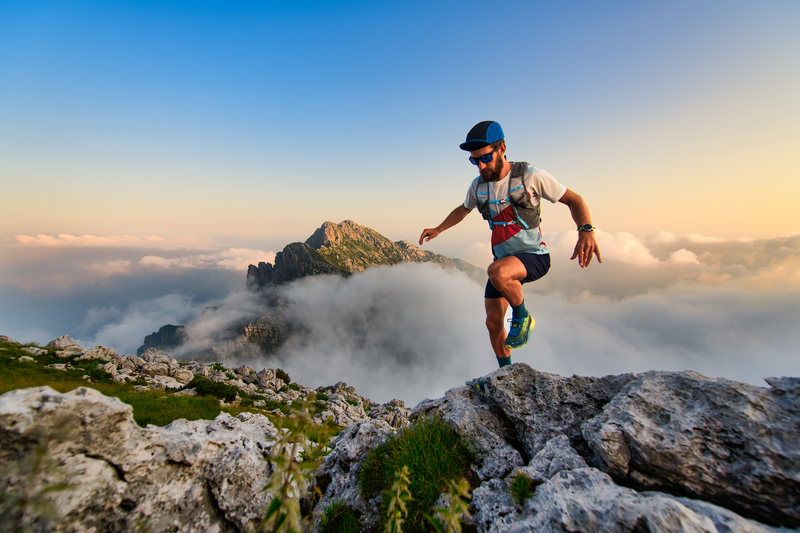
94% of researchers rate our articles as excellent or good
Learn more about the work of our research integrity team to safeguard the quality of each article we publish.
Find out more
REVIEW article
Front. Energy Res. , 15 July 2022
Sec. Bioenergy and Biofuels
Volume 10 - 2022 | https://doi.org/10.3389/fenrg.2022.863153
This article is part of the Research Topic Advancements in Biomass Feedstock Preprocessing: Conversion Ready Feedstocks, Volume II View all 16 articles
Biorefinery processes for converting lignocellulosic biomass to fuels and chemicals proceed via an integrated series of steps. Biomass is first pretreated and deconstructed using chemical catalysts and/or enzymes to liberate sugar monomers and lignin fragments. Deconstruction is followed by a conversion step in which engineered host organisms assimilate the released sugar monomers and lignin fragments, and produce value-added fuels and chemicals. Over the past couple of decades, a significant amount of work has been done to develop innovative biomass deconstruction and conversion processes that efficiently solubilize biomass, separate lignin from the biomass, maximize yields of bioavailable sugars and lignin fragments and convert the majority of these carbon sources into fuels, commodity chemicals, and materials. Herein, we advocate that advanced in silico approaches provide a theoretical framework for developing efficient processes for lignocellulosic biomass valorization and maximizing yields of sugars and lignin fragments during deconstruction and fuel and chemical titers during conversion. This manuscript surveys the latest developments in lignocellulosic biomass valorization with special attention given to highlighting computational approaches used in process optimization for lignocellulose pretreatment; enzyme engineering for enhanced saccharification and delignification; and prediction of the genome modification necessary for desired pathway fine-tuning to upgrade products from biomass deconstruction into value-added products. Physics-based modeling approaches such as density functional theory calculations and molecular dynamics simulations have been most impactful in studies aimed at exploring the molecular level details of solvent-biomass interactions, reaction mechanisms occurring in biomass-solvent systems, and the catalytic mechanisms and engineering of enzymes involved in biomass degradation. More recently, with ever increasing amounts of data from, for example, advanced mutli-omics experiments, machine learning approaches have begun to make important contributions in synthetic biology and optimization of metabolic pathways for production of biofuels and chemicals.
Plant biomass is the most abundant renewable source of carbon accessible to humanity. Lignocellulosic biomass is primarily comprised of three natural polymers: cellulose, hemicellulose, and lignin (Higuchi, 1997) (Figure 1). Cellulose is a linear, homologous polymer consisting of β-D-glucose units bonded together by β-1-4-glycosidic bonds. The degree of polymerization (DP) of cellulose is variable in different types of biomass (Hallac and Ragauskas, 2011). For example, the DP of cellulose chains ranges from 10,000 to 15,000 for native wood and cotton, respectively. The glucose polymers of cellulose are held together by van der Waals bonds and networks of strong H-bonds, enforcing crystalline regions and leading to the great strength and recalcitrance of cellulose (Shen and Gnanakaran, 2009). These cellulose microfibrils are entangled with hemicellulose and lignin within the plant cell wall. Hemicellulose is a heteropolysaccharide composed of pentose polymers (xylose and arabinose) and hexose polymers (glucose, galactose, and mannose) with DP ranging from 50 to 200 monosaccharides (Farhat et al., 2017; You et al., 2019) and sugar (uronic) acids (Huffman, 2003). Lignin is the third component and comprises 15 – 35 wt% of lignocellulose. Lignin is a three-dimensional amorphous polymer composed of three phenylpropanoid monolignols: ρ-coumaryl, coniferyl, and sinapyl alcohols, which in the lignin polymer are the ρ-hydroxyphenyl (H), guaicyl (G), and syringyl (S) units, respectively. The ratio of the three monolignols varies among plant phenotypes, resulting in many different lignin forms. The creation of a variety of linkages among these monolignols during lignin polymerization in the cell wall makes lignin a highly branched complex heterologous polymer. These linkages between H, G and S subunits are β-O-4′, β-5, α-O-4, 4-O-5′, β-β in primary and β-1′, and 5-5′ in minor content (Yoo et al., 2016). The predominant linkages in lignin are beta-aryl ether bonds, typically 50% in softwood and 60% in hardwood (Yoo et al., 2016). Several parameters affect the structure of lignocellulose, including the DP of cellulose fibers, the degree of crystallinity, how well the hemicellulose coats cellulose, the amount of lignin, and how the lignin protects the cellulose fibers. Therefore, the information on lignocellulose structure and composition will govern and direct strategies for deconstructing lignocellulose and converting it into value-added products.
