- 1School of Information Management, Shanghai Lixin University of Accounting and Finance, Shanghai, China
- 2Faculty of Business Information, Shanghai Business School, Shanghai, China
- 3School of Law, Yunnan University, Kunming, China
With the imminent threat of the energy crises, innovation in energy technologies is happening world-wide. The aim is to reduce our reliance on fossil fuels. Electric vehicles with fuel-cells that use hydrogen as an energy carrier are touted to be one of the most important potential replacements of the gasoline vehicle in both future transportation scenarios and emerging smart energy grids. However, hydrogen storage is a major technical barrier that lies between where we are now and the mass application of hydrogen energy. Further exploration of onboard hydrogen storage systems (OHSS) is urgently needed and, in this regard, a comprehensive technology opportunity analysis will help. Hence, with this research, we drew on scientific papers and patents related to OHSS and developed a novel methodology for investigating the past, present, and future development trends in OHSS. Specifically, we constructed a heterogeneous knowledge network using a unique multi-component structure with three core components: hydrogen carriers, hydrogen storage materials, and fuel cells. From this network, we extracted both the developed and underdeveloped technological solutions in the field and applied a well-designed evaluation system and prediction model to score the future development potential of these technological solutions. What emerged was the most promising directions of research in the short, medium, and long term. The results show that our methodology can effectively identify technology opportunities in OHSS, along with providing valuable decision support to researchers and enterprise managers associated with the development and application of OHSS.
1 Introduction
The imminent energy crisis and growing energy consumption is not only causing an unprecedented demand for reducing our reliance on fossil fuels, it is also prompting us to urgently explore new and sustainable energy carriers. Future energy carriers have to be efficient, clean, economical, and safe, and should allow for high volumetric and gravimetric storage densities (Abe et al., 2019; Tarhan and Çil, 2021). Hydrogen appears to be a very promising energy storage medium that may be a substitute for oil and other fossil fuels, and hydrogen fuel cells for vehicles is just one of its remarkable applications. Although electric vehicles are already considered a green solution in transportation scenario, vehicles equipped with a hydrogen fuel cell still outperform electric vehicle in several respects (Shi et al., 2019). First, most electrical power is generated by fossil fuels, while hydrogen is a zero emissions energy source. This means it can increase the environmental performance of power systems (Jannati and Nazarpour, 2018). Second, electric vehicles suffer from peak-time problems with the power grid, whereas hydrogen fuel cells have no such issues. However, there are two main barriers that lie between where we are now and the mass application of hydrogen energy. These are the ability to safely store hydrogen in sufficient quantities and devising the proper storage conditions for the energy produced.
Over the last 2 decades, research activities and innovation in the field of hydrogen storage has been driven by attempts to establish onboard hydrogen storage systems (OHSS) for cars powered by hydrogen fuel cells (Mori and Hirose, 2009; Weidenthaler and Felderhoff, 2011; Hwang and Varma, 2014). With the help of these researchers, hydrogen fuel cell vehicles have been put into small scale production (Nechaev et al., 2017). Although the market for mobile hydrogen energy storage continues to grow, further R&D efforts are still needed to achieve the desired energy and cost efficiency goals (Cumalioglu et al., 2008). Additionally, other aspects of OHSS, such as storage capacity, refilling times, and lifecycles, also need to be improved (Li M et al., 2019; Hassan et al., 2021). To actively respond to these changing technological requirements, we must understand the evolution and current state of technological development, explore new technological opportunities and research directions. Only this way will we further advance the globe’s transition towards low-carbon energy and sustainable development (Aaldering et al., 2019).
Previous research sheds light on knowledge recombination as the theoretical foundation for the analysis of technological evolution and opportunities. It is widely known that innovation often originates from combining existing scientific and technological knowledge—commonly called knowledge recombination (Edquist, 1997; Xiao et al., 2021). By recombining existing knowledge elements in new ways, or finding new relationships between already-combined elements, new knowledge can be generated. In fact, knowledge recombination is often considered to be a “shortcut” to higher-level technologies that comes at a low switching cost (Frenken et al., 2012). As Antonelli et al. (2010) points out, the countries that achieve higher growth rates in productivity are usually those that have a superior ability to recombine technologies so as to generate new technological knowledge. Several scholars highlight that the existing knowledge surrounding a technology greatly affects where efforts toward innovation should be allocated, as well as which technologies are likely to be implemented in future practice (Wu and Shanley, 2009; Roper and Hewitt-Dundas, 2015). In this regard, several hydrogen storage technology areas are reviewed and analyzed based on the perspective of knowledge recombination. In recent years, some review researches of hydrogen storage technology have been published, covering storage options (Elberry et al., 2021), materials and process design (Chanchetti et al., 2016; Turani-I-Belloto et al., 2021), energy carriers (Aziz, 2021) and fuel cells (Eriksson and Gray, 2017; Gong and Verstraete, 2017). Most studies have future-oriented feature, and have proved helpful to investigate development trends of hydrogen storage technology. Thus, understanding the knowledge existing in the field of OHSS is critically important when exploring future technological opportunities.
Technological knowledge can mainly be found in the science and technology (S&T) literature, i.e., scientific papers and patents (Li X et al., 2019). Using S&T information as data sources to obtain research opportunities seems a good way, especially in the energy storage field. Investigation shows that over 35% of technology opportunity analysis articles in energy storage technology are based on extant knowledge stock. Fundamental research in science is recorded by scientific papers, which act like seeds for the development of new technologies (Shibata et al., 2010). In previous studies, scientific data has been used as a data resource for recognizing research patterns (Ma et al., 2015), mining technical intelligence (Zhang et al., 2014), and monitoring technology development trends (Bakhtin et al., 2017). Patents, in addition to providing protection for inventions, are regarded as an up-to-date and reliable source of information reflecting technological advances and inventive activities (Kim and Lee, 2015). Surveys have shown that, patents contain up to 80% of the latest global technical information (Asche, 2017). For these reasons, patent data have been widely used to forecast technological innovation pathways (Zhou et al., 2018), formulate technology strategies (Yu and Zhang, 2019), analyze the interdisciplinarity of technology fusion (Ko et al., 2014), and visualize knowledge ecosystems (Schillaci et al., 2021).
Many empirical studies have indicated that the interdependence and interactions between science and technology have been increasing (Wang et al., 2015). Vast quantities of the latest technical information have been recorded and shared in scientific papers, which indicates that technological development is increasingly relying on science (Brandl, 1998). In fact, the progress of science is essentially exogenous, and technological advances are the result of R&D (Liu et al., 2018). This means technological application usually fall slightly behind scientific breakthroughs. Consequently, analyzing the gaps between scientific research and patents is an effective way of understanding the trends in technology innovation and can offer significant support when exploring technology opportunities (Yoon and Magee, 2018).
