- 1Navigation College, Dalian Maritime University, Dalian, China
- 2Research Institute of Intelligent Networks, Zhejiang Lab, Hangzhou, China
At the current stage of a global energy shortage, the optimization of marine energy consumption has received attention. In order to save marine energy consumption and reduce pollution, research on low-energy-consumption Unmanned Surface Vessels (USVs) and their green and low-carbon cooperative operation methods needs to be paid attention to. Due to the ever-changing environment of USVs in actual sea conditions and the increasingly complex tasks, the cooperation of a single swarm of USVs is incompetent. First, in order to cope with larger-scale and complex tasks, we construct the multilateral cooperative control system for USVs with topology scalability under a polymorphic network and propose the multilateral distributed control protocol. Second, in order to reduce the energy consumption of the cooperative operation of USVs, the low-energy-consumption and low-carbon USVs are selected with the lowest EEOI index as the optimization goal, and based on ad hoc on-demand distance vector routing (AODV), the optimal path between USVs is obtained. Then, the swarms are grouped by External Equitable Partition (EEP), and the green autonomous cooperative formation strategy is proposed. Finally, the simulation examples are used to verify the effectiveness of the method proposed in this article.
1 Introduction
Due to the lack of global energy and serious marine pollution, the pressure on energy conservation and emission reduction is unprecedented, and it is necessary to strengthen the work of saving energy, reducing consumption, and reducing the pollution of ships (Źarko et al., 2021; Yushuai et al., 2021). Under such environmental conditions, it has become the general trend to vigorously develop and use green USVs.
USV is an intelligent system that is unmanned (Zhenyu and Ge, 2018), green, and energy-saving (Bertaska et al., 2015) and navigates on the water surface by remote control or autonomous means (Zheng et al., 2021; Sharma et al., 2012). It has the characteristics of low cost, small size, and high controllability (Rui et al., 2017). A single USV has major limitations in its operations—a limited mission area, a lack of system autonomy, and a lack of sufficient fault tolerance. With the development of the marine economy and technology, mission-oriented USVs emerge as the times require, which consist of multiple USVs and operate in a swarm mode (Joseph et al., 2005). In the process of driving or when performing a certain task, maintaining a certain formation can greatly improve the work efficiency of task completion (Lewis and Tan, 1997) and has high fault tolerance and robustness (Zhengfeng et al., 2021). The USV control technology has continuously made new research progress. In the process of the development, it gradually established a USV autonomous cooperative control theory that meets the clustered marine operation tasks under the complex environment, with the characteristics of high reliability, high precision, strong adaptation, anti-interference, autonomous cooperation, low carbon, low-energy consumption, and the capabilities of fast swarm task response, cluster formation reconstruction, and transformation (Yan et al., 2021; Zhouhua et al., 2021). As a typical agent, the USVs’ cooperative control can be researched and designed based on a multi-agent system (MAS). The agents in the system use appropriate communication routing protocols and distributed cooperative control methods to make their states consistent (Garey and David, 1978; Durfee and Corkill, 1989), to solve the resource allocation problem (Qiaoyun et al., 2007; Chou and Abraham, 1982), the task division problem (Lei and Qi, 2002), and the communication problem between agents (Smith, 1980).
Due to the constantly changing environment of USVs under actual sea conditions and the increasingly complex tasks they face, the cooperation of a group of USVs is often incompetent under some special conditions, requiring USVs with different functions under a variety of different morphic networks to jointly perform tasks. For example, when performing a maritime search, rescue, and salvage tasks, the USV formation responsible for communication with the ground, salvage, and transportation needs to work together, though these three kinds of USVs, each with their own mission objectives, use different control algorithms under different communication networks. The key to distributed cooperative control is the information interaction between neighbor USVs. However, multiple groups of USVs are in different unilateral communication networks. After regrouping, they cannot directly exchange information and thus cannot achieve cooperative control under the traditional communication network. Therefore, the polymorphic network architecture has been proposed, of which the basic idea is to design an open network architecture with the characteristics of a “reconfigurable network” based on a fully dimensional definable platform (Julong et al., 2014), to meet the needs of the diversified development of the multilateral network (Jiangxing and Yuxiang, 2021; Li et al., 2019) and have achieved the goals of multilateral co-management, equality and openness, and high performance (Hui et al., 2019). The polymorphic network development paradigm adopts the idea of separating the network from the supporting environment and combines various existing or future networks (including business or service or management functions) in a modal form, dynamically loading and running a definable network support environment on the full dimension. Realize the symbiotic coexistence, independent evolution, and transformation of multiple network modalities in the same technical and physical environment (Jiangxing and Kaizhi, 2022) according to the modal customized software and hardware configuration, message format, routing protocol, exchange mode, forwarding logic, business characteristics, operation and maintenance specifications, security policies, etc. To sum up, the polymorphic network provides a channel for the communication required by the USVs under multiple unilateral communication networks. Therefore, in the face of increasingly complex working conditions and mission requirements, when designing the communication network between the different groups of USVs, we cannot borrow from the design concept of the traditional unilateral communication network because these USVs have the characteristics of independence, autonomy, cooperation, distribution, and heterogeneity. Also, it is necessary to build a multilateral distributed cooperative control system for USVs under a polymorphic network. The meaning of multilateral distributed cooperative control refers to the task-oriented regrouping of multiple groups of USVs under the polymorphic network by constructing a reasonable communication topology and designing a suitable routing protocol and distributed control protocol, in which case all USVs in each group can maintain communication with each other after regrouping, and the state eventually tends to be consistent. In this way, the cooperative control of multiple groups of USVs with different functions is realized. When designing a new communication network, we should consider the characteristics of distributed, multilateral co-management, plug-and-play, equal openness, scalable structure, and at the same time meeting the control requirements of each group of USVs. It is in line with the characteristics of polymorphic networks, so the future cooperative control of USVs will rely on polymorphic networks. Therefore, the polymorphic network is the basis for realizing the cooperative control of green USVs.
