- 1Navigation College, Dalian Maritime University, Dalian, China
- 2Research Institute of Intelligent Networks, Zhejiang Lab, Hangzhou, China
In order to reduce the carbon emission of the port and build a green port, a polymorphic distributed energy management method for the low carbon port microgrid with carbon capture and carbon storage device is proposed. Firstly, this paper presents a low carbon port microgrid in a polymorphic network environment to realize the information interaction among energy subjects in different modes and improve network communication performance among port power generation device, main grid, carbon capture and carbon storage device. Secondly, the energy management model of low-carbon port microgrid is constructed considering the additional carbon capture device and carbon storage device in the port. Then, based on the multi-agent consensus algorithm, a distributed energy management method is proposed, which is respectively oriented to the grid-connected operation mode, island operation mode and switching operation mode of the port microgrid, so as to achieve the economic, low carbon and reliable operation of the port microgrid. Finally, the simulation results of Matlab verify the effectiveness of the proposed method.
1 Introduction
Under the accelerated development of the global economy, the demand for cargo transportation in international trade is growing. As the node of global maritime transport, ports are embracing new development opportunities. However, the large amount of carbon dioxide emitted by the port aggravates environmental pollution (Kinnon et al., 2021) and leads to global warming, which hinders the sustainable development of the port. At the same time, under the influence of IMO regulations and the urgent demand for carbon neutrality in the world (Wang et al., 2018), it is critical to reduce
With the transformation of port energy, utilizing new energy sources to supply power for ports has become an effective way to reduce port carbon emissions (Zhang et al., 2021). However, considering the instability and uncertainty of new energy sources (Li et al., 2021; Wang et al., 2022), it is still necessary to include conventional power plants in the port microgrid to ensure the reliability of the port power supply. Energy management of port microgrid is the key to ensure its reliable operation and has been studied by many experts and scholars. The energy management problem is a complex system optimization problem with the objective of maximizing the operating economy and satisfying multiple constraints for safe and stable operation (Deng et al., 2021; Zhang et al., 2022a). To address the additional cost of specific types of load demand in ports, Kermani (Kermani et al., 2022) proposed an energy storage system that includes multiple energy storage devices to decline power peaks, reduce energy waste and ensure port economics. Kanellos (Gennitsaris and Kanellos, 2019) proposed a multi-agent based real-time load demand response system to limit port carbon emissions and minimize port operating costs to solve the problem of flexible loads and significant carbon emissions in ports. Most current energy management methods are classified as centralized or distributed algorithms (Yang et al., 2019; Yang et al., 1109; Huang et al., 2022), but for low carbon port microgrid that contains large scale clean energy, due to the distributed nature of its generation devices and loads, distributed algorithms have attracted widespread attention. To tackle the existence of multiple generation modes and load types in ports, Zhang (Zhang et al., 2020) proposed a novel distributed energy management approach for ports based on a multi-agent consensus algorithm to optimize port energy allocation and improve energy efficiency. Aiming at the damage caused by false data injection attacks on port power systems, Shan (Shan et al., 2022) proposed a distributed energy management strategy with a topology reconstruction mechanism to mitigate the impact of the attacks and improve the security of port power systems. Port microgrids are divided into two modes of operation: grid-connected mode and island mode. Meanwhile, the network contains a variety of power generation devices such as conventional power plants, photovoltaic power generation, wind turbine power generation, as well as carbon capture and carbon sequestration device, with the diversified mode. Due to the problems of single IP and low suppression of unknown threats in the existing communication networks (Guan et al., 2018; Hu et al., 2019), it is difficult to adapt to the distributed energy management of the low-carbon port microgrid. Therefore, polymorphic networks, that match the actual low carbon port microgrid, must be developed so that the communication network can obtain polymorphic convergence dynamic. And relevant scholars have already conducted wide exploration on polymorphic networks. Wu (Hu et al., 2020) introduced “structure definability” to all aspects of networks to improve the efficiency, performance, functionality and security of the Internet from the perspective of network architecture and to achieve the requirements of nowadays intelligent, diverse, highly robust and efficient networks. Hu (Hu et al., 2022) proposed a scheme of the polymorphic network element based on codesign of domain-specific software and a heterogeneous resource allocation and replacement method to realize efficient resource utilization. The results show that the proposed scheme can provide a basic platform to support the polymorphic network. In (Salamatian, 2011), Kave proposed two propositions: the Internet of the future should be polymorphic and it should be built on a strong foundation of network science. Zhang (Zhang et al., 2022b) constructed a multilateralised distributed cooperative control framework and proposed a communication topology reconfiguration method applicable to multiple multi-agent systems with different functions after networking in order to address the limitations of cooperative control of a single multi-agent system in a unilateral network environment. Thus it can be seen that the polymorphic network promotes the implementation of distributed control and optimization technology. As existing networks cannot meet the communication needs of modern smart ports, how to build a low-carbon port microgrid in a polymorphic network environment and realize its distributed energy management is the issue that needs attention.
Large-scale clean energy is connected to the port microgrid instead of traditional energy, which has achieved the reduction of pollution and carbon emission in the port to a certain extent. To further reduce carbon emissions, carbon capture devices need to be installed in ports to capture carbon dioxide, capturing the carbon dioxide emitted from conventional power plants in ports and then storing it through carbon sequestration devices, which can minimize the emission of carbon dioxide from port microgrid to the air (Damm and Fedorov, 2008) and promote the implementation of a green port. There have been a lot of researches on carbon capture and storage devices. Mostafa (Mostafa et al., 2018) demonstrated that carbon capture devices are more efficient in reducing
As shown above, this paper is dedicated to constructing a low-carbon port microgrid under the polymorphic network environment and proposes a distributed energy management method under various operating conditions to ensure reliable and economic operation of the port microgrid and to achieve pollution and carbon reduction in the port. The specific contributions are as follows.