FIGURE 1. Computational strategies used in process optimization for lignocellulose biomass deconstruction and upgrading to valuable fuels and chemicals.
Due to the complex nature of biomass and variation in its composition and structure, there is no universally optimized process for physical-chemical pretreatment and conversion of lignocellulosic biomass to valuable products (Ray et al., 2020). The recalcitrance of biomass to deconstruction and conversion is created in part by the crystallinity of cellulose, the complex interactions among cellulose, hemicellulose, and lignin, and the heterogeneity of lignin. This limits biomass deconstruction and escalates the pretreatment and enzymatic saccharification costs. To help optimize the processing steps, computational approaches have been used to develop a fundamental understanding of the interactions among biomass components and solvents for improving the accessibility to carbohydrate fibers before enzyme-catalyzed saccharification, the structure and function of biomass-degrading enzymes for engineering improved stability and activity under harsh pretreatment conditions, and the engineering of metabolic pathways in production hosts for improved titers and metabolic rates. The use of advanced computational techniques to optimize integrated pretreatment technologies (Figure 1, left panel) is discussed in Section 2. Computational methods, including quantum mechanical and molecular mechanical calculations, have been employed to help develop novel strategies for enzyme preparation, enzyme engineering, and enzyme mixture formulations to improve saccharification and lignolysis. Some of the latest studies on protein engineering approaches are discussed in Section 3 (Figure 1, middle panel). Finally, in Section 4 (Figure 1, right panel) of this review, we survey computational tools applied to system biology studies, including advanced technologies, multi-omics, and additional tools necessary for desired pathway engineering and fine-tuning to maximize yields from upgrading products produced during biomass deconstruction into value-added fuels, chemicals, and other products.
Lignocellulosic biomass, including human-inedible agricultural, forest, and herbaceous residues, stands out as a sustainable alternative for renewable, carbon-neutral production of fuels, chemicals, materials, and energy (Castilla-Archilla et al., 2019). However, the direct use of lignocellulosic biomass is restricted due to its recalcitrance to degradation, which is due to the strong covalent and hydrogen bond interactions among the complex chemical structure of its constituents, namely, cellulose, hemicellulose, and lignin, and thus, pretreatment is necessary (Gibson, 2012; Haghighi Mood et al., 2013). Existing approaches include biological, abiotic (physical, chemical, and physicochemical), and hybrid technologies (Figure 2) (Tu and Hallett, 2019). An ideal pretreatment technology would successfully disrupt the strong interactions among biopolymers, leading to their selective fractionation, minimize by-product formation, and be economically viable. Nevertheless, optimizing these objectives alone or in combination is essential to benefit the overall process, as each pretreatment technology, owing to its unique characteristics, is applicable to a specific biomass type and source. For example, physical pretreatment approaches are the most conventional methods for lignocellulosic biomass pretreatment. However, their limited scalability, high energy requirements, and multiple feedstock non-viability narrow their applicability. Chemical pretreatment methods involving hot water, dilute acid, ionic liquids, alkali, organic solvents (organosolv), and ammonia fiber expansion have been widely studied (Haghighi Mood et al., 2013; Bhardwaj et al., 2019). While requiring less energy and being generally non-toxic, biological pretreatments typically require longer retention times for effective pretreatment, hindering their commercial feasibility.
Pretreatment of lignocellulosic biomass facilitates the production of biologically available intermediates such as glucose, cellulose, and lignin fragments that can be converted to final products such as biohydrogen, biomethane, bioethanol, biomethanol, biobutanol, and bio-diesel. The efficiency of a given pretreatment process is a function of the constituents of the biomass and how they interact with the pretreatment process. Rigorous efforts to optimize a single biomass pretreatment technology or combinations of technologies have improved economic viability and environmental sustainability. However, the mission is still not accomplished, given the vast number of variables involved in optimizing pretreatment technologies. In this regard, computational tools that leverage experimental datasets have become essential in identifying sustainable and robust multi-product biorefinery methodologies. The field of computational chemistry and biology has become increasingly predictive in the twenty-first century, and active applications have been extended to studies, predictions, and optimization of biomass pretreatment technologies. These simulation approaches predict desired outputs based on existing experimental datasets in which pretreatment efficacy has been measured for a diverse set of feedstocks under a variety of pretreatment conditions. Computational methods used to understand and predict pretreatment efficacy have predominantly used atomistic physics-based modeling, but atomic scale machine learning methods are also being developed that have the potential to speed up pretreatment optimization.
Atomic-scale modeling techniques, including density functional theory (DFT) and molecular dynamics (MD), have been instrumental in advancing the understanding of experimental pretreatment results and predicting the properties of biomass-solvent systems at the level of atom-by-atom interactions among biomass, water, and solvent. DFT is a powerful tool for obtaining static properties such as local energy minima, reaction pathways, and transition states and calculating other thermodynamic properties for a relatively small system (tens to hundreds of atoms) (Cohen et al., 2012). For evaluation of larger systems (thousands to millions of atoms), MD simulations are critical in calculating equilibrium thermodynamic and physical properties (as a function of hydrogen bonds), sampling conformational states, and evaluating femtosecond to millisecond dynamics and the role of long-range forces (Rapaport et al., 1996; Frenkel and Smit, 2002). It is to be noted that classical MD simulations are limited to studying equilibrium states and properties of the system, and quantum chemistries such as bond-breaking and bond-forming are not considered. However, accurate force fields for calculating non-bonded interactions, including van der Waals and Coulombic interactions, are essential to understanding interactions between lignocellulosic biomass components and pretreatment solvents to design an efficient process. The total potential energy of the given lignocellulosic biomass system is calculated using empirical force fields such as GROningen MOlecular Simulation (GROMOS), Polymer Consistent Force Field (PCFF), and Chemistry at Harvard Macromolecular Mechanics (CHARMM). The MD methods using these force fields to study biomass–solvent interactions are discussed in the upcoming sections.
