- 1School of Electrical Engineering, Shenyang University of Technology, Shenyang, China
- 2State Grid Liaoning Electric Power Supply Co. Ltd., Shenyang, China
- 3State Key Laboratory of Operation and Control of Renewable Energy and Storage Systems (China Electric Power Research Institute), Beijing, China
- 4College of Information and Electrical Engineering, Shenyang Agricultural University, Shenyang, China
Under the path of global low-carbon development, increasing the proportion of renewable energy in the power grid will become the main goal in the future. But, it will also aggravate the problem of wind and solar curtailment. A joint optimal scheduling model of a renewable energy regional power grid with an energy storage system and concentrated solar power plant is proposed in this study. The proposed model takes the lowest comprehensive operation cost of the power grid as the optimization goal and considers various constraints of concentrated solar power plants, energy storage systems, thermal power units, wind power, and photovoltaic power generation. Finally, a modified IEEE 9-bus system is used to verify the validity. The results show that the model can effectively improve the system node voltage, promote the accommodation of wind and solar power, and alleviate the peak shaving of thermal power units under the premise of optimal economy pressure.
1 Introduction
Due to depletion of fossil fuels and their negative impact on the environment, the installed capacity of wind power (WP) and photovoltaic (PV) has also increased yearly. In the future, the use of renewable energy to gradually replace carbon-containing fossil energy will become the primary trend of power development (Mehigan et al., 2018; Li, Wang and Li, 2020; Li, Li and Wang, 2022). Restricted by the natural attributes of wind and solar power, WP and PV power generation has the characteristics of volatility and intermittency (Dong et al., 2020). When necessary, we have to curtail wind and solar power to ensure the safe operation of the power grid. The renewable energy regional power grid only relies on thermal power units to participate in peak shaving of the regional power grid. With the high proportion of the renewable energy regional power grid, it is difficult to absorb wind and solar power, resulting in waste of resources (Lv et al., 2020; Heptonstall et al., 2021). In addition, at the peak time of electricity consumption, the node voltage of the regional power grid is low, which increased the line loss and affects the power quality of the distribution network system (Wang, Gu and Liang, 2021). The abovementioned problems restrict the development of the renewable energy regional power grid. Therefore, in this study, we focus on considering the battery energy storage system (ESS) and concentrated solar power (CSP) plants as new flexible resources to participate in renewable energy regional power grid regulation.
In addition to PV, the CSP plant is another technology that uses solar power to generate electricity. Solar energy is concentrated through a concentrating device, and high-pressure steam is used to drive a steam turbine to generate electricity or heat. It is equipped with a heat storage device, which can store heat during low load periods and use solar energy and stored thermal energy to generate electricity during peak load periods. Also, it can not only promote the utilization of solar energy but also participate in system regulation as a flexible resource (Xu and Zhang, 2017; Jelley and Smith, 2015). Du et al. (2016) summarized the research progress of CSP technology; analyzed its characteristics from the perspectives of technology, principle, and benefit; and put forward suggestions for the development of CSP plants in China. Zhang, Dong, and Li (2021) discussed the impact of factors on the development of CSP plants in China, such as GDP growth, incentive policies, technological advances, grid absorptive capacity, and emission regulation schemes and then provided theoretical support for the future development of CSP in China. Dong, Yun, and Ma (2020) established a comprehensive energy system consisting of a CSP plant, a power-to-gas device, and a gas-fired unit and simulated the system with the minimum operating cost as the objective function. The results show that the proposed model can meet various load requirements. However, it is highly dependent on the external market and has certain uncertainties. Compared with WP and PV with output uncertainty, the output of the CSP plant is adjustable and controllable, and the adjustment speed is faster. It can be combined with renewable energy power generation to reduce wind and solar curtailment and improve renewable energy consumption capacity.
The ESS has the characteristics of fast response and flexible configuration (Deng et al., 2021). Adding an ESS to the regional power grid in conjunction with the CSP plant can effectively improve the flexibility of the regional power grid. Li and Wang (2021) pointed out that energy storage systems can improve the flexibility, economy, and safety of power systems; reviewed the research status of ESS and energy management systems in recent years; and studied their control methods and application scenarios. Li, Hui, and Lai (2013) proposed an output power control strategy based on fuzzy logic control for the energy storage system to manage the SOC of the energy storage system and smooth the WP/PV power for the problem of unstable WP/PV output power caused by intermittent characteristics.