With the help of scientific and patent data, different well-established methods have been developed to measure S&T knowledge, such as a systematic literature review, content analysis, meta-analysis, bibliometric analysis, patentometric analysis, among others. These methods not only describe the S&T knowledge of a certain technology field in its current state, but also its origins and recombination dynamics. This helps to reveal the evolutionary trends in technology innovation (Avila-Robinson and Miyazaki, 2013; Gerdsri et al., 2013; Rodríguez-Salvador et al., 2017). However, most of these methods have not been very good at capturing the latent knowledge structures in S&T information. In order to explore the technological solutions for OHSS and to provide a deeper insight into current status and research opportunities in this field, different analyses should be performed. In semantic frameworks, technological knowledge can be defined as a collection of knowledge elements that represents the core knowledge of the corresponding technology field. In OHSS, the situation is more complex, as innovation is mainly reflected in the emergence of a new technological solution, which consists of a complete set of technological components. Each technological component is formed by a set of well-organized S&T knowledge. So, if the latent knowledge structures in the field are not revealed, the method is not likely to support further analysis well. Additionally, previous studies are more reliant on pre-defined technological topics when characterizing knowledge structures, which may result in the extreme risk of omitting important emerging knowledge with development potential.
In this study, using scientific papers and patents as a data source, we propose a novel methodology based on a heterogeneous knowledge network. The methodology reviews research progress of key and emerging technologies for OHSS from the period of 2008–2019, and provides an inclusive view of the technological solutions and innovations in OHSS. It also helps to predict the future development potential of technological entities and solutions. The analytical process of the methodology is as follows. First, principal components analysis (PCA) is applied to extract the principal technological components of OHSS from a list of unrelated technological knowledge elements. Then, based on the extracted technological components, a heterogeneous knowledge network representing OHSS is constructed. Through network analysis, we can identify the knowledge points that represent key and emerging technologies, and track their evolutionary dynamics. Then, a well-designed evaluation system measures and predicts underdeveloped and possible future technological solutions. Finally, short-term, medium-term and long-term technology opportunities of OHSS are obtained from the results. Overall, the picture developed provides insights for both researchers and enterprise managers in the field of OHSS. We expect that the insights revealed should prompt technology-oriented steps towards a low-carbon economy. Additionally, we expected that the proposed methodology will be helpful in exploring latent innovation opportunities and the development potential of not only OHSS, but also other technological fields.
The rest of this study is organized as follows. Section 2 describes the data and the proposed methodology. Section 3 outlines the network construction process and the process of identifying the technological solutions. Section 4 evaluates the development potential of those solutions. Section 5 provides the conclusion and outlook for future research.
2 Data and Methodology
The overall framework of our proposed methodology is illustrated in Figure 1. It contains five streamlined modules: entity extraction, heterogeneous network construction, network analysis, the identification of technological solutions, and the evaluation and prediction of technological solutions.
2.1 Extracting the Entities
This module primarily extracts technological entities from scientific and patent data for subsequent analysis. Herein, a technological entity refers to any singular, identifiable and separate technique, method, material, tool or process used in the storage of hydrogen. The main steps of the entity extraction process are as follows.
Step (1) involves formulating a retrieval strategy to collect data related to OHSS. In Step (2), a natural language processing algorithm statistically analyzes the frequency of words and phrases (both referred to as words hereafter). The 500 most frequently used words are then selected and irrelevant words are manually eliminated. This refined set of words are now considered as keywords. In Step (3), keywords that ostensibly have the same meaning are merged to leave a list of unique technological entities. The entities in the unstructured text of the collected scientific papers and patents are then converted into frequency vectors, which can be used for document screening and further analysis. Finally, in Step (4), the entity vectors are analyzed and any documents that contain two or more entities are screened into the sample. The final result is a scientific dataset and a patent dataset of OHSS entity vectors.
2.2 Constructing the Heterogeneous Knowledge Network
In technology mining research, keyword co-occurrence networks are generally used to provide a topology of the potential relationships between technologies. However, most of these do not clearly indicate what kind of relationships they are, nor do they identify the knowledge structures in the given field. In this paper, we introduce a heterogeneous knowledge network to address this limitation that reveals insights into the knowledge structures of OHSS.
Heterogeneous knowledge networks can express detailed structured information with a focus on the interaction of core technologies by generating technological components for the selected technology field. In this network, the nodes are entities, and the technological components are clusters of technological entities of the same type (see Figure 2). Technological components represent the main types, or classes, of technological entities in the OHSS knowledge system. For example, technological entities such as hydrogen, ethanol and methanol belong to the component of energy carriers. Technological entities such as inorganic salts, metal hydrides and carbon nanotubes fall into the component of storage materials. Solid oxide fuel cells, alkaline fuel cells and direct fuel cells belong to the component of fuel cells. PCA is then applied to extract the essential components of OHSS. Through discussions with domain experts, we can identify the core technological components that constitute the knowledge structure of OHSS. The extracted technological components reflect mainstream hydrogen storage technologies described in the S&T text.
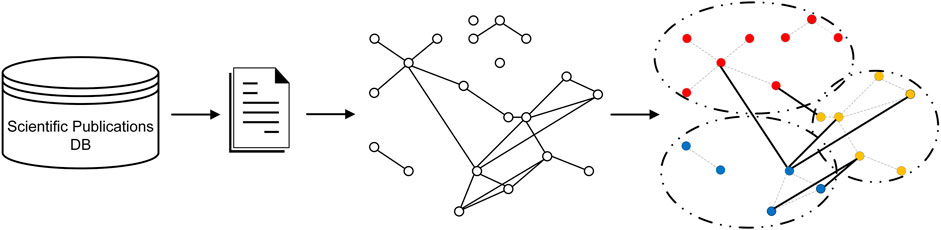
FIGURE 2. Schematic illustration of the co-occurrence associations in a heterogeneous knowledge network.
Additionally, the edges in the network represent connections between the different technological components; connections within the components are not included in this study. Compared to traditional network methods where any two nodes can connect to each other, here, the connections between nodes within the same component have been removed. This is because the most meaningful associations to us occur between the different components. An example of how heterogeneous knowledge networks using the multi-component structure might be effective is useful here. Below is an abstract of an article. Keywords are shown in bold, the codes in brackets represent their technological components.
The paper reviews the state of the art of hydrogen storage systems based on magnesium hydride (M), emphasizing the role of thermal management, whose effectiveness depends on the effective thermal conductivity of the hydride, but also depends on other limiting factors such as wall contact resistance and convective exchanges with the heat transfer fluid. For daily cycles, the use of phase change material to store the heat of reaction appears to be the most effective solution. The integration with fuel cells (proton exchange membrane fuel cell (F) and solid oxide fuel cell (F)) highlights the dynamic behavior of these systems, which is related to the thermodynamic properties of MgH2 (M). This allows for “self-adaptive” systems that do not require control of the hydrogen flow rate at the inlet of the fuel cell.
