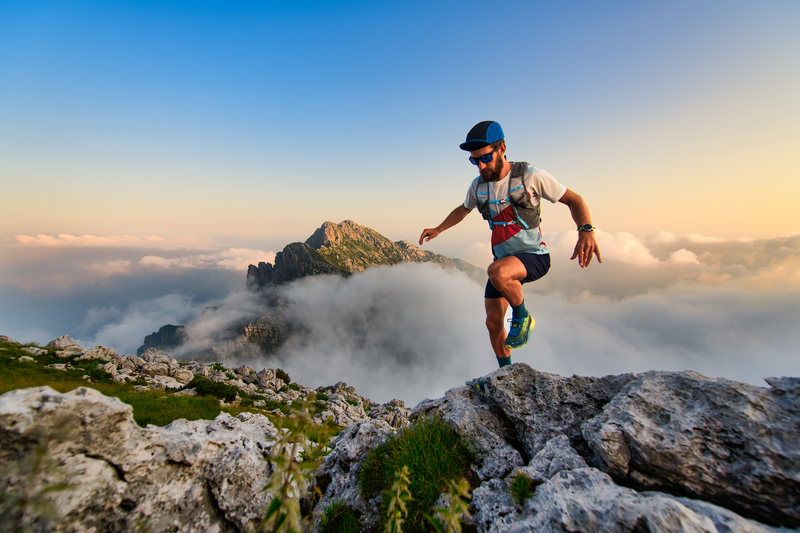
95% of researchers rate our articles as excellent or good
Learn more about the work of our research integrity team to safeguard the quality of each article we publish.
Find out more
OPINION article
Front. Energy Res. , 05 May 2022
Sec. Smart Grids
Volume 10 - 2022 | https://doi.org/10.3389/fenrg.2022.907027
This article is part of the Research Topic Advanced AI Applications for Modelling, Optimization, Control, and Planning of Smart Grid View all 39 articles
Excessive use of energy makes environmental problems increasingly prominent and gradually affects people’s production and life. Therefore, wind energy, solar energy, and other renewable new energy sources are gradually rising in the application of the power system, but these new energy sources are vulnerable to the environment, and the operation stability is poor, which seriously reduces the power quality, and cannot work well with the power grid, which restricts its development (Tu et al., 2018; Ren et al., 2020; Guo et al., 2021). With the overall improvement of family living standards, electric vehicles, electricity replacement, smart homes, and the popularity of new energy equipment, power load also presents rapid growth. Effective load monitoring can provide timely feedback on residents’ electricity situation, and then the network regulation to save electricity can effectively reduce the waste of energy (Zhang et al., 2019; Luo et al., 2020; Zheng et al., 2020). As an efficient and practical tool, artificial intelligence plays an important role in constructing the global perception of terminal electrical quantity feature recognition (Gao et al., 2018; Chahla et al., 2019; Liang et al., 2019). At present, many scholars conduct non-invasive load research through artificial intelligence-related technologies and use cloud terminals to build a cloud network of the power system. The overall steps can be seen in Figure 1. However, the practical application of the global perception of terminal power consumption will face many challenges. First, the current method used is only suitable for high-frequency sampling data, with high hardware requirements for the acquisition device. Second, intra-class differences between loads lead to insufficient generalization ability of existing load labels, and non-invasive load identification across datasets and regions is still difficult to achieve ideal results. This study clarifies the above issues and brings some views on different electrical feature identifications.