To develop green shipping, reduce marine pollution, and reduce carbon emissions, driven by specific tasks, how to build a low-carbon and low-energy-consumption USVs under the polymorphic network to efficiently and greenly achieve multilateral cooperative control is an issue that requires further research. Green and energy-saving USVs should be selected to achieve energy saving and emission reduction. The energy utilization efficiency of USVs can be evaluated by EEOI (the Ship Energy Efficiency Operational Indicator), which is an evaluation obtained by comprehensively considering the ship’s cargo capacity, sailing distance, fuel consumption, greenhouse gas emissions, and other factors (Chon et al., 2019). The smaller the EEOI index, the higher the energy efficiency of the ship. The reduction of EEOI can be achieved by optimizing the speed of the ship, the route, rational stowage, recovering the waste heat from the main engine exhaust gas, and using clean energy with low CO2 emissions (Jingmin, 2016). Based on the EEOI index, the energy efficiency index of the selectable USVs can be quantified, and the low-carbon and low-energy-consumption USVs can be selected. For the selected green USVs under the polymorphic network, although the information exchange between multiple groups of USVs can be realized, the temporary network cannot guarantee the realization of multilateral distributed cooperative control. Therefore, there are two key issues that need to be studied: one is how to analyze and reorganize the USVs originally under a unilateral communication network considering some suitable conditions, and the other one is how to design a reasonable ad hoc network topology and realize multilateral distributed cooperative control based on multi-agent multi-consensus theory (Olfati-Saber et al., 2007; Gambuzza and Mattia, 2019). The ad hoc network of the USVs adopts a distributed structure, and each node in the network has an equal status and makes independent decisions (Scott, 2013). Also, these nodes form the network in a self-organization manner (Scott et al., 2003), which makes the network have strong stability, openness, and invulnerability (Xueli et al., 2017; Yang et al., 2021). Adopting the AODV protocol is beneficial to save energy when USV exchange information (Bhagyalakshmi and Dogra, 2018; Mohsen and Naif, 2019) and obtain the optimal path for inter-swarm communication (Yuan et al., 2007; Qiang et al., 2011). Therefore, how to use the fewest routes to reorganize the selected low-carbon and low-energy-consumption USVs, build the topology of the ad hoc network of multiple groups of USVs in a green and low-energy-consumption manner, and design a multilateral distributed cooperative control strategy is a concerning issue.
In order to solve the aforementioned problems, under the polymorphic network, a green autonomous cooperative formation strategy for multiple groups of USVs is proposed to achieve low-carbon and low-energy-consumption multilateral cooperative control, which can cope with the current large-scale and complex maritime tasks, helping the shipping industry reduce pollution and carbon emission. Also, the contributions are as follows:
1) Utilize the EEOI index to quantify the energy efficiency operation index of the USVs under the optional unilateral communication network and construct a low-carbon and low-energy-consumption restructured USVs. Based on the AODV protocol of the wireless ad hoc network, the optimal network routing of the USVs is established so as to complete the low-energy-consumption networking of multiple groups of USVs.
2) According to the EEP, the multiple groups of USVs are reasonably grouped, and the communication topology reconstruction method is proposed under the polymorphic network. Also, based on the multi-agent multi-consensus algorithm, a green autonomous cooperative formation strategy of multiple groups of USVs is proposed to realize multilateral distributed cooperative control.
The rest of this article can be written as follows: in Section 2, the multilateral distributed cooperative control framework is introduced. In Section 3, we introduce some basic theoretical knowledge. In Section 4, we propose the communication topology reconstruction method and green autonomous cooperative formation strategy for multiple groups of USVs. In Section 5, we conduct an experimental simulation and analyze the data generated by the experiment.
2 Low-Energy-Consumption Multilateral Distributed Cooperative Control Framework for Low-Carbon Unmanned Surface Vessels
The low-energy-consumption multilateral distributed cooperative control framework of multiple groups of USVs is an ad hoc network system based on the polymorphic network, as shown in Figure 1. It can independently complete the networking process with the characteristics of distributed, plug-and-play, and scalable structure (Shirani et al., 2012). Multiple groups of USVs respond quickly and efficiently according to mission requirements. Under the polymorphic network, low-energy-consumption and low-carbon USVs are selected based on the EEOI index for re-networking. Meanwhile, based on AODV and EEP, the task-oriented routing protocol and communication topology of the reorganized low-energy-consumption and low-carbon USVs are designed to realize low-energy-consumption multilateral distributed cooperative control. Finally, green autonomous cooperative formation can be realized.
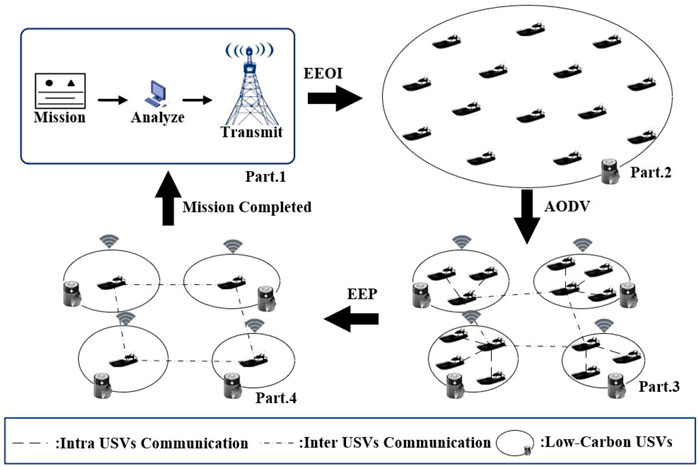
FIGURE 1. Low-energy-consumption multilateral distributed cooperative control framework for multiple USVs.
