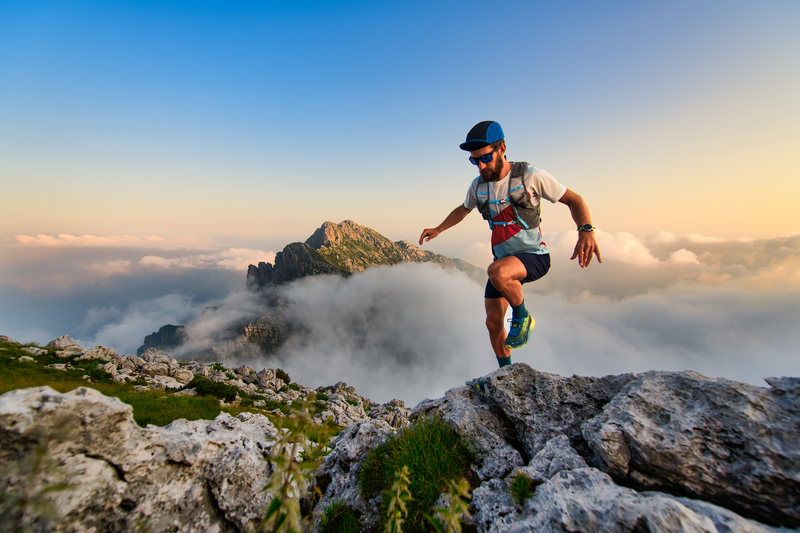
94% of researchers rate our articles as excellent or good
Learn more about the work of our research integrity team to safeguard the quality of each article we publish.
Find out more
ORIGINAL RESEARCH article
Front. Energy Res. , 07 July 2022
Sec. Smart Grids
Volume 10 - 2022 | https://doi.org/10.3389/fenrg.2022.919197
This article is part of the Research Topic Source-Grid-Load-Storage Collaborative and Interactive Optimization Control Technology of New Types of Active Distribution Network View all 11 articles
Multiple 5G base stations (BSs) equipped with distributed photovoltaic (PV) generation devices and energy storage (ES) units participate in active distribution network (ADN) demand response (DR), which is expected to be the best way to reduce the energy cost of 5G BSs and provide flexibility resources for the ADN. However, the standalone PV-integrated 5G BS has the characteristics of wide distribution, small volume, and large load fluctuations, which will bring strong uncertainty to the ADN by directly participating in the DR. Therefore, a system architecture for multiple PV-integrated 5G BSs to participate in the DR is proposed, where an energy aggregator is introduced to effectively aggregate the PV energy and ES resources of 5G BSs. Then, a two-stage optimal dispatch method is proposed. Specifically, in the large-timescale DR planning stage, an incentive mechanism for multiple PV-integrated 5G BSs to participate in the DR is constructed based on the contract theory, which ensures that multiple 5G BSs respond to and satisfy the peak-shaving demand of the ADN. In the small-timescale online energy optimization stage, based on the energy sharing mode among 5G BSs, a Lyapunov-based online energy optimization algorithm is proposed to optimize the shared energy flow between the internal layer and the interactive layer of 5G BSs, which further improves PV absorption and ensures operation stability of ES in the 5G BS. Simulation results show that the proposed two-stage optimal dispatch method can effectively encourage multiple 5G BSs to participate in DR and achieve the win–win effect of assisting the ADN peak-shaving and low-carbon economic operation of 5G BSs.
The explosive growth of mobile data and the popularization of smart devices have accelerated the deployment of fifth-generation (5G) communication systems (Singh et al., 2020). However, while ensuring wide network coverage and high communication service quality, the high-power consumption characteristic of 5G base stations (BSs) not only imposes high electricity bills for communication operators but also exacerbates the non-negligible carbon emission problem (Piovesan et al., 2019). On the one hand, 5G BSs are equipped with 64/32-channel massive multiple-input multiple-output (MIMO) to realize high bandwidth and high communication traffic (Fu et al., 2019; Chih -Lin et al., 2020; Zhou et al., 2021), which causes the power consumption of a 5G BS to be 2–3 times higher than that of an ordinary 4G BS. On the other hand, the deployment density of 5G BSs is much higher than that of 4G BSs as 5G uses higher-frequency bands for communication (Han et al., 2021). Moreover, the standard 5G BS demands a power of more than 11.5kW (Israr et al., 2021), and the number of 5G BSs in China is expected to reach 13.1 million by 2025, with a total power consumption of 200 billion kWh. Therefore, the high-power consumption characteristic of 5G BSs has become the primary constraint to the ultra-dense network deployment in the 5G era, and it also impacts the stable operation of an active distribution network (ADN) (Pedram and Wang, 2019).