Machine learning (ML) approaches have the potential to generate predictive models of biomass pretreatment efficacy and would provide potentially much faster ways to evaluate and optimize pretreatment technologies. However, ML approaches require either very large databases from which to learn how to predict pretreatment outcomes from inputs and/or the ability to account for and features the atomic scale forces governing biomass–solvent interactions. Recently, neural networks have been developed that provide insights into the atomistic properties of molecules and are trained to look for a specific “structure” or “moiety” with a defined interaction or activity (Grisafi and Ceriotti, 2019). They computed the atom-global information on the structure and composition utilizing Smooth Overlap of Atomic Properties (SOAP), long-distance equivariant (LODE), and similar ideas to improve the accuracy and efficiency of long-range information. This ML approach provides a representation of the system that accounts for both short-range atomistic interactions and long-range interactions, giving it the potential to help design and optimize an efficient pretreatment method.
The structures of cellulose, hemicellulose, and lignin and their interactions play an essential role in accurately predicting the pretreatment efficacies, i.e., creating the initial coordinates in terms of biopolymer structure is vital for investigating their properties and their interactions with solvents (Ciesielski et al., 2020). Typical tools for building structures of sugar polymers include “Cellulose Builder” (Gomes and Skaf, 2012) and “doGlycans” (Danne et al., 2017). While the former facilitates the construction of any length of various cellulose crystalline forms such as Iα, Iβ, II, and III, the latter enables a structural topology for cellulose and hemicellulose. In 2003, amorphous and crystalline cellulose models were compared using MD simulations for the first time. The study also analyzed various properties and showed that their conformational states, density, and hydrogen bonding networks were consistent with available experimental data (Mazeau and Heux, 2003). Since then, significant progress has been made in modeling cellulose crystal structures and their hydrogen-bonding networks (Ciesielski et al., 2020). MD simulations have also been employed to study twisted conformations of cellulose around their glycosidic linkages, as was observed in atomic force and transmission electron microscopy studies (Hanley et al., 1997; Bowling et al., 2001). The deviation of glycosidic linkages from 180° was suggested using MD simulations; however, the twisted microfibril was unstable and reverted to the original untwisted structure independent of the temperature (Matthews et al., 2011; Matthews et al., 2012). In another interesting study, the anti- and syn-conformations of four different glucan decasaccharides were evaluated using the CHARMM36 force field and compared with small-angle X-ray scattering to unveil the glycosidic linkage flexibility (Kameda et al., 2018). MD simulation of branched hemicelluloses revealed the higher stiffness of the glucomannan backbone compared to the xylan backbone (Martínez-Abad et al., 2017), stability of various conformers (Berglund et al., 2019), and impact of acetylation on cellulosic interactions (Busse-Wicher et al., 2014).
Similarly, “Lignin Builder” (Vermaas et al., 2018) enables the design of representative lignin linkages into models of lignin polymers (hardwood, softwood, and grass) for simulation studies. Several other approaches based on kinetic Monte Carlo and DFT have also been reported to generate lignin structures (Zhang et al., 2011; Dellon et al., 2017; Orella et al., 2019). Based on the structures developed, quantum chemical calculations using AM1, HF, and DFT/B3LYP levels of theory were employed to examine the interaction characteristics such as hydrogen bonding between cellulose-hemicellulose and covalent bond linkages for hemicellulose-lignin systems (Zhang et al., 2011). These studies predicted the potential to disrupt or dissociate C1-O bonds in xylan-lignin complexes and β-O bonds in lignin-glucomannan complexes during pretreatment. In addition, an exciting study highlighted the variability of interaction energy based on the orientation of these biopolymers, i.e., a stacked configuration between polymers affords higher interactions (Yang et al., 2019). As discussed in the next section, these builder tools have facilitated the building of various lignocellulosic models and the understanding of interactions between biomass and various solvents.
In this section, we will limit our discussions to molecular and ionic solvent-based pretreatment technologies. The limitless possibilities of molecular and ionic solvents limit the full exploration of every unique combination within the context of experimental methodologies. Computational methods have been used to help understand the dominant factors governing the efficacy of solvent-based pretreatment of lignocellulosic biomass. Typically, in biomass pretreatment, quantum chemical (QC) and MD simulations have been adapted to understand the interactions of the various biomass components with the pretreatment solvent, which in turn helps to understand and predict the fractionation abilities of the solvent (or solvent class) under consideration (Table 1). Also, pre-existing solubility parameters such as Hildebrand (Quesada-Medina et al., 2010), Hansen solubility parameters (HSP) (Hansen, 2007; Cheng et al., 2018), and Conductor like Screening Model for Real Solvents (COSMO-RS) models have been extensively studied for several chemical pretreatment technologies employing organic solvents, deep eutectic solvents, and ionic liquids (Balaji et al., 2012; Casas et al., 2013; Achinivu et al., 2021). Recently, ionic liquids (salts possessing organic cations with a melting point below 100°C) have attracted significant attention as a promising pretreatment solvent. Several modeling methods have been developed to understand how these solvents fractionate or solubilize lignocellulosic biomass. For instance, the solubility of lignin in a given solvent was determined based on the Hildebrand solubility and thermodynamic parameters such as activity coefficients and excess enthalpy (Casas et al., 2012). These studies concluded that more robust exothermic behavior and lower activity coefficient values are required for enhanced interaction/solubility for any given solute-solvent pair. In another instance, density functional theory (DFT) studies were employed to calculate the hydrogen bonding interaction between solvent (ionic liquid) and biopolymer (lignin) to determine the pretreatment efficacies of these solvents (Rashid et al., 2016; Zhang et al., 2017). Dispersion-corrected DFT models established the role of cations in regulating the solubilities of lignocellulosic components as a function π-stacking (Janesko, 2011). Ji and Lv suggested that both C-H···π and strong hydrogen bonding are critical to enhanced delignification performance using three solvent systems, namely para-toluenesulfonic acid, choline chloride-lactic acid eutectic, and 1-allyl-3-methylimidazolium chloride (Ji and Lv, 2020). Singh et al. have extensively studied the dissolution of cellulose in pure 1-ethyl-3-methylimidazolium acetate ([C2mim][OAc]) and mixtures of [C2mim][OAc] and water systems (Liu H. et al., 2010; Shi et al., 2014; Parthasarathi et al., 2015). The role of water as cosolvent was established in these studies, identifying the “ideal” IL-water ratio (4:1 for [C2mim][OAc] and water system) for maximum disruption of intermolecular hydrogen bonding within cellulose.