The framework is designed with the following core thinking: In the face of large-scale and complex maritime tasks, it is no longer completed by a single USV under the traditional unilateral communication network. Instead, it is realized by multiple groups of MAS composed of multiple low-carbon USVs under the polymorphic network through low-energy-consumption multilateral distributed cooperative control. When USVs perform tasks, after receiving the task sent by the base station on the shore, all agents should only rely on the information exchange between each other. Also, some agents with sufficient energy and low EEOI index are selected as virtual leaders to form a temporary MAS with their surrounding neighbors and are responsible for communicating with other agents in the MAS and with virtual leaders of other MASs. Through the appropriate routing protocol and communication topology, a low-energy-consumption multilateral distributed cooperative control system with autonomous and dynamic connection functions is established to realize green autonomous cooperative formation. When the task is completed, the MASs are eliminated, and all agents maintain the same level. With the new task generating, a new low-energy-consumption multilateral distributed cooperative control system will be formed.
The multilateral distributed cooperative control framework of multiple groups of USVs consists of two levels, namely, the task sender and the task executor. The task sender is to analyze and evaluate the task risk and difficulty level, divide the task reasonably, and send the task to USVs. The task executor, that is, the MAS corresponding to each group of USVs, can choose to accept or reject the tasks assigned by the nearby task senders through information exchange according to its own situation. When accepting tasks, it can form a cooperative control system with other MASs to achieve low-energy-consumption multilateral distributed cooperative control. The specific operation steps are as follows:
Step 1: Task sending. In the Part 1 of Figure 1. Analyze and evaluate the risks and difficulties of maritime tasks and deploy tasks to USVs through base stations on the shore.
Step 2: Task acceptance. The USVs accept the task and evaluate the energy consumption of the USV to perform the task according to the EEOI index of each USV. In the Part 2 of Figure 1 shows the green USVs selected based on the EEOI index. The green USV with low carbon and low-energy consumption is selected as the virtual leader, accepts the assigned task, and forms a MAS with the green USV with low carbon and low-energy consumption within a suitable distance in which the state of the USV can achieve consensus. The virtual leader is also responsible for establishing communication with virtual leaders of other MASs. As shown in the Part 3 of Figure 1, all virtual leaders form a temporary ad hoc network, temporarily forming a simple low-energy-consumption multilateral distributed cooperative control system. The selection principles of USVs with low carbon and low-energy consumption are as follows:
where EEOIi is the EEOI index of the i-th USV, j is the fuel type, FCj is the sailing fuel consumption, CFj is the carbon dioxide conversion coefficient, mcargo is the cargo capacity, and D is the cargo distance.
Step 3: Task execution. According to the specific requirements of the tasks accepted by the MAS composed of each USV, as shown in the Part 4 of Figure 1, the optimal routing design and structural topology design are carried out for the temporary ad hoc network of the virtual leaders of all MASs. The reconstructed ad hoc network realizes communication and achieves a multi-consistency state with the least routing and realizes low-energy-consumption multilateral distributed cooperative control to perform tasks.
Step 4: Mission complete. When a mission is completed, the cooperative control system disbands itself, the MAS of all USVs is disbanded, and the virtual leaders disconnect from communication and restore independence and maintain the same level of status as other USV agents. The entire system waits for the next task assignment, maintains the state in the Part 1 of Figure 1, and re-organizes the network according to the tasks and according to the above four steps.
The aforementioned multilateral distributed cooperative control framework of multiple groups of USVs is a kind of ad hoc network designed in the polymorphic network environment according to the actual characteristics of the application scenario. The low-carbon and low-energy-consumption USVs agents in this scene have a certain specificity in moving and performing different tasks; therefore, the constructed low-energy-consumption multilateral distributed cooperative control framework of the USVs not only inherits the characteristics of the polymorphic network and ad hoc network but also has the characteristics of green energy saving, pollution reduction, and carbon reduction. This framework can realize the green autonomous cooperative formation of multiple groups of USVs.
3 Preliminaries
3.1 Graph Theory
MAS is a loosely coupled system composed of multiple agents, which aims to describe the connection between agents in the system, reveal the information and control relationship between agents, and reflect the storage and sharing methods of information in the system and the distribution pattern of problem-solving capabilities. The network structure of the MAS system is represented by matrix
The Laplace matrix of the system is
It satisfies the relation:
3.2 Unmanned Surface Vessel Model
The dynamic equation of the considered low-carbon and low-energy-consumption USV is as follows (Alslaim et al., 2016):
where
3.3 Multi-Agent System
The USV model studied in this article is essentially an agent, and the USVs can be represented by MAS. The research on USVs is carried out by using a MAS controlled by a linear first-order integral. The state of its MAS satisfies the following dynamic equation:
where
Through the dynamic equation in Eq. 3, its discrete consistent control equation is obtained
which is
where
3.4 Mobile Ad Hoc Networks
Mobile ad hoc network (MANET) refers to a network that is temporarily composed of mobile nodes through wireless communication in which these nodes act as routers for each other to forward packets without relying on any fixed infrastructure and services. Due to the transmission coverage of mobile nodes being so limited that long-distance communication generally needs to be carried out by means of multi-hop transmission, mobile networks are also called mobile multi-hop wireless networks. The schematic diagram of the mobile ad hoc network is shown in Figure 2. Its operation is completely distributed, and the tasks related to network control and organization are all assigned to each node. Due to the independence of nodes, the networking of ad hoc networks has quite significant characteristics compared with other communication networks (Lei et al., 2011), i.e., distributed ad hoc architecture, wireless transmission technology, multi-hop routing, and mobile network topology.