Scholars have carried out much research on the optimal scheduling of renewable energy regional power grids. Chang et al. (2019) applied the CSP plant to the coal-based power system. Through the flexibility brought by the CSP plant for the system, the influence of the duck curve caused by high PV permeability is alleviated. The results showed that under the same PV permeability, the proposed control strategy reduces the total cost, abandoned solar power, and load loss power. Du et al. (2019) proposed a forward-looking stochastic unit commitment model for operating power systems with the CSP plant under high penetration of renewable energy and also analyzed the advantages of the CSP plant in the renewable energy system. Chen et al. (2019) combined the CSP plant and WP generation, proposed the joint dispatch model, and discussed the influence of time scale and energy storage parameters on the dispatch model. Zhao, Fang, and Wei (2019) utilized the schedulability of CSP to integrate it with WP, reduced the output uncertainty, improved the overall regulation efficiency, and proved that the addition of a CSP plant increases the reliability of the renewable energy power generation system. Pousinho et al. (2014) took WP and CSP plants in the Iberian Peninsula as a study case and then studied the short-term coordination of it. The results show that the co-generation system can increase power transmission and increase the profit of power generation enterprises. Li and Wang (2022) and Li et al. (2022) studied the microgrid scheduling method considering demand response and introduced a heuristic algorithm into the application of renewable energy optimal scheduling. Yao et al. (2012) proposed a short-term dispatch model for WP energy storage units in a new energy system, in which the two ESSs alternately use WP to curtail power for energy storage and discharge to the grid so as to maximize the use of wind energy. Li et al. (2021) considered the uncertainty and demand response of renewable energy and proposed a bi-level optimal scheduling method for an integrated energy system. Ma et al. (2021) proposed a regional power grid dispatching model using energy storage to coordinate renewable energy dispatching. The optimization goal is to maximize renewable energy consumption and power delivery and convert this problem into a mixed-integer programing problem to solve. Li et al. (2022) proposed a distributed BESS optimal dispatch model considering peak load transfer, which improves the voltage distribution in the distribution network and significantly improves the system voltage qualification rate. Luo et al. (2018) studied the power and voltage control strategy of the regional power grid with an energy storage system and large-scale new energy access and reduced the bus voltage fluctuation at the new energy access point discrete reactive power compensation strategy. However, few scholars have studied the combined model and CSP plant and energy storage constraints. Lu and Cheng (2021) focused on CSP plants (wind, photovoltaic, battery energy storage, and thermal power plants) and proposed a day-ahead scheduling model for renewable energy power generation systems. It is proved by simulation that the proposed model can reduce the load fluctuation of the renewable energy system and improve the energy storage rate of the system.
Based on the abovementioned analysis, it is of theoretical and practical value to study the wind–solar–thermal-storage combined power generation system combining the CSP plant and ESS in the renewable energy regional power grid to improve the wind–solar absorption capacity, but there are still problems that can be further studied. 1) There is still insufficient research on the joint optimal scheduling model of the ESS and CSP plant; 2) there is a lack of scheduling strategies to analyze the overall system economics of abandoning wind and solar power and thermal power output and rarely fully consider the system voltage deviation.
In summary, this study establishes a joint optimal scheduling model for a regional renewable energy power grid with the ESS and CSP plant, considering the various operating constraints of the CSP plant, ESS, thermal power units, WP, and PV. In order to further improve the solution accuracy of the model, the model is transformed into a mixed-integer linear programming (MLIP) problem to solve. Compared with the traditional scheduling model, the main contributions of our study are
1) We propose a novel optimal scheduling model for the renewable energy regional power grid with the CSP plant and ESS. In the proposed model, the CSP plant and ESS can improve the curtailment of wind and solar power as a flexible resource.
2) Compared with the traditional method, the model proposed in our study increases the WP and PV generation, improves the node voltage of the regional grid system, and reduces the operating cost of the renewable energy regional grid.
The rest of our study is organized as follows. Section 2 describes the renewable energy district grid architecture with the ESS and CSP plant. Section 3 describes the model and establishes an optimal dispatch model for renewable energy regional grids, including objective function, power balance, and constraints of the unit, and in Section 4 we use a numerical study to evaluate the effectiveness of the proposed method, and Section 5 draws conclusions.