1) Construct a low-carbon port microgrid based on the polymorphic network, in order to realize the information interaction among various energy subjects under different modes and improve the performance of network communication among the port power generation device, main grid and carbon capture and storage device.
2) Considering carbon capture and storage devices, the energy management model of the low-carbon port microgrid is constructed. With the objective of minimizing the operating cost of the port microgrid, the power purchase or selling cost of the main power grid and the cost of carbon, as well as considering the supply and demand balance of the port microgrid and other constraints, the energy management model is constructed to achieve economic, low-carbon and reliable operation of the port microgrid.
3) A distributed energy management method for various working conditions of the port microgrid is proposed in this paper. For both grid-connected and island operation modes of the port microgrid, the distributed energy management of the low-carbon port microgrid is implemented based on multi-agent leader-following consensus and average consensus respectively to ensure the reliability and economy of the port.
2 Analysis of Low-Carbon Port Microgrid Architecture Under Polymorphic Network
There is a carbon capture device, a carbon sequestration device, and various power generation devices such as the conventional power plant, photovoltaic power generation, and wind turbine power generation in the low-carbon port microgrid, as shown in Figure 1.
The port microgrid can be operated in grid-connected mode or island mode. In grid-connected mode, the loads in the port microgrid are powered by the main grid, conventional power plant, photovoltaic power generation, wind turbines, and storage devices. In island mode, the port microgrid is powered by the conventional power plant, photovoltaic power generation, wind turbines and storage devices.
To achieve low-carbon operation, the port microgrid uses the carbon capture device and storage device to treat the carbon dioxide emissions from the conventional power plant. Most of the carbon dioxide emitted is captured by the carbon capture device and a small amount is released into the air. The carbon dioxide captured by the carbon capture device is then encapsulated and stored in a carbon storage device, and this part of carbon dioxide can be used for various purposes, including as a raw material for P2G, making dry ice, etc.
Due to the diverse working conditions and device types of low-carbon port microgrids, it is difficult for the existing traditional communication network architecture to adapt to modern smart low-carbon ports. Therefore, this paper proposes a diversified, specialized and intelligent low-carbon port microgrid based on the polymorphic network, and its network architecture is shown in Figure 2. The low carbon port microgrid based on the polymorphic network is mainly divided into three layers: the data layer, the control layer, and the service layer, with different functions. The data layer is mainly responsible for the full-dimensional definition of the topology, protocols, software and hardware, and interfaces of the port microgrid, providing refined services for diversified applications and essential support for the realization of future network intelligence, flexibility, and diversity. The control layer undertakes the service layer upward to address and route between single modality and different modalities, and controls the data layer downward to calculate and convert the different requirements of the upper-layer business into the control information of the data layer. Through the polymorphic controller, the diversified routes are defined according to the service requirements, and the communication topology is constructed according to the constraint conditions to realize polymorphic addressing interconnection and on-demand switching, so as to lay the foundation for the subsequent distributed energy management. The service layer mainly realizes the distributed energy management of the low-carbon port microgrid. The essence of polymorphic network is the process of top-down functional fitting from business requirements to fine-grained resource partitioning on the basis of a fully-dimensional definable network structure. The three layers implement business and service fitting, service and route fitting, route and resource fitting which are driven by business requirements, respectively. First, various device operation models are established, then the low-carbon port microgrid energy management model is constructed, and finally the distributed energy management method is designed according to the port microgrid operation mode.
3 Low-Carbon Port Microgrid Energy Management Model
3.1 Objective Function
This paper aims to minimize the operating cost of polymorphic low-carbon port microgrid. The objective function includes three parts: one is the operating cost of the power generation and energy storage device; one is the cost of trading with the main grid; the other is the carbon cost. The function is as follows:
where,
3.1.1 The Operating Cost of the Power Generation and Energy Storage Device
The operating costs of power generation and energy storage devices include the conventional power plant generation costs, wind turbine generation costs, photovoltaic generation costs, and energy storage device operating costs. The details are as follows.
where,
Since the operating cost functions of the considered generation and storage devices are generally in the quadratic form (Teng et al., 2020), they can be uniformly expressed as:
where,
3.1.2 The Cost of Trading With the Main Grid
When the port microgrid is connected to the main grid, the port and the grid company agree on a trading price for electricity. When the port microgrid generates more electricity than it needs and still has a large surplus, it can sell the excess electricity into the market at the price agreed by the grid company to earn the difference; when the microgrid generates less electricity than it needs, it can buy the required electricity at the price agreed by the grid company to ensure the safe and reliable operation of the port. The cost of trading with the main grid is as follows
where,
3.1.3 The Carbon Cost
In the carbon cost, since the carbon storage device needs to store carbon dioxide through a series of methods, and the stored carbon dioxide requires a harsh storage environment, the cost of carbon sequestration should be additionally considered here. For this reason, the carbon cost includes the carbon storage costs and carbon tax costs. And the formula is as follows:
where,
In the cost of carbon, as the operation of the carbon capture device consumes electricity, it can be seen as an electrical load and is not additionally accounted for in the cost of carbon. However, carbon storage device requires various methods to sequester carbon dioxide. The sequestered carbon dioxide requires a harsh preservation environment, so the cost of carbon sequestration is also considered here. The energy consumption of a carbon capture device includes both operational and fixed energy consumption. Operational energy consumption is proportional to the mass of carbon dioxide captured. With the more carbon dioxide captured, the more operational energy is used. The amount of carbon dioxide that a carbon capture plant can capture is related to the quality of carbon dioxide emitted by conventional power plants. In contrast, the quality of carbon dioxide emitted by the port is related to the amount of electricity produced by the port plant. At the same time, the carbon capture plant consumes a certain amount of power to keep running, even when it is not in operation, and this is used as fixed energy consumption. The relevant equation for the carbon capture device is expressed as follows.
where,
The cost of carbon storage is relative to the quantity of carbon dioxide trapped by the carbon capture device, while its energy consumption is relative to the amount of carbon dioxide stored. The details are as follows
where,
Among the carbon cost, the formula for the carbon tax is as follows.
where,
3.2 Constraint
To ensure the reliable operation of the port microgrid, the following constraints should be followed.