4 Green Autonomous Cooperative Control Method for Multiple Groups of Low-Energy-Consumption Unmanned Surface Vessels
When multiple groups of USVs perform ad hoc networks based on polymorphic networks and perform large-scale and complex maritime tasks due to the differences in the EEOI index as well as communication distance and control algorithm of each USV in the swarm, and when designing the ad hoc network of USVs, USV with low-carbon and low-energy-consumption is first selected as the virtual leader agent according to the earlier indicators. With the virtual leader agent as the center, the low-carbon and low-energy-consumption USVs within a certain communication range form a MAS, and then multiple MASs form a low-energy-consumption multilateral distributed cooperative control system. To realize the low-energy-consumption multilateral cooperative control of multiple groups of USVs, first an ad hoc network is established between low-carbon and low-energy-consumption virtual leader agents through a suitable routing protocol, and then an appropriate topology design is carried out for the ad hoc network so that the entire cooperative control system can meet the multi-consensus requirements; eventually, the green autonomous cooperative formation of multiple groups of low-energy-consumption USVs can be realized.
4.1 Ad Hoc On-Demand Distance Vector Routing Protocol of Low-Energy-Consumption Unmanned Surface Vessels
When performing large-scale and complex tasks in the ocean, the high speed and random movement of USVs without infrastructure or supply measures as well as the changeable wind and waves at sea which affect the channel, posing challenges to the reliability of the routing and the energy of the network system. The routing refers to the process of transmitting information from the source to the destination through the network, and the routing protocol is the regulation that needs to be followed in the routing process. It is also an important mechanism to ensure that the relevant information can be effectively shared among routers. In the ad hoc network of USVs, USVs are affected by the wind and waves, and they always correct their tracks to keep sailing. Therefore, the USVs need to rebuild the topology at a relatively fast speed and transport the data to the destination in a reasonable way.
In the self-organizing network of USVs, commonly used routing protocols are divided into active routing protocols and reactive routing.
The DSDV (Destination-Sequenced Distance Vector) routing protocol is a typical active routing protocol. All USVs using this protocol will actively maintain routes where they can communicate with other nodes while moving. When the state of the USV changes, DSDV will update its state in time. Due to DSDV maintaining and updating the routing network of large-scale USV clusters frequently, although the delay is effectively reduced and network reliability is improved, it increases the operating cost of USVs and significantly increases energy consumption (AL-Dhief et al., 2018).
AODV is a typical reactive routing. USVs using this protocol do not have to maintain the routing at all times, and the protocol takes effect only when information is transmitted between USVs, in which case it can better adapt to the dynamic changes of the link as well as reduce the overhead of mutual communication between nodes. AODV has the characteristics of fast adaptation to a dynamic link environment, low overhead, and support for multicast. The routing algorithm used in this protocol is a dynamic algorithm. Even when the USV is moving at a high speed, the construction of the route can be completed quickly, and the information can be accurately delivered to the destination. If there are some errors, they can be repaired in a relatively short period of time based on a specific repair method, minimizing communication interruption time and thereby reducing the energy consumption of the USV routing network.
Through the introduction of DSDV and AODV protocols and under the condition of limited energy reserve of USVs, choosing the ADOV protocol can make the maintenance process of information more concise and effectively reduce the energy consumption of USVs.
The topology of the USV ad hoc network will continue to change according to the task situation and the surrounding environment, and the energy reserve is limited. To sum up, the AODV protocol is applicable to the continuous topology change, multi-hop, high dynamic, and large-scale USV network. Based on the AODV protocol, the low-energy-consumption USVs will only issue an application to build a route when it has to transmit data, and it is not necessary to maintain the state of the topology during the task execution, which can reduce the routing overhead. If the USV in the middle also has a reply mechanism, it only takes a relatively short time to find a route in this case, and the communication link can be reconfigured in a short time.
Three message types are defined in the AODV routing protocol: Route Request (RREQ) message, Route Reply (RREP) message, and Route Error (RERR) message (Deepak and Anandakumar, 2019), and three node types are defined: source node, intermediate node (forward node), and destination node (destination). If a node in an ad hoc network is to successfully transmit a data message, two conditions must be met:
1) Node wj is within the transmission range of node wi, which is
2) At the same time, all other nodes wj in the network that are sending data packets to the destination node wk satisfy:
where Δ is a constant greater than zero, representing the protection area.
As shown in Figure 3, in USVs 1, the virtual leader communicates with other members in the swarm with a routing distance of radius r, and communicates with the virtual leaders of USVs 2 and USVs 3 with a routing distance of radius R. Suppose to communicate with a swarm with a routing distance greater than R, a communication link needs to be established through the AODV routing protocol.
4.1.1 Reverse Route Establishment
When the AODV protocol in the ad hoc network of multiple groups of USVs starts to execute, when a USV sends the RREQ message to the destination USV, each node that the RREQ message passes through will be recorded to the reverse path of the source node. After the destination node is found, it is no longer necessary to broadcast the RREQ message. The RREP message will be propagated along this path, and the node that receives the RREQ message records the address of the previous hop node. While establishing a reverse route, sufficient time should be maintained so that the RREQ message traverses the entire network and generates an RREP message to the node sending the RREQ message (Parveen and Chaubey, 2019).
4.1.2 Forward Route Establishment
When the RREQ message reaches the node or destination node that contains the routing information of the destination node, the reverse path will be used to send the RREP message. A forward route is established while forwarding the RREP message. It can be said that the forward route is the opposite of the reverse route. Once the forward route is established, the source node can begin data transfer.
After a certain RREP message is forwarded by a node, it can receive another RREP message. The RREP message is discarded or forwarded, depending on its destination node sequence number. If a new RREP message has a higher destination node sequence number, the route will be updated and the new RREP message will be forwarded. If the destination node sequence number of the old RREP message and the new RREP message is the same and the new RREP message has a smaller hop count, the new RREP message will be preferentially selected and forwarded; otherwise, all RREP messages arriving later will be discarded. Other RREP messages are discarded, which reduces the number of RREP messages transmitted to the source node and ensures the update of routing information. When the source node receives the first RREP message, the source node can start the destination node data transmission (Kitts et al., 2012).