At present, powering BSs through distributed energy resources (DERs), such as photovoltaic (PV) generation and energy storage (ES), has become a common solution to reduce on-grid power consumption and build low-carbon wireless networks (Zhou et al., 2017; Hu et al., 2020). Although the introduction of DER devices increases the investment cost of communication operators, the cheapness and cleanliness of PV energy will greatly reduce the energy cost of BSs, and the spatio-temporal shifting characteristic of ES can further improve the absorption capacity of renewable energy. Moreover, 5G BSs are already equipped with battery backups during construction to ensure stable operation in case of power supply interruptions, which can be considered the inherent ES (Yong et al., 2021a; Tang et al., 2021). However, under the normal power supply status, large-scale distributed ESs in 5G BSs are always idle (Liao et al., 2020a; Ci et al., 2020). Therefore, on the basis of ensuring the uninterrupted power supply of the 5G BSs, if the energy source of the ES can be dispatched, the energy consumption flexibility of 5G BSs will be stimulated, and the high asset utilization of ES resources can be realized.
With the continuous transition from the traditional grid to smart grid (SG) and the widespread deployment of user-side smart meters (Khan and Jayaweera, 2019), PV-integrated 5G BSs participating in ADN demand response (DR) has become an irreplaceable way to reduce energy cost (Li et al., 2021). On the premise of ensuring the reliability of the 5G BS power supply, if the ES and PV resources in 5G BSs can be coordinated, more flexible dispatchable resources can be tapped to provide a powerful guarantee for the real-time balance of the source and load in the ADN. Moreover, communication operators can reduce electricity bills or obtain DR subsidy based on the time-of-use (TOU) tariff mechanism by fully exploiting the power utilization flexibility of PV-integrated 5G BSs and fully dispatching idle ES resources, thus reducing the energy cost of a 5G communication network (Yong et al., 2021b). Despite the advantages mentioned previously, the multiple PV-integrated 5G BSs participating in DR still confront several critical challenges, which are summarized as follows.
1) Because of the different spatio-temporal distributions of communication services (Huq et al., 2020) and light intensity, different degrees of power imbalance will occur in different PV-integrated 5G BSs, which increases the difficulty of 5G BS energy dispatch in the ADN. In addition, as 5G BSs belong to communication operators, the direct energy dispatch of 5G BSs by the ADN is difficult to guarantee the communication service quality of telecom users and involves user security and privacy leakage issues.
2) The participation of multiple 5G BSs in the DR increases the operation and maintenance cost of ESs and impairs the participation enthusiasm of communication operators. Meanwhile, the power supply demands of 5G BSs with different spatio-temporal distributions vary greatly, and the randomness of PV energy and BS load further exacerbates the uncertainty of ES dispatchable capacity. How to effectively encourage and quantify the participation of 5G BSs in DR is an important problem to be solved.
3) During the participation of multiple 5G BSs in the DR, the imbalance between PV generation and load demand will lead to an over-discharge of ESs or the curtailment of PV energy. In addition, the accurate prediction of PV generation and load demand increases the difficulty of ensuring the real-time energy balance and puts higher requirements on real-time energy scheduling decisions (Wang et al., 2020). How to manage the energy of 5G BSs participating in DR is another challenge.
Multiple 5G BSs can be incentivized to participate in the DR through energy aggregators. It was pointed out that energy aggregators can aggregate BSs into a limited number of groups and act as third parties to dispatch BSs to participate in the DR (Xu et al., 2015). By introducing energy aggregators, encouraging and quantifying the participation of 5G BSs in the DR can be modeled as a social welfare maximization problem based on the contract theory, that is, maximizing the total utility of energy aggregators and BSs. A contract theory–based direct trading framework was proposed by Zhang et al. (2016) to address the lack of enthusiasm for direct trading between small-scale electricity suppliers and electricity consumers. Given the low enthusiasm of electric vehicles to participate in the DR, a contract theory–based charging rate allocation criterion and access control scheme were proposed by Zhang et al. (2018). However, the application of the contract theory can only ensure that multiple 5G BSs participate in the DR regularly and quantitatively in a large timescale but cannot guarantee the low-carbon and stable operation of 5G BSs in a small timescale.
The energy optimization of 5G BSs aims to alleviate the imbalance between PV generation and load demand by fully utilizing renewable energy, thereby reducing the energy cost and maintaining the stability of ES. An energy sharing model with energy aggregators as physical carriers was proposed by Guo et al. (2014), which allowed BSs to coordinate with each other for addressing energy imbalance by simultaneously transmitting and receiving energy to and from energy aggregators. Considering the unpredictability characteristics of the shared energy of 5G BSs, the Lyapunov optimization algorithm can be used to optimize the energy sharing among BSs online and in real-time. Under the energy sharing mode, Liu et al. (2017) proposed an online energy management method based on Lyapunov optimization, aiming to fully absorb renewable energy and control the energy flow of the nano-grid group in real-time. Zhong et al. (2019) proposed an online Lyapunov optimization–based control algorithm to optimize the shared energy in real-time in the multi-user–shared centralized ES system.