2 Renewable Energy Regional Grid Structure With Energy Storage and Concentrated Solar Power Station
The CSP plant mainly comprises the concentrating heat collecting link subsystem, the heat storage link subsystem, and the power generation link subsystem. The solar field absorbs solar energy in the concentrating and heat-collecting link subsystem and further completes the energy transfer in the subsequent links through the heat-conducting working medium and then promotes the heat-conducting medium to enter the power-generating link subsystem and drives the steam turbine to generate electricity through water vapor. In addition, the thermal storage subsystem of the CSP plant can exchange heat for the thermally conductive working medium to realize heat storage and heat release (Chen et al., 2014). Energy storage is comprehensively considered from multiple dimensions such as efficiency, economy, and power quality. Lithium battery energy storage has obvious advantages and broad application prospects compared with other energy storage. Therefore, the framework structure of the renewable energy regional grid with the ESS and CSP plant established in our study is shown in Figure 1.
In Figure 1, the renewable energy regional grid scheduling model with the ESS and CSP plant comprises thermal power units, photovoltaic power generation, wind power generation, CSP plant, and lithium battery ESS. The power-to-heat part in the heat storage link subsystem of the CSP plant can consume part of the output of WP and PV in the form of heat energy. The ESS stores the excess WP and PV output in the energy storage system in the form of chemical energy when the renewable energy output is abundant. When the load is at the peak of electricity consumption, the energy storage in the CSP plant and the ESS can bear more peak regulation pressure for the thermal power unit and improve the flexibility of the regional renewable energy grid.
3 Joint Optimal Dispatch Model of Renewable Energy Regional Power Grid With Energy Storage and Concentrated Solar Power Station
3.1 Objective Function
The objective function of the joint optimal dispatch model proposed in our study is to minimize the cost of power conversion to heat in the CSP plant, the operating cost of thermal power units, and the penalty cost of wind and solar curtailment power. The formula can be expressed in Eq. 1.
where
The wind and solar curtailment power are expressed in Eq. 2.
where
3.2 Constraints
3.2.1 Concentrated Solar Power Plant Operation Constraints
The charging power of the heat storage system in the CSP plant comprises the heating power, WP, and PV electric heating power. The formula can be expressed by Eqs. 3, 4.
where
Moreover, because the heat storage system of the CSP plant cannot be charged and released simultaneously, the formula can be expressed by Eq. 5.
Capacity constraints of the energy storage system of the CSP plant can be within a limited range as shown in Eq. 6.
where
In addition, in order to ensure the response speed in the scheduling process and the life of the heat storage tank, it is necessary to make the heat storage capacity
3.2.2 Energy Storage Operation Constraints
The ESS should be continuously charged and discharged during operation. The charge, discharge, and remaining power of ESS must be greater than or equal to 0 and less than or equal to the maximum capacity. Also, the remaining power at the final moment and the starting moment should be equal. Constraints of the ESS are shown in Eqs. 7–13.
where
Same as the heat storage system of the CSP plant, the charging or discharging behaviors of the ESS cannot be performed at the same time. Also,
3.2.3 Other Constraints
3.2.3.1 System Power Balancing Constraints
The regional grid of renewable energy should ensure the balance constraints of active and reactive power. In the case of ignoring the line loss, each node power transmission to the power grid must be balanced at each operation period as described in Eq. 14.
where
Similarly, the reactive power balance constraint of the system can be expressed in Eq. 15.
where
3.2.3.2 Voltage Deviation Constraints
The node voltage limit constraint of the renewable energy system cannot exceed its allowable maximum and minimum voltage values, and the constraint is shown in Eq. 16.
where
3.2.3.3 Photovoltaic Constraints
The output power of the PV should be guaranteed to be less than the maximum power it can provide. The constraints are shown in Eq. 17.
where
3.2.3.4 Wind Power Constraints
Wind power should be ensured that the output power must be less than the maximum power it can provide. The constraints are shown in Eq. 18.
where
3.2.3.5 Thermal Power Unit Constraints
When the thermal power unit is running, the processing constraints of the thermal power unit are given by Eqs. 19, 20.
where
When the thermal power unit is less than the minimum technical output, its output is
Thermal power unit climbing constraints are given by Eq. 21.
where
Thermal power unit start and stop time constraints are given by Eq. 22
where
The joint optimal scheduling framework of the renewable energy regional grid with the ESS and CSP plant proposed in our study is shown in Figure 2.