The routing establishment process of the USVs is shown in Figure 4. When USV A needs to send a message to the destination USV H, USV A will broadcast an RREQ message to USVs B and E. The USV receiving the RREQ message will judge whether the reverse route needs to be updated according to the number of hops and select the best route according to the principle of the smallest number of hops. When USV B receives the RREQ message from USV A, it will compare whether the IP address of USV B is the same as the destination USV address in the RREQ message. If they are the same, add 1 to the serial number of USV B and generate an RREP message. Otherwise, USV B is not the destination USV. It will update the reverse route to USV A to establish a reverse route and forward the RREQ message to other USVs. By analogy, when the destination USV H receives the RREQ message from USV A, USV H generates an RREP message and returns to USV A along the reverse route. When USV A receives the RREP message from the destination USV H, USV A starts to transmit data. So far, the route selection from USV A to the destination USV H has been completed.
4.2 Topological Design of Unmanned Surface Vessels Based on External Equitable Partition
When studying ad hoc networks of USVs, the network topology is usually represented by the underlying network, which has been extensively studied on the issues of multi-consensus. The topology design of the underlying network of the USVs is carried out by graph division, and the nodes with a constant number of in-degrees are grouped into the same cell. EEP is a typical graph division, and the topology design of the communication network composed of virtual leader agents of each group of USVs in the re-networked multiple groups of low-energy-consumption USVs is performed to achieve green autonomous cooperative formation.
After multiple groups of low-energy-consumption USVs establish an ad hoc network based on the AODV protocol, the network routing composed of virtual leader agents of each group of MAS in the ad hoc network is represented by a directed graph
Given a directed graph
Definition 1: If any two cells ql and qk in π = {q1, q2, …, qm} are divided, including l = k, there is a constant dlk such that each node in ql has dlk neighbors in qk, then the division π = {q1, q2, …, qm} is said to be equitable.
Definition 2: Given a division π = {q1, q2, …, qm} of a graph
In EEP, the nodes in a cell do not necessarily have the same number of neighbors because the graph produced by the partition is not regular. While a cell in a fair partition has the same out-degree to every cell, in EEP, this only applies to the number of connections between different cells.
Divide a given graph
Through the feature matrix P, let N = PTP, where N ∈ Rm×m, and the element on the diagonal of N is the size of each cell |ql|. Because the diagonal terms of PTP are not zero, PTP is invertible. Moreover, there is the following relationship between the Laplacian matrix
and obtain
Multiplying both sides of Eq. 10 by
Define the relevant feature matrix as
Now, through the definition of directed graph, this article further illustrates the graph topology. Given a Laplacian
Let
The MAS model corresponding to the low-energy-consumption USVs is given:
In Eq. 13,
By dividing the graph π = {q1, q2, …, qm}, the multi-consensus condition is defined:
The asymptotically stable flow of consistent trajectories after system partitioning is defined as follows:
By designing the Laplacian operator of the controller u of the MAS, we get:
Let
In Eq. 18, Li is the hi × hi Laplace matrix associated with the exclusive set
Through the previous methods, the controllers u of multiple groups of low-energy-consumption USVs ad hoc networks are designed to change the structure diagram of the original system, and the expected topology diagram
Based on EEP, the topology design of the ad hoc network structure of USVs is carried out, and the MAS of the low-energy-consumption USVs is grouped according to the task requirements, so that the grouped MAS reaches a multi-consensus state, improves the efficiency of task execution, and achieves the green autonomous cooperative formation strategy.
5 Simulation
Under the polymorphic network, when facing complex maritime tasks, multiple groups of USVs form a low-energy-consumption multilateral distributed cooperative control system to achieve green autonomous cooperative formation.
5.1 Routing Design of Low-Energy-Consumption Unmanned Surface Vessels Under Ad Hoc On-Demand Distance Vector Routing Protocol
The simulation scenario is set as follows: by evaluating the EEOI index of each USV in the ad hoc network of the USVs, in the selection of the USVs with the smallest EEOI index, eight USVs are selected to form a network with the surrounding neighbors within the range of the communication radius r, respectively, forming the MAS of eight USVs. Based on the AODV protocol and the Prim algorithm, a node is used as the starting point, and the edge with the minimum weight of each vertex is gradually found to construct the minimum spanning tree so as to find the best route with the minimum number of hops. As shown in Figure 5, the eight black circles in the figure represent eight MAS, respectively, and the radius of the route established by each MAS is R (the dotted circle in the figure is the routing radius), where eight USVs are represented by eight nodes, and their coordinates are (3, 31) m, (12, 31) m, (6.5, 23) m, (9, 16) m, (18, 19) m, (23, 26) m, (6, 7) m, (13, 0.2) m. Let the radius of the route established by each USV be R = 10 m, and calculate the distance between the two points by Eq. 6. When d ⩽ R, a route can be established directly between two nodes. When d > R, no route can be established directly between nodes. Then, according to the AODV routing protocol, according to the principle of the smallest number of hops, find a suitable intermediate node.
A) The route is established with node 1 as the starting point and node 4 as the endpoint. According to Eq. 6, the distance between node 1 and node 4 is d = 16.16 m, which is greater than the routing radius R = 10 m of the node. The route between node 1 and node 4 cannot be established directly. The routing radius of node 1 includes node 2 and node 4, while the routing radius of node 3 includes node 1, node 2, and node 4. As shown in Figure 6, there are two routes from node 1 to node 4, namely, 1 → 2 → 3 → 4 and 1 → 3 → 4. Considering the principle of green energy saving and the minimum number of hops, the AODV protocol obtains the optimal route as 1 → 3 → 4.