As the construction of 5G BSs is still in its infancy, research on the optimal energy dispatch of multiple PV-integrated 5G BSs participating in the DR is still relatively lacking. Motivated by the aforementioned challenges, we propose a two-stage optimal dispatch method for multiple PV-integrated 5G BSs participating in the ADN DR to fully dispatch PV and ES resources by combining Lyapunov optimization and contract theory. The main contributions of this study are summarized as follows.
1) The system architecture of multiple PV-integrated 5G BSs participating in the ADN DR is proposed, where the energy aggregator is introduced as the third party to motivate and assist the PV-integrated 5G BSs to participate in the DR.
2) A contract theory–based DR incentive mechanism is proposed to guide the discharge behavior of 5G BSs with different discharge capacities in the large-timescale DR planning stage. The peak-shaving demand of the ADN and the profit of BSs can be satisfied by optimizing the contract items.
3) A Lyapunov-based online energy optimization algorithm is proposed to make real-time decisions on the shared energy of multiple 5G BSs in the small timescale. The complex energy sharing problem is transformed into a linear programing problem, and only real-time information is required to make online decisions.
The remainder of this study is organized as follows. Section 2 introduces the proposed system architecture and methodology of multiple PV-integrated 5G BSs participating in the ADN DR. Section 3 elaborates the proposed contract theory–based large-timescale DR planning mechanism. In Section 4, the small-timescale online energy optimization algorithm for multiple PV-integrated 5G BSs based on Lyapunov optimization is proposed. Section 5 analyzes the numerical results, followed by the conclusion in Section 6.
The system architecture of multiple PV-integrated 5G BSs participating in the ADN DR is shown in Figure 1, which consists of a 5G communication network, an ADN, and an energy aggregator. The 5G communication network comprises
1) Information flow interaction: in the DR planning stage, the incentive price signal of the aggregator and DR results of 5G BSs can be shared through two-way communication links. In the online energy optimization stage, the energy sharing requirements and results of 5G BSs also can be shared through two-way communication links.
2) Energy flow interaction: with the assistance of the aggregator, 5G BSs can be used as response loads or sources. Specifically, 5G BSs can serve as typical prosumers of the ADN, which can both generate and consume electricity.
In this study, we explore how the aggregator encourages and assists multiple 5G BSs to participate in the ADN DR during peak load periods. Since the DR during peak load periods and the real-time energy management of 5G BSs are optimization problems on different timescales, the participation of 5G BSs in the DR includes two stages, that is, DR planning and online energy optimization. As shown in Figure 2, we utilize a multi-timescale model (Yu et al., 2021) to divide the peak load period into
In the DR planning stage, a contract theory–based large-timescale DR planning method is proposed, which takes the peak-shaving demand of the ADN as the constraint and maximizes social welfare to obtain the optimal discharge power of 5G BSs participating in the DR. Specifically, as the DR agent, the aggregator needs to coordinate multiple 5G BSs with discharge capacity to provide peak-shaving services during the peak load period. Because of the differences in power consumption, PV energy, and the state of charge (SoC) of ES, 5G BSs show different discharge capacities. Therefore, the aggregator will design different contract items for BSs with different discharge capacities to encourage them to respond to the peak-shaving demand of the ADN. Each contract item stipulates the relationship between the discharge power and reward. Each BS will select a contract item matching its type to provide stable power to obtain the best utility. The details of the contract design and optimization will be introduced in Section 3.
In the online energy optimization stage, we focus on the impact of the real-time imbalance between the PV energy and load demand of BSs participating in the DR on the stable operation of ES. In the small timescale, the PV energy is first used to supply the load demand of the BS. When the PV energy is balanced with the load demand, the ES only needs to stably participate in the DR according to the contract item. When the PV energy is more than the load demand, surplus PV energy will be generated, which will affect the stable output of the ES and may cause PV energy abandonment. When the PV energy is less than the load demand, a load gap will be generated. To satisfy the communication reliability requirements, the ES needs to respond to the demands of the ADN while balancing the BS load gap, leading to over-discharge of ES. In addition, since the PV energy and load demand of BSs are usually independent, some BSs may lack sufficient PV energy to satisfy the load demand, while other BSs may abandon surplus PV energy because of the inability of complete absorption. Therefore, the aggregator is introduced as a shared energy carrier to support energy sharing among multiple 5G BSs, and a small-timescale online energy optimization algorithm is proposed to optimize the imbalance energy between the PV energy and load demand of multiple 5G BSs. The specific procedures will be introduced in Section 4.