1) Power balance constraint
where,
2) Output power constraints for power generation devices
where,
3) Energy consumption constraints for carbon capture devices
where,
4) Energy storage constraints
where,
4 Distributed Energy Management Method for Low Carbon Port Microgrid
The polymorphic port microgrid has two modes of operation: grid-connected mode and island mode. Since there are differences in the energy management methods of the two modes, the distributed energy management methods are designed in this section for the two operation modes and the operation mode switching states, respectively. The topology of the information flow of the low-carbon port microgrid based on the polymorphic network is shown in Figure 3A, and the topology of the energy flow is shown in Figure 3B, where information can be exchanged between different modes by routing. Information can be exchanged directly within the modes.
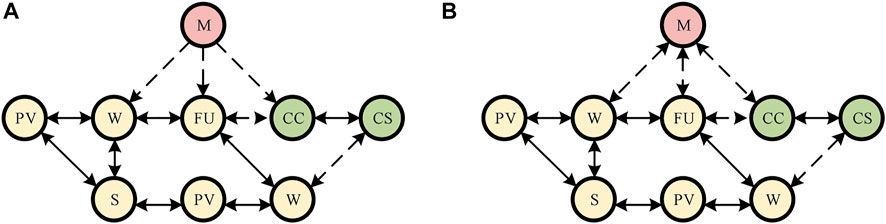
FIGURE 3. The topology of low carbon port microgrid based on polymorphic network. (A) is the topology of the information flow, (B) is the topology of the energy flow.
4.1 Graph Theory
A directed graph can be represented as
4.2 Grid Connection Mode
In the grid-connected mode, since the electricity price of the main grid is not affected by other factors, the main grid does not receive information, but only transmits information. However, in the energy flow, the main grid is able to exchange energy with the generation plant, carbon capture and storage plant. In the event that the port microgrid generates too much power, or does not generate enough power to meet the required load, it can sell power or buy power from the main grid.
Since port microgrids exhibit distributed characteristics, this section proposes a distributed energy management approach for port microgrids in grid-connected mode, where the model for the energy management problem can be written as the following equation.
Taking (1)–(8) into (13), the objective function is further expressed as:
Since in the objective function,
where,
The Lagrangian function of the model for this problem takes the form of:
where,
As the low carbon port microgrid distributed energy management model contains inequality constraints, in order to find its optimal solution, its KKT (Karush Kuhn Tucker) condition is analyzed.
According to (17), we get:
Bringing (18) into (17) gives the global optimum result as:
When in grid-connected mode, the iterative approach of electricity prices follows the leader-following consistency (Chen and Li, 2021), with the following iterative process. According to (21), the amount of power generated by each generating unit can be obtained as:
The above equation represents that the
According to (21), the power generation capacity of each generation unit can be obtained as follows.
It can be obtained that the power generation capacity of each generation unit is as follows.
where,
Then, based on the known estimated local mismatch values
where,
where,
For the above algorithm, for a connected undirected graph G, when the step size of the algorithm satisfies
where, the value of
The low carbon port microgrid under the grid-connected mode considered in this paper can both buy electricity from and sell electricity to the port’s main grid, ensuring a balance between energy supply and demand and enabling the economic operation of the port microgrid.
4.3 Island Mode
In island mode, the port microgrid cannot buy power from the main grid and has to be powered by a generation device to supply the load. In this case, not only the economy of the port must be considered, but also its security. The economic optimization of the port microgrid is achieved on the basis that the port load demand can be met. In this section, the incremental cost of the islanding model is corrected by adding a penalty factor to meet the supply-demand balance of the port microgrid. The iterative formula used is as follows.
where,
According to (26), the power generation capacity of each generation unit can be obtained by
where,
Then, according to the known
where,
For a connected undirected graph G, when the step size of the above algorithm satisfies
In the island model considered in this paper, security and the guarantee of supply and demand balance are more important as the port microgrid is not connected to the main grid. The essence of the algorithm is to follow the average consensus and add a penalty factor for feedback gain to correct the incremental cost in order to achieve safety and economy of port operation.
4.4 Switching Mode
Low carbon port microgrid switching mode operation refers to the process of switching from grid-connected mode to island mode or from island mode to grid-connected mode. In the switching process, it is necessary to realize the economical operation of the port microgrid as much as possible on the basis of ensuring the supply and demand balance. At this time, the iterative process of the electricity price
where,
According to the above equation, the power generation capacity of each generation unit can be obtained by:
where,
Then, based on the known estimated local mismatch values
Among them,
Then, the power exchanged with the main grid can be expressed as:
The switching mode considered in this paper is the switching of the port microgrid between grid-connected mode and island mode. With the algorithm used in this paper, the safe and economic operation of the port microgrid can be achieved on the basis of ensuring a balance between supply and demand in the port microgrid.
5 Simulation Results
In this paper, Matlab is used to simulate and verify the proposed method. The considered low carbon port microgrid consists of a conventional power plant, two photovoltaic power generation devices, two wind power generation devices, a storage device, a carbon capture device, a carbon sequestration device and the main grid. The energy flow topology of the low-carbon port microgrid based on the polymorphic network is shown in Figure 4 and the parameters of the power generation device are shown in Table 1. The following simulation cases are conducted in grid-connected mode, island mode and switching mode respectively.
5.1 Grid-Connection Mode
For this case, the operation of a polymorphic port microgrid in grid-connected mode is considered and divided into two scenarios: buying electricity from and selling electricity to the main grid.