B) The route is established with node 6 and node 4 as the endpoints. According to Eq. 6, the distance between node 6 and node 4 is d = 86 m, which is greater than the routing radius of the node R = 10 m. The route between node 6 and node 4 cannot be established directly. The routing radius of node 6 includes node 5, and the routing radius of node 5 includes node 5 and node 4. As shown in Figure 7, the route from node 6 to node 4 is derived as 6 → 5 → 4 based on the AODV protocol.
C) The route is established with node 8 and node 4 as the endpoints. According to Eq. 6, the distance between node 8 and node 4 is d = 655.64 m, which is greater than the routing radius of the node R = 10 m. The route between node 8 and node 4 cannot be established directly. The routing radius of node 8 includes node 7, and the routing radius of node 7 includes node 8 and node 4. As shown in Figure 8, the route from node 6 to node 4 is derived as 8 → 7 → 4 based on the AODV protocol.
Through the simulation results, it can be seen that in the multilateral distributed cooperative control system of multiple groups of low-carbon and low-energy-consumption USVs, the virtual leader agent of each group of low-energy-consumption USVs achieves the goal of establishing routes with long-distance nodes with the minimum number of hops based on the AODV protocol, which follows the green energy-saving concept of realizing communication between swarms with the least routing and effectively reduces the energy consumption caused by the re-networking of multiple groups of low-carbon and low-energy-consumption USVs facing complex tasks under the polymorphic network.
5.2 Topology Design of Low-Energy-Consumption Unmanned Surface Vessel Communication Network Under External Equitable Partition
The simulation scenario is set as follows: after the route formation of the low-energy-consumption USVs is completed, in order to enable the low-energy-consumption USVs to further perform tasks according to the assignment of tasks, it is necessary to design the topology of the communication network of the low-energy-consumption USVs based on the connectivity of network routing. The established network routing of the low-energy-consumption USVs is shown in Figure 9.
Based on graph theory, the Laplacian operator for network routing in Figure 9 is:
Let the initial state of the eight USVs be x0 = [1; 3; 5; 7; 2; 4; 6; 8] and the time to be t ∈ [1, 10]. Through the dynamic equation
According to the task requirements, the following topology design is carried out on the structure of the system through EEP: node 1, node 2, and node 3 are divided into a group for operations, node 5 and node 6 are divided into a group, node 7 and node 8 are divided into a group, and the bidirectional link from node 1 to node 2 is added through controller u, the route from node 5 to node 6 is added, and the route from node 7 to node 8 is added; delete the route from node 2 to node 3. Get the following 4 grouping sets, which are
Transpose
The characteristic matrix P of Figure 11 and the Laplace matrix
The relation of
The exclusive set after the graph is divided as
The experimental results show that on the basis of ensuring the connectivity of the entire network routing of multiple groups of low-energy-consumption USVs, the topology design of network routing is carried out based on EEP, improving the work efficiency of multiple groups of low-energy-consumption USVs, achieving the expected multi-consensus state, realizing low-energy-consumption multilateral cooperative control, and finally realizing the green autonomous cooperative formation of multiple groups of low-energy-consumption USVs.
6 Conclusion
In the polymorphic network environment, both artificial intelligence and ad hoc networks have been rapidly developed and widely used. In this article, a low-energy-consumption networking method for USVs that perform large-scale and complex tasks has been presented. Combined with the characteristics of the polymorphic network, such as multilateral co-management, green energy saving, and scalable structure, a low-energy-consumption multilateral distributed cooperative control system framework for multiple groups of USVs has been proposed. First, through the evaluation of the EEOI index, low-carbon and low-energy-consumption USVs have been selected to form a system for the task. Second, a green networking method for low-energy-consumption USVs with minimum routings has been proposed. In addition, a distributed cooperative control protocol based on the MAS multi-consensus algorithm has been designed to realize the multilateral cooperative control of multiple groups of low-energy-consumption USVs. Furthermore, the green autonomous cooperative formation can be realized, and USVs can perform tasks efficiently and quickly in groups. Finally, the effectiveness of the proposed method has been verified by a simulation example, which provides technical support and a theoretical basis for realizing green energy-saving autonomous cooperative control of USVs in a polymorphic network environment.
Data Availability Statement
The original contributions presented in the study are included in the article/Supplementary Material; further inquiries can be directed to the corresponding authors.
Author Contributions
YL and GX constructed low-energy-consumption multilateral distributed cooperative control framework for ad hoc of low-carbon USVs. QS built the USV model, designed the method, adapted the article, and processed the experimental simulations data. YL collated and proofread the whole article. WL corrected the format of the references. All authors have read and agreed to the publication of the manuscript.
Funding
This research is supported by the National Key R&D Program of China (2019YFB1802501) and Key Research Project of Zhejiang Lab (2021LE0AC02); High Level Talents Innovation Support Plan of Dalian (Young Science and Technology Star Project) (under Grant No. 2021RQ058); the National Natural Science Foundation of China (under Grant Nos. 51939001, 61976033, 61751202, 61903092, and U1813203); the Science and Technology Innovation Funds of Dalian (under Grant No. 2018J11CY022); and the Liaoning Revitalization Talents Program (under Grant Nos. XLYC1908018 and XLYC1807046).
Conflict of Interest
The authors declare that the research was conducted in the absence of any commercial or financial relationships that could be construed as a potential conflict of interest.
Publisher’s Note
All claims expressed in this article are solely those of the authors and do not necessarily represent those of their affiliated organizations, or those of the publisher, the editors, and the reviewers. Any product that may be evaluated in this article, or claim that may be made by its manufacturer, is not guaranteed or endorsed by the publisher.