There are a total of three distinct keywords in this text. Among them, proton exchange membrane fuel cell and solid oxide fuel cell represent fuel cells, and magnesium hydride (MgH2) represents hydrogen storage materials. In a traditional keyword co-occurrence network, connections would be generated between all of these nodes. However, a discussion on the relationship between proton exchange membrane fuel cells and solid oxide fuel cells seems unnecessary. By identifying the core technological components, we found that the research on the co-occurrence relationships between components have more significance than the co-occurrence within components. Capturing such associations between different components more accurately reveals the knowledge structures within OHSS. This allows us to more easily explore the interactions between key technologies and also to predict potential links. Therefore, using a heterogeneous knowledge network to present the knowledge structures of a selected technological field obviously reduces network redundancy since all edges in the network can be explained. An illustration of the network is provided in Figure 2.
These components were then represented as nodes (entities) in a weighted heterogeneous network, where the edges reflect the co-occurrence frequencies of pairwise entities at the document-level. An adjacency matrix was used to calculate edge weights, formulated as
in which
This network can also be represented as a graph
where
2.3 Analyzing the Dynamics of the Network
To analyze the evolution of the network, we divided the sample into three different time intervals (2008–2011, 2012–2015, and 2016–2019). This allowed us to track how the network developed over time (see Figure 3). Once we determined the number of core technological components in each interval, we were able to explore the overall changes in composition and concentration of the network entities. In this manner, the entities constituting each technological component highlight the degree of development in field. We tracked the dynamic of the heterogeneous knowledge network, and measured the core technological entities, entity pairs and emerging technological entities. To analyze the tendencies for key technologies to change, we used centrality as an indicator.
Centrality is a basic concept in network analysis. Different centrality indicators generally measure the importance or prominence of an element within a network in distinct ways. By identifying a central node, we can determine the key technologies of a field. Centrality includes three indicators, degree centrality (
Degree centrality (
where
Betweenness centrality (
where
Closeness centrality (
where
2.4 Identifying the Technological Solutions
This module focuses on identifying all known and potential technological solutions in the given field—OHSS in our case. Here, multi-component structures are extracted from the heterogeneous knowledge network constructed in Section 2.2 to identify the technological solutions. Multi-component structures are formed by the entities in different technological components as well as the associated connections between these entities. As the heterogeneous knowledge network is generated from data in the scientific papers, this step essentially extracts the solutions hidden in scientific research. The analysis procedure is shown in Figure 4.
It is generally accepted that scientific research provides a fundamental basis for technology-oriented innovations. There are three main layers in the technology-oriented innovation processes: science, technology and industry. Therefore, according to the idea that scientific papers contain important information about future technological developments, two types of technological solutions can be derived by detecting the technology gaps between scientific data and patent data. One is solutions that have already been developed, which we can find in patents. The other is underdeveloped technologies that have some basis in research but have not yet been patented.
The prior art of each patent is identified by calculating and matching keywords in the patent document (See Figure 5 for calculation process). To begin with, the values of the entities in each component are calculated based on their word vectors, which is the sum of the corresponding word frequency. Then, the entity with the highest word frequency is used to represent the corresponding component. In this way, the technological solution of a patent can be determined. For example, in Figure 5, Cn, M1, and F2 are the entities with the highest word frequency among the three technological components respectively, so the final technological solution is Cn-M1-F2.
2.5 Evaluating and Predicting the Development Potential of Technological Solutions
To analyze the development potential of these technological solutions and predict which solutions will be the most promising, we established a comprehensive indexing system that measures the utility of each entity. From these figures, we can then calculate the total utility of a technological solution. The indexing system includes six indicators—four indicators for evaluating the importance of a technology and two indicators for evaluating the potential of a technology. Fuller descriptions of these indicators are given in Table 1.
With these values calculated, we applied each index to predict the technological innovation opportunities available. To validate our method, we divided the dataset into two parts: Dataset 1 covering the period 2008–2017, and Dataset 2 for the period 2018–2019. Dataset 1 was used to derive importance, and Dataset 2 was used to calculate the frequency indicators. The testing procedures are shown in Figure 6. First, we used a linear regression to construct the prediction model. Dataset 1 (2008–2017) was used to calculate the value of each indicator, which was taken as the independent variable of the prediction model. Dataset 2 (2018–2019) was used to calculate the frequency of each entity in the technological solutions, which was taken as the dependent variable. Second, the formula for the technology prediction model was derived by a regression analysis and its validity was also verified. Finally, we used all the historical data from 2008 to 2019 to predict the utility value of each technological entity. Hence, the total utility value of each technological solution was calculated and the most promising future solutions were identified. The results of all these steps are discussed in the next section.
3 Data Analysis and Results
3.1 The Entities Extracted
We used the Web of Science (WoS) and the Derwent Innovations Index (DII) databases as our data sources. The selected time range for the data was from 2008 to 2019. The search strategy implemented was TS = [((hydrogen storage) OR (solid storage) OR (onboard storage)) AND ((fuel cell vehicle) OR (fuel cell car) OR (fuel cell bus) OR (fuel cell))]. From this search, 4,039 scientific papers and 3,828 patent documents were retrieved.
The data were cleaned and transformed with the natural language processing tool in the text-mining software VantagePoint. We then assembled a word-frequency list. The 500 most frequently used words were used as our initial sample, and we manually removed the common words that had no specific meaning to OHSS (e.g., gas, fuel cell). We also removed descriptive words that indicated technological performance, such as parameters, qualities, and functions (e.g., 12.1 mm, tensile strength, filling pressure). Thus, 167 keywords were refined from our starting list of 500 high-frequency terms. Next, we merged keywords that had the same meaning. For example, proton exchange membrane fuel cell and polymer electrolyte membrane fuel cell were merged into PEMFC. H2 and hydrogen gas were merged into hydrogen. After these processing steps, 66 unique technological entities remained. We then transformed the unstructured documents into entity frequency vectors and used them to form a 4,039 × 66 scientific document-entity matrix and a 3,828 × 66 patent document-entity matrix. From the document-entity matrices, we selected any document that included two or more entities to result in two filtered datasets.