5.1.1 Purchase Power From the Main Grid
When the low-carbon port microgrid generation cannot meet the required load of the port, the port will purchase power from the main grid to meet the port load demand. In this section, in order to verify the accuracy and convergence of the algorithm, the proposed model is solved using the centralized algorithm and the distributed algorithm, respectively. The simulation results of the centralized algorithm are shown in Figure 5A, and the results obtained by the distributed algorithm are shown in Figures 5B–D. The total load demand of the port microgrid is 110 MW.
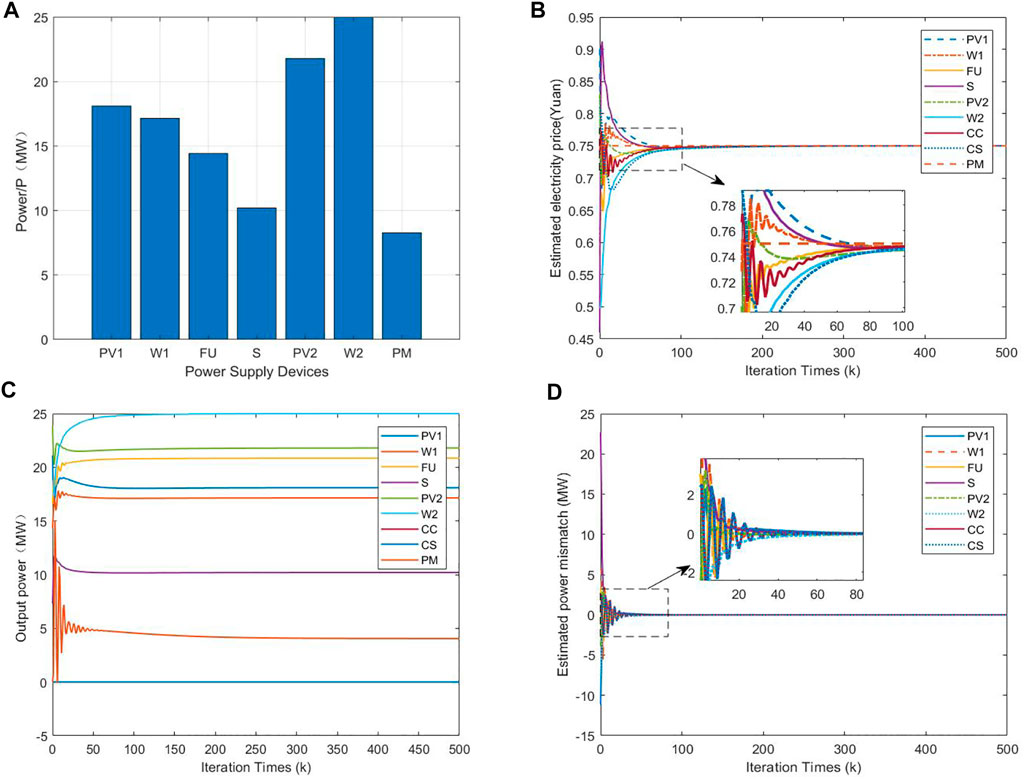
FIGURE 5. Simulation results of power purchase from the main grid in grid-connected mode. (A) is the results of centralized algorithm, (B) is the dynamic curve of the electricity price
The minimum cost result obtained in the centralized algorithm with grid-connected power purchase is 4,005.39 ¥ of each supply device as shown in Figures 5B–D. Since electricity price from the main grid is relatively high, the amount of electricity supplied from the main grid is lower. At the same time, the figure shows that the traditional power plant supplies less power and emits less
5.1.2 Sell Power to the Main Grid
When the generation capacity of the low carbon port microgrid is larger than the load required by the port itself, the port will sell electricity to the main grid to earn a profit. In this simulation case, the total load demand of the port is 100 MW, and the simulation results are shown in Figures 6A–D.
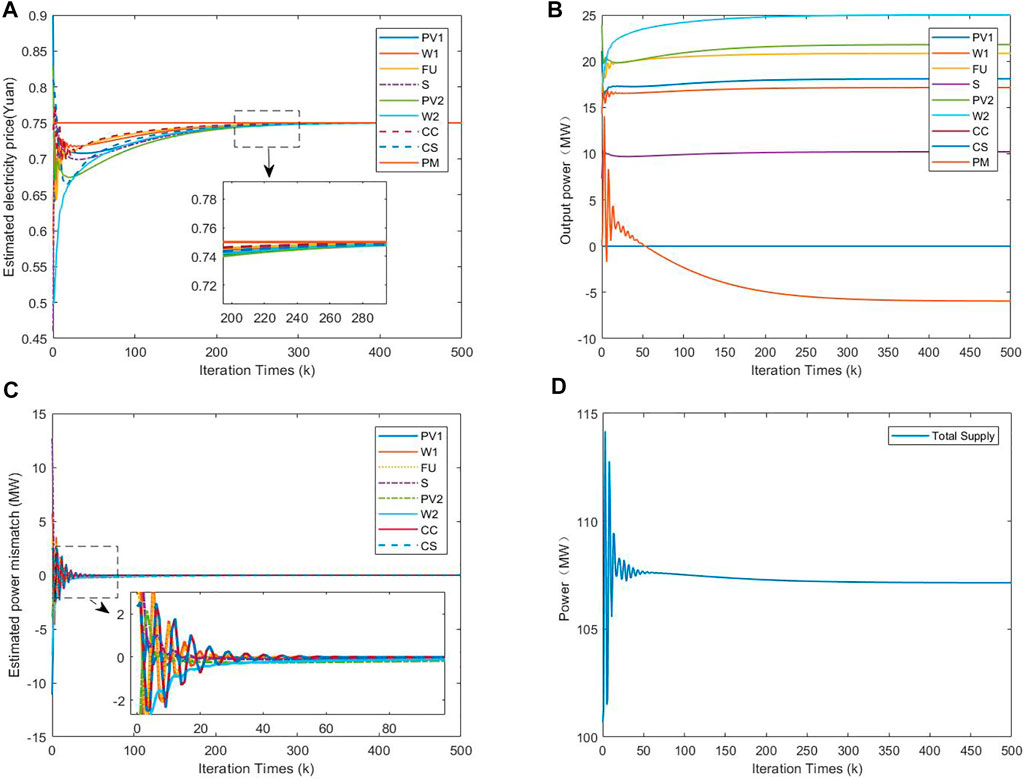
FIGURE 6. Simulation results of power selling to the main grid in grid-connected mode. (A) is the dynamic curve of the electricity price
In grid-connected mode, when the port load is 100 MW, the operating cost of the low carbon port microgrid is 3,338.28 ¥, the carbon dioxide emitted into the air is 4.44 t, and the carbon dioxide treated by the carbon capture and storage device is 10.36 t. The incremental cost (electricity price) converges to the electricity price of the main power grid when
As shown in Figure 5 and Figure 6, most of the carbon dioxide emitted from conventional power plants will be treated by carbon capture and storage devices, incurring carbon treatment costs. Although carbon dioxide is still being emitted into the air, but most of it is treated by carbon capture and storage devices, which can greatly reduce the pollution to the environment. A small proportion of the carbon dioxide emitted into the atmosphere will be subject to a carbon tax, resulting in higher power generation cost, so the port microgrid produces more electricity from photovoltaic and wind power than conventional power plants. Regardless of whether the port generates less or more than the required port load, the total generation can satisfy the supply-demand balance constraint, and its mismatch value eventually stabilizes at 0, as shown in Figure 5D and Figure 6C. If the port generates less than the required load for the port, it needs to buy electricity from the main grid, in this case
