- 1College of Electrical and Information Engineering, Hunan University, Changsha, China
- 2State Grid Hunan Extra High Voltage Substation Company, Changsha, China
- 3Substation Intelligent Operation and Inspection Laboratory of State Grid Hunan Electric Power Co., Ltd, Changsha, China
Introduction
5G communication has excellent performance, such as high speed, high capacity, and large bandwidth, which is applied to terminal mass measurement and accurate control of power internet of things (Chen et al., 2019; Hui et al., 2020). However, due to the update of 5G network technology and the more intensive deployment of base stations (BSs) (Wu et al., 2021), the power consumption of BSs has increased sharply. The power consumption of a 5G base station (BS) at full load is close to 4 kW, about three times that of a 4G BS (Han et al., 2021), which increases the pressure on electricity expenditure faced by communication operators. In addition, the replacement of the lead-acid battery and the construction of 5G BS will bring much demand for the lithium battery (Tang et al., 2020), whereas the BS battery is only used as a standby power supply, with high investment cost and low energy storage utilization rate for communication operators.
Research on energy consumption management and energy-saving technology of BSs has been conducted to reduce the energy consumption by improving the efficiency of power amplifiers (Quaglia and Cripps, 2018; Cappello et al., 2019), shutting down some channels and deep dormancy idle base stations (Pervaiz et al., 2018; Wu et al., 2020; Alnoman and Anpalagan, 2021). However, the backup energy storage battery and renewable energy of the BS are not fully utilized in the existing research. Therefore, considering the configuration of renewable energy, the adjustability of energy storage battery, and the space-time characteristics of communication load, this study proposes a hierarchical distributed operational framework for renewables-assisted 5G base station clusters and smart grid interaction, which is conducive to promoting the flexible conversion of various energy sources, assisting base station operators to reduce expenses and create profits.
Interactive Operational Modeling of Renewables-Assisted 5G BS Clusters
The participation of renewables-assisted 5G BS clusters in the electricity market can provide flexible resources for the power system and improve the renewable energy consumption capacity of the smart grid (Saxena et al., 2017). On the one hand, communication operators participate in energy market transactions; comprehensively consider the communication load of BSs, the distributed photovoltaic (PV) power generation on the roof of BS’s equipment room and energy storage, and electricity price; and conduct power purchase and sales transactions to reduce the total electricity expenditure of communication operators. On the other hand, communication operators participate in auxiliary service market transactions; and provide auxiliary electric energy according to the compensation price signal during peak and valley periods to obtain the auxiliary regulation profit (He et al., 2016). In addition to conducting electricity market transactions, renewables-assisted 5G BS clusters can transfer energy between BS clusters in different regions. Electric energy is consumed from places with sufficient PV power and energy storage resources to places with insufficient PV power and energy storage resources. In this study, for the interests of communication operators, the loss cost of the energy storage battery (Yan et al., 2018), the power purchase cost of the BS power load, and the profitability of ancillary services are considered in the objective function.
The power consumption of the BS consists of static power consumption determined by the backup power, ventilator, and other hardware auxiliary facilities of communication equipment and dynamic power consumption related to the communication load depending on the number of connected mobile users and the communication traffic demand of mobile users. Due to the movement of mobile users, the communication load of the base station shows flexibility in time and space, and the power consumption of BS can be changed by adjusting the communication load (Zhou et al., 2021). In this model, an area is evenly divided into multiple blocks, and all mobile users in each block are equivalent to a user aggregation point. The changes in spatial mobility and communication quality requirements of all users in the block are transformed into the changes in bandwidth resources and transmission rate requirements at different times of user aggregation point. The BS can adjust its own communication load rate by selecting the accessed mobile users and then the power consumption of the BS. When the BS is connected to a user aggregation point, all mobile users in the user aggregation point area access the BS. Combining the above, the constraints of this study are power balance constraints, BS communication resource constraints, mobile user communication quality constraints, and energy storage battery constraints (Gao et al., 2017; Zhang et al., 2022).