References
AL-Dhief, F. T., Sabri, N., Salim, M. S., Fouad, S., and Aljunid, S. A. (2018). Manet Routing Protocols Evaluation: Aodv, Dsr and Dsdv Perspective. Matec Web Conf. 150, 06024. doi:10.1051/matecconf/201815006024
Alslaim, M. N., Devi, P. M., and Sharmila, B. (2016). Comparative Study of Manet Routing Protocols. Asia. Jour. Rese. Soci. Scie. Hum. 6, 1924. doi:10.5958/2249-7315.2016.00337.3
Bertaska, I. R., Shah, B., and von Ellenrieder, K. (2015). Experimental Evaluation of Automatically-Generated Behaviors for Usv Operations. Ocean. Eng. 106, 496–514. doi:10.1016/j.oceaneng.2015.07.002
Bhagyalakshmi, , and Dogra, A. K. (2018). Q-Aodv: A Flood Control Ad-Hoc on Demand Distance Vector Routing Protocol. In 2018 First International Conference on Secure Cyber Computing and Communication (ICSCCC). 294–299. doi:10.1109/icsccc.2018.8703220
Chon, S., Haiyan, W., Chao, L., and Ye, Z. (2019). Dynamic Prediction and Optimization of Energy Efficiency Operational Index (Eeoi) for an Operating Ship in Varying Environments. J. Mar. Sci. Eng. 7, 402. doi:10.3390/jmse7110402
Chou, T. C. K., and Abraham, J. A. (1982). Load Balancing in Distributed Systems. IIEEE Trans. Softw. Eng. SE-8, 401–412. doi:10.1109/TSE.1982.235574
Deepak, S., and Anandakumar, H. (2019). “Aodv Route Discovery and Route Maintenance in Manets,” in 2019 5th International Conference on Advanced Computing & Communication Systems (ICACCS), 1187–1191. doi:10.1109/ICACCS.2019.8728456
Durfee, E. H., and Corkill, D. D. (1989). Trends in Cooperative Distributed Problem Solving. IEEE Trans. Knowl. Data Eng. 1, 63–83. doi:10.1109/69.43404
Gambuzza, L. V., and Frasca, M. (2019). A Criterion for Stability of Cluster Synchronization in Networks with External Equitable Partitions. Automatica 100, 212–218. doi:10.1016/j.automatica.2018.11.026
Garey, M. R., Graham, R. L., and Johnson, D. S. (1978). Performance Guarantees for Scheduling Algorithms. Operations Res. 26, 3–21. doi:10.2307/169888
Hui, L., Xiangui, W., and Zhili, L. (2019). Systems and Methods for Managing Top-Level Domain Names Using Consortium Blockchain.
Jiangxing, W., and Kaizhi, H. (2022). Innovating the Paradigm of Network Development and Constructing a Polymorphic Network Environment ‖ Introduction to the Special Topic of “Key Technologies of Polymorphic Network Environment”. J. Commun. 43, 1.
Jiangxing, W., and Yuxiang, H. (2021). The Development Paradigm of Separation between Network Technica System and Supporting Environment. Inf. Commun. Technol. Policy 47, 1–11. doi:10.12267/j.issn.2096-5931.2021.08.001
Jingmin, H. (2016). Analysis of Ship Energy Efficiency Operation Index and Energy Efficiency Management Practice. World Shipp. 39, 5. doi:10.16176/j.cnki.21-1284.2016.07.007
Joseph, C., John, L., and Andrew, P. (2005). “Scout - a Low-Cost Autonomous Surface Platform for Research in Cooperative Autonomy,” in Proceedings of OCEANS 2005 MTS/IEEE. doi:10.1109/OCEANS.2005.1639838
Julong, L., Dongnian, C., and Yuxian, H. (2014). Research on Reconfigurable Information Communication Basal Network Architecture. J. Commun. 35, 12. doi:10.3969/j.issn.1000-436x.2014.01.015
Kitts, C., Mahacek, P., Adamek, T., Rasal, K., Howard, V., Li, S., et al. (2012). Field Operation of a Robotic Small Waterplane Area Twin Hull Boat for Shallow-Water Bathymetric Characterization. J. Field Robot. 29, 924–938. doi:10.1002/rob.21427
Lei, H., Huang, B., and Chunxia, Z. (2011). Geographic Routing Algorithm Based on Link Quality in Mobile Ad-Hoc Networks. Comput. Sci. 38, 51–54. doi:10.3969/j.issn.1002-137X.2011.10.010
Lei, T., and Qi, F. (2002). Cooperative Distributed Planning in Artificial Intelligence. Comput. Eng. Appl. 38, 4. doi:10.1007/s11769-002-0038-4
Lewis, M. A., and Tan, K.-H. (1997). High Precision Formation Control of Mobile Robots Using Virtual Structures. Aut. Robots 4, 387–403. doi:10.1023/A:1008814708459
Li, H., Han, Y., Li, G., Wang, H., Lv, S., Ma, J., et al. (2019). Prototype and Testing Report of a Multi-Identifier System for Reconfigurable Network Architecture under Co-governing. Sci. Sin.-Inf. 49, 1186–1204. doi:10.1360/N112019-00070
Mohsen, S. A., and Naif, D. A. (2019). Routing Protocols Design and Performance Evaluation in Wireless Mesh Networks. Int. J. Technol. Diffusion 10, 60–74. doi:10.4018/IJTD.2019010105
Olfati-Saber, R., Fax, J. A., and Murray, R. M. (2007). Consensus and Cooperation in Networked Multi-Agent Systems. Proc. IEEE 95, 215–233. doi:10.1109/JPROC.2006.887293
Parveen, S., and Chaubey, V. K. (2019). An Enhanced Ad Hoc on Demand Distance Vector Routing Protocol for Vehicular Ad Hoc Networks (Vanet’s). SSRN Electron. J., 799–805. doi:10.2139/ssrn.3355137