Next, we used VantagePoint’s PCA module to analyze the filtered data, discovering 14 principal factors which covered 65% of the total terms. Figure 7 shows the map of these factors, reflecting main technology topics and their relationships. From discussions with domain experts, we extracted three core technological components: hydrogen carrier (C), hydrogen storage material (M), and fuel cell (F), which constitutes the knowledge structure of the OHSS field. Within these components, hydrogen carrier involved 15 technological entities and 39 keywords; hydrogen storage material contained 43 technological entities and 108 keywords; and fuel cell contained 8 technological entities and 20 keywords.
3.2 The Heterogeneous Knowledge Network Constructed
We then constructed a weighted heterogeneous knowledge network based on the 66 technological entities (nodes). These entities had 959 edges between them reflecting co-occurrence across nodes at the document-level. According to the technological component each node belonged to, there were three types of edges in total (see Table 2)—527 edges represented hydrogen carrier—hydrogen storage material (C-M) component connections, which is the most frequently occurring connection. The second-most frequently occurring connection was hydrogen carrier—fuel cell (C-F) with 255 edges. And connections between hydrogen storage material—fuel cell (M-F) represented only 167 edges, which is the lowest frequency. Table 2 also shows an example of the connections between different components. As can be seen, the connection between hydrogen and metal-organic framework is part of the C—M family of edges. So studies associated with this edge focus on technological solutions that take hydrogen as an energy carrier and a metal-organic framework as a hydrogen storage material.
Now suppose that all the nodes in one component could form a cross-component connection with all the nodes in other components. Then the network would have 1,109 cross-component connections. However, the number of connections in the current network is 257, indicating that many potential associations still exist. However, even if the OHSS research were to be quite mature, it is unlikely that every cross-component connection would be formed. It is for this reason that tracing the developments trend of research and identifying the connections that are likely to form is of such great significance to those interested in technology opportunity analysis.
3.3 The Dynamics of the Network Revealed
Figures 8–10 illustrate the heterogeneous knowledge networks of OHSS for the periods 2008–2011, 2012–2015, and 2016–2019, respectively. As can be seen, the overall network density is increasing over time, and this intensification appears to speed up from the second period to the third period. However, from 2012 to 2015, we only see the addition of one more node over the former period. Additionally, several new connections form between the existing nodes. During the period from 2016 to 2019, 18 newly generated nodes were added to the network, and we also see 48 new connections. The total scale of the network grew to 60 nodes and 126 edges. Within this, the highest number of single-node connections grew from 20 during 2008–2011 to 46 during 2016–2019.
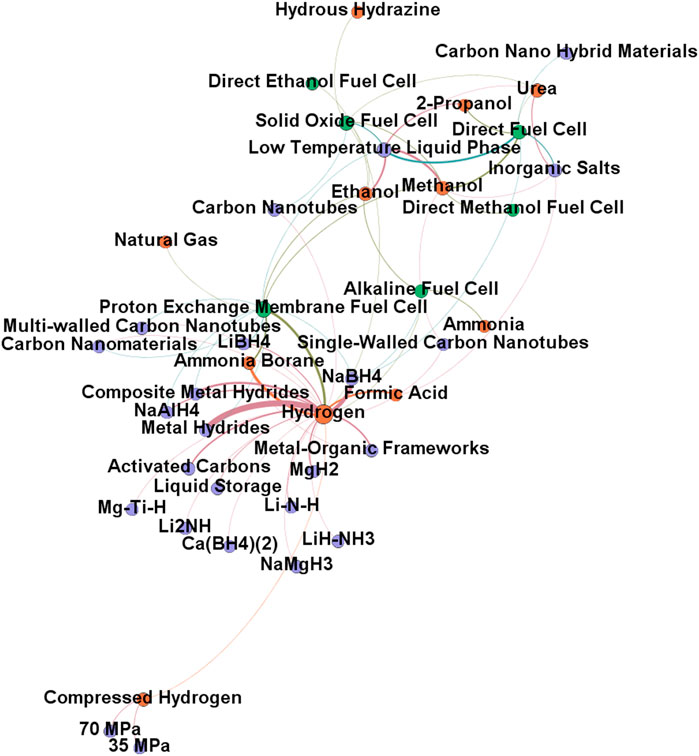
FIGURE 8. Schematic diagram of the OHSS heterogeneous knowledge network during 2008–2011. Note: The number of nodes is 41; The weight of edges is 169; The number of edges is 63.
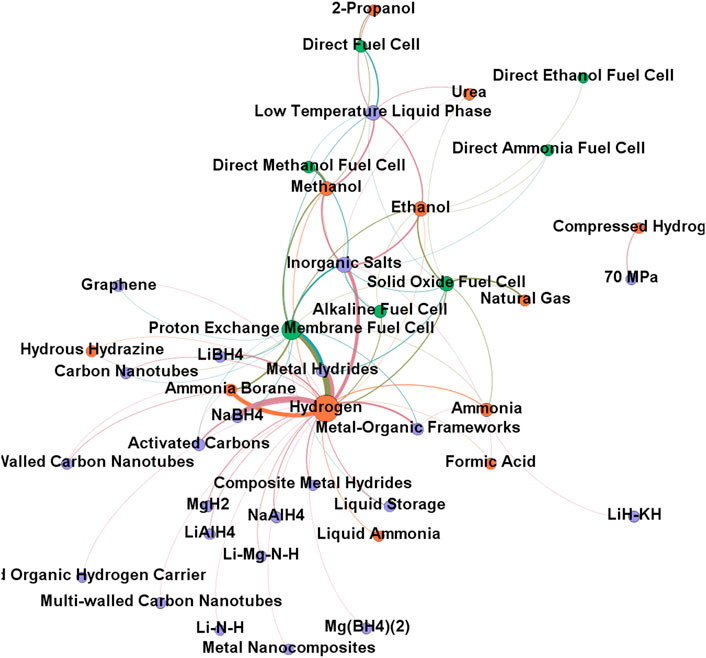
FIGURE 9. Schematic diagram of the OHSS heterogeneous knowledge network during 2012–2015. Note: The number of nodes is 42; The weight of edges is 276; The number of edges is 71.