TABLE 1. Molecular simulation techniques used for understanding interactions between biomass-solvent.
Interestingly, the simulation studies suggested repacking de-crystallized cellulose into an amorphous form with high water content in pure [C2mim][OAc]. A recent study by Achinivu et al. and team has screened various structurally and functionally distinct amines and developed a toolset to provide rapid identification of effective pretreatment solvents (Achinivu et al., 2021). This study employed a theoretical analysis (validated by an experimental dataset) to develop a predictive model for a given class of solvent. In the first step, the interaction of biomass with organic solvents with various functional groups was studied using HSP and COSMO-RS toolsets to reveal amines as better solvents for lignocellulosic pretreatment. Then, the differences in the interactions of various amines were studied using the quantum theory of atoms in molecules (QTAIM) and QC calculations (interaction energies and natural bond orbital (NBO) analysis) to suggest the importance of electrostatic interactions and hydrogen bonding between amines and lignin for enhanced solubility. Various computational studies of ionic liquid solvent systems in the pretreatment of biomass have heavily relied on COSMO-RS predictions and hydrogen bond basicity to predict the biopolymer solubilities in the ionic liquid (Chu and He, 2019; Iqbal et al., 2019). Yao et al. extended the application of COSMO-RS prediction to demonstrate the synergistic advantages of multiple ions in a cholinium-based ionic liquid system for the pretreatment of sorghum biomass (Yao et al., 2021). Mood et al. showed that solubility parameters of ionic liquids and deep eutectic solvents calculated using COSMO-RS are predictive of lignin solubility under the like-dissolves-like principal and developed a new method to calculate solubility parameters from MD simulation trajectories, allowing predictions to be made for much larger lignin polymers (Mohan et al., 2021; Mohan et al., 2022).
In summary, molecular simulations are necessary to support experiments and provide critical missing links that experiments cannot access, considering the complexity of the lignocellulosic biomass structure. MD simulations are used to account for the dynamical behavior of molecular systems and understand solvent–biomass interactions during pretreatment. In contrast, reactive force fields, which rely on the bond order, bond distance, and bond energy, provide a detailed explanation of bond breaking and bond-forming reactions during simulations. Multiscale modeling has been used to successfully investigate physical and chemical properties, reaction mechanisms, and overall system dynamics. These tools and approaches also help understand the structure-activity relationships and assist in developing novel solvents and maximizing yields or selectivity in multi-product biorefinery settings. These computational toolsets have the potential to speed up the design, development, and deployment of novel solvents for a robust biorefinery and, when eventually combined with machine learning, will provide tools for rapidly evaluating potential new solvents and deconstruction processes. Despite all these developments in both computational and experimental techniques, a knowledge gap still exists in developing a robust multi-product biorefinery, namely: 1) complete insights into the whole molecular structure of biomass; 2) efficient and robust processes for the conversion of biomass to chemicals; 3) mechanistic understanding of the role of solvents during pretreatment; and 4) key interactions and orientations of polymer components. In addition, some of these simulations are fundamentally expensive as they may take several days depending on the complexity of the molecule involved. The combination of experiments including imaging and spectroscopy, computational modeling, and/or machine learning is expected to contribute to the future design of stratified structures of lignocellulosics and generate a large number of structured materials and chemicals in the future. Furthermore, the understanding developed through the optimization of biomass pretreatment using these simulation tools will promote overall process yields while reducing energy requirements and carbon footprints.
A variety of computational methods utilize new advances in computing to understand the underlying mechanisms of the biocatalysts used to promote efficient biotransformation of substrates into value-added products. These computational methods, which differ in computational cost and accuracy, can simulate biocatalytic processes at different molecular levels. For instance, protein-ligand or enzyme-substrate docking is a molecular modeling technique that can predict a ligand’s position and orientation (substrate, a small molecule) when it is bound to a protein/enzyme (Kearsley et al., 1994; Friesner et al., 2004; Wang and Pang, 2007; Zsoldos et al., 2007). Modeled interactions between an enzyme and its substrate(s) provide insights into predicting the activation or inhibition of an enzyme and providing information for rational enzyme design. In this method, dealing with receptor flexibility in docking methodologies is still challenging due to the large number of degrees of freedom of the enzyme the calculation needs to consider (Totrov and Abagyan, 2008; Cerqueira et al., 2009; Antunes et al., 2015). The enzyme-ligand complex is often the starting point for molecular dynamics (MD) simulations, which are used to analyze the physical movements of atoms and molecules and the time evolution of enzyme-substrate interactions (Levitt and Warshel, 1975; Warshel, 1976). MD simulations explore the time evolution of a particular interacting system for a fixed period of time on nano to microsecond time scales, and the trajectories of atoms and molecules are determined by numerically solving Newton’s equations of motion. Theoretical studies utilizing MD simulation provide insights into the intricate dynamics of biological macromolecules (protein or protein-ligand complex) through observing crucial interactions (e.g., hydrophobic interactions, van der Waals, hydrogen bonds), thus understanding protein folding and unfolding, protein stability, and conformational changes (Levitt, 1982; Moal and Bates, 2012; Khan et al., 2016). However, simulation accuracy is strongly dependent on the quality of ligand parameterization, which can be improved by using high-accuracy quantum mechanics/molecular mechanics (QM/MM) methods (Warshel and Levitt, 1976; Brunk and Rothlisberger, 2015). This method combines the strengths of ab initio QM calculation (accuracy) and MM (speed) methods. However, it demands the high computational cost of conformational searching and the limitations of implicit solvation effects. In the last two decades, hybrid QM/MM calculations have become a powerful approach to studying enzymatic reactions (Martí et al., 2004; Mulholland, 2005; Riccardi et al., 2006; Senn and Thiel, 2009; Warshel, 2014).