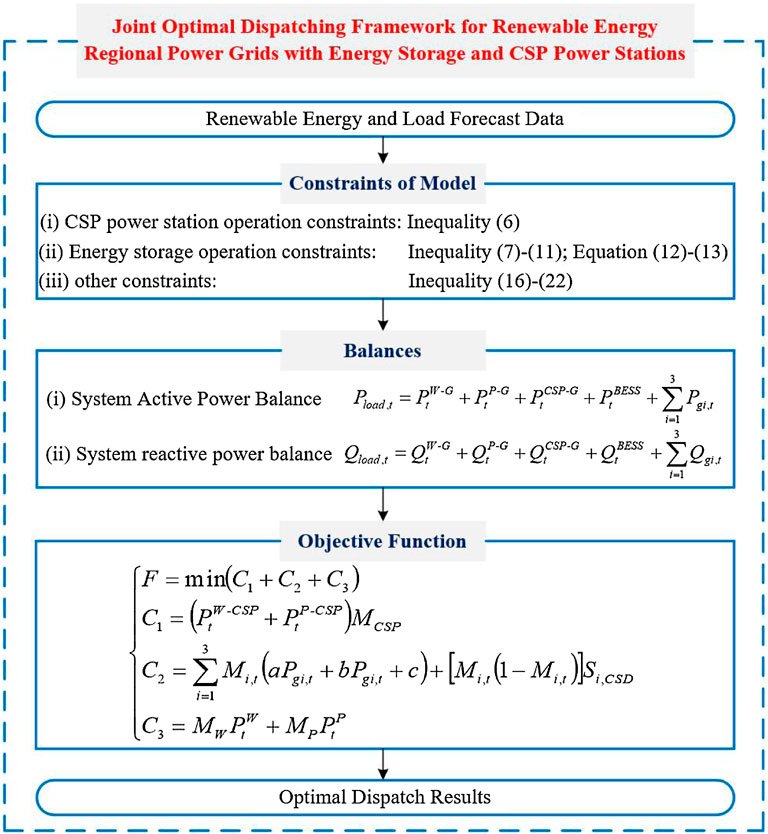
FIGURE 2. Joint optimal scheduling framework of the renewable energy regional grid with the ESS and CSP plant model.
4 Cases Analysis
4.1 Overview of the Cases System
In our study, the modified IEEE9 node system is used to analyze the cases. We use Yalmip to build mathematical models of the joint optimal scheduling of the renewable energy regional grid with the ESS and CSP plant model and the CPLEX commercial solver to solve the optimization function in Matlab (R2018B). The case study is carried out on a configuration environment with i7-11700K CPU and 64 GB RAM. The 9-node model diagram is shown in Figure 3.
In Figure 3, three thermal power units with installed capacities of 250, 190, and 130 MW at nodes 1, 2, and 3, respectively; the ESS with an installed capacity of 10 MW at nodes 4, 5, and 6, respectively; a CSP plant with an installed capacity of 50 MW at node 9; a PV with an installed capacity of 100 MW at node 5; and a WP with an installed capacity of 120 MW at node 4 are shown.
The operating parameters of the thermal power unit are shown in Table 1.
The operation and parameters of the CSP plant are shown in Table 2.
The load predicted power, WP predicted power, PV predicted power, and solar radiation index of the renewable energy regional grid system of a typical day are shown in Figure 4.
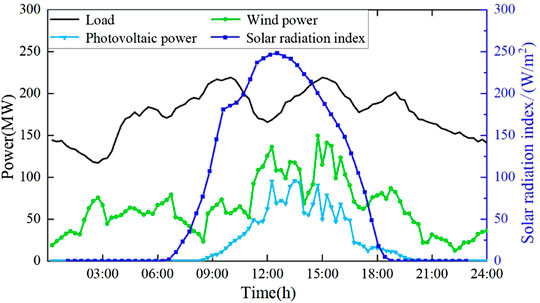
FIGURE 4. Typical daily load, wind power, photovoltaic, and solar radiation index prediction curve. Analysis of the results of the cases
4.2 Analysis of the Results of the Cases
In order to verify the effectiveness of the proposed optimal scheduling model for the renewable energy regional power grid with the ESS and CSP plant, we set the following three models for comparison.
Model 1: The traditional day-ahead scheduling model is adopted for the renewable energy regional power grid, and the joint optimal scheduling of the ESS and CSP plant is not considered.
Model 2: A day-ahead optimal scheduling model considering the ESS is adopted for the renewable energy regional power grid.
Model 3: The scheduling model proposed in this study is adopted for the renewable energy regional power grids, and the joint optimal scheduling of the ESS and CSP plant is considered.
Figure 5A–C show the day-ahead scheduling results of model 1, model 2, and model 3, respectively.