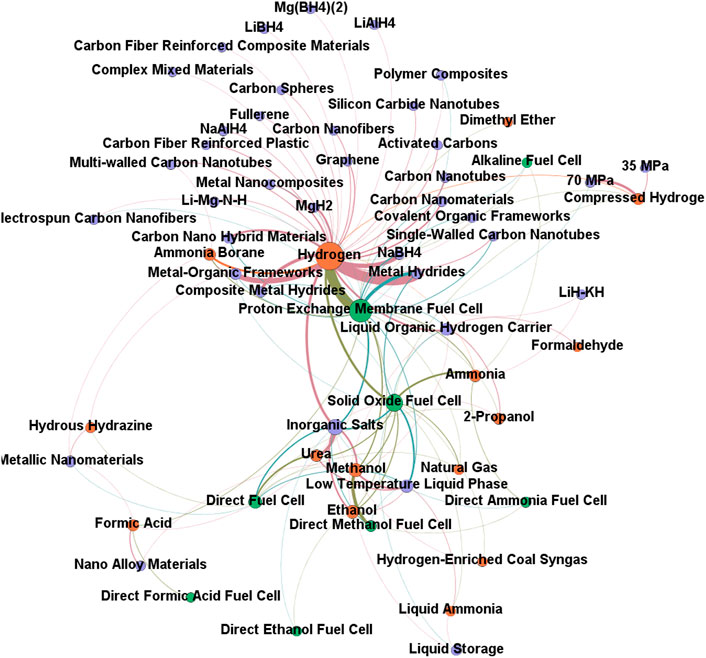
FIGURE 10. Schematic diagram of the OHSS heterogeneous knowledge network during 2016–2019. Note: The number of nodes is 60; The weight of edges is 504; The number of edges is 123.
In the first period, the research is distributed relatively evenly between each entity, forming a balanced distribution network. No evident research tendency can be seen. However, during the period 2012–2015, we see several core nodes with high centrality emerge. From 2016 to 2019, this trend develops further, and the importance of those core nodes continues to increase. As shown in Figures 8–10, aside from hydrogen, two new hydrogen carriers become essential—methanol and urea. Additionally, inorganic salts, metal hydrides, low-temperature liquid phase and metal-organic frameworks become the most commonly used material for hydrogen storage. PEMFCs and solid oxide fuel cells also become the most popular fuel cells in mass application.
Supplementary Appendices SA.1, SA.2, and SA.3 show the top 15 nodes associated with three different centrality measures during the three periods respectively. Comparing these three tables shows us how these core nodes have aggregated, transferred, and disseminated knowledge in the different periods. Further, integrating our analyses of the three centrality measures in Figures 8–10 reveals the main development trends in the network. The results show that the OHSS knowledge network has a star topology, in which all nodes connect to a few core nodes. This forms a radial network pattern with a few nodes as kernels. The most representative technologies are inorganic salts, low-temperature liquid phase, metal-organic frameworks, and liquid organic hydrogen carriers. Research on inorganic salts first appeared during 2008–2011 and was widely put into application in the following two periods. Eventually, it surpassed the popularity of low-temperature liquid phase and metal hydrides, becoming a core technology in the field of hydrogen storage. Moreover, metal organic frameworks becomes more and more central throughout the three periods to the point that it becomes a key core technology in the field during the last period 2016–2019. Liquid organic hydrogen carriers does not appear in the top 15 centralized technological entities in terms of any centrality dimensions in the first two periods. However, it suddenly appears as the top centralized technology from 2016 to 2019. Thus, this promising hydrogen storage technology has become a hot direction of technological innovation. A converse example is found in low-temperature liquid phase. Although it appeared in the top 15 centrality list during 2012–2015 and 2016–2019, its centrality ranking has declined significantly over time. This combined with a downward trend in newly formed connections shows that low-temperature liquid phase has become a relatively inactive research field.
Figure 11 shows the top 10 weighted edges for the three periods. This chart shows that hydrogen -metal hydrides, hydrogen - PEMFC, hydrogen—NaBH4, and metal hydrides - PEMFC are the most important technology combinations during each period. In addition, since 2012–2015, the weight of hydrogen—metal—organic frameworks has gradually increased, indicating that many studies have been conducted on this technology combination. This is usually a sign that it has great potential for further development.
Turning to the newly emerging nodes and edges during 2016–2019, Supplementary Appendix SB presents the top 15 representative entity pairs for further analysis. These technologies have emerged in recent years and corresponding research is relatively limited. Therefore, these technology combinations could be clues as to the technology opportunities and innovations of tomorrow. Technologies such as carbon fiber reinforced plastic, carbon nanofibers, carbon spheres, fullerene, and silicon carbide nanotubes have found new roles in OHSS. Other technologies such as electrospun carbon nanofibers, covalent organic frameworks, and carbon nanocomposites are newly applied in combination with PEMFCs. We believe that these technology combinations provide insights into the future research and innovation trends of OHSS.
3.4 The Technological Solutions Identified
Technological solutions are multi-component structures in the knowledge network. As mentioned above, in our knowledge networks, there are three core technological components: hydrogen carriers (C), hydrogen storage materials (M) and fuel cells (F). Therefore, we are primarily interested in technological solutions with a three-component structure; structures including only one or two technological components were excluded. We found and extracted 38 of these C-M-F chains, i.e., technological solutions, in the networks, as shown in Table 3.
The number of patents associated with a technological solution is a simple and effective way to reveal technology opportunities. The higher the number of patents related to technological solutions, the higher the degree of technological development. Among the 38 identified technological solutions, 28 were developed technological solutions. In addition, if a technological solution is associated with research activity but there are no or few corresponding patents, then that technology is underdeveloped and likely holds promise for future development. We identified 10 underdeveloped technological solutions, which have scientific research foundations but with no corresponding patents during the period of study. These are all potential technology opportunities for innovative firms to focus on.
4 Evaluation and Technology Prediction
4.1 The Technological Solutions Evaluated
After identifying the developed and underdeveloped technological solutions, the next step is to evaluate the development potential of these technological solutions for technology prediction. Before calculating the utility of the technological solutions, each entity of the technological solution needs to be evaluated. Using a regression analysis, we established a prediction model based on data over the full period of study, 2008 to 2019.
Following the indexing system in outlined in Section 2.4, we identified the relationships between the entities and the indicators. First, we took the data for each technological entity from 2008 to 2017 and calculated the value of each indicator as our independent variables. Then we took the frequency data for each entity during 2018–2019 as the dependent variables. All results were then normalized. The results of this exercise are shown in Supplementary Appendix SC.1, and the results of the regression analysis, conducted with SPSS software, are shown in Supplementary Appendix SC.2.
The regression analysis shows a correlation coefficient R of 0.995, a determination coefficient R2 of 0.989, and significance at the 0.001 level, which verifies the validity of the proposed model. Consequently, we find a prominent linear correlation between the dependent variable Y and the independent variables I1, I2, I3, I4, P1, and P2. With this regression coefficient, we constructed a utility model as follows:
4.2 The Future Technological Solutions Predicted
To predict future entity utility levels, we used data from the full period (2008–2019). The indicator values are given in Supplementary Appendix SC.3, and Figure 12 shows the values of Y from the above prediction model, which are the future utility levels of each technological entity. The future utility of the technological solutions are derived by summing the utility levels of each constituent entity. Table 4 shows the total utility values of 38 published technological solutions, including the 28 developed technological solutions and the 10 underdeveloped technological solutions.