In this study, we assume that the BS with more remaining ES energy and lower load demand has a larger discharge capacity and is more willing to participate in the DR to obtain higher rewards. For the aggregator, BSs can be classified into different types based on the discharge capacity. Assuming that in the
The discharge capacity of a BS is determined by historical statistics of the source-load imbalance energy and ES energy in the first time slot of the
where
Therefore, the BS type of
Considering the information security and privacy, the BS type of a specific BS is unknown to the aggregator, which means that the information is asymmetric (Zhou et al., 2019a). We assume that the aggregator can learn that there are a total of
The aggregator can design a contract including
The principle of the aggregator contract design and BS contract signing is to maximize their own utility. Considering there are
where
The utility of the type
where
According to the principle of contract design and signing, the problem of incentivizing 5G BSs to participate in the DR is transformed into the social welfare maximization problem. The expected social welfare is defined as the total utility of the aggregator and
The aggregator faces three constraints from the BS side when optimizing the contract design, namely, the individual rationality (IR) constraint, the incentive compatibility (IC) constraint, and the monotonicity constraint (Chen and Zhu, 2017). The IR constraint means that the BSs should receive non-negative utility after signing the contract and participating in the DR. The IC constraint means that a BS can only obtain maximum utility when signing the contract item designed for its own type. The monotonicity constraint means that the higher the BS type, the higher the reward will be. Therefore, the social welfare maximization problem is formulated as
where
The formulated optimization problem includes
The utility of the type
where the first inequality satisfies the IC constraint, the second inequality satisfies the BS type definition, and the third inequality satisfies the IR constraint. Combining the first and third inequalities, we can draw that if the type
We consider three adjacent BS types, that is,
Based on
Combining (9) and (11), it can be further concluded as
Therefore, we can derive that all IC constraints hold if the IC constraint between the adjacent types holds. Through IC constraint dimensionality reduction, the number of IC constraints will be reduced from
Based on the aforementioned analysis,
By checking the Hessian matrix, it can be known that the objective function of
where
By replacing
Then, the iteration is terminated and the contract design is completed. The discharge power and corresponding reward of 5G BSs participating in the DR can be obtained based on the contract.
On the basis of obtaining the optimal discharge power of 5G BSs participating in the DR, we analyze the energy flow of BSs in the small timescale and propose the energy sharing strategy among multiple 5G BSs to further reduce the energy cost of the 5G communication network. Specifically, we classify the BSs into energy output BSs and energy input BSs, where energy output BSs share energy to absorb the surplus PV energy and energy input BSs receive the shared energy to balance the load gap. We define
where
1) For
2) For
Therefore, in the process of energy sharing,
The set of shared energy of BSs in time slot
where
Without the energy sharing mode, the set of discharge energy of ESs in time slot
where
The energy level of ES can be regarded as an ES queue, the length of which represents the remaining energy of the ES. We define the set of ES queues in time slot
The reason for ensuring the stability of ES queues during 5G BSs participating in the DR is that the frequent and excessive discharge of ESs will seriously shorten the life span and increase the maintenance cost of ES.
The objective of the online energy optimization problem is to realize the full absorption of PV energy by energy sharing among 5G BSs, while ensuring the stability of ES queues. The PV absorption rate is denoted as the percentage of PV energy that can be absorbed by 5G BSs, which is given by
where
The focus of online energy optimization is formulated to improve the PV absorption rate during the whole peak load period to minimize the energy cost of multiple 5G BSs participating in the DR by real-time decision-making on the shared energy among BSs, which can be expressed as
As
The Lyapunov function is defined as
As (25) is a maximization problem, we define the drift-minus-reward (Li et al., 2020) to maximize the PV absorption rate while ensuring the queue stability, which is given by
where
where
Proof: see Supplementary material.
Therefore,
Since
In this section, we validate the performance of the proposed two-stage optimal dispatch method by simulations. We consider a scenario in which the aggregator signs the DR contract with 10 5G BSs during the peak load period. The BSs provide peak-shaving service for the ADN from 10:00 a.m. to 11:00 a.m. The feed-in tariff is CNY 0.42/ kWh, and the peak-shaving demand is 37.5 kW. The case analysis uses 1 min as the small timescale and 1 h as the large timescale. The minimum load demand is 2.193 kW when the BS is in a no-load operation state, and the maximum load demand is 10 kW when the BS is in a full-load operation state (China Mobile Research Institute, 2020). Considering that 10 5G BSs are geographically close to each other, we assume that the PV output of each BS adopts the same data. The typical PV output curve of 5G BSs from 10:00 a.m. to 11:00 a.m. is shown in Figure 4, and the simulation parameter settings of the DERs in 5G BSs are shown in Table 1. Moreover, since the daily dynamic load data of 5G BSs are still undisclosed, we assume that the load demand of 10 5G BSs obeys the random distribution within the load demand interval (2.193 kW, 10 kW).
