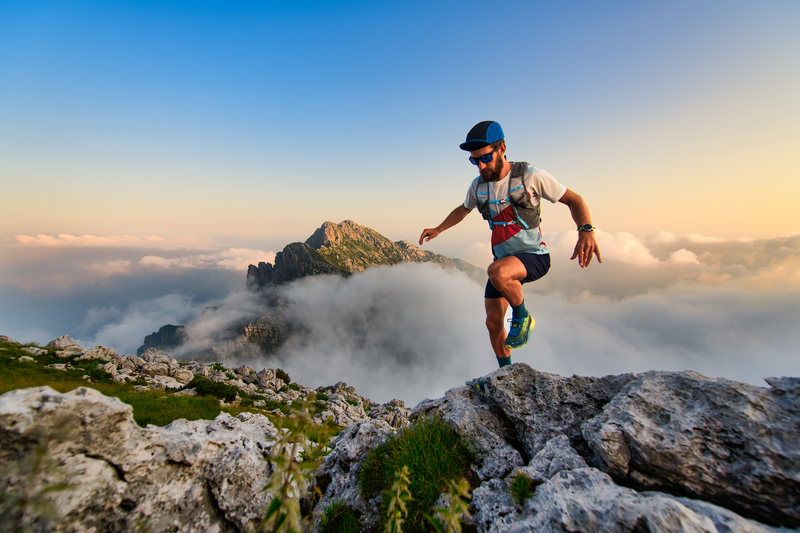
94% of researchers rate our articles as excellent or good
Learn more about the work of our research integrity team to safeguard the quality of each article we publish.
Find out more
ORIGINAL RESEARCH article
Front. Energy Res. , 23 May 2022
Sec. Wind Energy
Volume 10 - 2022 | https://doi.org/10.3389/fenrg.2022.864645
This article is part of the Research Topic Wind Turbine Wakes and Wind Farms View all 6 articles
The actuator line method is a widely used technique to model wind turbines in computational fluid dynamics, as it significantly reduces the required computational expense in comparison to simulations using geometrically resolved blades. Actuator line coupled to an aeroelastic solver enables not only the study of detailed wake dynamics but also aeroelastic loads, flexible blade deformation and how this interacts with the flow. Validating aeroelastic actuator line predictions of blade loading, deflection and turbine wakes in complex inflow scenarios is particularly relevant for modern turbine designs and wind farm studies involving realistic inflows, wind shear or yaw misalignment. This work first implements a vortex-based smearing correction in an aeroelastic coupled actuator line, and performs a grid resolution and smearing parameter study which demonstrates significant improvement in the blade loading and in the numerical dependencies of predicted thrust and power output. A validation is then performed using a 2.3 MW turbine with R = 40 m radius, comparing against blade resolved fluid-structure interaction simulations and full-scale measurement data, in both laminar and turbulent inflows including both high shear and high yaw misalignment. For an axisymmetric laminar inflow case, the agreement between blade resolved and actuator line simulations is excellent, with prediction of integrated quantities within 0.2%. In more complex flow cases, good agreement is seen in overall trends but the actuator line predicts lower blade loading and flapwise deflection, leading to underpredictions of thrust by between 5.3% and 8.4%. The discrepancies seen can be attributed to differences in wake flow, induction, the reliance of the actuator line on the provided airfoil data and the force application into the computational domain. Comparing the wake between coupled actuator line and blade resolved simulations for turbulent flow cases also shows good agreement in wake deficit and redirection, even under high yaw conditions. Overall, this work validates the implementation of the vortex-based smearing correction and demonstrates the ability of the actuator line to closely match blade loading and deflection predictions of blade resolved simulations in complex flows, at a significantly lower computational cost.
Modern wind turbine design employs large rotor swept areas and hub heights to maximise power output and efficiency of the turbine, while decreasing installation and maintenance cost per unit of energy produced. Structural and mass constraints result in increasingly flexible blades, with larger cone angles and prebend, which brings added complexity to both aeroelastic and aerodynamic computations (Veers et al., 2019). Large wind turbines are also more affected by inflow variation over the rotor extent due to atmospheric turbulence, shear, veer, and wakes, and therefore can experience greater loading variation during a single rotation. Potential methods of improving wind farm efficiency through yaw misalignment or power de-rating strategies (summarised in Kheirabadi and Nagamune (2019)) further complicates the accurate prediction of both turbine loading and wake behaviour. This combination of flexible deformation, control, turbulence and wake interaction means that robust and well-validated high-fidelity modelling techniques are essential for planning and operating modern wind farms.
Blade resolved (BR) simulations model the complete geometric blade shape, with sufficient resolution to capture the boundary layer. Wind farm simulations using such refined computational grids are currently in most cases computationally unrealistic. Therefore, modelling wind turbines with the actuator disc (AD) or actuator line (AL) methods provides a feasible alternative. Rather than capture the blade geometry, the turbine rotor is represented by body forces smeared across a disc representing the rotor area (AD) or along lines representing individual blades (AL), which substantially reduces the required resolution. Combining the AL with Large Eddy Simulations (LES) allows important time-varying and turbulent effects to be captured, including detailed wake dynamics and tip vortices (Troldborg, 2008; Sørensen et al., 2015). Wake flow predictions using the AL have been compared against wind tunnel measurements for both single wind turbine and wind farm scenarios, finding good agreement in both the near and far wake (Wu and Porté-Agel, 2011; Sørensen et al., 2015; Howland et al., 2016; Stevens et al., 2018; Doubrawa et al., 2020). Comparing the actuator methods with blade resolved simulations in non-sheared inflow, Troldborg et al. (2015) found that the AD and AL created similar wakes to resolved blades provided that the inflow was turbulent. However, under laminar conditions, resolving the blade boundary layer resulted in higher turbulence and eddy viscosity in the near wake, leading to significant differences in the wake several diameters downstream.
The ability of the AL to provide accurate blade loading predictions and include the mutual interaction between wind turbine and flow is important for a number of reasons. Clearly, the predicted blade loading (along with the computational setup) dictates the AL wake. Aeroelastic tools based on the blade element momentum (BEM) method are highly dependent on aerodynamics modules when a specific inflow is desired, and reliant on simple engineering models for describing the inflow if used in a wind farm scenario. Whereas, with the AL method, wind farm simulations can be conducted in which the turbine loading is calculated directly in response to inflow which includes turbulent and time-varying phenomena such as wake meandering and wake interaction. Modelling the influence of flexibility in the AL is possible through coupling with an aeroelastic tool (Sørensen et al., 2015), the effect of which was studied in Hodgson et al. (2021). Particularly for large turbines, modelling flexible deformation resulted in noticeable differences in blade loading, damage equivalent loads and power production for the AL, which were not seen for a standalone BEM tool, due to the ability of the blades to interact with the flow. Andersen and Sørensen (2018) studies correlations between inflow, turbine operation and wake flow, and demonstrates the importance of both having detailed knowledge of turbine inflow, and modelling the mutual turbine and flow interaction. In a comparison between fully resolved FSI simulations, measurement data and an aeroelastic blade element momentum (BEM) tool, Grinderslev et al. (2021) likewise showed discrepancies between CFD-based FSI and BEM-based simulations in predicting blade loading due to complex inflow, indicating the importance of using higher-fidelity methods. Other validations of blade loading predictions using the AD and AL, without modelling flexibility, have been performed against the MEXICO and NEW MEXICO experiments (Sarmast et al., 2016; Nathan et al., 2017).
As the purpose of the AL is to enable detailed wake and blade loading studies while still reducing computational effort, the dependencies and trade-offs linking grid resolution (Δx), Gaussian smearing length (ϵ), turbine radius (R) and number of actuator line points (nAL) have been the subject of significant prior work. Nathan (2018) describes the required nAL in terms of the porosity of the resulting AL force distribution in the domain; for Δx = R/32 and ϵ = 2Δx, nAL = 20 was sufficient to remove visible oscillations in the downstream velocity profile. Regarding the smearing length ϵ, Troldborg (2008) suggests ϵ = 2Δx is a good compromise between resolution and numerical stability; other approaches suggest varying ϵ along the blade length related to the chord length (Shives and Crawford, 2013) or an equivalent elliptic planform chord length (Jha et al., 2014). Other observations relate to the ratio ϵ/Δx; increasing ϵ for a given Δx leads to increasing power without convergence; while for a given ϵ/Δx ratio, increasing grid resolution decreases the blade loading (Martínez-Tossas et al., 2014; Meyer Forsting et al., 2019b).
The dependency of the blade forces on ϵ leads to loading overpredictions, particularly in regions of considerable change such as around the root and tip. Therefore, classical AD tip corrections (Glauert, 1935) or Glauert-type tip corrections Shen et al. (2005) are often applied to ALs. However, they have no physical basis for application in ALs, do not address the fundamental cause of the loading overpredictions, only act on the blade tip, and require turbine-specific tuning. A recent improvement to the actuator line which aims to decouple the smearing length and blade loading is the vortex-based smearing correction (Meyer Forsting et al., 2019a; Martínez-Tossas and Meneveau, 2019; Dağ and Sørensen, 2020). It is based on the recognition that ϵ determines the minimum resolution of the vortex at each blade station and so acts as a viscous inner core, equivalent to a Lamb-Oseen vortex. This viscous behaviour of the force smearing reduces the total circulation, decreases the blade induction and results in a higher angle of attack and blade loading overpredictions. The vortex-based smearing correction uses a near wake model (Pirrung et al., 2016) to calculate the total circulation and hence can recover the missing induction caused by the force smearing. This allows the angle of attack, and hence the blade loading, to be corrected along the entire blade length without any tuning of parameters.