There has been exhaustive research to improve individual enzyme characteristics through either rational design or directed evolution strategies. The rational approach to protein engineering via computational methods facilitates development in this field. Simulation of an enzyme structure, substrate, or complex cam provides molecular and structural mechanisms of enzymatic action. This section highlights the increasing evidence of computational modeling methods as a powerful tool in the study and engineering of hydrolases and oxidoreductases, especially for their application as biocatalysts in lignocellulose deconstruction. We compiled and tabulated the computational methods in the studies cited below in Table 2.
Cellulases are divided into three groups: endoglucanases (EC 3.2.1.4), cellobiohydrolases (EC 3.2.1.91), and β-glycosidases (3. 2. 1. 21), that work synergistically to catalyze the conversion of cellulose to glucose in a process known as saccharification. Endoglucanases (EGs) catalyze the breaking of internal glycosidic bonds of the amorphous part of the cellulose chain, producing new ends of glucose polymers (Medve et al., 1998). Cellobiohydrolases (CBHs), also called exoglucanases, bind to these newly created ends and catalyze hydrolysis of glycosidic bonds in glucose polymers, producing cellobiose (Teeri, 1997). Finally, β-glycosidases catalyze the break of glycosidic bonds in cellobiose, producing glucose monomers (Riou et al., 1998; Decker et al., 2001) and are typically the rate-limiting step in the full conversion of cellulose to glucose. Due to the recalcitrant nature of lignocellulosic, saccharification of cellulose to glucose is slow, and in the second generation of conversion of lignocellulose to ethanol requires several-fold more active hydrolytic enzymes than for saccharification from starch (Balan, 2014). Low activity and high costs of cellulases are the bottlenecks for their industrial use in the valorization of lignocellulosic biomass. Some endoglucanases and cellobiohydrolases are composed of a catalytic domain (CD) and a carbohydrate-binding domain (CBD). The CBD increases enzymatic activity on specific and solid substrates and helps disrupt the crystalline structure of cellulose. Several MD simulation studies have been conducted to understand cellulase adsorption to cellulose and the role of CBDs. A hundred nanosecond timescale MD simulations of Cel7A from Geotrichum candidum strain 3C (GcaCel7A) were studied in three different forms: free form, in complex with a cellononaose substrate, and in complex with microfibrils of cellulose. These simulations revealed a significant difference in the dynamics of substrate-bound enzymes compared with free enzymes (Borisova et al., 2015). Similar studies on substrate-bound enzymes revealed the cellulose-binding site was highly conserved in other cellulases, Cel7A from Heterobasidion irregulare (HirCel7A), Heterobasidion jecorina (HjeCel7A), and Cel7D from Phanerochaete chrysosporium (PchCel7D), and PfCBH1 from Penicillium funiculosum when bound with cellononaose and microcrystalline cellulose (Momeni et al., 2013; Ogunmolu et al., 2017). Forty nanosecond MD simulations of Trichoderma reesei Cel6A and Cel7A showed the flexible glycosylated linkers of CBD bind nonspecifically to cellulose and can serve as the rate-limiting step in cellulose degradation (Payne et al., 2013; Knott et al., 2014). While MD simulations have been used to study the interaction between cellulases and cellulose and cellulase CBDs and cellulose, QM/MM simulations have been used to reveal the transition state of oligosaccharide hydrolysis (Liu J. et al., 2010; Li et al., 2010; Wang et al., 2011; Wang et al., 2016; Iglesias-Fernández et al., 2017; Zong et al., 2019; Bharadwaj et al., 2020; Pereira et al., 2021). Li et al. elucidated the mechanism of enzymatic catalysis of cellulase Cel7A from Trichoderma reesei (Li et al., 2010). At the level of accuracy of the applied theory, detailed structural and energetic information revealed an S(N)2-type-like mechanism via loose transition state structures. In similar work using QM/MM, an endocyclic mechanism for PcCel45A was revealed in which an acyclic oxocarbenium-like transition state is stabilized, leading to the opening of the glucopyranose ring and the formation of an unstable acyclic hemiacetal that can be readily decomposed into hydrolysis products (Pereira et al., 2021).
Hemicellulases are a group of enzymes that catalyze the hydrolysis of galactans, xylans, and mannans. The primary enzyme is endoxylanase (EC 3.2.1.8), which hydrolyzes β-d xylano pyranosyl linkages of xylan to form xylooligosaccharides. Secondly, β-D xylosidase (EC 3.2.1.3, xylobiase) catalyzes the hydrolysis of xylobiose or xylooligosaccharides from the nonreducing end, producing D-xylose sugar in the hydrolysates. Control of the desired xylooligosaccharide size range is one of the most challenging studies in xylose degradation, and several endoxylanase engineering attempts have been aimed at changing the range of xylooligosaccharides produced. For example, Pollet et al. engineered the BsXynA xylanase from Bacillus subtilis by replacing a Tyr at the binding site with an Ala and improved the variety of xylooligosaccharides produced by the enzyme (Pollet et al., 2010). A similar catalytic pattern in T-Xyn xylanase from Talaromyces thermophilus F1208 was revealed by double mutations at a region near the N-terminal and the C-terminal, which resulted in the absence of xylose monomer product (Li et al., 2017). Atomistic MD simulations were used to understand the mechanisms underlying these efficiency losses. The MD trajectory analysis suggested that the mutation-induced binding pocket tilting resulted in an additional hydrophobic contact between the reducing end of xylooligosaccharides and Trp128 (Ngenyoung et al., 2021).