According to the initial ES capacity of the 5G BSs, the minute-level power consumption, and the PV output curve, the optimal contract can be obtained, and the corresponding parameters are shown in Table 2. The results show that the response power coincides with the discharge capacity, which implies that the 5G BSs with different discharge capacities choose the contract items that conform to their own types. The optimal discharge power is provided to obtain the optimal reward. The utilities of BSs and the aggregator increase with the BS type, which indicates that the aggregator prefers to sign the contract with BSs with higher discharge capacity. Moreover, we can find that the sum of the response power is equal to 37.5 kW, which accurately satisfies the peak-shaving demand of the ADN.
The optimal discharge power and discharge reward versus the BS type are shown in Figure 5A. It can be seen that the optimal discharge power and discharge reward increase monotonously with the BS type, indicating that the contract satisfies the monotonicity constraint, and the discharge capabilities of BSs are well-exploited. Figure 5B shows the utility of 5G BSs versus the type of the contract item. It can be seen that maximum utility can be achieved only when the 5G BS signs the contract item conforming to its type, indicating that the contract satisfies the IC constraint. Moreover, all 5G BSs that sign the contract can obtain non-negative utility, which is consistent with the IR constraint. In addition, the utility of the 5G BS is proportional to the BS type, which will increase the motivation of the higher type of 5G BS to sign the contract and participate in the DR.
Figure 6 shows the comparison of ES SoC with and without energy sharing. Simulation results show that 5G BSs participating in the DR without energy sharing will lead to an over-discharge or insufficient dispatching of ESs. At the end of DR, the SoCs of BS1 and BS2 are lower than the minimum capacity limit, while those of BS6 and BS7 still have enough dispatchable space. By contrast, the proposed Lyapunov-based online energy optimization algorithm with energy sharing can achieve higher discharge stability and a more balanced power level of ESs.
Figure 7 shows the shared energy and source-load imbalance energy of 10 5G BSs during the DR period. The red curve and the blue cylinder represent the shared energy optimized by the proposed online energy optimization algorithm and the imbalance energy, respectively. When the red curve is tangential to the blue cylinder, it means that the shared energy completely compensates for the imbalance energy. At this point, the ES unit is in a stable output state and only needs to output constant power based on the contract item. From 10:00 a.m. to 11:00 a.m., most BSs have surplus PV energy due to sufficient sunlight, and only a few BSs with high power consumption require shared energy. Based on the proposed online energy optimization algorithm, it can be seen that most BSs can interact with each other to compensate for the imbalance in energy through energy sharing, which further promotes PV energy consumption, reduces the curtailment of PV output, and ensures the output stability of ES units.
Table 3 shows the comparison of the PV absorption rate before and after the two-stage optimal dispatch. Compared with not participating in the DR, 5G BSs participating in the DR can improve the PV absorption rate by 17.47% and make it up to 99.87%. The reason is that the contract-based DR planning ensures rough absorption of the overall PV output during the entire peak load period, while the Lyapunov-based online energy optimization algorithm ensures the approximately complete and real-time absorption of the PV output, thus realizing the maximum absorption of PV energy during the entire peak load period.
In this study, we propose a two-stage optimal dispatch method based on the contract theory and Lyapunov optimization for the typical scenario of multiple PV-integrated 5G BSs participating in the ADN DR. The following conclusions are obtained:
1) The system architecture for multiple PV-integrated 5G BS participating in the ADN DR was proposed, which is composed of aggregators, a 5G communication network, and an ADN.
2) A contract theory–based large-timescale DR planning method was proposed for modeling the interaction between 5G BSs and the aggregator. Simulation results show that the proposed method can improve the motivation of 5G BSs to participate in the DR and satisfy the peak-shaving demand of the ADN.
3) A Lyapunov-based small-timescale online energy optimization algorithm was proposed for making real-time decisions on energy sharing of 5G BSs. The numerical results show that the proposed method can improve the PV absorption rate up to 99.87%, which achieves approximately complete absorption of PV energy and ensures the stability of the ES queue.
Further research will be conducted in three directions, including:
1) Implementation of energy dispatch for large-scale 5G BSs at multiple timescales including day-ahead, intra-day, and real-time and the systematical evaluation of the respond capacity of a PV-integrated 5G BS for ancillary services.
2) Energy sharing scheme and a demand response incentive mechanism that take into account power transmission losses.