This work aims to improve the coupled actuator line through implementing the vortex-based smearing correction for aeroelastic ALs, and validating against blade resolved fluid-structure interaction simulations and measurement data across a range of complex flow scenarios. First, the implementation of the vortex-based smearing correction into the coupled aeroelastic AL framework is described, along with extensions to allow for flexible deformations and prebend. The vortex-based smearing correction has been extensively verified in a standard AL formulation (Meyer Forsting et al., 2019b; Meyer Forsting and Troldborg, 2020), but to the authors’ knowledge this is its first application in a coupled aeroelastic AL. A parameter study is conducted and compared with the tip correction by Shen et al. (2005), to demonstrate the blade loading improvements and reduced numerical dependencies, and provide recommendations for smearing parameter and grid resolution. Finally, a validation against experimental data and blade resolved simulations is presented using the NM80 turbine (Grinderslev et al., 2021; Schepers et al., 2021), the largest turbine for which this comparison is currently possible. Predictions of blade loading, tip deflection, integrated quantities and wake flow are studied, across five inflow scenarios. These cases include both laminar and turbulent flow cases, with high shear and high yaw misalignment. The contribution of this paper is therefore both comparing two high-fidelity aeroelastic-coupled turbine models - actuator line and blade resolved - and quantitatively describing the differences, and also validating the actuator line for blade loading and deflection predictions in challenging inflow conditions.
All numerical simulations are conducted with EllipSys3D, a three-dimensional multi-block Navier Stokes solver (Sørensen, 1995; Michelsen, 1992, 1994). The incompressible governing equations are expressed in general curvilinear coordinates and solved using a finite volume method in a collocated grid arrangement. Time advancement is achieved using a second-order accurate three-level implicit method, which uses sub iterations within each time step. Throughout all simulations, an improved version of the SIMPLEC algorithm is used for solving the pressure correction equation (Shen et al., 2003), with the Rhie/Chow interpolation technique to avoid odd/even pressure decoupling. For laminar flow simulations, unsteady RANS (URANS) simulations are conducted with turbulence modelling by the k − ω SST model (Menter, 1993), and a third-order QUICK scheme used to discretise convective terms. For turbulent inflow simulations, the Improved Delayed Detached Eddy Simulation (IDDES) version of k − ω SST (Shur et al., 2008; Gritskevich et al., 2012) is used, with convective terms discretised by a hybrid scheme combining a fourth order Central Differencing for DES regions and a third-order QUICK scheme (Leonard, 1979) for URANS regions, with switching based on a limiter function (Strelets, 2001). Overset mesh capabilities including grid hole-cutting, and volume grid deformation are also utilised in the blade resolved simulations (Zahle et al., 2009).
Sheared inflow is applied through a power law at the inlet boundary of the computational domain,
where α is the shear exponent, Uref is a reference velocity, z is height and U is the inlet velocity profile.
In the turbulent simulation cases, turbulent fluctuations are generated using the Mann spectral model (Mann, 1994; Mann, 1998), and applied on a plane inside the computational domain (Troldborg, 2008; Gilling et al., 2009). The Mann spectral model is based on a linearisation of the Navier-Stokes equations and uses rapid distortion theory to model the spectral tensor. The generated turbulence field contains turbulent velocity components which are Gaussian, anisotropic, homogeneous and stationary, with second order statistics matched to the neutral atmosphere. Taylor’s frozen flow hypothesis is used in order to link the streamwise spatial dimension with time. As the shear profile is enforced at the inlet and turbulence applied on a plane inside the domain, they are linearly superimposed. Both the shear profiles and Mann model turbulence used in the validation cases are matched to measurements from the DanAero experiments, described further in Section 3.2.1.
The NM80 turbine, which has a rotor radius R = 40m and rated power 2.3 MW, is chosen for this work. It should be noted that the NM80 is small relative to new turbine designs, and therefore shows only a small influence of flexibility of the blade loading (Grinderslev et al., 2021). Nevertheless, this specific turbine was chosen due to the availability of experimental data from the DanAero measurement campaign (Bak et al., 2010), which allows for validation against real turbine data as well as a higher fidelity CFD wind turbine modelling technique (the blade resolved simulations, see Section 2.3.3). The DanAero measurements used blade-mounted strain gauges and pressure tabs on a commercial NM80 to measure blade loading, with inflow conditions characterised by a met mast located ≈250 m away. Through all CFD simulations, blade flexibility is always included, and the turbine is modelled without tower and nacelle.
Wind turbines are modelled using the actuator line (AL) method (Sørensen and Shen, 2002), in which body forces are applied on rotating lines to represent the rotor blades. The actuator line method is advantageous due to the reduction in computational requirements compared to blade resolved simulations, and can be used directly in simple structured grids. The AL used in this work is fully coupled to the aeroelastic BEM-based tool Flex5 (Øye, 1996) based on a loose numerical coupling. Flex5 has a reduced set of 28 degrees of freedom and can model the main deflection modes, but thin beam and small deflection assumptions mean that non-linear effects, such as torsional deflections, are not captured (Branlard, 2019). In order to approximate the influence of torsion in Flex5, it is possible to add a static contribution to the blade twist to represent a mean profile of torsional deflection (Andersen et al., 2021). In the EllipSys3D—Flex5 coupling, EllipSys3D extracts the velocity components at the actuator positions and transfers them to Flex5, which calculates the blade loads and deflections. The loads and deformed blade positions are passed back to EllipSys3D, where they are used to update the actuator positions and the magnitude of the applied body force (for further details of the EllipSys3D—Flex5 coupling see Sørensen et al. (2015) and Hodgson et al. (2021)).
The local velocities along the rotating actuator lines are used to determine the local velocity triangle and relative blade velocity, and hence the flow angle ϕ and the local angle of attack α, for each blade segment. Lift and drag forces are then found based on lift and drag coefficients CL(α, Re) and CD(α, Re), as a function of the Reynolds number Re (based on chord length and relative velocity) and angle of attack α, given as tabulated data. Projection gives the normal and tangential forces, Fx and Fθ. When applied in the computational domain these body forces must be smeared over multiple cells to avoid numerical singularities. The smearing is a 3D Gaussian distribution, achieved by applying a convolution to the local load f2D using a regularisation kernel ηϵ,
where Nb is the number of blades and r = ‖x − rei‖ is the Euclidean norm (the distance between the grid point and the actuator line points on the ith actuator line, denoted by unit vector ei).
Actuator methods are highly dependent on the quality of the aerofoil data provided. For this work the polars used are based on extraction from blade resolved CFD simulations assuming a fully turbulent boundary layer (Hansen et al., 1997; Schepers et al., 2021). In the last 10% of the blade the 3D CFD polar is replaced with 2D CFD data (Bangga, 2018; Bangga and Lutz, 2021) to prevent tip loss effects being double-counted. In the IEA Wind Task 29 this CFD-extracted polar was shown to significantly outperform previous versions found from wind tunnel testing (Schepers et al., 2021) when comparing blade loading predictions with DanAero measurements. The use of this polar intends to mitigate the impact of differences caused solely by aerofoil data on the actuator line performance.
The blade resolved simulations used for validation also couple the turbine model to an aeroelastic tool to predict blade deformation. This is through a Python framework, which couples EllipSys3D and the aeroelastic code HAWC2 (Larsen and Hansen, 2007). Unlike Flex5, HAWC2 has a multi-body formulation which accounts for the non-linear effects of large deflections. This means that it does include torsional deflection, although for a relatively small and stiff turbine such as the NM80 this is not expected to have a significant impact (see Section 5.1.2 for results which isolate the impact of torsion). This coupling is also a loose numerical coupling; every timestep the CFD mesh is deformed based on predicted displacements and rotations from HAWC2, before the flow is solved by EllipSys3D. Different to the EllipSys3D-Flex5 AL coupling, the blade forces are computed in CFD, while the aeroelastic solver calculates only the deflections based on the given loads. For further details and validation of the blade resolved simulations and FSI framework, see Grinderslev et al. (2021).
The vortex-based smearing correction intends to decouple actuator line blade loading predictions from the Gaussian kernel ηϵ used to apply the body forces into the computational domain (Meyer Forsting et al., 2019a; Dağ and Sørensen, 2020). This dependency exists because the smearing length ϵ determines the minimum resolution of the vortex at each blade station and so acts as a viscous inner core. Through using the equivalence between this viscous core and a Lamb-Oseen vortex, and calculating the total circulation with a near-wake model (Pirrung et al., 2016), the vortex-based smearing correction corrects for the missing induction at the blade station. This changes the angle of attack and hence the predicted blade loading. Proofs of the theoretical basis and a detailed description of the method are available in (Meyer Forsting et al., 2019a; Meyer Forsting et al., 2020).
When implemented in the coupled AL, the vortex-based smearing correction (herein referred to as the smearing correction) is integrated into the load calculation of Flex5. Rather than providing the blade loads directly as in the standard AL implementation, corrections to the spanwise, normal and tangential velocity components are taken from the smearing correction and then added to the velocity components extracted from EllipSys3D. This allows a modular approach which is easily included in the original angle of attack and load calculation of Flex5. The implementation was verified by a direct comparison with a standard actuator line using the smearing correction; with no flexibility or controller, the coupled and standard actuator line behave identically.