5.2 Island Mode
For this case, the operation of a polymorphic port microgrid in island mode is considered. Unlike the grid-connected mode, in the island mode, it is not possible to purchase power from the main grid, so it is essential to ensure the economics and the security of the polymorphic port microgrid. In this section, the centralized algorithms and the distributed algorithms are used to solve the proposed model, and the obtained simulation results are shown in Figures 7A–D.
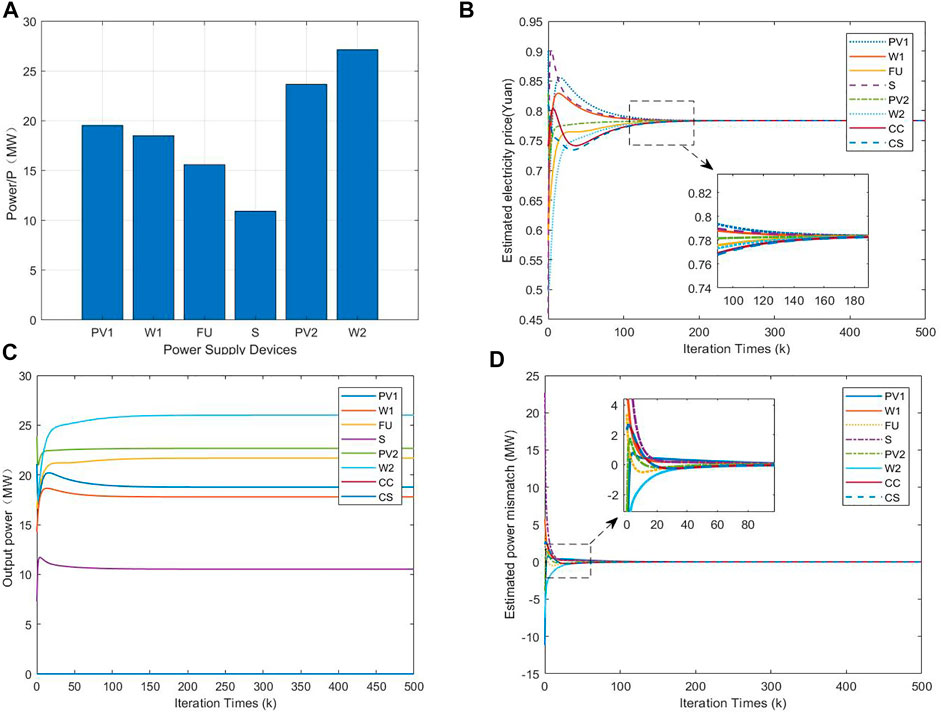
FIGURE 7. Simulation results in island mode. (A) is the results of centralized algorithm, (B) is the dynamic curve of the electricity price
The results obtained using the centralized algorithm are shown in Figure 7A, which shows the power supply of each power supply device, and the lowest cost result is 4,035.78 ¥. By using distributed algorithms, the low-carbon port microgrid costs 4,116.80 ¥, and emits 4.61 t of
The cost is reduced compared to the grid-connected mode because the port’s power generation device powers all loads. At the same time, as the island mode cannot purchase power from the main grid, to ensure the safety of the port during island mode operation and to meet the balance of supply and demand of the port microgrid, the conventional power plant generates more power and emits more carbon dioxide into the air, illustrating that the grid-connected mode can effectively reduce carbon emissions from the port and is more friendly to the environment. In terms of convergence speed, the island model uses average consensus, which is significantly faster than the grid-connected model. The simulation results for both the grid-connected and island modes, which eventually reach convergence, prove the effectiveness of the proposed algorithm.
5.3 Switching Mode
During the operation of the port microgrid, the possible emergencies will lead to its failure to connect with the main grid, changing it from grid-connected mode to island mode, which will make the supply-demand balance and security unable to be guaranteed. While the port, as an essential transport hub node, is obliged to operate continuously and reliably. Therefore, it is necessary to ensure the safety and reliability of the port microgrid during operation. In this section, a distributed energy management algorithm is used to study the switching mode of the port microgrid, which is divided into two switching modes: “grid-connected–island–grid-connected” and “island–grid-connected–island” for energy management. The simulation results are shown in Figures 8A–D and Figures 9A–D.