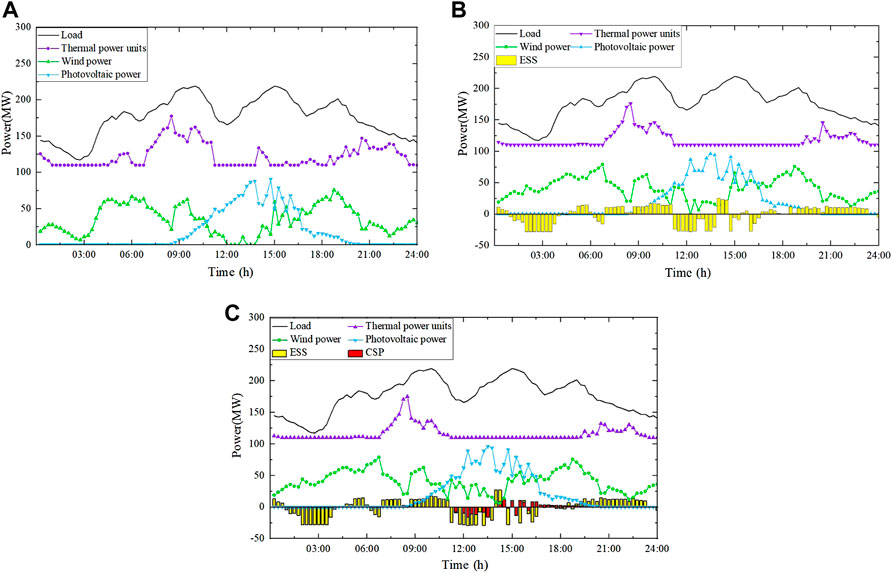
FIGURE 5. The power balance of regional renewable energy grid with models 1,2 and 3 (A) the day-ahead scheduling results of model 1; (B) the day-ahead scheduling results of model 2; (C) the day-ahead scheduling results of model 3.
In Figure 5A, the thermal power generator unit in model 1 undertakes the primary load power consumption of the system. Due to the high output of WP during 00:00–05:00, the load demand during this period is limited. The WP exhibits anti-peak shaving characteristics, which makes the WP consumption of the regional grid of renewable energy insufficient. In addition, the solar radiation index is high during 12:00–16:30, and the PV output is mainly concentrated during this time period, which will also lead to serious PV consumption problems. In Figure 5B, that is, in model 2, the problem of WP and PV absorption in the above two periods in the system is well-solved due to the access of the ESS. The output power of WP and PV increases significantly during the abovementioned period. Also, the discharge of the ESS alleviates the peak of electricity consumption during 19:00–22:00. In Figure 5C, the ESS and CSP plant are used as flexible resources in the regional power grid to further improve the WP and PV consumption problem during the abovementioned period in model 3. Compared with model 1 without the ESS and CSP, model 2 only has the ESS involved in system scheduling. In model 3, due to the coordinated regulation of the ESS and the CPS plant, the power curve of the thermal power unit is smoother, and the primary active power output is maintained during the off-peak period.
Figure 6 shows the active output power curves of the ESS and CSP plant in model 2 and model 3.
In Figure 6, the ESS in model 2 and model 3 is charged more and less discharged during 00:00–05:00, absorbing the curtailed wind generated by less load in the renewable energy system. More charging and less discharging during 12:00–14:30 can absorb the solar curtailment power caused by the high solar radiation index and intense solar power, among which the radiation index is high during this period. Also, the CSP plant passes electricity to heat during this period, by converting the wind and solar curtailment power in the regional power grid into heat for storage, consuming more abandoned energy with the ESS, and improving the utilization rate of renewable energy. Furthermore, during 14:30–20:00, the electric energy is provided during the peak load period to cooperate with the ESS and CSP plant to relieve the peak regulation pressure of the thermal power unit. During the rest period, the charging and discharging of the ESS is alternately performed to play its role in the renewable energy regional power grid regulating effect.
Figures 7–9 are the power comparison diagram and the abandoned solar and power wind power comparison diagram of the models 1, 2, and 3 thermal power units, respectively.
In Figures 7–9, compared with model 1 and model 2, in model 3, due to the coordinated regulation of the ESS and CPS plant, the thermal power unit has the least output. The proposed scheduling model alleviates the peak load regulation pressure of thermal power units obviously. In addition, the improvement effect of wind and solar curtailment power is most obvious in Figure 8 and Figure 9. During 00:00–05:00 and 12:00–17:00, the wind curtailment power decreased significantly, and the absorption effect of solar curtailment power is remarkable during 12:00–14:30.