3) Multi-entity benefit allocation method for participating in the DR under deregulated power markets.
The original contributions presented in the study are included in the article/Supplementary Material; further inquiries can be directed to the corresponding author.
XZ: conceptualization, methodology, software, investigation, formal analysis, and writing—original draft; ZAW: data curation and writing—original draft; ZZ: visualization and writing—original draft; HL: conceptualization, supervision, and writing—review and editing; XM: conceptualization, supervision, and writing—review and editing; XY: visualization and writing—review and editing GL: resources, supervision, and writing—review and editing; ZOW: visualization and writing—review and editing; ZL: visualization and writing—review and editing; YL: resources and supervision.
Authors are grateful to the financial support from Science and Technology Project of State Grid Corporation of China (KJ21-1-56).
XY, GL, ZOW, ZL, and YL were employed by Information and Communication Company, State Grid Tianjin Electric Power Company. The remaining authors declare that the research was conducted in the absence of any commercial or financial relationships that could be construed as a potential conflict of interest.
All claims expressed in this article are solely those of the authors and do not necessarily represent those of their affiliated organizations, or those of the publisher, the editors, and the reviewers. Any product that may be evaluated in this article, or claim that may be made by its manufacturer, is not guaranteed or endorsed by the publisher.
Proof of (27):
Thus, we can have (28)
The proof is completed.
Chen, J., and Zhu, Q. (2017). Security as a Service for Cloud-Enabled Internet of Controlled Things under Advanced Persistent Threats: A Contract Design Approach. IEEE Trans.Inform.Forensic Secur. 12 (11), 2736–2750. doi:10.1109/tifs.2017.2718489
Chih-Lin, I., Han, S., and Bian, S. (2020). Energy-efficient 5G for a Greener Future. Nat. Electron 3 (4), 182–184. doi:10.1038/s41928-020-0404-1
China Mobile Research Institute (2020). 5G Telecom Power Target Network White Paper 2020. Available: https://max.book118.com/html/2020/0913/8105107075002142.shtm (updated September 14, 2020).
Ci, S., Zhou, Y., Xu, Y., Diao, X., and Wang, J. (2020). Building a Cloud-Based Energy Storage System through Digital Transformation of Distributed Backup Battery in Mobile Base Stations. China Commun. 17 (4), 42–50. doi:10.23919/jcc.2020.04.005
Dragicevic, T., Pandzic, H., Skrlec, D., Kuzle, I., Guerrero, J. M., and Kirschen, D. S. (2014). Capacity Optimization of Renewable Energy Sources and Battery Storage in an Autonomous Telecommunication Facility. IEEE Trans. Sustain. Energy 5 (4), 1367–1378. doi:10.1109/tste.2014.2316480
Fu, S., Su, Z., Jia, Y., Zhou, H., Jin, Y., Ren, J., et al. (2019). Interference Cooperation via Distributed Game in 5G Networks. IEEE Internet Things J. 6 (1), 311–320. doi:10.1109/jiot.2017.2743116
Guo, Y., Xu, J., Duan, L., and Zhang, R. (2014). Joint Energy and Spectrum Cooperation for Cellular Communication Systems. IEEE Trans. Commun. 62 (10), 3678–3691. doi:10.1109/tcomm.2014.2353632
Han, J., Liu, N., Huang, Y., and Zhou, Z. (2021). Collaborative Optimization of Distribution Network and 5G Mobile Network with Renewable Energy Sources in Smart Grid. Int. J. Electr. Power & Energy Syst. 130, 107027. doi:10.1016/j.ijepes.2021.107027
Hu, S., Chen, X., Ni, W., Wang, X., and Hossain, E. (2020). Modeling and Analysis of Energy Harvesting and Smart Grid-Powered Wireless Communication Networks: A Contemporary Survey. IEEE Trans. Green Commun. Netw. 4 (2), 461–496. doi:10.1109/tgcn.2020.2988270
Huq, K., Mumtaz, S., Zhou, Z., Chandra, K., Otung, I., and Rodriguez, J. (2020). “Energy-efficiency Maximization for D2D-Enabled UAV-Aided 5G Networks,” in 2020 IEEE International Conference on Communications, Dublin, Ireland, 7-11 June 2020, 1–6. doi:10.1109/icc40277.2020.9149150
