 related shock propagation in the global solar photovoltaic cell trade. Appl. Energy 290, 116744. doi:10.1016/j.apenergy.2021.116744
Wang, M., Liu, P., Gu, Z., Cheng, H., and Li, X. (2019). A scientometric review of resource recycling industry. Int. J. Environ. Res. Public Health 16 (23), 4654. doi:10.3390/ijerph16234654
Wang, P., Wang, Q., Han, R., Tang, L., Liu, Y., Cai, W., et al. (2021a). Nexus between low-carbon energy and critical metals: literature review and implications (in Chinese). Resour. Sci. 43 (4), 669–681. doi:10.18402/resci.2021.04.03
Wang, X., Li, H., Yao, H., Zhu, D., and Liu, N. (2018). Simulation analysis of the spread of A supply crisis based on the global natural graphite trade network. Resour. Policy 59, 200–209. doi:10.1016/j.resourpol.2018.07.002
Wang, X., Wang, A., and Zhu, D. (2022). Simulation analysis of supply crisis propagation based on global nickel industry chain. Front. Energy Res. 10, 919510. doi:10.3389/fenrg.2022.919510
Wang, Y., and Ge, J. (2020). Potential of urban cobalt mines in China: an estimation of dynamic material flow from 2007 to 2016. Resour. Conserv. Recycl. 161, 104955. doi:10.1016/j.resconrec.2020.104955
Watari, T., Nansai, K., and Nakajima, K. (2020). Review of critical metal dynamics to 2050 for 48 elements. Resour. Conserv. Recycl. 155, 104669. doi:10.1016/j.resconrec.2019.104669
Wu, C., Gao, X., Xi, X., Zhao, Y., and Li, Y. (2021). The stability optimization of the international lithium trade. Resour. Policy 74, 102336. doi:10.1016/j.resourpol.2021.102336
Xia, Y., Fan, J., and Hill, D. (2010). Cascading failure in watts-strogatz small-world networks. Phys. A Stat. Mech. its Appl. 389 (6), 1281–1285. doi:10.1016/j.physa.2009.11.037
Xiarchos, I., and Fletcher, J. (2009). Price and volatility transmission between primary and scrap metal markets. Resour. Conserv. Recycl. 53 (12), 664–673. doi:10.1016/j.resconrec.2009.04.020
Xu, C., Dai, Q., Gaines, L., Hu, M., Tukker, A., and Steubing, B. (2020). Future material demand for automotive lithium-based batteries. Commun. Mat. 1, 99. doi:10.1038/s43246-020-00095-x
Yan, W., Cao, H., Zhang, Y., Ning, P., Song, Q., Yang, J., et al. (2020). Rethinking Chinese supply resilience of critical metals in lithium-ion batteries. J. Clean. Prod. 256, 120719. doi:10.1016/j.jclepro.2020.120719
Yan, W., Wang, Z., Cao, H., Zhang, Y., and Sun, Z. (2021). Criticality assessment of metal resources in China. iScience 24 (6), 102524. doi:10.1016/j.isci.2021.102524
Zeng, X., and Li, J. (2021). Emerging anthropogenic circularity science: principles, practices, and challenges. iScience 24 (3), 102237. doi:10.1016/j.isci.2021.102237
Zeng, X., and Li, J. (2015). On the sustainability of cobalt utilization in China. Resour. Conserv. Recycl. 104, 12–18. doi:10.1016/j.resconrec.2015.09.014
Zhai, M., Wu, F., Hu, R., Jiang, S., Li, W., Wang, R., et al. (2019). Critical metal mineral resources: current research status and scientific issues (in Chinese). Bull. Natl. Nat. Sci. Found. China 33 (2), 106–111. doi:10.16262/j.cnki.1000-8217.2019.02.002
Zhao, Y., Gao, X., Sun, X., Si, J., Sun, X., and Wu, T. (2022). The impact of structural changes of trade dependence network on cobalt price from the perspective of industrial chain (in Chinese). Resour. Sci. 44 (5). doi:10.18402/resci.2022.05.00
Zhao, Z., Chen, D., Wang, L., and Han, C. (2018). Credit risk diffusion in supply chain finance: a complex networks perspective. Sustainability 10 (12), 4608. doi:10.3390/su10124608
Zheng, B., Zhang, Y., and Chen, Y. (2021). Asymmetric connectedness and dynamic spillovers between renewable energy and rare earth markets in China: evidence from firms' high-frequency data. Resour. Policy 71, 101996. doi:10.1016/j.resourpol.2021.101996
Zhong, W., An, H., Gao, X., and Sun, X. (2014). The evolution of communities in the international oil trade network. Phys. A Stat. Mech. its Appl. 413, 42–52. doi:10.1016/j.physa.2014.06.055
Zhou, M., Huang, J., and Chen, J. (2022). Time and frequency spillovers between political risk and the stock returns of China's rare earths. Resour. Policy 75, 102464. doi:10.1016/j.resourpol.2021.102464
Keywords: supply chain, risk, clean energy, network, propagation, critical metals
Citation: Sun X (2022) Supply chain risks of critical metals: Sources, propagation, and responses. Front. Energy Res. 10:957884. doi: 10.3389/fenrg.2022.957884
Received: 31 May 2022; Accepted: 12 July 2022;
Published: 11 August 2022.
Edited by:
Fu Zhao, Purdue University, United StatesReviewed by:
Yanfang Zhang, Nanjing University of Aeronautics and Astronautics, ChinaJinghan Di, Renmin University of China, China
Copyright © 2022 Sun. This is an open-access article distributed under the terms of the Creative Commons Attribution License (CC BY). The use, distribution or reproduction in other forums is permitted, provided the original author(s) and the copyright owner(s) are credited and that the original publication in this journal is cited, in accordance with accepted academic practice. No use, distribution or reproduction is permitted which does not comply with these terms.
*Correspondence: Xiaoqi Sun, c3VueGlhb3FpQHN6dS5lZHUuY24=