Further analysis is carried out on node voltage during the peak period of the renewable energy regional power grid. Because during 20:00 is the peak load period, the voltage drop of the system point node is relatively significant, so this time is selected as a typical time. Then, we set three cases to study the role of the ESS and CSP plant in voltage support of the renewable energy regional power grid. Figure 10 is the node voltage distribution map at typical times.
In the analysis of Figure 10, the per-unit value of all node voltages is in the range of 0.95–1 p.u. When the ESS is connected to model 2, due to the voltage regulation effect of the ESS system, the system node voltage is slightly improved. In model 3, because the CSP plant provides both active and reactive power to the system, the voltage at node 6 and node 9 improves obviously. In summary, the proposed model can effectively avoid the voltage drop problem and improve the voltage quality of the renewable energy regional power grid.
Table 3 shows the output cost of thermal power units, the penalty cost of curtailing solar and wind power, and the total rate of wind and solar curtailment under three models.
In Table 3, compared with model 1, model 3 reduces the thermal power unit output cost from 8.6506 × 105 (CNY) to 8.2333 × 105 (CNY) and reduces the wind and solar curtailment power penalty cost from 1.0671 × 106 (CNY) to 4.2604 × 104 (CNY). In addition, the wind curtailment rate is reduced from 0.2294% to 0.0973%, and the solar curtailment rate is reduced from 0.0421% to 0.0033%. From the abovementioned cases, we found that the model which we proposed can effectively reduce the operating cost and increase the new energy consumption of the renewable energy regional power grid.
5 Conclusion
This study proposes a joint optimal scheduling model for the regional power grid with the ESS and CSP plant, which considers the constraints of renewable energy operation, power balance, voltage deviation, and other constraints in the renewable energy regional power grid. Through the case analysis, the following conclusions are drawn:
1) The proposed scheduling model can fully utilize the energy time-shift characteristics of the ESS and CSP plant and also improves the flexibility of the ESS and CSP plant by absorbing wind and solar power generation and alleviating the peak load regulation pressure of thermal power units. Moreover, this significantly reduces the operating cost of the regional power grid.
2) The proposed scheduling model reduces the system solar curtailment power rate of the traditional scheduling model from 0.2294% to 0.0973%. The system wind curtailment power rate ranges from 0.0421% to 0.0033%. Also, the unit voltage of the system node is maintained above 0.98 p.u. at the peak period of electricity consumption, which improves the voltage quality of the system.
The construction cost of the electricity-to-heat transfer part in the CSP plant is not considered in our research, so the application value of the model proposed in our study in areas with low wind and solar curtailment power needs further consideration. In future research, we consider further increasing the research on the uncertainty of power supply and load, consider the impact of user demand response on renewable energy systems, and study the specific implementation plan of the CSP plant electric heating part.
Data Availability Statement
The raw data supporting the conclusions of this article will be made available by the authors, without undue reservation.
Author Contributions
YD: conceptualization, methodology, and writing—review and editing. ZH: investigation, formal analysis, software, and data curation. XL: validation and software. SM: supervision and financial support. FG and WL: writing–review and editing.
Funding
This work was supported by the Liaoning Electric Power Co., Ltd. Science and Technology Project of State Grid under Grant No.2021YF-81, Liaoning BaiQianWan Talents Program.
Conflict of Interest
Author ZH was employed by State Grid Liaoning Electric Power Supply Co. Ltd.
The remaining authors declare that the research was conducted in the absence of any commercial or financial relationships that could be construed as a potential conflict of interest.
Publisher’s Note
All claims expressed in this article are solely those of the authors and do not necessarily represent those of their affiliated organizations, or those of the publisher, the editors, and the reviewers. Any product that may be evaluated in this article, or claim that may be made by its manufacturer, is not guaranteed or endorsed by the publisher.