According to Figure 12, we can see that hydrogen, inorganic salts, and SOFC have the highest utility. The technological solution hydrogen—inorganic salts—SOFC has the highest utility, which is verified in Table 4. Among all technological solutions, the top three technological solutions are hydrogen—inorganic salts—SOFC, hydrogen—carbon nanomaterials—SOFC and hydrogen—metal hydrides—SOFC. These developed technological solutions are currently fundamental and mainstream technologies in OHSS. As such, there should be substantial opportunities for further development over the next 2–3 years; however, these opportunities will be coupled with fierce competition. Firms should therefore choose the technological solutions that most suit their needs, capabilities, and development goals and, accordingly, formulate short-term research and development plans.
In terms of underdeveloped technological solutions, the top three technological solutions are hydrogen—metal-organic frameworks—SOFC, hydrogen—liquid organic hydrogen carriers—SOFC, and hydrogen - complex metal hydrides—SOFC. As can be seen, the technological solutions that combine the new hydrogen storage material “metal - organic frameworks”, “liquid organic hydrogen carriers” and “complex metal hydrides” with the traditional technology combination of “hydrogen and SOFC” are the most prominent and promising future technological solutions. Consequently, in the medium term (4–10 years), firms should focus on these technological solutions.
Additionally, after traversing every possible C-M-F chain in fully connected heterogeneous knowledge network of OHSS, we can exclude some obviously unreasonable technological solutions. For example, hydrogen cannot be stored in a low-temperature liquid state, and carbon nanomaterials, complex metal hydrides, carbon nanocomposites, and metal organic frameworks are limited to forming technological solutions with hydrogen. There are also several solutions that are still in the conceptual stage and have no real basis in scientific research yet. These are long-term opportunities worthy of investment over the next 10 years, as shown in Table 5.
5 Conclusion and Prospect
As a power technology with zero carbon emissions, OHSS is an important element of future transportation scenarios and emerging smart grids. Over the last decade, there have been several promising breakthroughs that will take OHSS from an experimental technology to one of mass production with wide-spread applications. Thus, exploring new technology opportunities in OHSS is of critical importance to researchers, specialists, and enterprise managers. To this end, we developed a novel methodology based on a heterogeneous knowledge network to provide insights into the efforts, knowledge gaps, and dynamics of this thriving field. The methodology begins with scientific papers and patents as the basis for analyzing the technological composition of the field. From this, we built a heterogeneous knowledge network and applied a series of well-designed evaluation indicators that revealed developed and underdeveloped technological solutions over the short-term, medium-term and long-term. Our results with OHSS suggest that the framework is a very effective solution for technology opportunity analysis and prediction.
The main contributions of this study are fourfold: 1) We make a holistic review of scientific and technological evolution in OHSS. 2) We present a novel methodology based on heterogeneous knowledge networks for identifying technological solutions. We applied this methodology to the field of OHSS; however, the methods can be generalized to any scientific field. 3) We offer an evaluation and prediction model for generating more accurate predictions of the potential within a technological solution. 4) We provide a comprehensive technology opportunity analysis of the field of OHSS.
Our analysis of OHSS identified three core technological components, including 66 technological entities, among which metal hydrides, inorganic salts, low-temperature liquid phrase and metal-organic frameworks are the most significant hydrogen storage materials at present. Of these, liquid organic hydrogen carriers are the fastest growing emerging hydrogen storage material in recent years. The fuel cell technologies that received the most attention are PEMFCs and SOFCs. PEMFCs have a stronger development momentum and are leading to intense competition. Methanol and ethanol are considered to be extremely efficient hydrogen carriers with tremendous growth potential in the future. In addition, other hydrogen sources, such as isopropanol and urea, also shows good application prospects for hydrogen storage.
We also identified 38 technological solutions, of which 28 are developed and 10 are underdeveloped. In the short-term, the solutions with the highest combined utility value are the ideal technology selections, such as hydrogen—inorganic salts—SOFC, hydrogen—carbon nanomaterials—SOFC and hydrogen—metal hydrides—SOFC. The underdeveloped technological solutions, which have relatively high utility level but are still in the stage of fundamental research are medium-term prospects for enterprise planning. These solutions include hydrogen—metal—organic frameworks—SOFC, hydrogen—liquid organic hydrogen carriers—SOFC and hydrogen - complex metal hydrides—SOFC. Solutions with little to no basic research and that have not been developed in practice are long-term technology directions with great R&D potential. These solutions include hydrogen—carbon nanocomposites—SOFC, hydrogen—metal—organic frameworks—AFC and hydrogen—carbon nanocomposites—AFC.
This study focuses on understanding the technological knowledge of OHSS. The results present some common technological entities associated with OHSS, but, through association analysis, they also reveal some new findings about the possible innovation pathways of OHSS. Hence, from one perspective, the proposed methodology can be seen as a tool for seeking technological solutions from existing OHSS research. Yet, from another perspective, it can be used a decision support system that produces insights into technology trends and potential directions of development that can help to create cutting-edge technologies, optimize business strategies, and maximize profits.
However, as with all studies, our has some limitations and future development work that needs to be considered. First, these results should be interpreted with caution, as we assumed that technological innovations mainly originate from the recombination of existing heterogeneous technological knowledge. The assumption that new technologies and ideas strictly emerge on the basis of old technologies could lead to biased outcomes, as many technologies are disruptive technologies or mutational technologies which may not stem from previous themes. To enhance the efficiency and practicality of the proposed methodology, some additional quantitative measures could be considered. Second, there are other important data sources, such as project data, product data, and market data that can contribute to further knowledge discovery activities. Thus, integrating additional data sources into the proposed methodology could generate a more reliable picture of future technological innovation. Third, although we can effectively forecast future technological solutions based on the proposed evaluation index system, the corresponding indexes could be further improved. Future research might take into account technical performance and technical risk. Finally, the results presented in this research may be limited because the research focuses on a single technology field. In the future, we plan to apply the proposed methodology to other fields to check its robustness.
Data Availability Statement
The raw data supporting the conclusion of this article will be made available by the authors, without undue reservation.
Author Contributions
XS: conceptualization, methodology, experimental investigation, software, and original draft preparation. LC: validation, data curation, visualization, and reviewing and editing. ZL and YC: read and supervision. All authors provided critical feedback and helped shape the research, analysis and manuscript.
Funding
This work was supported by National Natural Science Foundation of China (71964034 and 71871023), and Science and Technology Commission of Shanghai Municipality (“Scientific and Technological Innovation Action Program” Soft Science Research Project, Grant No. 22692198700).
Conflict of Interest
The authors declare that the research was conducted in the absence of any commercial or financial relationships that could be construed as a potential conflict of interest.