The original implementation of the smearing correction for the standard AL assumed a straight blade with no coning, matching the cylindrical coordinate system of the near-wake model. This means that to use the smearing correction while taking advantage of the benefits of the coupled system (i.e. modelling displacement of the actuator positions), flexible deformation and prebend should be accounted for through coordinate transformations in the smearing correction. This is done by an extension to an existing projection for rotor coning (Li et al., 2022). Instead of a single cone angle by which all radial stations and velocities can be projected, angles are computed individually for each blade station, as demonstrated in Figure 1. For the radial stations, the angle between the coned and deformed position (θtotal) is computed for each actuator line point, and then each station is projected by cos(θcone + θtotal). However, it is the coordinate system local to the blade section that is required in the lift and drag calculation, and therefore the velocities are projected by the angle cos(θcone + θlocal), where θlocal is the relative change in angle between sections, relative to the coned position. These modifications allow the deformed position of the actuator lines to be accounted for in the smearing correction at every timestep.
FIGURE 1. Straight, coned and deformed blade stations, with relevant angles for projecting radial stations and velocity components.
To verify the new implementation of the vortex-based smearing correction into the coupled actuator line, and to compare numerical dependencies with the tip correction of Shen et al. (2005), a parameter study is conducted. All simulations in the parameter study use a computational domain of dimensions 32R × 16R × 16R in the streamwise, lateral, and vertical directions, respectively. An equidistant refined region of size 16R × 4R × 4R (extending 6R in front and 10R behind the rotor plane) is used in the vicinity of the turbine and to resolve the wake. Discretisation with 1152 × 384 × 384 cells leads to a refined region with cell length 0.625 m, equating to 64 cells per radius for the NM80 turbine. This grid is then used on different grid levels as appropriate (i.e. at one-half, one-quarter or one-eighth of the number of grid points to give cell lengths of 1.25, 2.5 or 5 m respectively). The inflow velocity is 8 ms−1. Turbulence generated with the Mann spectral model (Mann, 1994, 1998) is applied as body forces on a plane inside the domain (Gilling et al., 2009), resulting in a turbulence intensity of 10%. The simulation length is 750 s, of which the final 600 s is used to calculate statistics.
Five simulation cases are used for comparison with DanAero and blade resolved results, of varying inflow complexity. Details are given in Table 1. The three laminar cases are directly matched to the IEA29 Wind Task (Grinderslev et al., 2021; Schepers et al., 2021), which are based on DanAero field measurements. The first turbulent case (Case 4) is the same as Case 2 but including turbulence, and is also identical to a turbulent case considered in the IEA29 Wind Task (Schepers et al., 2021). The second turbulent case (Case 5) is identical in setup to the laminar Case 3, but with the same turbulent fluctuations as added in Case 4.
TABLE 1. Simulation Cases. Pitch is defined as positive when decreasing the angle of attack. Yaw is defined as positive when the turbine rotates clockwise as seen from above (i.e. the blades rotate towards downstream from the top position).
Turbulence is generated using the Mann model (as described in Section 2.2). The turbulence box used for both Case 4 and Case 5 was originally made for the IEA29 Wind Task (Schepers et al., 2021), has dimensions 720 × 800 × 460 m and is discretised with 181 × 201 × 116 points (corresponding to a grid spacing of 4 m). As the Mann box is relatively short in length it is recycled during the simulation time. The same turbulence box is applied in both Case 4 and Case 5. The applied shear profiles are enforced at the domain inlet and are identical to those in the simulations by Grinderslev et al. (2021), which are fitted to met mast data from the DanAero experiments. Laminar simulations were run until the rotor thrust was converged to a less than 1% change over 5 revolutions, while turbulent simulations consisted of a spin-up simulation lasting 25 revolutions, and a successor lasting 50 revolutions over which statistics were calculated.
The blade resolved computations require three overlapping mesh groups; a rotor grid, a disc grid and a background grid. The rotor grid is grown from the blade surface mesh and has a first cell size sufficient to ensure y+ values of less than 1. The disc grid rotates with the rotor grid and is cylindrical with pre-cut holes around the blades; it has a radius of 56 m and extends 25 m from the rotor centre both upstream and downstream. See Grinderslev et al. (2021) for a full description of the setup, overset capabilities and mesh deformation.
The background grid has a length of 2000 m (50R), and a radius in the crossflow direction of 500 m (12.5R), with the rotor placed 800 m (20R) from the inlet. Dimensions, block discretisation, rotor position and boundary conditions are shown in Figure 2. A symmetry condition is enforced at the ground plane which is 57.2 m (1.4R) below the rotor, and at the domain sides. This ensures no development of the prescribed inflow shear profile between the inlet and rotor position. For turbulent simulations, the turbulent fluctuations are applied as body forces on a plane 8R upstream of the rotor. The mesh has a refined region extending approximately 8.5R upstream and 6R downstream of the rotor plane, and 1.5R in the radial direction from the rotor centre, in order to minimise distortion of the Mann box turbulence and to resolve the near wake. The total number of cells for the fully resolved case is 56.4 × 106, of which 36.1 × 106, 6.1 × 106 and 14.2 × 106 make up the background grid, disc grid and rotor grid respectively.
FIGURE 2. Computational Domain: (A) Background mesh in the rotor plane on grid level 2. (B) Overall domain size and boundary conditions with rotor plane mesh in white.
The coupled AL simulations are conducted on the background grid of the blade resolved setup, but using half of the number of mesh points (resulting in the rotor area mesh visualised in Figure 2A). This gives a discretisation of 37 cells per radius, and a total number of cells of 4.5 × 106. This resolution is similar to the 32 cells per radius discretisation used in Troldborg (2008), which was sufficient to resolve tip vortices; additionally, it is sufficient to reduce the thrust and power coefficient grid-based uncertainty to less than 0.2% and 1% respectively compared to using 64 cells per radius (see Section 4.2 for further discussion).The choice to perform the AL simulations on the background grid with lower resolution attempts to provide the best possible comparison while avoiding instabilities in the actuator line simulations caused by very small and skewed cells at block corners inside the background grid, as visible in Figure 2. Due to these varying cell sizes within the rotor area, even at a lower resolution care had to be taken to ensure numerical stability with both the choice of smearing parameter and timestep. The smearing parameter was set at ϵ = 2Δxmax, where Δxmax was the largest cell size within the rotor area, so that ϵ ≥ 2Δx was always obeyed. Based on the mean cell size, this led to ϵ = 2.9Δxmean (see Section 4.1 for further discussion). To ensure that the actuator line tip did not travel more than one cell at a time, the timestep was based on the smallest cell size in the rotor area; this was found to be particularly important for simulations including turbulence, where the deflections change more rapidly. Therefore, while the timestep in the blade resolved simulations is 0.125° of rotation per timestep (equating to Δt = 0.001694s for Case 1 and Δt = 0.001286s for Case 2 and Case 3), the actuator line simulations require a timestep of Δt = 0.00102s, despite the coarser grid level. This timestep restriction from the mesh increases the computational cost of the AL by more than a factor of 18 compared to conducting the simulations in an equidistant spaced grid with an equivalent AL resolution.
The Gaussian smearing length ϵ and the grid resolution Δx are numerical parameters which govern the actuator line force application into the domain and the subsequent resolution of the blade loading and wake. It is important to investigate the impact of these numerical choices on the coupled AL, particularly regarding the implementation of the vortex-based smearing correction in comparison to the previously used tip correction by Shen et al. (2005) (herein referred to as the Shen correction). First, in Section 4.1 the dependency of the blade loading on the smearing length ratio ϵ/Δx is investigated, at a fixed grid resolution. Then in Section 4.2 a grid study is conducted to quantify thrust and power coefficient uncertainties, while using the preferred ϵ/Δx ratio identified in Section 4.1.
The dependency of AL wind turbine wakes, blade loading and power prediction on the Gaussian smearing length ϵ is the subject of significant previous work (Troldborg, 2008; Shives and Crawford, 2013; Jha et al., 2014; Nathan, 2018). Recently, the implementation of the smearing correction has been shown to significantly reduce the smearing parameter dependency of the blade loading of a standard actuator line, while less impact was seen on the turbine wake (Meyer Forsting et al., 2020). However, it remains important to verify these observations specifically in relation to the coupled AL used in this work, to demonstrate the effectiveness of the smearing correction and to inform future studies. Figure 3 shows the tangential loading coefficient Ct for different ϵ values (presented in relation to both the grid cell size as ϵ/Δx and rotor radius as R/ϵ), for both the Shen correction (Figure 3A) and the smearing correction (Figure 3B). The Cartesian grid described in Section 3.1 is used, with a grid resolution in the refined region of 16 cells per radius.
FIGURE 3. Mean tangential load coefficient Ct under turbulent inflow with varying smearing parameter, at grid resolution R/Δx = 16. (A) Shen correction. (B) Smearing correction.
The smearing correction significantly smooths the tangential load distribution, particularly visible at the blade root and around r/R = 0.78. This is despite the fact that constants used in the Shen correction (c1 = 0.125, c2 = 30 and c3 = 0.1) have been set specifically for the NM80 turbine, while the smearing correction requires no tuning. The performance related to smearing parameter dependency is also a substantial improvement over that of the Shen correction; the percentage difference in the loads at r/R = 0.78 between ϵ/Δx = 2 and ϵ/Δx = 4 is 5%, whereas for the Shen correction it is 14%. The dependency of the normal loads (not shown for brevity) shows a similar result, with a 3% and 6% difference respectively. However, the smearing correction does not completely remove the dependency of the blade loading on ϵ; the trend of continuously decreasing loading with decreasing ϵ observed in Martínez et al. (2012) remains, as it stems from an error in the angle of attack estimation which is not tackled by the smearing correction (Meyer Forsting et al., 2020).