References
Chang, P., Tang, Y., Huang, Y., Zhao, J., Li, C., and Yuan, C. (2019). Optimal Operation of Concentrating Solar Power Station in Power System with High Penetration of Photovoltaic Generation, in 2019 IEEE PES Asia-Pacific Power and Energy Engineering Conference (APPEEC), Macao, China: IEEE, 1–6. doi:10.1109/APPEEC45492.2019.8994347
Chen, R., Sun, H., Guo, Q., Li, Z., Deng, T., Wu, W., et al. (2015). Reducing Generation Uncertainty by Integrating CSP with Wind Power: An Adaptive Robust Optimization-Based Analysis. IEEE Trans. Sustain. Energy 6 (2), 583–594. doi:10.1109/TSTE.2015.2396971
Chen, R., Sun, H., Li, Z., and Liu, Y. (2014). Grid Dispatch Model and Interconnection Benefit Analysis of Concentrating Solar Power Plants with Thermal Storage. Automation Electr. Power Syst. 38 (19), 1–7. doi:10.7500/AEPS20140313001
Deng, X., Huang, Y., Chen, Y., Chen, C., Yang, L., Gao, Q., et al. (2021). Multi-scenario Physical Energy Storage Planning of Integrated Energy Systems Considering Dynamic Characteristics of Heating Network and Demand Response. Front. Energy Res. 9, 588. doi:10.3389/fenrg.2021.783588
Dong, H., Li, S., Dong, H., Tian, Z., and Hillmansen, S. (2020). Coordinated Scheduling Strategy for Distributed Generation Considering Uncertainties in Smart Grids. IEEE ACCESS 8 (8), 86171–86179. doi:10.1109/ACCESS.2020.2992342
Dong, H., Yun, Y., and Ma, Z. (2020). Low-carbon Optimal Operation of Integrated Energy System Considering Multienergy Conversion and Concentrating Solar Power Plant Participation. Power Syst. Technol. 44 (10), 3689–3700. doi:10.13335/j.1000-3673.pst.2020.0449
Du, E., Zhang, N., Hodge, B.-M., Wang, Q., Lu, Z., Kang, C., et al. (2019). Operation of a High Renewable Penetrated Power System with CSP Plants: A Look-Ahead Stochastic Unit Commitment Model. IEEE Trans. Power Syst. 34 (1), 140–151. doi:10.1109/TPWRS.2018.2866486
Du, E., Zhang, N., Kang, C., and Miao, M. (2016). Reviews and Prospects of the Operation and Planning Optimization for Grid Integrated Concentrating Solar Power. Proc. CSEE 36 (21), 5765–5775. doi:10.13334/j.0258-8013.pcsee.161251
Heptonstall, P. J., and Gross, R. J. K., (2021). A Systematic Review of the Costs and Impacts of Integrating Variable Renewables into Power Grids. Nat. Energy 6, 72–83. doi:10.1038/s41560-020-00695-4
Jelley, N., and Smith, T. (2015). Concentrated Solar Power: Recent Developments and Future Challenges. Proc. Institution Mech. Eng. Part A J. Power Energy 229 (7), 693–713. doi:10.1177/0957650914566895
Li, X., Hui, D., and Lai, X. (2013). Battery Energy Storage Station (BESS)-based Smoothing Control of Photovoltaic (PV) and Wind Power Generation Fluctuations. IEEE Trans. Sustain. Energy 4 (2), 464–473. doi:10.1109/TSTE.2013.2247428
Li, X., Ma, R., Gan, W., and Yan, S. (2022). Optimal Dispatch for Battery Energy Storage Station in Distribution Network Considering Voltage Distribution Improvement and Peak Load Shifting. J. Mod. Power Syst. Clean Energy 10 (1), 131–139. doi:10.35833/MPCE.2020.000183
Li, X., Wang, S., and Wang, S. (2019). A Review on Energy Management, Operation Control and Application Methods for Grid Battery Energy Storage Systems. Csee Jpes 7 (5), 1026–1040. doi:10.17775/CSEEJPES.2019.00160
Li, Y., Han, M., Yang, Z., and Li, G. (2021). Coordinating Flexible Demand Response and Renewable Uncertainties for Scheduling of Community Integrated Energy Systems with an Electric Vehicle Charging Station: A Bi-level Approach. IEEE Trans. Sustain. Energy 12 (4), 2321–2331. doi:10.1109/TSTE.2021.3090463
Li, Y., Li, J., and Wang, Y. (2022). Privacy-preserving Spatiotemporal Scenario Generation of Renewable Energies: A Federated Deep Generative Learning Approach. IEEE Trans. Ind. Inf. 18 (4), 2310–2320. doi:10.1109/TII.2021.3098259
Li, Y., Li, K., Yang, Z., Yu, Y., Xu, R., and Yang, M. (2022). Stochastic Optimal Scheduling of Demand Response-Enabled Microgrids with Renewable Generations: An Analytical-Heuristic Approach. J. Clean. Prod. 330, 129840. doi:10.1016/j.jclepro.2021.129840