A secondary binding site (SBS) on the surface of the GH11 xylanases has been discovered in a few endoxylanases from Bacillus subtilis (PDB ID: 2QZ3) (Cuyvers et al., 2011), Aspergillus niger (PDB ID: 2QZ2) (Vandermarliere et al., 2008), and Bacillus circulans (BcX) (PDB ID: 1XNB) (Ludwiczek et al., 2007). Recently, MD simulations of PxXyn11B from Paenibacillus xylanivorans A57 revealed an essential role of SBS in the activity and conformational mobility of the enzyme, demonstrating that the SBS stabilizes ligand binding, allowing it to be bound within the active site for a longer time period and resulting in more controlled enzymatic breakdown to products (Briganti et al., 2021). These findings explain the observed enzyme kinetics and shed light on the product control of the xylanase enzymes by protein engineering.
Lignin-modifying enzymes (LMEs) are enzymes produced by fungi and bacteria that catalyze bond breaking of a variety of bonds in lignin polymers to degrade lignin to bioavailable substrates. In nature, these lignin fragments are consumed by microbes, and in synthetic biology applications they are fed to organisms engineered to convert them into biofuels and bioproducts. LMEs include peroxidases, such as lignin peroxidase (LiP, EC 1.11.1.14), manganese peroxidase (MnP, EC 1.11.1.13), versatile peroxidase (VP, EC 1.11.1.16), and many phenol oxidases of the laccase type (EC 1.10.3.2). LiP and MnP contain a heme-iron in their active sites that participates as a reducing agent in the general peroxidase catalytic mechanism. The heme-iron is first oxidized by hydrogen peroxide, and electrons are then shuttled from lignin through soluble mediators such as phenolic veratryl alcohol or, in the case of MnP, Mn(II). VP shares the structural and catalytic properties of both LiP and MnP (Ruiz-Dueñas and Martínez, 2009). Laccases are multi-copper oxidases that catalyze one-electron oxidation of a wide range of phenolic compounds (Hamid and Khalil ur, 2009; Pollegioni et al., 2015). Various computation-aided studies have attempted to improve the catalytic efficiency of LDEs through understanding lignin-aromatic compound binding modes, critical structures that impact oxidative power, and electron transfer pathways, using the approaches elaborated in the following paragraphs.
Molecular docking studies have been carried out to predict the binding modes of aromatic substrates and lignin model compounds to LiP, MnP, and laccase, and MD simulations were performed to study the resulting enzyme-substrate complexes (Borrelli et al., 2005; Chen et al., 2011; Fernández-Fueyo et al., 2014; Singh et al., 2021). Rational enzyme engineering of MnP6 from C. subvermispora has also been carried out with the help of computational methods (Acebes et al., 2016). Acebes et al. started by using the protein energy landscape exploration (PELE) algorithm to inspect the active sites of both systems, ABTS-MnP6 and ABTS-MnP4. These explorations showed that in the energetically minimum structure of MnP4 located at the main heme channel, two histidines, H220 and H142, interacted, forming hydrogen bonds with the negatively charged sulfonates of ABTS. Furthermore, the high-performance molecular dynamics simulations-DESMOND were recently used to perform deep, rigorous structural and functional fluctuation analyses of docked complexes between lignin model compounds and LiP. The findings demonstrated that LiP interacts with chlorinated compounds through ionic interaction, while hydrophobic and H-bond contacts have been observed in all lignin-model compounds (Singh et al., 2021).
The oxidative power (redox potential) of LDE is a critical factor in the successful degradation of bulky and recalcitrant lignin substrates. QM/MM simulations have been used to identify specific amino acids that influence the oxidative power of LiP, which suggested mutations with higher oxidative abilities or with the capacity to function under different pH conditions (Castro et al., 2016; Pham et al., 2016; Kohler et al., 2018; Singh et al., 2021). Recently, using ab initio molecular dynamic simulations and climbing-image Nudge Elastic Band-based transition state searches, Pham et al. suggested the effect of lower pH on LiP activity is via protonation of aliphatic hydroxyl groups, which resulted in lower energetic barriers for bond-cleavages, particularly β-O-4′ bonds (Pham et al., 2021). Molecular mechanical free-energy perturbation (QM/MM-FEP) methods in combination with explicit solvent simulations have been used to study the redox potentials (RP), acidity constants, and isomerization reactions of the laccases (Hong et al., 2011; Vázquez-Lima et al., 2012; Li J. et al., 2015; Götze and Bühl, 2016). More recently, the pH dependence and effect of mutants on the laccase redox potentials at the T1 site were studied with QM/MM approaches. The authors found that the oxidation state of the surrounding residues affected the T1 copper site redox potential by about 0.2–0.3 V and was changed to −1.37 V when the replacement of a protonation state corresponded to a neutral environment. The predicted change in the redox potential of the F463M mutant (−0.1 V) was consistent with observations for a related laccase (Götze and Bühl, 2016).