The terminal power global perception of electrical quantity feature identification mainly includes a non-invasive terminal, low-voltage intelligent switch, home energy router, and intelligent interactive terminal (Rousseeuw and Hubert, 2018; Wang et al., 2020). Non-invasive terminal identification is the core technology of electrical feature identification. By analyzing the electrical parameters such as voltage and current at the measurement point, the user’s total load is decomposed, as well as the use status of individual electrical equipment, energy consumption, and other energy consumption information. Therefore, accurate identification of load is the core problem of electrical feature identification. Cui et al. (2022) adopted image recognition technology. In order to prevent the identification of electrical equipment with a single feature as a label, due to the lack of information, a non-invasive load classification and recognition method is proposed based on time-series two-dimensional visualization and transfer learning. In addition to the use of image recognition technology, to improve the edge terminal distribution data identification analysis ability through analyzing distribution data, extraction from multiple angles distribution data flow features, and using the K-means clustering method to meet the requirements of distribution data flow identification, scholars put forward a marginal terminal based on the characteristics of the distribution data flow protocol identification method (Huang et al., 2021). In the same clustering method, starting from the steady-state current analysis of home electrical load, Zhang (2021) proposed an identification method based on wavelet packet energy characteristics, improved the BP neural network, made wavelet packet decomposition of the steady-state current signal, extracted the high- and low-frequency characteristics of signals, and made the load characteristics more accurate. The neural network can play a good role in identifying problems. Bi et al. (2021), based on power sliding window event detection method, deep belief network load identification method, and load identification, realized electricity time and electricity consumption behavior load classification calculation analysis. Compared with the traditional intelligent algorithm, the method has higher accuracy and can identify the accurate load online. A different neural network can play a different effect. Different from the traditional random initialization convolutional neural network of the kernel learning method, Li (2021a) put forward an improved neural network recognition model to deconvolution feature extraction technology for electrical equipment fusion image feature mapping matrix extraction. The trained model can improve the recognition accuracy of electrical equipment to realize the intelligent recognition of equipment. In terms of deep learning, the classic deep learning model-LSTM network is also used for load decomposition. In order to better judge the current moment load state, Liu (2021) introduced the attention mechanism and bidirectional network to establish three improved models. Furthermore, for the LSTM network, Huang’s study (2021) is based on the NILM technology for low-frequency datasets. For the problem of too few low-frequency sampling features, a load decomposition algorithm based on the combination of LSTM and attention mechanism is proposed to input the segmented power sequence into the trained RNN network and use the attention mechanism to focus on the corresponding input near the key data, to improve the training speed and accuracy. In view of the existing non-invasive load monitoring algorithm, including weak generalization ability of download monitoring in different scenarios, insufficient simultaneous action processing ability of multiple loads, and lack of external information utilization, a non-invasive load monitoring method based on an intelligent decision support system is proposed. Human–computer interaction using integrated components of the decision support system gives load monitoring higher flexibility and scalability (Zhu, 2018).
For the power grid, the terminal power consumption global perception of electrical quantity feature identification is mainly used to prevent the occurrence of hazards and provide visualization and detectable convenience for the power industry. In the construction of terminal global perception, the industrial load is also important, and the industrial load data record important information such as the user’s power consumption mode and electricity demand level. However, it will lead to outliers doping in the recording data due to interference. The identification and correction method for power load outlier of industrial users is put forward, which improves the accuracy of prediction data. It can also be used to analyze the power consumption law of large industrial users, which helps build a global perception system with a prediction function (Zhao et al., 2017). Electrical feature identification of terminal electricity global perception also plays an important role in the power market, based on the probability prediction of abnormal online real-time identification method, using variable Bayes inference for probability prediction mode training, so as to realize the online identification of abnormal data (Liu et al., 2021). In terms of fault detection, for the intelligent demand for fine load data and refined power behavior mining technology, Yi (2021) put forward the multi-target particle group algorithm non-invasive load identification method, accurate identification with non-invasive load monitoring, using BP neural network training to get each load switching probability distribution curve, correct the identification results, accurately identify common household electrical faults, and prevent disasters. Global perception has an important role in fire prevention, which utlizes the historical data collected by sensors for fusion processing and calculation. K-means, an unsupervised learning clustering algorithm based on machine learning is used to cluster the historical data of equipment according to the industry. Moment is used to compare the difference between prediction features and monitoring features to judge whether the electrical equipment works abnormally (Li, 2021b). Finally, network security is also extremely important. As the main national power transmission network, it cannot be attacked by criminals. Therefore, combined with machine learning, the security detection technology for different power grid embedded terminals is proposed to realize the comprehensive security detection of the power grid embedded terminals (Zhang, 2020).