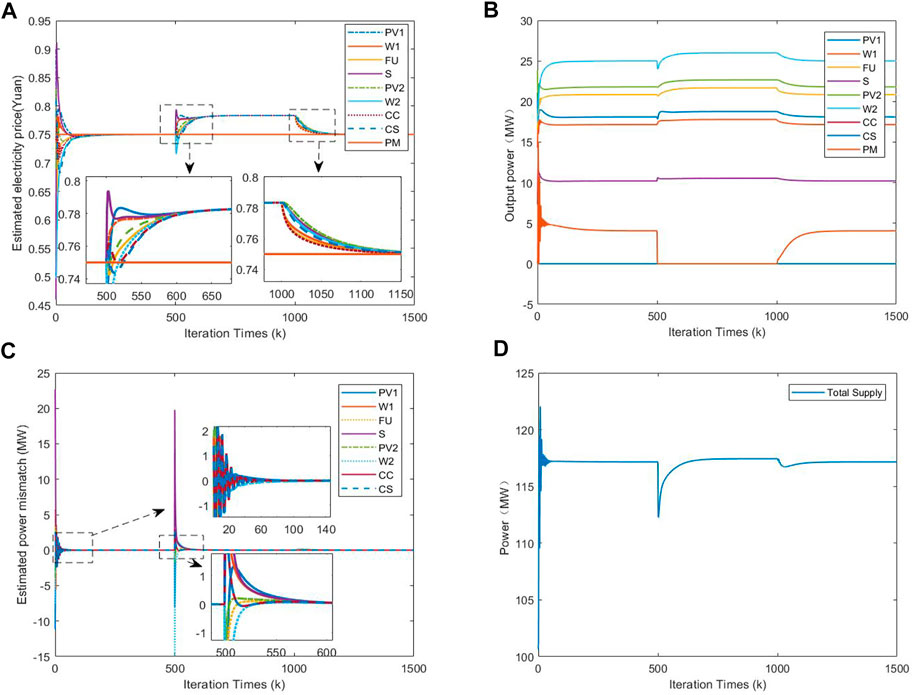
FIGURE 8. Simulation results of “grid-island-grid” switching mode. (A) is the dynamic curve of the electricity price
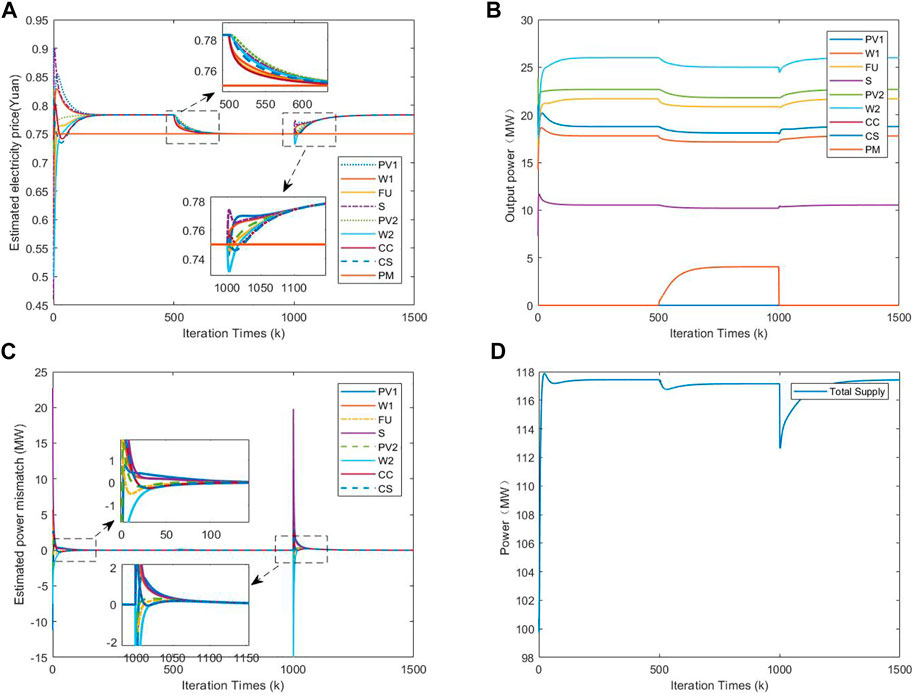
FIGURE 9. Simulation results of “island-grid-island” switching mode. (A) is the dynamic curve of the electricity price
5.3.1 Switching “Grid-Island-Grid”
From Figure 8, it can be seen that at
5.3.2 Switching “Island-Grid-Island”
At
In switching mode, the low-carbon port microgrid is still able to reduce the carbon emissions of the port. The simulation results show that the carbon emission is less in grid-connected mode than in island mode, the reason is that in island mode, the conventional power plant needs to generate more electricity to maintain the safe and stable operation of the port microgrid, so the carbon emission is more in island mode.
However, the low-carbon port microgrid is able to operate safely, economically, and at a low carbon level, whether switching from grid-connected mode to island mode or from island mode to grid-connected mode. The simulation results further validate the effectiveness of the algorithm.
6 Conclusion
The large amount of carbon emissions from the port leads to serious environmental pollution problems, so building low carbon ports is of great practical importance. In this paper, a low-carbon port microgrid with carbon capture and storage devices has been constructed in a polymorphic network environment, and its energy management problems have been investigated, and distributed solutions have been proposed for various operation modes. Firstly, a low carbon port microgrid in a polymorphic network environment has been proposed, which consists of a data layer, a control layer and a service layer, enabling the information interaction among various energy bodies in different modes and improving the performance of network communication among the power generation device, the main grid and the carbon capture and storage device. Secondly, the energy management model of a low carbon port microgrid has been constructed to minimize the operating cost of the low carbon port microgrid. Then, applicable distributed energy management methods have been proposed for various operating conditions of the port microgrid. For both grid-connected and island operation modes, the distributed energy management of the low carbon port microgrid has been implemented based on the multi-agent leader-following consensus and average consensus, respectively. In addition, the port microgrid grid-connected and island operation switching model has been discussed. Finally, the simulation results have verified the effectiveness of the proposed low-carbon port microgrid energy management method. The distributed energy management method proposed in this paper has reduced the operating cost and carbon emissions of the port microgrid, as well as realized the economical, safe and stable operation of the port.