The extant peroxidases (LiP and VP), which have high redox potentials, proved their ability to degrade non-phenolic lignin by using a tryptophanyl radical interacting with the bulky polymer at the surface of the enzyme (Ayuso-Fernández et al., 2018). LiP oxidizes different non-phenolic lignin model compounds, including β-O-4 linkage-type arylglycerol-aryl ethers, forming a radical cation through one-electron oxidation. Radical cation formation leads to side-chain cleavage, demethylation, intramolecular addition, and rearrangements (Kirk et al., 1986; Miki et al., 1986; Wong, 2009). Oxidation of non-phenolic aromatic substrates of high redox potential such as veratryl alcohol (VA) was mediated through the tryptophan radical (Trp171) present in LiP from Phanerocheate chrysosporium, which has been elucidated through QM/MM calculations at the B3LYP/CHARMM level of theory (Bernini et al., 2012; Romero et al., 2019). The experimental work was performed to validate these calculations through the catalytic engineering activity of Coprinus cinereus peroxidase (CiP) (Smith et al., 2009). By mimicking the surroundings of Trp171 in Phanerocheate chrysosporium LiP, some specific acid residues were introduced to the catalytic Trp178 in CiP to create variant D178W/R257E/R271D. The EPR characterization crucially showed that [Fe(IV) = O Trp-179(*)] in engineered CiP was the reactive intermediate with veratryl alcohol (Smith et al., 2009). Similar works reported the electron transfer mechanism of VA at Tyr181 in Trametopsis cervina LiP (Miki et al., 2013), at Trp164 in VP from Pleurotus eryngii (Pogni et al., 2006; Bernini et al., 2014; Acebes et al., 2017) and ABTS oxidation at Y247 in Klebsiella pneumoniae dye-decolorizing peroxidase (KpDyP) (Nys et al., 2021).
The MD, QM, and QM/MM simulations were mainly presented as auxiliary tools to explain the experimental evidence in aspects like cellulose and lignin fractionation reported for these enzymes. A broad set of molecular and computational tools allowed the creation of models and more efficient screening methods. We believe that, during subsequent years and advances in hardware, software, and algorithms, more accurate and predictive computational tools will greatly benefit studies aimed at rational protein design to improve the catalytic activity of a given hydrolase and oxidoreductase for a specific substrate. The conjunction of these experimental and computational techniques will help design more efficient biocatalysts for lignocellulosic bioconversion.
In addition to the three classes of synergistic cellulolytic enzymes and the various lignin modifying enzymes described above, additional auxiliary enzymes have been implicated as being required for maximum bioconversion of lignocellulosic biomass to compounds amenable to biological uptake and conversion. Besides cellulolytic enzymes that cleave off various monosaccharide side chains, other enzymes are required for the deprotection of the polysaccharide backbone. Polysaccharide sulfatases remove sulfate ester groups (Bäumgen et al., 2021), while carbohydrate esterases catalyze the cleavage of O- and N-acetyl groups from carbohydrates (Davies et al., 2005). In contrast to the carbohydrate esterases (CEs), the sulfatases are not included in the Crazy database but are listed in the SulfAtlas database instead (Barbeyron et al., 2016).
Lignin-degrading auxiliary enzymes enable lignin degradation through the sequential action of several proteins that may include oxidative H2O2 (Kumar and Chandra, 2020). This group includes cellobiose dehydrogenase, aryl alcohol oxidases, glyoxal oxidase, glucose oxidase, and pyranose 2-oxidase (Kumar and Chandra, 2020). Lignin-degrading auxiliary enzymes cannot alone catalyze complete depolymerization of lignin and typically work synergistically with additional enzymes. The auxiliary enzyme classes, such as redox enzymes, act in conjunction with other CAZymes, including lytic polysaccharide monooxygenases (LPMOs), lignin peroxidases and laccases. LPMO activity has been described as crucial during cellulose hydrolysis and is currently present in commercial cellulase preparations. These enzymes have also been studied by molecular dynamics simulations to understand binding properties and to design rational engineering approaches (Liu et al., 2018; Guo et al., 2020). In particular, MD simulations suggest roles for both aromatic and acidic residues in the substrate-binding of LPMO from the white-rot fungus Heterobasidion irregulare (Liu et al., 2018). This study provided additional insight into cellulose binding by C1-specific LPMOs, giving a molecular-level picture of active site substrate interactions. Furthermore, a combination of information from calculations run on the HotSpot Wizard 3.0, dezyme web server, and MD simulations in the study of LPMO from Myceliophthora thermophila C1, was used to rationally design a mutant (R17L) LPMO with a 1.8-fold increase in specific activity and a 1.92-fold increase in catalytic efficiency (kcat/Km). The increased degree of the reducing sugar yield from microcrystalline cellulose and three plant biomass materials during hydrolysis using cellulase in combination with the R17L LPMO mutant was approximately two times higher than with the WT LPMO (Guo et al., 2020).
Systems biology follows a holistic approach to analyzing cell biology from subcellular levels to the entire organism using computer-aided tools and mathematical models (Kitano, 2002). System biology tools include the following advanced omics technologies: Genomics – the set of studies on the structure, function, evolution, mapping, and editing of genomes (Khoury et al., 2009); Transcriptomics - the complete set of RNA transcripts (Piétu et al., 1999); Proteomics – investigation of protein production, degradation, modification, and their interactions (Dupree et al., 2020); and Metabolomics - chemical processes involving small molecule substrates, intermediates, and products of cell metabolism (Clish, 2015). Each omics platform requires data handling, annotation of biomolecules, design and analytic assumptions, statistical power analysis, and data archiving and sharing. However, a single omics analysis can’t fully solve the complexities of microbial biology. Multi-omics techniques have opened new avenues for exploring microbial diversity by contributing to available databases (metabolite, RNA, DNA, and protein databases) at a scale not imagined previously. Integrating such diverse data types requires data normalization, statistical power analysis, and big-data machine-learning tools for a multi-omics analysis (Libbrecht and Noble, 2015; Min et al., 2016). Available computational tools for interpretation and analysis of analysis of omics data and integration of genomics and metabolomics data include MapMan (Thimm et al., 2004), Pathway Studio (Yuryev et al., 2009); for transcriptomics and proteomics data include iCluster (Shen et al., 2009), SteinerNet (Tuncbag et al., 2012), Paintomics (Hernández-de-Diego et al., 2018); for transcriptomics and metabolomics data include Paintomics (Hernández-de-Diego et al., 2018), PRIMe (Akiyama et al., 2008), MEtaboAnalyst 5.0 (Pang et al., 2021) and for multi-omics data include IntegrOmics (Lê Cao et al., 2009), 3Omics (Kuo et al., 2013), Qiagen Ingenuity Pathway Analysis (Krämer et al., 2014). Multi-omics approaches have been applied in several research areas, from bio-based fuel production to biopharmaceutical development to studies of diseases. This section will highlight some multi-omics-aided studies for lignocellulose valorization.