Additionally, it should be noted that for the smearing correction, there is a larger difference in predicted Ct between ϵ = 3Δx and ϵ = 4Δx than between ϵ = 2Δx and ϵ = 3Δx (visible in the inset of Figure 3B). This is due to the induction of neighbouring blades affecting one another; in the smearing correction only the self-induction at each blade is accounted for, as demonstrated in Meyer Forsting et al. (2019b). Therefore, if the simulations conducted here had been performed at a higher grid resolution while using the same range of ϵ/Δx ratios, the error related to only accounting for self-induction would be reduced, and the observed dependency of the blade loading on ϵ/Δx would be further decreased. However, it is relevant to note for resolutions typically used in AL wind farm simulations e.g. (Andersen et al., 2017; Archer and Vasel-Be-Hagh, 2019).
Based on these results, 2 ≤ ϵ/Δx ≤ 3 is preferred, and is used in all subsequent validation cases (see Section 3.2.2 for further details), roughly agreeing with the recommendations by Troldborg (2008). This range guarantees numerical stability while reducing the smearing effect on the wake. Additionally, even at a resolution of 16 cells per radius the difference in blade loading when changing ϵ between 2 − 3Δx is very small if using the smearing correction, as the main issue related to differences in induction of neighbouring blades is mitigated.
The refinement of the computational grid affects the blade loading, the resolution of tip vortices and turbulent structures, and is also directly linked to the appropriate smearing parameter ϵ and number of actuator line points nAL. As described in Nathan (2018), an insufficient number of actuator line points results in discontinuous force distribution which affects the flow, induction and thrust prediction. In the following grid study, nAL = 39 is used as it was found to be sufficient to give convergence of power and thrust coefficients with respect to nAL to within 0.2% at all grid resolutions. Figures 4A,B show the convergence of the mean power coefficient
FIGURE 4. Grid refinement study under turbulent inflow using ϵ =2Δx and nAL = 39. (A) Mean power coefficient
Generally both
Validation is performed using the NM80 turbine, on three laminar and two turbulent cases which represent a wide range of inflow conditions. The NM80 is the largest turbine for which both measurement data and an aeroelastic-coupled blade resolved model are available for validation against. The coupled actuator line is denoted “AL coupled” (either with the smearing correction (“SC”) or the Shen correction (“Shen”)); the blade resolved simulations are denoted ‘BR coupled’. Also shown are data from the DanAero measurements (Bak et al., 2010), in which it should be noted that only the pressure contribution to the blade loading is given, and not the viscous contribution. For all plots, an azimuth angle of 0° represents the blade at the top of its rotation.
The three laminar inflow cases cover axisymmetric, high shear and high yaw misalignment scenarios. Shear profiles were compared both upstream and alongside the rotor to ensure that the different grid refinements did not affect the flow development (and hence the comparison between results). In both positions U matched that of the blade resolved simulation to within 1% for all three cases across the domain height, providing confidence in the simulation setup.
Case 1 is a laminar inflow case, with uniform inflow velocity of Uhub = 6.1ms−1. The turbine has no yaw misalignment, and the blades are pitched slightly (by 0.15°) to decrease the angle of attack. Figure 5 shows the blade load distributions at 0° (top position) and the azimuthal variation in tip deflections. Only 0° is shown due to the axisymmetric nature of the case resulting in minimal azimuthal variations in loading (as can also be seen in the deflection plots).
FIGURE 5. Case 1: axisymmetric. (A) Normalised normal and tangential blade loads distribution at 0° azimuth; (B) Normalised flapwise and edgewise tip deflection.
Along the length of the blade there is an extremely good agreement between the blade resolved and both actuator line simulations for this case, despite the overprediction of tangential blade loading at the blade tip for the Shen correction. At the 0° position, in the outer half of the blade the maximum difference between the blade resolved and AL coupled (SC) results is less than 3% in Cn. All CFD simulations also show a stall region in the inboard region of the blade and predict the effect on blade loading similarly. This very close agreement between the CFD methods indicates that the aerofoil data used in the actuator line represents the blade resolved turbine well, for the angles of attack experienced in this case. The DanAero measurements show lower normal loading and significantly higher tangential loading than the CFD methods. This discrepancy could be due to the assumed axisymmetry and uniformity of the inflow, which is precisely enforced in the CFD methods, while there may be a greater degree of azimuthal variation in measurement data.
As expected, the edgewise tip deflection is dominated by gravity; the flapwise deflection also shows only a slight gravitational variation due to the minimal flow variation over the rotor area. Although the overall trends and magnitude of deflection are very similar, there is a small offset between the blade resolved and actuator line results (although the smearing correction slightly improves this in the flapwise direction). The differences in prebend modelling between the two aeroelastic solvers (described further in Section 6) account for 25% of the total discrepancy seen here, out of a total of only
Case 2 involves a small negative yaw angle of γ = −6.02° and a large shear exponent of α = 0.25. Additionally, the rotational speed is increased in comparison to Case 1, the turbine has a rotor tilt of 5° and the blades are pitched to increase the angle of attack by 4.75°. In Figure 6, blade loading and deflection predictions are shown: blade loading distributions at azimuth 0°; azimuthal loading variation at 75% radius; and flapwise and edgewise tip deflections. This is also the validation case for which the HAWC2 aeroelastic solver (which is coupled to the BR setup) predicts the highest torsional displacement Δθ; a mean of 0.43° and azimuthal variation amplitude of 0.11° at 95% radius. Therefore, the impact of the torsional deflection modelling of the two aeroelastic solvers is investigated by including two extra configurations: coupled BR modelled as completely stiff in torsion, and coupled AL with the mean BR torsional deflection profile added as a static twist contribution (as discussed in Section 2.3.2).
FIGURE 6. Case 2: high shear and low yaw. (A) Normalised normal and tangential blade loads distribution at 0° azimuth; (B) Normalised normal and tangential blade loads at 75% radius; (C) Normalised flapwise and edgewise tip deflection.
Compared to the extremely close agreement between blade resolved and actuator line loading for Case 1, the discrepancies between the two CFD simulation methods increase for this case. Along the blade length for both tangential and normal directions, the actuator line predicts lower loading than the coupled BR. The azimuthal loading at 75% radius has a near constant offset in Cn, between 4.7% and 5.1% lower across the rotation; while in the tangential direction this difference varies between 2.8% and 5.3% lower, between the 180° and 0° positions respectively. When modelling mean torsion in the coupled AL, there is a slight increase in blade loading and flapwise deflection. The reverse is true when stiffening the torsional degree of freedom in the coupled BR. However, although there is a slightly improved agreement achieved by matching the torsion modelling between the methods, these results clearly indicate that it accounts for only a small portion of the discrepancies seen here. Hence, particularly as this is the case with the highest torsional deflections, investigation of the torsion modelling is not included in subsequent validation cases.
All results have a clear 1P azimuthal load variation, caused by the applied inflow shear profile. The small yaw angle appears to have little impact in the CFD simulations, which all have the blade loading minima at 180° and very similar magnitude of azimuthal variation (ΔCn of 0.147, 0.137 and 0.136 for blade resolved, AL coupled (SC) and AL coupled (Shen) respectively). This is unlike the DanAero measurements, which have an asymmetric azimuthal variation with a minima at 200°, and also display a higher azimuthal variation in Cn, but lower in Ct, than the CFD simulations. While in CFD the yaw angle of the turbine can be fixed exactly, in the DanAero measurements the yaw is a mean over the measurement timeframe. This is potentially the reason for discrepancy in the trough location as a mean yaw angle may not be entirely representative of the conditions experienced by the turbine. Additionally, the presence of the tower for the real turbine may cause some of the differences between the measurement and CFD results.
The constant offset in flapwise deflection reflects the lower blade loading for the AL methods; the blade resolved simulation predicts a flapwise deflection Δtip/R = 0.0078 larger at 0° and Δtip/R = 0.0050 larger at 180°, compared to the coupled AL (SC). The smearing correction results in a greater variation in flapwise deflection than the Shen correction, and therefore slightly improved agreement with the coupled BR results. A reason for the discrepancies between BR and AL methods could be the aerofoil data performing less well at the angles of attack experienced in this case. At azimuth 0°, the coupled AL (SC) predicts an angle of attack between 13° and 11°, between 0.4 and 0.9R; this is significantly closer to the aerofoil data CLmax of between 14° and 20° than the range experienced for Case 1 (which has 5°–4°), which indicates that the aerofoil data may contribute to the discrepancies seen. Additionally, the smearing of the AL forces inevitably results in differences in apparent blade chord distribution and length, as well as in the formation of tip vortices, compared to the meshed blade in the coupled BR. This all contributes to different wake behaviour and thus changes in induction. However, there is again good agreement between the overall magnitude and trends.
Case 3 has the same rotor tilt, blade pitch, and fixed rotational speed as Case 2, but a reduced hub height velocity of Uhub = 8.43ms−1, slightly higher shear exponent of α = 0.26, and a very high yaw misalignment angle of γ = −38.34°. Figure 7 shows blade load distributions at azimuth 0°; azimuthal loading variation at 75% radius; and flapwise and edgewise tip deflections.
FIGURE 7. Case 3: high shear and high yaw. (A) Normalised normal and tangential blade loads distribution at 0° azimuth; (B) Normalised normal and tangential blade loads at 75% radius; (C) Normalised flapwise and edgewise tip deflection.
The combination of shear and yaw results in an apparent 2P azimuthal variation in the blade loading and deflections, which can be linked to the changing velocity experienced by the blade. Due to the yaw angle, from 0° the blade first moves in the upstream direction, but experiences decreasing loading from the shear profile. From 90° to 270° the blade travels away from the yawed wind direction, while the shear-induced loading has a minimum at 180° and then increases until reaching 0° again. This helps to explain the plateau in azimuthal normal loading (and to a lesser extent in flapwise deflection) between 0° and 90°, the minimum around 170°, and the steep increase to a peak which occurs at 270°. The CFD methods show peaks and troughs of loading in the same azimuthal locations, but similar to Case 2, the DanAero measurements have slight differences in overall trends, with a peak in normal loading at 250° rather than 270° and a far less pronounced trough at 180°. It should also be noted that the tangential blade load distribution demonstrates the beneficial smoothing effect of the smearing correction, compared to the spike visible for the Shen correction.