In this paper, only the low carbon operation in the port microgrid is achieved, but not the zero carbon emission of the port. In the future, we can consider abandoning the use of traditional power plants of the port to supply electricity. The port microgrid has been kept in grid-connected mode, and when its own generation device cannot meet its own load, it purchases insufficient electricity from the main grid to realize the zero carbon operation of the port.
Data Availability Statement
The original contributions presented in the study are included in the article/Supplementary Material, further inquiries can be directed to the corresponding author.
Author Contributions
QS and JS provide research ideas for energy management and microgrid; QX and GX provide formal analysis of the polymorphic network. JS and FY write the original manuscript and are responsible for revisions of the paper. All authors have read and agreed to the published version of the manuscript.
Funding
This research is supported by the National Key R&D Program of China (2019YFB1802501) and Key Research Project of Zhejiang Lab (2021LE0AC02); High Level Talents Innovation Support Plan of Dalian (Young Science and Technology Star Project) (under Grant No. 2021RQ058); National Natural Science Foundation of China (under Grant Nos. 51939001, 61976033, 61751202, 61903092, U1813203); the Science and Technology Innovation Funds of Dalian (under Grant No. 2018J11CY022); the Liaoning Revitalization Talents Program (under Grant Nos. XLYC1908018, XLYC1807046).
Conflict of Interest
The authors declare that the research was conducted in the absence of any commercial or financial relationships that could be construed as a potential conflict of interest.
Publisher’s Note
All claims expressed in this article are solely those of the authors and do not necessarily represent those of their affiliated organizations, or those of the publisher, the editors and the reviewers. Any product that may be evaluated in this article, or claim that may be made by its manufacturer, is not guaranteed or endorsed by the publisher.
References
Akbari-Dibavar, A., Mohammadi-Ivatloo, B., Zare, K., Khalili, T., and Bidram, A. (2021). Economic-Emission Dispatch Problem in Power Systems with Carbon Capture Power Plants. IEEE Trans. Ind. Appl. 57 (4), 3341–3351. doi:10.1109/tia.2021.3079329
Chen, W., and Li, T. (2021). Distributed Economic Dispatch for Energy Internet Based on Multiagent Consensus Control. IEEE Trans. Automatic Control 66 (1), 137–152. doi:10.1109/TAC.2020.2979749
Damm, D. L., and Fedorov, A. G. (2008). Conceptual Study of Distributed CO2 Capture and the Sustainable Carbon Economy. Energy Convers. Manag. 49 (6), 1674–1683. doi:10.1016/j.enconman.2007.11.011
De, A., Wang, J., and Tiwari, M. K. (2021). Fuel Bunker Management Strategies within Sustainable Container Shipping Operation Considering Disruption and Recovery Policies. IEEE Trans. Eng. Manage. 68 (4), 1089–1111. doi:10.1109/tem.2019.2923342
Deng, X., Guan, P., Hei, C., Li, F., Liu, J., and Xiong, N. (2021). An Intelligent Resource Allocation Scheme in Energy Harvesting Cognitive Wireless Sensor Networks. IEEE Trans. Netw. Sci. Eng. 8 (2), 1900–1912. doi:10.1109/tnse.2021.3076485
Fang, S., Xu, Y., Li, Z., Ding, Z., Liu, L., and Wang, H. (2019). Optimal Sizing of Shipboard Carbon Capture System for Maritime Greenhouse Emission Control. IEEE Trans. Ind. Appl. 55 (6), 5543–5553. doi:10.1109/tia.2019.2934088
Gennitsaris, S. G., and Kanellos, F. D. (2019). Emission-Aware and Cost-Effective Distributed Demand Response System for Extensively Electrified Large Ports. IEEE Trans. Power Syst. 34 (6), 4341–4351. doi:10.1109/tpwrs.2019.2919949
Guan, P., Deng, X., Liu, Y., and Zhang, H. (2018). Analysis of Multiple Clients' Behaviors in Edge Computing Environment. IEEE Trans. Veh. Technol. 67 (9), 9052–9055. doi:10.1109/tvt.2018.2850917
Hu, Y., Cui, Z., Li, Z., Dong, Y., Cui, P., and Wu, J. (2022). Construction Technologies of Polymorphic Network Environment Based on Codesign of Domain-Specific Software/Hardware[J]. J. Commun. 43 (04), 3–13. doi:10.11959/j.issn.1000–436x.2022086
Hu, Y., Li, D., Sun, P., Yi, P., and Wu, J. (2020). Polymorphic Smart Network: An Open, Flexible and Universal Architecture for Future Heterogeneous Networks. IEEE Trans. Netw. Sci. Eng. 7 (4), 2515–2525. doi:10.1109/tnse.2020.3006249
Hu, Y., Ying, P., Sun, P., and Wu, J. (2019). Research on the Full-Dimensional Defined Polymorphic Smart Network[J]. J. Commun. 40 (08), 1–12. doi:10.11959/j.issn.1000–436x.2019192
Huang, B., Li, Y., Zhan, F., Sun, Q., and Zhang, H. (2022). A Distributed Robust Economic Dispatch Strategy for Integrated Energy System Considering Cyber-Attacks. IEEE Trans. Ind. Inf. 18 (2), 880–890. doi:10.1109/tii.2021.3077509