Hierarchical Partition and Distributed Optimization Methodology
In the optimization model, whether the BSs and the user aggregation points are connected is formulated as a binary variable. When the scale of the BS and the mobile user increases, the coupled binary variables increase exponentially, and the electric energy between the BS clusters is also coupled, which will cause a solving difficulty. Therefore, a hierarchical partition and distributed optimization methodology are proposed for the solution. Firstly, the upper layer optimizes the connection between the BSs and user aggregation points, and then the lower layer optimizes energy storage scheduling, electricity market transactions, and the electric power transmission between BS clusters with the distributed method based on ADMM (Rajaei et al., 2021).
The purpose of the upper layer is to coordinate the BS load in different areas by adjusting the connection relationship between the BS and the user aggregation points so that the BS power load matches the PV resources to promote PV local consumption and, at the same time, the BS power load in the high electricity price region is transferred to the low electricity price region to reduce the cost of communication operators. The objective of the upper layer is the minimum electricity purchase cost and the minimum PV power curtailment, the connection between the BS and the user aggregation points is taken as a decision variable, and the electric power purchased and the PV power curtailed are introduced as auxiliary variables. Constraints include base station bandwidth resource constraints, mobile user communication quality constraints, and mobile user access constraints. After the upper layer model is solved, the result of the connection between the BS and the user aggregation points is passed to the lower layer.
In the lower layer, the problem of BS clusters in multiple functional areas is decomposed into multiple regional sub-problems based on the ADMM principle and is solved iteratively. In each iteration, each sub-problem aims to minimize the operating cost and power purchase cost, maximize the benefit, update its energy storage charging and discharging power. Firstly, each sub-problem update its energy storage charging and discharging power, traded electric power, transmitted electric power. Secondly, each regional sub-problem broadcasts the updated transmit power to other regional sub-problem. Thirdly, update Lagrangian multiplier variable. This iterative process continues until a consensus is reached on the transmitted electric power between regional sub-problems. In other words, both the original and dual residuals meet the error requirements, the iteration is terminated, and the solution is complete.
Taking 100 renewables-assisted 5G base stations evenly distributed in an area of 6 × 6 km, including three functional sub-areas as an example, the base stations are all 600 m apart, and there are 3,600 user aggregation points in total. These three sub-areas are commercial area, residential area, and university campus area, which have different communication load characteristics and implement different electricity prices. The simulation result of the connection relationship between the BS and the user aggregation point at a certain time is shown in Figure 1, in which the three sub-areas are represented by three colored squares, respectively.
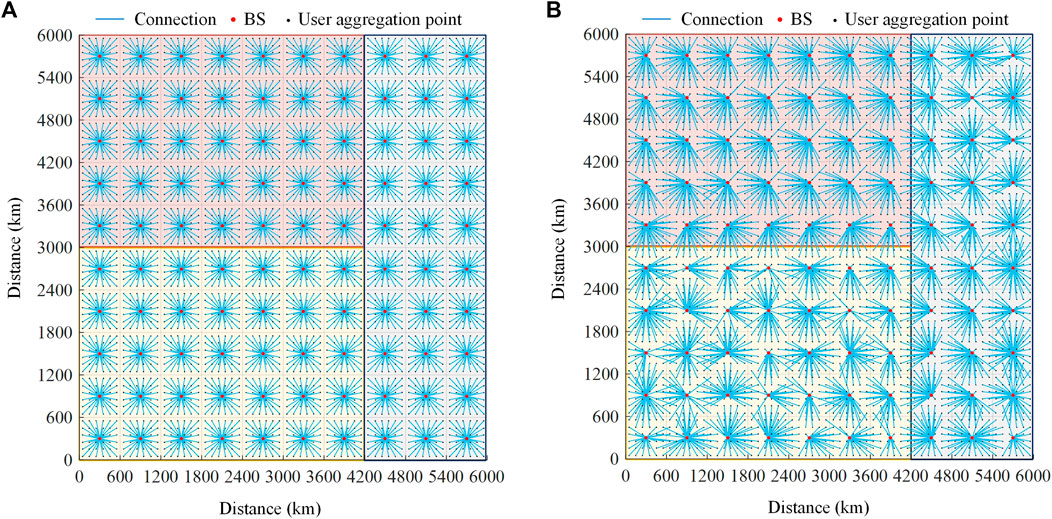
FIGURE 1. Connection diagram of BS and user aggregation point before and after optimization. (A) Connection diagram of BS and user aggregation point before optimization. (B) Optimized connection diagram of BS and user aggregation point.