Similar to Case 2, along the blade length at azimuth 0°, and throughout the azimuthal variation at 75% radius, the AL simulations consistently predict a lower blade loading. In the 75% radius azimuthal plot, the maximum difference between blade resolved and AL simulations occurs at 170°, near the bottom of the blade rotation, where the AL coupled (SC) predicts a 10.5% lower Cn, and 19.8% lower Ct. This difference appears to consist partially of an offset to lower values, similar to as seen in Case 2, but also the AL simulations display a larger azimuthal variation in loading; for AL coupled (SC) this is ΔCn = 0.28, compared to 0.25 and 0.18 for the coupled BR and DanAero respectively. Again, potential causes of these differences between AL and BR could be the aerofoil data performance and the smearing of the actuator line. At azimuth 0°, the angle of attack is between 10° and 8°; although not as close to CLmax as seen for Case 2, it is much closer than for Case 1 and varies significantly during the blade rotation. Also, in a case with high yaw misalignment, the inflow angles may mean the 2D airfoil data of the AL fails to capture 3D flow effects such as radial flow along the blades. As the AL smearing effectively extends the blade length and chord, this both changes the induction and results in slightly increased blockage between the blade and ground plane, which reduces the speed-up seen under the rotor (partially due to the symmetry condition on the ground plane) and hence may result in a lower minimum of blade loading.
In flapwise deflection, a comparable offset between blade resolved and actuator line result exists as in Case 2. Here the most significant differences between the smearing correction and Shen correction are seen; the load distribution plots at 0° demonstrate how the Shen correction reduces the loading more in the final 5–10% of the blade length than the smearing correction, causing the decreased flapwise deflection. The coupled AL (SC) results match well to the overall trend of the blade resolved results, but at a offset towards lower values similar in magnitude to Case 2 (a difference of Δtip/R = 0.0048, or 19cm). The torsional contribution to angle of attack in the blade resolved simulations is smaller than for Case 2, with a mean Δθ of 0.26° and amplitude of variation of 0.17°. As demonstrated for Case 2, although a very slightly improved agreement could be obtained by including the mean torsion in the coupled AL, the differences between CFD methods primarily originates in the AL or BR turbine modelling rather than aeroelastics. Overall, a similar assessment can be made for Case 3 to that in Case 2: the good comparison between blade resolved and coupled AL simulations indicates that the coupled AL is appropriate for determining blade loading and deflections, even in high yaw cases such as this.
The turbulent flow cases are identical to Case 2 and Case 3 respectively, except with the addition of Mann model generated turbulence resulting in a turbulence intensity (TI) of 3.66%. The difference in computational methods between laminar and turbulent cases is the use of IDDES rather than just the k − ω SST model for turbulence modelling, and the use of a fourth order central differencing scheme for convective term discretisation in the DES regions. In addition to the shear profile comparison conducted to verify the flow scenario consistency in the laminar cases, for the turbulent simulations velocity time-series were compared from points 1 and 2R in front of the rotor plane. There were no observed differences in flow development, but it should be noted that there are less small-scale turbulent fluctuations resolved in the AL simulations due to both the coarser grid resolution and hence the larger required smearing length used in applying turbulent fluctuations into the domain. Only the coupled AL with the smearing correction and the coupled BR are presented in the following section.
Case 4 is identical to Case 2 (α = 0.26, γ = −6.02°), but with a turbulence intensity of 3.66%. In Figure 8, azimuthal distributions of blade loading are given at 75% and 90% radius, along with flapwise and edgewise tip deflections. It is immediately apparent from comparing Figures 6, 8 that the addition of turbulence causes only minor changes in the trends of mean azimuthal blade load and deflection variation. For both CFD methods, the loading at 75% radius and the tip deflections are slightly increased in comparison to the laminar simulations.
FIGURE 8. Case 4: high shear, low yaw and turbulence. (A) Normalised normal and tangential blade loads at 75% radius; (B) Normalised normal and tangential blade loads at 90% radius; (C) Normalised flapwise and edgewise tip deflection.
For the two CFD methods the location of the minimum loading remains at 180°, rather than 200° as seen for the DanAero results. As also discussed in Section 5.1.2, this could be due to tower effects combined with the variability in yaw angle in the measurements. The discrepancy increases for the 90% radius position; for the CFD methods it is still at 180°, while for the DanAero it is 210°. Additionally, at 90% radius the difference between the BR and AL results grows; at 0° increasing from ΔCn = 0.072 and ΔCt = 0.010 for 75% radius, to ΔCn = 0.111 and ΔCt = 0.033 at 90% radius. At the 90% radius the AL agrees extremely well with the DanAero measurements (except the minimum position), while the BR overpredicts compared to the measurements. The standard deviations of the azimuthal averages are very similar between the blade resolved and actuator line, with the somewhat higher values for the blade resolved perhaps reflecting the higher grid resolution capturing more small-scale turbulence. However, overall conclusions based on shape and relative differences are the same as for the laminar case; the AL simulations with the smearing correction agree well with both the blade resolved and DanAero results, but with an overall reduction in loading compared to the coupled BR.
Velocity magnitude—instantaneous snapshots and mean profiles—are shown across vertical and horizontal planes in the refined region of the mesh in Figure 9. The blade positions are represented by the actual mesh surface for the blade resolved cases, and an isosurface of the applied body forcing for the AL.
FIGURE 9. Case 4: High shear, low yaw and turbulence. Instantaneous velocity magnitude at centreline planes and mean velocity plots at marked locations. Blades shown by surface mesh for BR, and iso-surface of applied body force for AL. (A) Vertical plane and vertical mean velocity profiles. (B) Horizontal plane and horizontal mean velocity profiles.
From the instantaneous plots it is clear that the higher resolution of the background grid in the blade resolved simulations allows tip vortices to be captured; they are not visible for the actuator line despite the relatively high resolution of 37 cells per radius. Additional small-scale turbulence in the near wake is also present for the blade resolved cases, partially due to the very high resolution of the rotor mesh and disc grid. Considering the mean velocity profiles at x = 2R, 4R, and 6R downstream, there is good agreement between BR and AL simulations, with some key differences. For the horizontal planes, although both predict almost no wake redirection due to the small yaw angle, there is a difference in velocity magnitude at the wake centre. The BR near wake appears to break down more quickly, and reaches a near Gaussian profile at 6R downstream, while the AL does not. This reflects the conclusions of Troldborg et al. (2015), which showed that the blade resolved model produces additional vorticity generated by the small-scale turbulence of the blade boundary layer, leading to a faster near wake breakdown. The wake deficit is also slightly larger for the BR cases in both the horizontal and vertical mean profiles, linked to the consistently higher blade loading predictions and thrust (discussed in Section 5.3). However, despite the differences in resolution and modelling approaches, the overall wake structure, expansion and magnitude of the deficit are similar between the AL and BR scenarios.
Case 5 is identical to Case 3 (α = 0.26, γ = −38.34°) with the addition of turbulence. Figure 10 shows azimuthal distributions of blade loading at 75% and 90% radius, and tip deflections. Again, the overall trends and mean values are very similar to that observed in the laminar version of this case. Akin to the observations for Case 4, when considering the loading at 90% radius, the AL has good alignment with the DanAero measurements, particularly in the tangential direction. The coupled BR results appear to overpredict the blade loading compared to measurements in the final quarter of the rotation.
FIGURE 10. Case 5: high shear, high yaw and turbulence. Note, the standard deviation of DanAero data from Case 3 is added for reference, although the experimental TI does not match the simulated TI. (A) Normalised normal and tangential blade loads at 75% radius; (B) Normalised normal and tangential blade loads at 90% radius; (C) Normalised flapwise and edgewise tip deflection.
One difference between the laminar and turbulent results is the improvement in the agreement between the AL and BR in the normal loading and flapwise deflection. The deeper trough in normal loading seen in the Figure 7 AL results is not replicated here, and at 75% radius the offset between BR and AL is almost constant; at 160° azimuthal position ΔCn = 0.043 for Case 5, compared to ΔCn = 0.081 for Case 3. The only difference between Case 3 and Case 5 is the inclusion of turbulence, and therefore the cause of this improved comparison could be due to the turbulence resulting in changes in induction, turbine wake and speed-up between rotor and ground plane. Troldborg et al. (2015) found a better agreement between AL and BR wakes when the inflow was turbulent than when it was laminar; therefore in this case, a more similar wake could lead to changes in induction and hence blade loading predictions. Additionally, the smearing correction only corrects for the missing induction of the first 90° of the wake helix (Meyer Forsting et al., 2020); in the laminar case where there is no ambient turbulence to initiate the wake breakdown, the missing induction beyond 90° will play a role in the overall rotor induction. Introducing turbulence results in less stable vortex cores in the trailed vorticity, faster wake breakdown, and thus more similar induction and blade loading between BR and AL.
Figure 11 shows instantaneous velocity magnitude over vertical and horizontal planes in the refined region of the mesh, and mean wake profiles at x = 2R, 4R and 6R downstream. Again, there are clear tip vortices and additional small-scale turbulence in the near wake of the blade resolved simulation. Even in the instantaneous snapshots, the major regions of wake deficit are very similar between the two simulations.