Publisher’s Note
All claims expressed in this article are solely those of the authors and do not necessarily represent those of their affiliated organizations, or those of the publisher, the editors and the reviewers. Any product that may be evaluated in this article, or claim that may be made by its manufacturer, is not guaranteed or endorsed by the publisher.
Supplementary Material
The Supplementary Material for this article can be found online at: https://www.frontiersin.org/articles/10.3389/fenrg.2022.899245/full#supplementary-material
References
Aaldering, L. J., Leker, J., and Song, C. H. (2019). Analysis of Technological Knowledge Stock and Prediction of its Future Development Potential: The Case of Lithium-Ion Batteries. J. Clean. Prod. 223, 301–311. doi:10.1016/j.jclepro.2019.03.174
Abe, J. O., Popoola, A. P. I., Ajenifuja, E., and Popoola, O. M. (2019). Hydrogen Energy, Economy and Storage: Review and Recommendation. Int. J. Hydrogen Energy 44 (29), 15072–15086. doi:10.1016/j.ijhydene.2019.04.068
Antonelli, C., Krafft, J., and Quatraro, F. (2010). Recombinant Knowledge and Growth: The Case of ICTs. Struct. Change Econ. Dyn. 21 (1), 50–69. doi:10.1016/j.strueco.2009.12.001
Asche, G. (2017). 80% of Technical Information Found Only in Patents”–Is There Proof of This? World Pat. Inf. 48, 16–28. doi:10.1016/j.wpi.2016.11.004
Ávila-Robinson, A., and Miyazaki, K. (2013). Dynamics of Scientific Knowledge Bases as Proxies for Discerning Technological Emergence - the Case of MEMS/NEMS Technologies. Technol. Forecast. Soc. Change 80 (6), 1071–1084. doi:10.1016/j.techfore.2012.07.012
Aziz, M. (2021). Liquid Hydrogen: A Review on Liquefaction, Storage, Transportation, and Safety. Energies 14 (18), 5917. doi:10.3390/en14185917
Bakhtin, P., Saritas, O., Chulok, A., Kuzminov, I., and Timofeev, A. (2017). Trend Monitoring for Linking Science and Strategy. Scientometrics 111 (3), 2059–2075. doi:10.1007/s11192-017-2347-5
Borgatti, S. P. (2005). Centrality and Network Flow. Soc. Netw. 27 (1), 55–71. doi:10.1016/j.socnet.2004.11.008
Brandl, J. E. (1998). Pasteur's Quadrant: Basic Science and Technological Innovation. J. Pol. Anal. Manage. 17 (4), 734–736. doi:10.1002/(sici)1520-6688(199823)17:4<734::aid-pam10>3.0.co;2-g
Chanchetti, L. F., Oviedo Diaz, S. M., Milanez, D. H., Leiva, D. R., de Faria, L. I. L., and Ishikawa, T. T. (2016). Technological Forecasting of Hydrogen Storage Materials Using Patent Indicators. Int. J. Hydrogen Energy 41 (41), 18301–18310. doi:10.1016/j.ijhydene.2016.08.137
Cumalioglu, I., Ertas, A., Ma, Y., and Maxwell, T. (2008). State of the Art: Hydrogen Storage. J. Fuel Cell Sci. Technol. 5 (3). doi:10.1115/1.2894462
Edquist, C. (1997). Systems of Innovation Approaches–Their Emergence and Characteristics. Available at: https://www.researchgate.net/publication/272396959_Systems_of_Innovation_Approaches_-_Their_Emergence_and_Characteristics.
Elberry, A. M., Thakur, J., Santasalo-Aarnio, A., and Larmi, M. (2021). Large-scale Compressed Hydrogen Storage as Part of Renewable Electricity Storage Systems. Int. J. Hydrogen Energy 46 (29), 15671–15690. doi:10.1016/j.ijhydene.2021.02.080
Eriksson, E. L. V., and Gray, E. M. (2017). Optimization and Integration of Hybrid Renewable Energy Hydrogen Fuel Cell Energy Systems - A Critical Review. Appl. Energy 202, 348–364. doi:10.1016/j.apenergy.2017.03.132
Freeman, L. C. (1977). A Set of Measures of Centrality Based on Betweenness. Sociometry 40 (1), 35. doi:10.2307/3033543
Frenken, K., Izquierdo, L. R., and Zeppini, P. (2012). Branching Innovation, Recombinant Innovation, and Endogenous Technological Transitions. Environ. Innovation Soc. Transitions 4, 25–35. doi:10.1016/j.eist.2012.06.001
Gerdsri, N., Kongthon, A., and Vatananan, R. S. (2013). Mapping the Knowledge Evolution and Professional Network in the Field of Technology Roadmapping: a Bibliometric Analysis. Technol. Analysis Strategic Manag. 25 (4), 403–422. doi:10.1080/09537325.2013.774350
Gong, A., and Verstraete, D. (2017). Fuel Cell Propulsion in Small Fixed-Wing Unmanned Aerial Vehicles: Current Status and Research Needs. Int. J. Hydrogen Energy 42 (33), 21311–21333. doi:10.1016/j.ijhydene.2017.06.148
Hassan, I. A., Ramadan, H. S., Saleh, M. A., and Hissel, D. (2021). Hydrogen Storage Technologies for Stationary and Mobile Applications: Review, Analysis and Perspectives. Renew. Sustain. Energy Rev. 149, 111311. doi:10.1016/j.rser.2021.111311
Hwang, H. T., and Varma, A. (2014). Hydrogen Storage for Fuel Cell Vehicles. Curr. Opin. Chem. Eng. 5, 42–48. doi:10.1016/j.coche.2014.04.004
Jannati, J., and Nazarpour, D. (2018). Multi-objective Scheduling of Electric Vehicles Intelligent Parking Lot in the Presence of Hydrogen Storage System under Peak Load Management. Energy 163, 338–350. doi:10.1016/j.energy.2018.08.098
Kim, J., and Lee, S. (2015). Patent Databases for Innovation Studies: A Comparative Analysis of USPTO, EPO, JPO and KIPO. Technol. Forecast. Soc. Change 92, 332–345. doi:10.1016/j.techfore.2015.01.009
Ko, N., Yoon, J., and Seo, W. (2014). Analyzing Interdisciplinarity of Technology Fusion Using Knowledge Flows of Patents. Expert Syst. Appl. 41 (4), 1955–1963. doi:10.1016/j.eswa.2013.08.091
Li M, M., Bai, Y., Zhang, C., Song, Y., Jiang, S., Grouset, D., et al. (2019). Review on the Research of Hydrogen Storage System Fast Refueling in Fuel Cell Vehicle. Int. J. Hydrogen Energy 44 (21), 10677–10693. doi:10.1016/j.ijhydene.2019.02.208