Discussion and Conclusion
The high electricity cost of BSs is an urgent problem for the popularization of 5G communication. Renewables-assisted 5G base station clusters and smart grid interactions can enable flexible conversion of PV power, energy storage, and BS dynamic loads. Based on this, the flexible transfer characteristics of BS communication load and the potential utilization space of the backup battery are considered, and the interactive operational modeling of renewables-assisted 5G BS clusters is formulated. BS clusters participate in the power energy market and auxiliary service market to reduce the cost of electricity purchase and obtain income from auxiliary services. In addition, in order to solve the difficult problem caused by the coupling of massive binary variables and real variables, a hierarchical partition and distributed optimization methodology is proposed. In the future, it is necessary to further refine the transaction rules for BS clusters to participate in the auxiliary service market, such as formulating more specific pricing strategies to promote the mutual conversion of various energy sources such as power sources and loads and improve the flexibility of the power system.
Author Contributions
YF: writing the original draft and editing. BW: conceptualization. JW: formal analysis. MT and HR: visualization and contribution to the discussion of the topic.
Funding
This work was supported by the Hunan Natural Science Foundation of China under Grant no. 2021JJ10019.
Conflict of Interest
BW was employed by State grid Hunan Extra High Voltage Substation Company and Substation Intelligent Operation and Inspection Laboratory of State Grid Hunan Electric Power Co., Ltd.
The remaining authors declare that the research was conducted in the absence of any commercial or financial relationships that could be construed as a potential conflict of interest.
Publisher’s Note
All claims expressed in this article are solely those of the authors and do not necessarily represent those of their affiliated organizations or those of the publisher, the editors, and the reviewers. Any product that may be evaluated in this article, or claim that may be made by its manufacturer, is not guaranteed or endorsed by the publisher.
References
Alnoman, A., and Anpalagan, A. (2021). Computing-Aware Base Station Sleeping Mechanism in H-CRAN-Cloud-Edge Networks. IEEE Trans. Cloud Comput. 9 (3), 958–967. doi:10.1109/TCC.2019.2893228
Cappello, T., Pednekar, P., Florian, C., Cripps, S., Popovic, Z., and Barton, T. W. (2019). Supply- and Load-Modulated Balanced Amplifier for Efficient Broadband 5G Base Stations. IEEE Trans. Microw. Theory Techn. 67 (7), 3122–3133. doi:10.1109/TMTT.2019.2915082
Chen, H., Wang, X., Li, Z., Chen, W., and Cai, Y. (2019). Distributed Sensing and Cooperative Estimation/detection of Ubiquitous Power Internet of Things. Prot. Control Mod. Power Syst. 4 (2), 151–158. doi:10.1186/s41601-019-0128-2
Gao, Y., Xue, F., Yang, W., Yang, Q., Sun, Y., Sun, Y., et al. (2017). Optimal Operation Modes of Photovoltaic-Battery Energy Storage System Based Power Plants Considering Typical Scenarios. Prot. Control Mod. Power Syst. 2 (4), 397–406. doi:10.1186/s41601-017-0066-9
Han, J., Liu, N., Huang, Y., and Zhou, Z. (2021). Collaborative Optimization of Distribution Network and 5G Mobile Network with Renewable Energy Sources in Smart Grid. Int. J. Electr. Power & Energy Syst. 130, 107027. doi:10.1016/j.ijepes.2021.107027
He, G., Chen, Q., Kang, C., and Xia, Q. (2016). Optimal Offering Strategy for Concentrating Solar Power Plants in Joint Energy, Reserve and Regulation Markets. IEEE Trans. Sustain. Energy 7 (3), 1245–1254. doi:10.1109/TSTE.2016.2533637