FIGURE 11. Case 5: High shear, high yaw and turbulence. Instantaneous velocity magnitude at centreline planes and mean velocity plots at marked locations. Blades shown by surface mesh for BR, and iso-surface of applied body force for AL. (A) Vertical plane and vertical mean velocity profiles. (B) Horizontal plane and horizontal mean velocity profiles.
The mean wake profiles also demonstrate very good agreement between the cases, with the majority of differences occurring in the near wake. In the BR simulations, the small-scale turbulence from the blade root and nacelle gap region again results in differences in the near wake breakdown and shape, particularly at the wake centre. However, in the horizontal profiles this difference is not present at x = 4R or beyond, and the magnitude of wake redirection is very similar between the two methods. The relatively small refined region does not allow the extent of redirection in the far wake to be examined, but these results can be seen as an encouraging result for the performance of the actuator line for wake predictions in high yaw cases.
In addition to blade loading, tip deflections and wakes, it is important to assess the differences between the actuator line and blade resolved predictions of integrated quantities. In Table 2, the thrust and torque are given for each case simulated. For the turbulent simulations (Case 4 and Case 5), the result is given as a mean ± standard deviation over the 50 revolutions.
TABLE 2. Total thrust and torque comparisons for all validation cases. For Case 4 and Case 5 (with turbulent inflow), the mean ± standard deviation is provided.
For the axisymmetric Case 1, thrust and torque values match extremely well, with effectively identical values between coupled BR and coupled AL (SC) (a difference of 0.2%). This extremely good agreement reflects the close alignment of the blade loading seen in Figure 5 and highlights the benefits of using the coupled AL, as the simulations required 13x less CPU hours than needed for the coupled BR. For Case 2 and Case 3, the blade resolved CPU hour requirements were around 15 and 24 times larger than AL respectively (although the grid resolution and overset mesh cells account for a 12.5 times difference). Additionally, the small AL timestep required by the background mesh (as discussed in Section 3.2.2) significantly increases the computational cost of the AL compared to an equivalent AL resolution on equidistant spaced grid; therefore the AL would generally be even more favourable in comparison to BR.
For both Case 2 (high shear) and Case 3 (high shear and high yaw), the coupled AL underpredicts thrust in comparison to the blade resolved case (by 6.6% and 6.8% respectively for the SC), and also underpredicts torque (by 10.9% and 16.6% respectively for the SC). Again, this mirrors the blade loading differences in Figure 6 and Figure 7, where the AL consistently predicts lower Cn and Ct along the blade length. When torsional deflection was included in the coupled AL as a static twist contribution in Case 2, thrust increased by less than 1%; likewise the coupled BR without the torsional DOF experienced a thrust decrease by the same percentage. Reflecting the small difference in blade loading, the almost negligible change in integrated quantities again highlights that the torsional deflection modelling is not a significant cause of the differences between the CFD methods for this particular turbine.
In the two turbulent cases the comparison between coupled AL and coupled BR is very similar to that for the equivalent laminar cases. The AL predicts lower thrust (by 8.4% and 5.3% respectively for Case 4 and Case 5) and lower torque (8.9% and 5.3% respectively) than the blade resolved. The standard deviations of both quantities are very similar between methods, with the largest difference being a 6.1 kNm lower σ (a 7.5% change) for the torque of Case 4. The addition of turbulence causes an increase in the mean of all quantities, except the torque of the blade resolved simulation for Case 5 which slightly decreases. The computational time for both AL and BR simulations increased with the inclusion of turbulence, but the ratio between them remained similar; for Case 5 the blade resolved simulation took 17 times longer than the AL.
Comparing the two different smearing/tip corrections used for the AL, the Shen correction consistently results in a slight thrust decrease and a torque increase compared to the smearing correction. The smearing correction provides a correction to the velocity components to account for the missing induction due to the effective viscous core at the blade station, which results in a change to the angle of attack along the blade; while the Shen correction is applied solely at the blade tip in the load computation. For this turbine, the difference in angle of attack leads to an increase in the thrust, despite the overall smoothing effect on the load distribution.
Based on the mesh refinement study undertaken in Section 4.2, the predicted thrust values for actuator line simulations with either the Shen or smearing correction are independent of grid resolution to within 0.2%. Although performed on a mesh with a smaller refined region, and with some important differences in simulation setup, the mesh dependency of the BR simulations were examined by comparing two grid levels (the current refinement using blocks of 32 × 32 × 32 cells, and a coarser grid using blocks of 16 × 16 × 16 cells) in Grinderslev et al. (2021). This gave changes in thrust and torque of up to 2% for Case 1 and 2 between the coarse and fine grids, but −4% and −10.8% for thrust and torque respectively in Case 3. However, this difference was attributed to the poor overset connectivity in the high yaw case at coarse grid resolutions. Therefore, the differences in thrust and torque shown in Table 2 between coupled BR and coupled AL in Cases 2–5 are larger than the mesh-related uncertainties.
The aims of this work were to improve the coupled aeroelastic actuator line through implementing the vortex-based smearing correction, and to validate against fully resolved fluid-structure interaction simulations and measurement data. Relating to the first aim, the results presented in Section 4 clearly show how the turbine outputs such as blade loading, power and thrust are less dependent on smearing parameter and grid resolution when using the smearing correction. Additionally, smoother blade load distributions are also seen across all three laminar validation cases, where the loads using the Shen correction frequently show spikes around the blade tip. Although occasionally this higher tip loading appears to agree better with the coupled BR results, it must be noted that the coefficients in the Shen correction have been tuned specifically for this turbine operating in this loading region (at high CT values), and so is not guaranteed to function well at higher wind speeds. The smearing correction however requires no prior adjustments and operates without tuning bias towards certain regions of operation. Additionally, based on the Section 4 results, this agreement will be significantly more numerically dependent than it is for the smearing correction.
Relating to the second aim of actuator line validation, both the laminar and turbulent cases show a good agreement between blade resolved and actuator line simulations. In the axisymmetric case the blade loading and tip deflection are very well aligned, with integrated quantities agreeing to within 0.2% when using the smearing correction in the coupled AL. For more complex cases involving shear and yaw, there is consistently lower blade loading predicted by the AL, which results in a lower flapwise tip deflection, thrust and torque. However, despite these differences in integrated quantities, in the overall magnitude, trends and shape, the AL performs very well compared to the blade resolved method. This is despite the significantly reduced computational expense of running the simulations, in part due to using a coarser grid resolution. Flexibility is included in all simulations conducted in this work, but when using a relatively small and stiff turbine it is unlikely to significantly alter the blade loading in comparison to the stiff configuration, as found in Grinderslev et al. (2021). However, the reason for using the NM80 is that it is the largest turbine for which both measurement data and an aeroelastic-coupled blade resolved model are available, and therefore provides the best possible validation for the coupled AL for future applications. As the work on the DTU 10 MW RWT by Hodgson et al. (2021) indicates, for larger turbines including flexibility can have a significant effect on predictions of power, blade loading and damage equivalent loads, as the interaction between flow and blade deformation becomes increasingly important.
The two CFD methods are coupled to two different aeroelastic solvers; the AL is coupled to Flex5, which provides the blade loads and displacements based on extracted velocity components; whereas the BR is coupled to HAWC2, which provides the displacements based on loads extracted from the CFD mesh. Flex5 was used for the AL coupling as it is significantly faster than HAWC2 for each individual aeroelastic computation (due to the reduced set of degrees of freedom and modal-based approach) and hence is computationally realistic for aeroelastic coupled wind farm simulations. The main aeroelastic modelling difference between Flex5 and HAWC2 is in the torsional deflection Δθ, which Flex5 does not include, while HAWC2 does. However, for this relatively small and stiff turbine, the extra results included for Case 2 which isolate the torsional degree of freedom indicate that this does not significantly contribute to the observed discrepancies. Including the mean torsional deflection as a static change in blade twist may be a useful addition in Flex5 for analysis of larger and more flexible turbine designs. Another difference between the modelling in Flex5 and HAWC2 is the way in which blade pitch is applied; in Flex5 it is applied as a twist contribution, while in HAWC2 the blade is rotated around the pitch axis. However, comparisons of the two tools in axisymmetric inflow with varying pitch angles showed a negligible impact of this difference on the blade loading; for future turbine with longer blades and larger prebend, this may need to be taken into further consideration.
Comparing structural eigenfrequencies, the DanAero measurements have a first flapwise frequency of 0.955 Hz and a first edgewise frequency of 1.84 Hz, while HAWC2 gives 0.93 and 1.84 Hz respectively, and Flex5 gives 0.922 and 1.83 Hz. The very close agreement between these (less than 1% difference between HAWC2 and Flex5) suggests that this is also unlikely to be the source of significant discrepancies in results. Finally, the method of modelling the blade prebend also differs between the aeroelastic solvers. The multibody formulation of HAWC2 can precisely match the desired prebend; while Flex5 computes a mode shape which gives a best fit to the input values, and performs several initialisation computations at the simulation start in order to ensure stability. However, the difference between input prebend and mode shape in Flex5 is less than 2 cm at the blade tip for the NM80 turbine; this accounts for 25% of the difference between predicted deflections of coupled BR and coupled AL for Case 1, and 5–10% in all other cases. Therefore, these very small or negligible aeroelastic differences suggest that it is the CFD methods for turbine modelling which primarily cause the discrepancies in results, rather than the aeroelastic solvers that they are coupled to.