Israr, A., Yang, Q., Li, W., and Zomaya, A. Y. (2021). Renewable Energy Powered Sustainable 5G Network Infrastructure: Opportunities, Challenges and Perspectives. J. Netw. Comput. Appl. 175, 102910. doi:10.1016/j.jnca.2020.102910
Khan, Z. A., and Jayaweera, D. (2019). Smart Meter Data Based Load Forecasting and Demand Side Management in Distribution Networks with Embedded PV Systems. IEEE Access 8, 2631–2644. doi:10.1109/ACCESS.2019.2962150
Li, K., Yan, J., Hu, L., Wang, F., and Zhang, N. (2021). Two-stage Decoupled Estimation Approach of Aggregated Baseline Load under High Penetration of Behind-The-Meter PV System. IEEE Trans. Smart Grid 12 (6), 4876–4885. doi:10.1109/tsg.2021.3105747
Li, P., Sheng, W., Duan, Q., Li, Z., Zhu, C., and Zhang, X. (2020). A Lyapunov Optimization-Based Energy Management Strategy for Energy Hub with Energy Router. IEEE Trans. Smart Grid 11 (6), 4860–4870. doi:10.1109/tsg.2020.2968747
Liao, H., Zhou, Z., Ai, B., and Guizani, M. (2020). “Learning-based Energy-Efficient Channel Selection for Edge Computing-Empowered Cognitive Machine-To-Machine Communications,” in 2020 IEEE 91st Vehicular Technology Conference (VTC2020-Spring),, Antwerp, Belgium, 25-28 May 2020, 1–6. doi:10.1109/vtc2020-spring48590.2020.9128780
Liao, H., Zhou, Z., Zhao, X., Zhang, L., Mumtaz, S., Jolfaei, A., et al. (2020). Learning-based Context-Aware Resource Allocation for Edge-Computing-Empowered Industrial IoT. IEEE Internet Things J. 7 (5), 4260–4277. doi:10.1109/jiot.2019.2963371
Liu, N., Yu, X., Fan, W., Hu, C., Rui, T., Chen, Q., et al. (2017). Online Energy Sharing for Nanogrid Clusters: A Lyapunov Optimization Approach. IEEE Trans. Smart Grid 9 (5), 4624–4636. doi:10.1109/TSG.2017.2665634
Lu, X., Li, K., Xu, H., Wang, F., Zhou, Z., and Zhang, Y. (2020). Fundamentals and Business Model for Resource Aggregator of Demand Response in Electricity Markets. Energy 204, 117885. doi:10.1016/j.energy.2020.117885
Pedram, M., and Wang, L. (2019). Energy Efficiency in 5G Cellular Network Systems. IEEE Des. Test. 37 (1), 64–78. doi:10.1109/MDAT.2019.2960342
Piovesan, N., Temesgene, D. A., Miozzo, M., and Dini, P. (2019). Joint Load Control and Energy Sharing for Autonomous Operation of 5G Mobile Networks in Micro-grids. IEEE Access 7, 31140–31150. doi:10.1109/access.2019.2903499
Singh, R., Sicker, D., and Huq, K. (2020). “MOTH- Mobility-Induced Outages in THz: A beyond 5G (B5G) Application,” in 2020 IEEE 17th Annual Consumer Communications & Networking Conference (NV, USA: Las Vegas), 1–9. doi:10.1109/ccnc46108.2020.9045401
Tang, G., Yuan, H., Guo, D., Wu, K., and Wang, Y. (2021). “Reusing Backup Batteries as BESS for Power Demand Reshaping in 5G and beyond,” in IEEE INFOCOM 2021-IEEE Conference on Computer Communications, Vancouver, BC, Canada, 10-13 May 2021, 1–10. doi:10.1109/infocom42981.2021.9488760
Wang, F., Li, K., Liu, C., Mi, Z., Shafie-Khah, M., and Catalao, J. P. S. (2018). Synchronous Pattern Matching Principle-Based Residential Demand Response Baseline Estimation: Mechanism Analysis and Approach Description. IEEE Trans. Smart Grid 9 (6), 6972–6985. doi:10.1109/tsg.2018.2824842
Wang, F., Xuan, Z., Zhen, Z., Li, K., Wang, T., and Shi, M. (2020). A Day-Ahead PV Power Forecasting Method Based on LSTM-RNN Model and Time Correlation Modification under Partial Daily Pattern Prediction Framework. Energy Convers. Manag. 212, 112766. doi:10.1016/j.enconman.2020.112766
Wei, H., Xiao, L., Li, Y., and Zhou, S. (2016). “Queue-aware Energy-Efficient Scheduling and Power Allocation in Small-Cell Networks with Interference,” in 2016 IEEE Wireless Communications and Networking Conference (Doha, Qatar: IEEE), 1–6. doi:10.1109/wcnc.2016.7565102
Xu, J., Duan, L., and Zhang, R. (2015). Cost-aware Green Cellular Networks with Energy and Communication Cooperation. IEEE Commun. Mag. 53 (5), 257–263. doi:10.1109/mcom.2015.7105673