Combination of artificial intelligence and electrical features of terminal global perception technology can realize clear image access, automatically identify the distribution of load, realize real-time storage and update of data, AI intelligent analysis forecast danger, realize the perception of power grid operation situation, and grid emergency dispatching disposal, combined with the distribution network terminal and cloud platform, jointly realize the security of terminal electricity. In terms of the application of the global perception of terminal electricity consumption, the safety prevention of electrical appliances, the accurate charging of the power market, and the security protection of the power network have been studied by different scholars. However, there are still many directions to be improved in the research of terminal power global perception technology of artificial intelligence and electrical quantity feature identification.
At present, the proposed method is only applicable to high-frequency sampling data, which requires high hardware requirements of the acquisition device, so it is very important to improve the ability of the acquisition device. Within-class differences between loads lead to insufficient generalization ability of existing load labels, and non-invasive load identification across datasets and regions is still difficult to achieve ideal results. It is still difficult to design representative polymorphic models for multi-state loads. Therefore, how to reduce the application cost while ensuring the accuracy of load identification and how to select suitable load labels to minimize the difference in similar loads and maximize the difference in different loads will be the core of the future non-intrusive load identification research.
ZT: writing the original draft and editing. BL: visualization and contribution to the discussion of the topic. AW: supervising.
The authors declare that the research was conducted in the absence of any commercial or financial relationships that could be construed as a potential conflict of interest.
This work was supported by the research and demonstration on key technologies of safe, smart distribution and utilization in the tourism area based on the industrial internet (No. GZKJXM20210350).
ZT and BL are employed by Electric Power Research Institute of Guizhou Power Grid Co., Ltd. AW is employed by Guigang Power Supply Bureau of Guangxi Power Grid Co., Ltd.
The authors declare that the research was conducted in the absence of any commercial or financial relationships that could be construed as a potential conflict of interest.
All claims expressed in this article are solely those of the authors and do not necessarily represent those of their affiliated organizations, or those of the publisher, the editors, and the reviewers. Any product that may be evaluated in this article, or claim that may be made by its manufacturer, is not guaranteed or endorsed by the publisher.
Bi, S., Qi, M., and Yu, H. (2021). Research on the Application of Artificial Intelligence Technology in Electricity Consumption Behavior Analysis. Electron. Des. Eng. 29 (1), 147–151.
Chahla, C., Snoussi, H., and Merghem, X. (2019). A Novel Approach for Anomaly Detection in Power Consumption Data. Prague, Czech Republic: 8th International Conference on Pattern Recognition Applications and Methods.
Cui, H., Wu, Y., and Jiang, Y. (2022). Current Sequence Visualization Method of Non-intrusive Load Recognition. Electric Power Automation Equipment. doi:10.16081/j.epae.202203002
Gao, J., Kang, D., and Lei, X. (2018). Identification of Bad Data in Power System Based on Improved Fuzzy Clustering Analysis. Electr. Automation 40 (5), 30–33.
Guo, H., Lu, J., and Yang, P. (2021). Review on Key Techniques of Non-intrusive Load Monitoring. Electric Power Automation Equipment 41 (1), 135–146.
Huang, G., Liang, G., and Yi, J. (2021). Protocol Identification Method of Power Distribution Edge Computing Terminal Based on Data Flow Characteristics. Electric Eng. 22, 123–126.
Huang, X. (2021). Non-intrusive Load Association Decomposition Technology for Energy Router. doi:10.27061/d.cnki.ghgdu.2021.001455
Li, Y. (2021). Research on Key Technology of Electrical Fire Detection System Based on Data Fusion Technology. doi:10.27730/d.cnki.ghngy.2021.000342
Li, Z. (2021). Electrical Equipment Identification and Fault Early Warning Based on Machine Learning and Big Data Technology. doi:10.27139/d.cnki.ghbdu.2021.000320
Liang, X., Zhao, B., Ma, Q., Sun, B., and Cui, B. (2019). Terminal Access Data Anomaly Detection Based on Random forest for Power User Electric Energy Data Acquisition System. Int. Conf. Adv. Inf. Networking Appl., 166–175. doi:10.1007/978-3-030-15032-7_14
Liu, X., Tang, Y., and Lu, J. (2021). Online Real Time Anomaly Recognition Method for Power Consumption of Electric Energy Data Acquisition Terminal Based on Probability Prediction. Power Syst. Prot. Control. 49 (19), 99–106.