Although it is valuable to include actual measurement data when performing a validation such as this, and the results provide a useful guide to trends and levels of loading, the nature of such measurements means that the comparison with CFD methods can never be exact. The first major difference is the presence of hub and tower; the tower in particular appears to cause noticeable differences in the azimuthal loading variation between CFD and measured results, particularly in the high shear cases. Although the pitch and rotational speed were fixed, the yaw offset is a mean based on the upstream wind measurements and so is unlikely to exactly represent the precise fixed angle achievable in CFD. There is also uncertainty in the inflow when comparing CFD simulations to measurement data, as the inflow measurements were taken from a met mast ≈250 m away from the turbine. Due to the force measurement method, only pressure forces (not viscous forces) are included in the DanAero results, which may affect the comparison, especially in the tangential direction. Also, the integrated pressure forces are highly sensitive to the coarse distribution of pressure tabs at the trailing edge (Grinderslev et al., 2021).
While in the BR setup the geometry of the blade surface is directly meshed, the formulation of the actuator line method means that it is highly reliant on the quality of the aerofoil data provided. Initially, three different versions of the NM80 aerofoil data were found, which gave substantial differences in blade loading, even for the axisymmetric laminar Case 1. The latest NM80 aerofoil data developed at IAG Stuttgart in connection with the ongoing IEA Task 47 (IEA, 2022) are used here, which were created through extraction from a blade resolved CFD simulation. However some uncertainties still exist due to the position of extraction of the aerofoil data, the smoothing techniques used between lift and drag curves at each section, and how the transition from 2D to 3D effects at the blade tip is accounted for, particularly in high yaw with more radial flow along the blade. However, with a high quality of aerofoil data, this validation demonstrates that the actuator line has the potential to perform extremely well.
This work first presents the implementation of the vortex-based smearing correction into the aeroelastic coupled actuator line, and then performs a validation against fully resolved fluid-structure interaction simulations and measurement data. A parameter study comparing with the tip correction by Shen et al. (2005) demonstrates that the vortex-based smearing correction is capable of significantly reducing the dependency of turbine outputs such as power, thrust, and blade loading, on numerical choices such as the Gaussian smearing length ϵ and grid resolution Δx.
Three laminar cases and two turbulent cases are used for the validation with the NM80 turbine, including high shear and high yaw. For the axisymmetric laminar case, there is very good agreement between the coupled actuator line and blade resolved simulations in both blade loading and integrated quantities, which agreed to within 0.2%. For cases including high shear and high yaw, the actuator line consistently predicted slightly lower blade loading and flapwise deflection, which led to underpredictions of thrust by between 5.3% and 8.4% across the four complex inflow cases. The overall trends of the actuator line results are very similar to the blade resolved, despite the lower mean values. The discrepancies seen when comparing geometrically resolved blades with the applied body forces of the actuator line can be attributed to differences in wake flow, induction, and the fundamental formulation of the AL which relies on the quality of provided airfoil data and the application of the forces into the computational domain. Considering these differences, there is very good alignment between the actuator line and blade resolved simulations, even for the most challenging case; a turbulent and sheared inflow with the turbine operating at −38° yaw misalignment. Comparing the wakes in the two turbulent simulations also indicates good agreement in wake shape, deficit and redirection between the two cases, despite the fact that the actuator line simulations were conducted at a lower grid resolution due to mesh constraints. Overall, the coupled actuator line is capable of closely matching the blade loading and near wake predictions of the blade resolved simulations and measurement data. This is particularly relevant for studies on wind farms involving realistic inflows, shear and yaw misalignment.
The structural model of the considered wind turbine is not publicly available, and the structural and fluid solvers are licensed. All data and parts of the code covering the vortex-based smearing correction, and results data from the simulations conducted for this work, are available upon request.
Ideas generation and discussion (EH, CG, AM, NT, NS, JS, and SA); implementation of vortex-based smearing correction in coupled AL (EH with help from AM); actuator line simulations (EH); blade resolved simulations (CG); blade resolved setup (CG and NS); results analysis (EH and CG with help from NT and SA); paper writing (EH); paper editing (EH, CG, AM, NT, NS, JS, and SA).
The PhD of main author Emily Louise Hodgson is funded by the European Union Horizon 2020 research and innovation program under grant agreement no. 861291 as part of the Train2Wind Marie Skłodowska-Curie Innovation Training Network (https://www.train2wind.eu/). The DanAero projects, from which experimental data was obtained, were funded partly by the Danish Energy Authorities, (EFP 2007. Journal nr.: 33033-0074 and EUDP 2009-II. Journal nr. 64009-0258) and partly by eigenfunding from the project partners. Flow cases and initial CFD work was initialized during the project “Participation in IEA Task 29: Full Scale Wind Turbine Aero -dynamics, -elasticity and -acoustics” funded by EUDP (Journal nr. 64018-0084). Computational resources were provided by the DTU Risø cluster Sophia (DTU Computing Center, 2021).
The authors declare that the research was conducted in the absence of any commercial or financial relationships that could be construed as a potential conflict of interest.
All claims expressed in this article are solely those of the authors and do not necessarily represent those of their affiliated organizations, or those of the publisher, the editors and the reviewers. Any product that may be evaluated in this article, or claim that may be made by its manufacturer, is not guaranteed or endorsed by the publisher.
Thanks to DTU Senior Scientists Frederik Zahle and Ebba Dellwik for their insightful and thorough feedback and advice during the paper writing process.
Andersen, S. J., Karikari-Boateng, A., Bowie, A., Greaves, P., Madariaga, A., Simon, E., et al. (2021). Total Control: Deliverable 3.9: Predictive Wind Field Model. Tech. Rep.
Andersen, S. J., and Sørensen, J. N. (2018). Instantaneous Response and Mutual Interaction Between Wind Turbine and Flow. J. Phys. Conf. Ser. 1037, 072011. doi:10.1088/1742-6596/1037/7/072011
Andersen, S. J., Sørensen, J. N., and Mikkelsen, R. F. (2017). Turbulence and Entrainment Length Scales in Large Wind Farms. Phil. Trans. R. Soc. A 375, 20160107. doi:10.1098/rsta.2016.0107
Archer, C. L., and Vasel-Be-Hagh, A. (2019). Wake Steering via Yaw Control in Multi-Turbine Wind Farms: Recommendations Based on Large-Eddy Simulation. Sustain. Energy Technol. Assessments 33, 34–43. doi:10.1016/j.seta.2019.03.002
Bak, C., Aagaard Madsen, H., Schmidt Paulsen, U., Gaunaa, M., Fuglsang, P., Romblad, J., et al. (2010). Dan-aero Mw: Detailed Aerodynamic Measurements on a Full Scale Mw Wind Turbine.
Bangga, G. (2018). Comparison of Blade Element Method and Cfd Simulations of a 10 Mw Wind Turbine. Fluids 3, 73. doi:10.3390/fluids3040073
Bangga, G., and Lutz, T. (2021). Aerodynamic Modeling of Wind Turbine Loads Exposed to Turbulent Inflow and Validation with Experimental Data. Energy 223, 120076. doi:10.1016/j.energy.2021.120076
Branlard, E. S. P. (2019). Flexible Multibody Dynamics Using Joint Coordinates and the Rayleigh‐Ritz Approximation: The General Framework Behind and Beyond Flex. Wind Energy 22, 877–893. doi:10.1002/we.2327
Dağ, K. O., and Sørensen, J. N. (2020). A New Tip Correction for Actuator Line Computations. Wind Energy 23, 148–160. doi:10.1002/we.2419
Doubrawa, P., Quon, E. W., Martinez‐Tossas, L. A., Shaler, K., Debnath, M., Hamilton, N., et al. (2020). Multimodel Validation of Single Wakes in Neutral and Stratified Atmospheric Conditions. Wind Energy 23, 2027–2055. doi:10.1002/we.2543
Forsting, A. M., and Troldborg, N. (2020). Generalised Grid Requirements Minimizing the Actuator Line Angle-Of-Attack Error. J. Phys. Conf. Ser. 1618, 052001. doi:10.1088/1742-6596/1618/5/052001
Gilling, L., Sørensen, N., and Rethore, P. (2009). Imposing Resolved Turbulence by an Actuator in a Detached Eddy Simulation of an Airfoil. In EWEC 2009 Proceedings Online (EWEC).
Glauert, H. (1935). “Airplane Propellers,” in Aerodynamic Theory (Berlin, Heidelberg: Springer), 169–360. doi:10.1007/978-3-642-91487-4_3
Grinderslev, C., González Horcas, S., and Sørensen, N. N. (2021). Fluid-Structure Interaction Simulations of a Wind Turbine Rotor in Complex Flows, Validated Through Field Experiments. Wind Energy 24, 1426–1442. doi:10.1002/we.2639
Gritskevich, M. S., Garbaruk, A. V., Schütze, J., and Menter, F. R. (2012). Development of DDES and IDDES Formulations for the K-ω Shear Stress Transport Model. Flow. Turbul. Combust. 88, 431–449. doi:10.1007/s10494-011-9378-4
Hansen, M., Sørensen, N., and Michelsen, J. (1997). Extraction of Lift, Drag and Angle of Attack from Computed 3-d Viscous Flow Around a Rotating Blade. 1997 European Wind Energy Conference.
Hodgson, E. L., Andersen, S. J., Troldborg, N., Forsting, A. M., Mikkelsen, R. F., and Sørensen, J. N. (2021). A Quantitative Comparison of Aeroelastic Computations Using Flex5 and Actuator Methods in Les. J. Phys. Conf. Ser. 1934, 012014. doi:10.1088/1742-6596/1934/1/012014
Howland, M. F., Bossuyt, J., Martínez-Tossas, L. A., Meyers, J., and Meneveau, C. (2016). Wake Structure in Actuator Disk Models of Wind Turbines in Yaw Under Uniform Inflow Conditions. J. Renew. Sustain. Energy 8, 043301. doi:10.1063/1.4955091
IEA (2022). IEA Wind - Task 47. Tech. Rep. Availableat: https://iea-wind.org/task47/.