Kermani, M., Shirdare, E., Parise, G., Bongiorno, M., and Martirano, L. (2022). A Comprehensive Technoeconomic Solution for Demand Control in Ports: Energy Storage Systems Integration. IEEE Trans. Ind. Appl. 58 (2), 1592–1601. doi:10.1109/tia.2022.3145769
Kinnon, M. M., Razeghi, G., and Samuelsen, S. (2021). The Role of Fuel Cells in Port Microgrids to Support Sustainable Goods Movement. Renew. Sustain. Energy Rev. 147 (3), 1–17. doi:10.1016/j.rser.2021.111226
Li, Y., Gao, D. W., Gao, W., Zhang, H., and Zhou, J. (2021). A Distributed Double-Newton Descent Algorithm for Cooperative Energy Management of Multiple Energy Bodies in Energy Internet. IEEE Trans. Ind. Inf. 17 (9), 5993–6003. doi:10.1109/tii.2020.3029974
Li, Y., Gao, D. W., Gao, W., Zhang, H., and Zhou, J. (2020). Double-Mode Energy Management for Multi-Energy System via Distributed Dynamic Event-Triggered Newton-Raphson Algorithm. IEEE Trans. Smart Grid 11 (6), 5339–5356. doi:10.1109/tsg.2020.3005179
Lin, S., Zhen, L., Wang, W., and Tan, Z. (2022). Green Berth and Yard Space Allocation Under Carbon Tax Policy in Tidal Ports. Marit. Policy & Manag., 1–22. doi:10.1080/03088839.2022.2047816
Mostafa, S. S., Hedengren, J. D., and Powell, K. M. (2018). Performance Comparison of Low Temperature and Chemical Absorption Carbon Capture Processes in Response to Dynamic Electricity Demand and Price Profiles. Appl. Energy 228, 577–592. doi:10.1016/j.apenergy.2018.06.098
Salamatian, K. (2011). Toward a Polymorphic Future Internet: A Networking Science Approach. IEEE Commun. Mag. 49 (10), 174–178. doi:10.1109/mcom.2011.6035832
Shan, Q., Zhang, X., Zhang, Q., and Sun, Q. (2022). Distributed Energy Management for Port Power System Under False Data Injection Attacks. Complexity 2022, 1. doi:10.1155/2022/5995281
Teng, F., Shan, Q., and Li, T. (2020). Intelligent Ship Integrated Energy System and its Distributed 488 Optimal Scheduling Algorithm. ACTA Autom. Sin. 46 (9), 1809–1817. doi:10.1038/s41598-022-10958-7
Wang, X., Gao, D. W., Wang, J., Yan, W., Gao, W., Muljadi, E., et al. (2018). Implementations and Evaluations of Wind Turbine Inertial Controls with FAST and Digital Real-Time Simulations. IEEE Trans. Energy Convers. 33 (4), 1805–1814. doi:10.1109/tec.2018.2849022
Wang, X., Zhao, T., and Parisio, A. (2022). Frequency Regulation and Congestion Management by Virtual Storage Plants. Sustain. Energy, Grids Netw. 29 (1), 100586. doi:10.1016/j.segan.2021.100586
Yang, L., Sun, Q., Zhang, N., and Li, Y. (2022). Indirect Multi-Energy Transactions of Energy Internet with Deep Reinforcement Learning Approach. IEEE Trans. Power Syst., 1. doi:10.1109/TPWRS.2022.3142969
Yang, T., Yi, X., Wu, J., Yuan, Y., Wu, D., Meng, Z., et al. (2019). A Survey of Distributed Optimization. Annu. Rev. Control 47, 278–305. doi:10.1016/j.arcontrol.2019.05.006
Yu, S., and Wang, C. (2015). Schedule Coordination and Cargo Allocation Optimization for Liner Container Shipping Network Under Carbon Emission Trading Mechanism. J. Wuhan Univ. Technol. Transp. Sci. Eng. 39 (5), 1073–1078. doi:10.3963/j.issn.2095-3844.2015.05.038
Zhang, N., Sun, Q., Yang, L., and Li, Y. (2022). Event-Triggered Distributed Hybrid Control Scheme for the Integrated Energy System. IEEE Trans. Ind. Inf. 18 (2), 835–846. doi:10.1109/tii.2021.3075718
Zhang, Q., Shan, Q., and Li, T. (2020). “Large Port Energy Management Based on Distributed Optimization,” in 2020 7th International Conference on Information, Cybernetics, and Computational Social Systems (ICCSS), Guangzhou, China, 13-15 November 2020, 108–113. doi:10.1109/ICCSS52145.2020.9336919
Zhang, R., Xiao, G., Shan, Q., Zou, T., Li, D., and Teng, F. (2022). Communication Topology Reconstruction Method for Multi-Agent Cooperative Control in Polymorphic Networks[J]. J. Commun. 43 (04), 50–59. doi:10.11959/j.issn.1000–436x.2022077
Keywords: energy management, polymorphic, carbon tax, distributed, port microgrid
Citation: Shan Q, Song J, Xu Q, Xiao G and Yu F (2022) Polymorphic Distributed Energy Management for Low-Carbon Port Microgrid With Carbon Capture and Carbon Storage Devices. Front. Energy Res. 10:951192. doi: 10.3389/fenrg.2022.951192
Received: 23 May 2022; Accepted: 21 June 2022;
Published: 14 July 2022.
Edited by:
Yushuai Li, University of Oslo, NorwayReviewed by:
Feng Tao, Southwest Jiaotong University, ChinaLingxiao Yang, Anhui University, China
Weihang Yan, National Renewable Energy Laboratory (DOE), United States
Copyright © 2022 Shan, Song, Xu, Xiao and Yu. This is an open-access article distributed under the terms of the Creative Commons Attribution License (CC BY). The use, distribution or reproduction in other forums is permitted, provided the original author(s) and the copyright owner(s) are credited and that the original publication in this journal is cited, in accordance with accepted academic practice. No use, distribution or reproduction is permitted which does not comply with these terms.
*Correspondence: Qi Xu, eHVxaUB6aGVqaWFuZ2xhYi5jb20=