Liu, Z. (2021). Research on Non-intrusive Residential Load Disaggregation Method Based on Deep Learning. doi:10.27623/d.cnki.gzkyu.2021.001900
Luo, J., Wang, W., and Wang, Z. (2020). Surface Electromyography Classification Method Based on Temporal Two Dimensionalization and Convolution Feature Fusion. Pattern Recognition Artif. Intelligence 33 (7), 588–599.
Ren, H., Hou, Z. B., and Vyakaranam, B. (2020). Power System Event Classification and Localization Using a Convolutional Neural Network. Front. Energ. Res. 8, 1–11. doi:10.3389/fenrg.2020.607826
Rousseeuw, P. J., and Hubert, M. (2018). Anomaly Detection by Robust Statistics. Wires Data Mining Knowl Discov. 8 (2), 1236–1250. doi:10.1002/widm.1236
Tu, J., Zhou, M., and Song, X. (2018). Comparison of Supervised Learning-Based Non-intrusive Load Monitoring Algorithms. Electric Power Automation Equipment 38 (12), 128–134.
Wang, F., Liu, W., and Chen, X. (2020). Abnormal Data Identification of Synchronous Line Loss Based on the Approximate equality of Rank Sum. Trans. China Electrotechnical Soc. 35 (22), 4771–4783.
Yi, L. (2021). Research on Non-invasive Load Feature Extraction and Identification Algorithm. doi:10.27139/d.cnki.ghbdu.2021.000210
Zhang, C. (2021). Research and Application of Non-intrusive Household Electrical Application Load Identification Method. doi:10.27272/d.cnki.gshdu.2021.004622
Zhang, N., Dai, H., and Hu, Z. (2019). A Source-Grid-Load Coordinated Planning Model Considering System Flexibility Constraints and Demand Response. Electric Power 52 (2), 61–69.
Zhang, T. (2020). Research on Application of Artificial Intelligence in Grid Embedded Terminal Security Detection. doi:10.27461/d.cnki.gzjdx.2020.000742
Zhao, T., Wang, J., and Ma, L. (2017). Outlier Detection and Correction Method for Industrial Loads Based on Nonparametric Regression Analysis. Automation Electric Power Syst. 41 (18), 53–59.
Zheng, S., Liang, Q., and Peng, X. (2020). Fuzzy Clustering Based Abnormal Power Consumption Behavior Detection on Resident Level. Electr. Meas. Instrumentation 57 (19), 40–44.
Keywords: global perception, artificial intelligence, feature identification, neural network, electrical features
Citation: Tan Z, Liu B and Wu A (2022) Artificial Intelligence and Feature Identification Based Global Perception of Power Consumer: Definition, Structure, and Applications. Front. Energy Res. 10:907027. doi: 10.3389/fenrg.2022.907027
Received: 29 March 2022; Accepted: 06 April 2022;
Published: 05 May 2022.
Edited by:
Bo Yang, Kunming University of Science and Technology, ChinaReviewed by:
Yixuan Chen, The University of Hong Kong, Hong Kong SAR, ChinaCopyright © 2022 Tan, Liu and Wu. This is an open-access article distributed under the terms of the Creative Commons Attribution License (CC BY). The use, distribution or reproduction in other forums is permitted, provided the original author(s) and the copyright owner(s) are credited and that the original publication in this journal is cited, in accordance with accepted academic practice. No use, distribution or reproduction is permitted which does not comply with these terms.
*Correspondence: Bin Liu, MjMyMjE1NzgxNEBxcS5jb20=
Disclaimer: All claims expressed in this article are solely those of the authors and do not necessarily represent those of their affiliated organizations, or those of the publisher, the editors and the reviewers. Any product that may be evaluated in this article or claim that may be made by its manufacturer is not guaranteed or endorsed by the publisher.
Research integrity at Frontiers
Learn more about the work of our research integrity team to safeguard the quality of each article we publish.