Li, Y., Wang, C., and Li, G. (2020). A Mini-Review on High-Penetration Renewable Integration into a Smarter Grid. Front. Energy Res. 8, 84. doi:10.3389/fenrg.2020.00084
Li, Y., Wang, R., and Yang, Z. (2022). Optimal Scheduling of Isolated Microgrids Using Automated Reinforcement Learning-Based Multi-Period Forecasting. IEEE Trans. Sustain. Energy 13 (1), 159–169. doi:10.1109/TSTE.2021.3105529
Lu, X., and Cheng, L. (2021). Day-ahead Scheduling for Renewable Energy Generation Systems Considering Concentrating Solar Power Plants. Math. Problems Eng. 2021, 1–14. doi:10.1155/2021/9488222
Luo, J., Zhou, B., Zhang, Y., and Ai, X. (2018). Joint Control Strategy Study of Active Power and Voltage in Regional Power Network Considering Energy Storage. Mod. Electr. Power 35 (6), 1–8. doi:10.19725/j.cnki.1007-2322.2018.06.001
Lv, X., Li, D., Wang, J., and Gong, C. (2021). “Auxiliary Service Strategy Model of Power Plant Participating in Peak Load Regulation,” in 3rd International conference on air pollution and environmental engineering,(Electr network IOP). 631, 012092, doi:10.1088/1755-1315/631/1/012092
Ma, R., Li, X., Li, W., Gao, D., Zhang, J., and Song, N. (2021). Cooperative Scheduling Strategy of Energy Storage Systems for Regional Grid Supplied by Renewable Energy. Power Gener. Technol. 42 (1), 31–39. doi:10.12096/j.2096-4528.pgt.20027
Mehigan, L., DeaneÓ, J. P., Gallachóir, B. P., and Bertsch, V. (2018). A Review of the Role of Distributed Generation (DG) in Future Electricity Systems. Energy 163 (15), 822–836. doi:10.1016/j.energy.2018.08.022
Pousinho, H. M. I, Mendes, V. M. F, CollaresPereira, M, and Pereira Cabrita, C (2014). Self-scheduling for Energy and Spinning Reserve of Wind/CSP Plants by a MILP Approach. Energy 78, 524–534. doi:10.1016/j.energy.2014.10.039
Wang, X., Gu, L., and Liang, D. (2021). Decentralized and Multi-Objective Coordinated Optimization of Hybrid AC/DC Flexible Distribution Networks. Front. Energy Res. 9, 423. doi:10.3389/fenrg.2021.762423
Xu, T., and Zhang, N. (2016). Coordinated Operation of Concentrated Solar Power and Wind Resources for the Provision of Energy and Reserve Services. IEEE Trans. Power Syst. 32 (2), 1. doi:10.1109/TPWRS.2016.2571561
Yao, D. L., Choi, S. S., Tseng, K. J., and Lie, T. T. (2012). Determination of Short-Term Power Dispatch Schedule for a Wind Farm Incorporated with Dual-Battery Energy Storage Scheme. IEEE Trans. Sustain. Energy 3 (1), 74–84. doi:10.1109/TSTE.2011.2163092
Zhang, X., Dong, X., and Li, X. (2021). Study of China's Optimal Concentrated Solar Power Development Path to 2050. Front. Energy Res. 9. doi:10.3389/fenrg.2021.724021
Keywords: renewable energy, joint optimal scheduling strategy, energy storage system, concentrated solar power plant, wind and solar curtailment
Citation: Dong Y, Han Z, Li X, Ma S, Gao F and Li W (2022) Joint Optimal Scheduling of Renewable Energy Regional Power Grid With Energy Storage System and Concentrated Solar Power Plant. Front. Energy Res. 10:941074. doi: 10.3389/fenrg.2022.941074
Received: 11 May 2022; Accepted: 24 May 2022;
Published: 11 July 2022.
Edited by:
Yang Li, Northeast Electric Power University, ChinaReviewed by:
Jun Yin, North China University of Water Resources and Electric Power, ChinaChen Liang, Nanjing University of Information Science and Technology, China
Sumei Liu, Beijing Forestry University, China
Copyright © 2022 Dong, Han, Li, Ma, Gao and Li. This is an open-access article distributed under the terms of the Creative Commons Attribution License (CC BY). The use, distribution or reproduction in other forums is permitted, provided the original author(s) and the copyright owner(s) are credited and that the original publication in this journal is cited, in accordance with accepted academic practice. No use, distribution or reproduction is permitted which does not comply with these terms.
*Correspondence: Shaohua Ma, mash_dq@sut.edu.cn