Yong, P., Zhang, N., Liu, Y., Hou, Q., Li, Y., and Kang, C. (2021). Exploring the Cellular Base Station Dispatch Potential towards Power System Frequency Regulation. IEEE Trans. Power Syst. 37 (1), 820–823. doi:10.1109/TPWRS.2021.3124141
Yong, P., Zhang, N., Hou, Q., Liu, Y., Teng, F., Ci, S., et al. (2021). Evaluating the Dispatchable Capacity of Base Station Backup Batteries in Distribution Networks. IEEE Trans. Smart Grid 12 (5), 3966–3979. doi:10.1109/tsg.2021.3074754
Yu, H., Zhou, Z., Jia, Z., Zhao, X., Zhang, L., and Wang, X. (2021). Multi-timescale Multi-Dimension Resource Allocation for NOMA-Edge Computing-Based Power IoT with Massive Connectivity. IEEE Trans. Green Commun. Netw. 5 (3), 1101–1113. doi:10.1109/tgcn.2021.3076582
Zhang, B., Jiang, C., Yu, J-L., and Han, Z. (2016). A Contract Game for Direct Energy Trading in Smart Grid. IEEE Trans. Smart Grid 9 (4), 2873–2884. doi:10.1109/TSG.2016.2622743
Zhang, K., Mao, Y., Leng, S., He, Y., Maharjan, S., Gjessing, S., et al. (2018). Optimal Charging Schemes for Electric Vehicles in Smart Grid: A Contract Theoretic Approach. IEEE Trans. Intell. Transp. Syst. 19 (9), 3046–3058. doi:10.1109/tits.2018.2841965
Zhong, W., Xie, K., Liu, Y., Yang, C., Xie, S., and Zhang, Y. (2019). Online Control and Near-Optimal Algorithm for Distributed Energy Storage Sharing in Smart Grid. IEEE Trans. Smart Grid 11 (3), 2552–2562. doi:10.1109/TSG.2019.2957426
Zhou, C., Feng, C., and Wang, Y. (2021). Spatial-temporal Energy Management of Base Stations in Cellular Networks. IEEE Internet Things J. 99, 1. doi:10.1109/jiot.2021.3121325
Zhou, Z., Guo, Y., He, Y., Zhao, X., and Bazzi, W. M. (2019). Access Control and Resource Allocation for M2M Communications in Industrial Automation. IEEE Trans. Ind. Inf. 15 (5), 3093–3103. doi:10.1109/tii.2019.2903100
Zhou, Z., Liao, H., Zhao, X., Ai, B., and Guizani, M. (2019). Reliable Task Offloading for Vehicular Fog Computing under Information Asymmetry and Information Uncertainty. IEEE Trans. Veh. Technol. 68 (9), 8322–8335. doi:10.1109/tvt.2019.2926732
Zhou, Z., Sun, C., Shi, R., Chang, Z., Zhou, S., and Li, Y. (2017). Robust Energy Scheduling in Vehicle-To-Grid Networks. IEEE Netw. 31 (2), 30–37. doi:10.1109/mnet.2017.1600220nm
Keywords: multiple PV-integrated 5G BSs, active distribution network, demand response, Lyapunov optimization, energy sharing
Citation: Zhang X, Wang Z, Zhou Z, Liao H, Ma X, Yin X, Lv G, Wang Z, Lu Z and Liu Y (2022) Optimal Dispatch of Multiple Photovoltaic Integrated 5G Base Stations for Active Distribution Network Demand Response. Front. Energy Res. 10:919197. doi: 10.3389/fenrg.2022.919197
Received: 13 April 2022; Accepted: 11 May 2022;
Published: 07 July 2022.
Edited by:
Tianguang Lu, Shandong University, ChinaReviewed by:
Bingchen Wang, ASML (United States), United StatesCopyright © 2022 Zhang, Wang, Zhou, Liao, Ma, Yin, Lv, Wang, Lu and Liu. This is an open-access article distributed under the terms of the Creative Commons Attribution License (CC BY). The use, distribution or reproduction in other forums is permitted, provided the original author(s) and the copyright owner(s) are credited and that the original publication in this journal is cited, in accordance with accepted academic practice. No use, distribution or reproduction is permitted which does not comply with these terms.
*Correspondence: Zhenyu Zhou, emhlbnl1X3pob3VAbmNlcHUuZWR1LmNu
Disclaimer: All claims expressed in this article are solely those of the authors and do not necessarily represent those of their affiliated organizations, or those of the publisher, the editors and the reviewers. Any product that may be evaluated in this article or claim that may be made by its manufacturer is not guaranteed or endorsed by the publisher.
Research integrity at Frontiers
Learn more about the work of our research integrity team to safeguard the quality of each article we publish.