Qiang, M., Danlin, Y., and Zongfu, Y. (2011). Energy Effective Routing Algorithm Based on Aodv. Comput. Eng. Des. 32, 4. doi:10.1080/01932691003662381
Qiaoyun, M., Liu, H., and Xueguang, C. (2007). Analysis and Modeling of Task Allocation Problem in MAS[J]. J. Huazhong Univ. Sci. Technol. (Nat. Sci. Ed.) 2007 (01), 54–57. doi:10.13245/j.hust.2007.01.017
Rui, S., Yuanchang, L., and Richard, B. (2017). A Multi-Layered Fast Marching Method for Unmanned Surface Vehicle Path Planning in a Time-Variant Maritime Environment. Ocean. Eng. 129, 301–317. doi:10.1016/j.oceaneng.2016.11.009
Scott, R., Dowdell, J., and Perkins, C. (2003). Ad Hoc on-demand Distance Vector (Aodvv2) Routing. Internet Draft Draft Ietf Manet Aodv Txt 6, 90. doi:10.1109/MCSA.1999.749281
Scott, R. (2013). Mobile Ad-Hoc Networks (Manet). IETF Work. Group Chart. doi:10.1109/GreenCom-CPSCom.2010
Sharma, D. K., Kumar, C., Jain, S., and Tyagi, N. (2012). “An Enhancement of Aodv Routing Protocol for Wireless Ad Hoc Networks,” in 2012 1st International Conference on Recent Advances in Information Technology (RAIT), 290. doi:10.1109/RAIT.2012.6194522
Shirani, R., Marc, H., and Yifeng, Z. (2012). “Combined Reactive-Geographic Routing for Unmanned Aeronautical Ad-Hoc Networks,” in Wireless Communications & Mobile Computing Conference, 820–826. doi:10.1109/iwcmc.2012.6314310
Smith, S. (1980). The Contract Net Protocol: High-Level Communication and Control in a Distributed Problem Solver. Comput. IEEE Trans. C 29, 1104–1113. doi:10.1109/TC.1980.1675516
Xueli, Z., Qian, Q., Qingwen, W., and Yongqiang, L. (2017). “An Adaptive Density-Based Routing Protocol for Flying Ad Hocnetworks,” in 2nd International Conference on Materials Science, Resource and Environmental Engineering. doi:10.1063/1.5005315
Yan, S., Songlin, Y., Yuxiao, H., Weisun, K., and Chengjin, Z. (2021). Comprehensive Optimization Analysis of Green Energy Catamaran Unmanned Craft. Ship Sci. Technol. 43, 5. doi:10.3404/j.issn.1672-7649.2021.03.020
Yang, D., Sun, Y., Wei, Q., Zhang, H., and Li, T. (2021). Topology Prediction and Structural Controllability Analysis of Complex Networks without Connection Information. IEEE Trans. Syst. Man. Cybern. Syst. 1, 1–13. doi:10.1109/TSMC.2021.3131490
Yuan, G., Zhengzhong, W., and Jiande, L. (2007). Optimization to Ad Hoc On-Demand Distance-Vector Routing Protoco through Path Collection. Comput. Eng. 33, 4. doi:10.1016/j.cageo.2006.02.011
Yushuai, L., Wenzhong, G., Wei, G., and Huaguang, Z. (2021). A Distributed Double-Newton Descent Algorithm for Cooperative Energy Management of Multiple Energy Bodies in Energy Internet. IEEE Trans. Industrial Inf. 17, 5993–6003. doi:10.1109/TII.2020.3029974
Źarko, K., Darijo, M., and Romana Capor, H. (2021). Analysis of Sea Pollution by Sewage from Vessels. Sustainability 14, 1–21. doi:10.3390/su14010263
Zheng, W., Guangfu, L., and Jia, R. (2021). Dynamic Path Planning for Unmanned Surface Vehicle in Complex Offshore Areas Based on Hybrid Algorithm. Comput. Commun. 166, 49–56. doi:10.1016/j.comcom.2020.11.012
Zhengfeng, L., Longhui, Z., Naxin, W., and Xiaofeng, K. (2021). Study on Path Planning and Following Control of Unmanned Surface Vehicles in Restricted Areas. J. Ship Mech. 25, 10. doi:10.3969/j.issn.1007-7294.2021.09.001
Zhenyu, G., and Ge, G. (2018). Research Status and Progress in Cooperative Formation of Multiple Autonomous Surface Vehicles. Inf. Control 47, 13. doi:10.13976/j.cnki.xk.2018.7381
Keywords: low-carbon USV, polymorphic network, low-energy consumption, multilateral distributed control protocol, green autonomous cooperative formation strategy
Citation: Lu Y, Shan Q, Xiao G, Liang Y and Liu W (2022) Green Polymorphic Cooperative Formation Strategy of Low-Carbon Unmanned Surface Vessels. Front. Energy Res. 10:953485. doi: 10.3389/fenrg.2022.953485
Received: 26 May 2022; Accepted: 20 June 2022;
Published: 25 July 2022.
Edited by:
Bonan Huang, Northeastern University, ChinaReviewed by:
Yongfeng Gao, Dalian University of Technology, ChinaXiting Peng, Shenyang University of Technology, China
Peiyuan Guan, University of Oslo, Norway
Copyright © 2022 Lu, Shan, Xiao, Liang and Liu. This is an open-access article distributed under the terms of the Creative Commons Attribution License (CC BY). The use, distribution or reproduction in other forums is permitted, provided the original author(s) and the copyright owner(s) are credited and that the original publication in this journal is cited, in accordance with accepted academic practice. No use, distribution or reproduction is permitted which does not comply with these terms.
*Correspondence: Qihe Shan, c2hhbnFpaGVAMTYzLmNvbQ==; Geyang Xiao, eGd5YWxhbkBvdXRsb29rLmNvbQ==