Li X, X., Xie, Q., Daim, T., and Huang, L. (2019). Forecasting Technology Trends Using Text Mining of the Gaps between Science and Technology: The Case of Perovskite Solar Cell Technology. Technol. Forecast. Soc. Change 146, 432–449. doi:10.1016/j.techfore.2019.01.012
Liu, J., Lu, K., and Cheng, S. (2018). International R&D Spillovers and Innovation Efficiency. Sustainability 10 (11), 3974. doi:10.3390/su10113974
Ma, J., Porter, A. L., Aminabhavi, T. M., and Zhu, D. (2015). Nano-enabled Drug Delivery Systems for Brain Cancer and Alzheimer's Disease: Research Patterns and Opportunities. Nanomedicine Nanotechnol. Biol. Med. 11 (7), 1763–1771. doi:10.1016/j.nano.2015.06.006
Mori, D., and Hirose, K. (2009). Recent Challenges of Hydrogen Storage Technologies for Fuel Cell Vehicles. Int. J. Hydrogen Energy 34 (10), 4569–4574. doi:10.1016/j.ijhydene.2008.07.115
Nechaev, Y. S., Makotchenko, V. G., Shavelkina, M. B., Nechaev, M. Y., Veziroglu, A., and Veziroglu, T. N. (2017). Comparing of Hydrogen On-Board Storage by the Largest Car Companies, Relevance to Prospects for More Efficient Technologies. Ojee 06, 73–79. doi:10.4236/ojee.2017.63005
Rodríguez-Salvador, M., Rio-Belver, R. M., and Garechana-Anacabe, G. (2017). Scientometric and Patentometric Analyses to Determine the Knowledge Landscape in Innovative Technologies: The Case of 3D Bioprinting. PLoS One 12 (6), e0180375. doi:10.1371/journal.pone.0180375
Roper, S., and Hewitt-Dundas, N. (2015). Knowledge Stocks, Knowledge Flows and Innovation: Evidence from Matched Patents and Innovation Panel Data. Res. Policy 44 (7), 1327–1340. doi:10.1016/j.respol.2015.03.003
Schillaci, C. E., Marku, E., Castriotta, M., and Di Guardo, M. C. (2021). Knowledge Creation in Patent Ecosystems: Insights from Singapore. Jkm 26, 1061–1082. doi:10.1108/JKM-11-2020-0859
Shi, S., Zhang, H., Yang, W., Zhang, Q., and Wang, X. (2019). A Life-Cycle Assessment of Battery Electric and Internal Combustion Engine Vehicles: A Case in Hebei Province, China. J. Clean. Prod. 228, 606–618. doi:10.1016/j.jclepro.2019.04.301
Shibata, N., Kajikawa, Y., and Sakata, I. (2010). Extracting the Commercialization Gap between Science and Technology - Case Study of a Solar Cell. Technol. Forecast. Soc. Change 77 (7), 1147–1155. doi:10.1016/j.techfore.2010.03.008
Tarhan, C., and Çil, M. A. (2021). A Study on Hydrogen, the Clean Energy of the Future: Hydrogen Storage Methods. J. Energy Storage 40, 102676. doi:10.1016/j.est.2021.102676
Turani-I-Belloto, K., Castilla-Martinez, C. A., Cot, D., Petit, E., Benarib, S., and Demirci, U. B. (2021). Nanosized Ammonia Borane for Solid-State Hydrogen Storage: Outcomes, Limitations, Challenges and Opportunities. Int. J. Hydrogen Energy 46 (10), 7351–7370. doi:10.1016/j.ijhydene.2020.11.224
Wang, M.-Y., Fang, S.-C., and Chang, Y.-H. (2015). Exploring Technological Opportunities by Mining the Gaps between Science and Technology: Microalgal Biofuels. Technol. Forecast. Soc. Change 92, 182–195. doi:10.1016/j.techfore.2014.07.008
Wehmuth, K., and Ziviani, A. (2013). DACCER: Distributed Assessment of the Closeness CEntrality Ranking in Complex Networks. Comput. Netw. 57 (13), 2536–2548. doi:10.1016/j.comnet.2013.05.001
Weidenthaler, C., and Felderhoff, M. (2011). Solid-state Hydrogen Storage for Mobile Applications: Quo Vadis? Energy Environ. Sci. 4 (7), 2495. doi:10.1039/c0ee00771d
Wu, J., and Shanley, M. T. (2009). Knowledge Stock, Exploration, and Innovation: Research on the United States Electromedical Device Industry. J. Bus. Res. 62 (4), 474–483. doi:10.1016/j.jbusres.2007.12.004
Xiao, T., Makhija, M., and Karim, S. (2021). A Knowledge Recombination Perspective of Innovation: Review and New Research Directions. J. Manag.. doi:10.1177/01492063211055982
Yoon, B., and Magee, C. L. (2018). Exploring Technology Opportunities by Visualizing Patent Information Based on Generative Topographic Mapping and Link Prediction. Technol. Forecast. Soc. Change 132, 105–117. doi:10.1016/j.techfore.2018.01.019
Yu, X., and Zhang, B. (2019). Obtaining Advantages from Technology Revolution: A Patent Roadmap for Competition Analysis and Strategy Planning. Technol. Forecast. Soc. Change 145, 273–283. doi:10.1016/j.techfore.2017.10.008
Zhang, Y., Porter, A. L., Hu, Z., Guo, Y., and Newman, N. C. (2014). "Term Clumping" for Technical Intelligence: A Case Study on Dye-Sensitized Solar Cells. Technol. Forecast. Soc. Change 85, 26–39. doi:10.1016/j.techfore.2013.12.019
Keywords: technology opportunities analysis, heterogeneous knowledge network, principal component analysis, network analysis, onboard hydrogen storage system
Citation: Shi X, Cai L, Li Z and Cui Y (2022) Exploring Technological Solutions for Onboard Hydrogen Storage Systems Through a Heterogeneous Knowledge Network: From Current State to Future Research Opportunities. Front. Energy Res. 10:899245. doi: 10.3389/fenrg.2022.899245
Received: 18 March 2022; Accepted: 20 May 2022;
Published: 20 June 2022.
Edited by:
Quan Xie, Curtin University, AustraliaCopyright © 2022 Shi, Cai, Li and Cui. This is an open-access article distributed under the terms of the Creative Commons Attribution License (CC BY). The use, distribution or reproduction in other forums is permitted, provided the original author(s) and the copyright owner(s) are credited and that the original publication in this journal is cited, in accordance with accepted academic practice. No use, distribution or reproduction is permitted which does not comply with these terms.
*Correspondence: Lingfei Cai, bGZjYWlAc2JzLmVkdS5jbg==