Hui, H., Ding, Y., Shi, Q., Li, F., Song, Y., and Yan, J. (2020). 5G Network-Based Internet of Things for Demand Response in Smart Grid: A Survey on Application Potential. Appl. Energy 257, 113972. doi:10.1016/j.apenergy.2019.113972
Pervaiz, H., Onireti, O., Mohamed, A., Ali Imran, M., Tafazolli, R., and Ni, Q. (2018). Energy-Efficient and Load-Proportional eNodeB for 5G User-Centric Networks: A Multilevel Sleep Strategy Mechanism. IEEE Veh. Technol. Mag. 13 (4), 51–59. doi:10.1109/MVT.2018.2871740
Quaglia, R., and Cripps, S. (2018). A Load Modulated Balanced Amplifier for Telecom Applications. IEEE Trans. Microw. Theory Techn. 66 (3), 1328–1338. doi:10.1109/TMTT.2017.2766066
Rajaei, A., Fattaheian-Dehkordi, S., Fotuhi-Firuzabad, M., and Moeini-Aghtaie, M. (2021). Decentralized Transactive Energy Management of Multi-Microgrid Distribution Systems Based on ADMM. Int. J. Electr. Power & Energy Syst. 132, 107126. doi:10.1016/j.ijepes.2021.107126
Saxena, N., Roy, A., and Kim, H. (2017). Efficient 5G Small Cell Planning with eMBMS for Optimal Demand Response in Smart Grids. IEEE Trans. Ind. Inf. 13 (3), 1471–1481. doi:10.1109/TII.2017.2681105
Tang, F., Liu, L., Dai, H., Xu, N., Wang, C., and Zhang, N. (2020). “Integrated Energy Service for “5G” in China: Market Prediction and Case Study,” in 2020 IEEE 4th Conference on Energy Internet and Energy System Integration (EI2), Wuhan, China (IEEE), 944–949. doi:10.1109/EI250167.2020.9346922
Wu, J., Li, Y., Zhuang, H., Pan, Z., Wang, G., and Xian, Y. (2021). SMDP-based Sleep Policy for Base Stations in Heterogeneous Cellular Networks. Digital Commun. Netw. 7 (1), 120–130. doi:10.1016/j.dcan.2020.04.010
Wu, J., Wong, E. W. M., Chan, Y.-C., and Zukerman, M. (2020). Power Consumption and GoS Tradeoff in Cellular Mobile Networks with Base Station Sleeping and Related Performance Studies. IEEE Trans. Green Commun. Netw. 4 (4), 1024–1036. doi:10.1109/TGCN.2020.3000277
Yan, G., Liu, D., Li, J., and Mu, G. (2018). A Cost Accounting Method of the Li-Ion Battery Energy Storage System for Frequency Regulation Considering the Effect of Life Degradation. Prot. Control Mod. Power Syst. 3 (1), 43–51. doi:10.1186/s41601-018-0076-2
Zhang, K., Zhou, B., Or, S. W., Li, C., Chung, C. Y., and Voropai, N. (2022). Optimal Coordinated Control of Multi-Renewable-To-Hydrogen Production System for Hydrogen Fueling Stations. IEEE Trans. Ind. Appl. 58 (2), 2728–2739. doi:10.1109/TIA.2021.3093841
Keywords: smart grid, renewable energy, base station clusters, distributed optimization, internet of things
Citation: Fan Y, Wang B, Wei J, Tan M and Ran H (2022) A Hierarchical Distributed Operational Framework for Renewables-Assisted 5G Base Station Clusters and Smart Grid Interaction. Front. Energy Res. 10:943189. doi: 10.3389/fenrg.2022.943189
Received: 13 May 2022; Accepted: 19 May 2022;
Published: 30 June 2022.
Edited by:
Bo Yang, Kunming University of Science and Technology, ChinaReviewed by:
Da Xu, China University of Geosciences Wuhan, ChinaBaling Fang, Hunan University of Technology, China
Copyright © 2022 Fan, Wang, Wei, Tan and Ran. This is an open-access article distributed under the terms of the Creative Commons Attribution License (CC BY). The use, distribution or reproduction in other forums is permitted, provided the original author(s) and the copyright owner(s) are credited and that the original publication in this journal is cited, in accordance with accepted academic practice. No use, distribution or reproduction is permitted which does not comply with these terms.
*Correspondence: Juan Wei, d2VpanVhbmJhQGhudS5lZHUuY24=