Jha, P. K., Churchfield, M. J., Moriarty, P. J., and Schmitz, S. (2014). Guidelines for Volume Force Distributions within Actuator Line Modeling of Wind Turbines on Large-Eddy Simulation-Type Grids. J. Sol. Energy Eng. Trans. ASME 136. doi:10.1115/1.4026252
Kheirabadi, A. C., and Nagamune, R. (2019). A Quantitative Review of Wind Farm Control with the Objective of Wind Farm Power Maximization. J. Wind Eng. Industrial Aerodynamics 192, 45–73. doi:10.1016/j.jweia.2019.06.015
Larsen, T., and Hansen, A. (2007). How 2 HAWC2, the User’s Manual. Roskilde, Denmark: Risø National Laboratory. Tech. Rep.
Leonard, B. P. (1979). A Stable and Accurate Convective Modelling Procedure Based on Quadratic Upstream Interpolation. Comput. Methods Appl. Mech. Eng. 19, 59–98. doi:10.1016/0045-7825(79)90034-3
Li, A., Gaunaa, M., Pirrung, G. R., Meyer Forsting, A., and Horcas, S. G. (2022). How Should the Lift and Drag Forces Be Calculated from 2-d Airfoil Data for Dihedral or Coned Wind Turbine Blades? Wind Energy Sci. Discuss. 2022, 1–40. doi:10.5194/wes-2021-163
Mann, J. (1994). The Spatial Structure of Neutral Atmospheric Surface-Layer Turbulence. J. Fluid Mech. 273, 141–168. doi:10.1017/S0022112094001886
Mann, J. (1998). Wind Field Simulation. Probabilistic Eng. Mech. 13, 269–282. doi:10.1016/S0266-8920(97)00036-2
Martínez, L., Leonardi, S., Churchfield, M., and Moriarty, P. (2012). A Comparison of Actuator Disk and Actuator Line Wind Turbine Models and Best Practices for Their Use. doi:10.2514/6.2012-900
Martínez-Tossas, L. A., Churchfield, M. J., and Leonardi, S. (2014). Large Eddy Simulations of the Flow Past Wind Turbines: Actuator Line and Disk Modeling. Wind Energy 1–20. doi:10.1002/we.1747–
Martínez-Tossas, L. A., and Meneveau, C. (2019). Filtered Lifting Line Theory and Application to the Actuator Line Model. J. Fluid Mech. 863, 269–292. doi:10.1017/jfm.2018.994
Menter, F. (1993). Zonal Two Equation K-W Turbulence Models for Aerodynamic Flows. doi:10.2514/6.1993-2906
Meyer Forsting, A. R., Pirrung, G. R., and Ramos-García, N. (2019a). A Vortex-Based Tip/Smearing Correction for the Actuator Line. Wind Energy Sci. 4, 369–383. doi:10.5194/wes-4-369-2019
Meyer Forsting, A. R., Pirrung, G. R., and Ramos-García, N. (2020). Brief Communication: A Fast Vortex-Based Smearing Correction for the Actuator Line. Wind Energy Sci. 5, 349–353. doi:10.5194/wes-5-349-2020
Meyer Forsting, A. R., Pirrung, G. R., and Ramos-García, N. (2019b). The Wake of an Actuator Line with a Vortex-Based Tip/Smearing Correction in Uniform and Turbulent Inflow. J. Phys. Conf. Ser. 1256, 012020. doi:10.1088/1742-6596/1256/1/012020
Michelsen, J. A. (1992). Basis 3D—A Platform For Development Of Multiblock PDE Solvers. Lyngby, Denmark: Danmarks Tekniske Universitet. Tech. Rep.
Michelsen, J. A. (1994). Block Structured Multigrid Solution of 2D and 3D Elliptic PDE’s. Tech. Rep. AFM, DTU.
Nathan, J. (2018). Application of Actuator Surface Concept in LES Simulations of the Near Wake of Wind Turbines. Ph.D. thesis.
Nathan, J., Meyer Forsting, A. R., Troldborg, N., and Masson, C. (2017). Comparison of Openfoam and Ellipsys3d Actuator Line Methods with (New) Mexico Results. J. Phys. Conf. Ser. 854, 012033. doi:10.1088/1742-6596/854/1/012033
Pirrung, G. R., Madsen, H. A., Kim, T., and Heinz, J. (2016). A Coupled Near and Far Wake Model for Wind Turbine Aerodynamics. Wind Energy 19, 2053–2069. doi:10.1002/we.1969
Sarmast, S., Shen, W. Z., Zhu, W. J., Mikkelsen, R. F., Breton, S. P., and Ivanell, S. (2016). Validation of the Actuator Line and Disc Techniques Using the New Mexico Measurements. J. Phys. Conf. Ser. 753, 032026. doi:10.1088/1742-6596/753/3/032026
Schepers, J., Boorsma, K., Madsen, H., Pirrung, G., Bangga, G., Guma, G., et al. (2021). IEA Wind TCP Task 29, Phase IV: Detailed Aerodynamics of Wind Turbines. Tech. Rep. doi:10.5281/zenodo.4813068
Shen, W. Z., Michelsen, J. A., Sørensen, N. N., and Nørkær Sørensen, J. (2003). An Improved Simplec Method on Collocated Grids for Steady and Unsteady Flow Computations. Numer. Heat. Transf. Part B Fundam. 43, 221–239. doi:10.1080/713836202
Shen, W. Z., Sørensen, J. N., and Mikkelsen, R. (2005). Tip Loss Correction for Actuator/Navier-Stokes Computations. J. Sol. Energy Eng. Trans. ASME 127, 209–213. doi:10.1115/1.1850488
Shives, M., and Crawford, C. (2012). Mesh and Load Distribution Requirements for Actuator Line Cfd Simulations. Wind Energy 16, a–n. doi:10.1002/we.1546
Shur, M. L., Spalart, P. R., Strelets, M. K., and Travin, A. K. (2008). A Hybrid RANS-LES Approach with Delayed-DES and Wall-Modelled LES Capabilities. Int. J. Heat Fluid Flow 29, 1638–1649. doi:10.1016/j.ijheatfluidflow.2008.07.001
Sørensen, J. N., Mikkelsen, R. F., Henningson, D. S., Ivanell, S., Sarmast, S., and Andersen, S. J. (2015). Simulation of Wind Turbine Wakes Using the Actuator Line Technique. Phil. Trans. R. Soc. A 373, 20140071. doi:10.1098/rsta.2014.0071
Sørensen, N. N. (1995). General Purpose Flow Solver Applied to Flow over Hills. Roskilde, Denmark: Risø National Laboratory.
Sørensen, J. N., and Shen, W. Z. (2002). Numerical Modeling of Wind Turbine Wakes. J. Fluids Eng. Trans. ASME 124, 393–399. doi:10.1115/1.1471361
Stevens, R. J. A. M., Martínez-Tossas, L. A., and Meneveau, C. (2018). Comparison of Wind Farm Large Eddy Simulations Using Actuator Disk and Actuator Line Models with Wind Tunnel Experiments. Renew. Energy 116, 470–478. doi:10.1016/j.renene.2017.08.072
Troldborg, N., Zahle, F., Réthoré, P.-E., and Sørensen, N. N. (2015). Comparison of Wind Turbine Wake Properties in Non-Sheared Inflow Predicted by Different Computational Fluid Dynamics Rotor Models. Wind Energy 18, 1239–1250. doi:10.1002/we.1757
Veers, P., Dykes, K., Lantz, E., Barth, S., Bottasso, C. L., Carlson, O., et al. (2019). Grand Challenges in the Science of Wind Energy. Science 366, eaau2027. doi:10.1126/science.aau2027
Wu, Y.-T., and Porté-Agel, F. (2011). Large-Eddy Simulation of Wind-Turbine Wakes: Evaluation of Turbine Parametrisations. Boundary-Layer Meteorol. 138, 345–366. doi:10.1007/s10546-010-9569-x
Keywords: validation, actuator line method, wind turbine modelling, fluid-structure interaction, vortex-based smearing correction, blade resolved, large eddy simulations, yaw misalignment
Citation: Hodgson EL, Grinderslev C, Meyer Forsting AR, Troldborg N, Sørensen NN, Sørensen JN and Andersen SJ (2022) Validation of Aeroelastic Actuator Line for Wind Turbine Modelling in Complex Flows. Front. Energy Res. 10:864645. doi: 10.3389/fenrg.2022.864645
Received: 28 January 2022; Accepted: 19 April 2022;
Published: 23 May 2022.
Edited by:
Paolo Schito, Politecnico di Milano, ItalyReviewed by:
Alessandro Bianchini, University of Florence, ItalyCopyright © 2022 Hodgson, Grinderslev, Meyer Forsting, Troldborg, Sørensen, Sørensen and Andersen. This is an open-access article distributed under the terms of the Creative Commons Attribution License (CC BY). The use, distribution or reproduction in other forums is permitted, provided the original author(s) and the copyright owner(s) are credited and that the original publication in this journal is cited, in accordance with accepted academic practice. No use, distribution or reproduction is permitted which does not comply with these terms.
*Correspondence: Emily L. Hodgson, ZW1saEBkdHUuZGs=
Disclaimer: All claims expressed in this article are solely those of the authors and do not necessarily represent those of their affiliated organizations, or those of the publisher, the editors and the reviewers. Any product that may be evaluated in this article or claim that may be made by its manufacturer is not guaranteed or endorsed by the publisher.
Research integrity at Frontiers
Learn more about the work of our research integrity team to safeguard the quality of each article we publish.