The massive development in next-generation sequencing (NGS) technologies has led to extensive publicly available genomics databases. However, genome mining is dependent solely on computational methods and bioinformatics tools to interconnect the complex biological networks within a single species and across microbial species. The recent development in genome mining tools such as antiSMASH, ClustScan, BAGEL, SMURF, NP.searcher, and PRISM has untapped the metabolic potential of microorganisms for biomass degradation (Lee N. et al., 2020). These tools can be used to scan the genomes of multiple organisms simultaneously to predict homologous genes based on highly conserved sequences. In their review, Ren et al. have covered the progress of genome-mining tools for predicting natural products of pharmaceutical importance (Ren et al., 2020). The majority of them are used for pathway prediction by identifying essential genes involved in metabolite synthesis and utilizing Basic Local Alignment Search Tool (BLAST) or hidden Markov models (HMMs) for genome mining (Ren et al., 2020). In one metagenomic study, stable isotope probing (SIP) was used to identify and characterize the microbiome in different soil layers for lignocellulose degradation (Wilhelm et al., 2019). The identification and genomic content of bacterial consortia were assessed using 16S rRNA gene amplicon and shotgun metagenomics. In another study, the comparative genomic analysis of C. subvermispora and P. chrysosporium revealed the presence of more than seven genes encoding laccases in C. subvermispora. In contrast, there was no gene encoding for laccase for lignin degradation in P. chrysosporium. The same study identified that the C. subvermispora genome contains as many as three times more genes for MnP than P. chrysosporium (Fernandez-Fueyo et al., 2012).
Proteomic analysis is used to identify enzymes present and to quantify the expression of enzymes and has proved helpful for identifying the CAZymes in microbes involved in biomass deconstruction. LC-MS/MS-based secretome profiling from several microorganisms grown on different substrates revealed the presence of laccases, auxiliary proteins, and hydrolases (Sethupathy et al., 2021). Proteomic analysis of the ligninolytic bacterium Arthrobacter phenanthrenivorans Sphe3 on a medium containing three different carbon sources identified several enzymes involved in catalysis of aromatic degradation, including phenanthrene (Vandera et al., 2015). The study also identified genes involved in catabolite repression in the presence of glucose. When exposed to three xenobiotics, a similar study performed in Sphingobium chungbukense DJ77 identified major proteins to map catabolic pathways for naphthalene, phenanthrene, and biphenyl (Lee et al., 2016). The proteomic analysis of the secretomes and enzymes from different microorganisms can be used to begin to understand the full complement of enzymes involved in lignin depolymerization and facilitate increasing the ligninolytic activity of commercially used enzyme cocktails.
Synthetic biology involves engineering new biological systems for the practical purposes of providing them with new and/or improved metabolic abilities. Synthetic biology exploits both traditional metabolic engineering tools (such as plasmid-mediated) and more sophisticated modern genome engineering tools (such as CRISPR/Cas9 and CRAGE) for combinatorial strain development for the industrial production of compounds (Wang et al., 2019; Gauttam et al., 2021). Then, the genome editing era and multi-omics technology led to new synthetic biology, metabolic engineering, and systems biology tools for metabolic pathway analysis and engineering. These tools contributed to the discovery of novel native metabolic pathways to degrade lignin and assimilate its aromatic products (Brown and Chang, 2014). For example, the combinatorial genomic and proteomic analysis of Pandoraea sp. ISTKB grown in the presence of vanillic acid and kraft lignin has revealed a unique aerobic pathway for lignin degradation (Kumar and Kim, 2018). This “-CoA” mediated degradation pathway for phenylacetate and benzoate has been reported in merely 4–5% of sequenced bacterial genomes. The comparative analysis also revealed the presence of ligninolytic enzymes such as peroxidases, oxidases, oxidoreductases, laccases, oxygenases, and etherizes in Pandoraea sp (Kumar and Kim, 2018). The information accumulated through multi-omics approaches can be integrated to rebuild models for novel lignin-degrading pathways in novel microorganisms with ligninolytic potential.
Besides revealing novel ligninolytic microbes and enzymes, several studies have been performed using synthetic biology to produce high-value end products from lignin using engineered microorganisms. Most microorganisms utilize glucose; however, deconstructed lignocellulosics also consist of alternative sugars such as xylose and arabinose that are also economically attractive sources of fermentable and upgradable sugars. For example, an Escherichia coli MS04 strain was engineered to assimilate xylose anaerobically and tolerate high acetate concentrations (Fernández-Sandoval et al., 2012). This strain was used to produce ethanol from corn stover hydrolysate (Parra-Ramírez et al., 2018). Among eukaryotes, oleaginous yeasts such as Rhodosporidium toruloides, Cutaneotrichosporon oleaginosus, and Lipomyces starkeyi can readily metabolize many substrates, including xylose and aromatics, and show excellent tolerance against a wide range of potentially toxic intermediates (Yaegashi et al., 2017; Valdés et al., 2020). Naturally, very few microbes can decompose lignin into vanillin; nevertheless, there are reports for microbial production of vanillin from lignin (Nguyen et al., 2021). For example, the deletion of the vdh gene resulted in the conversion of ferulic acid to vanillin in Pycnoporus cinnabarinus (Tilay et al., 2010), Amycolatopsis sp. (Fleige et al., 2013), and Pseudomonas fluorescens (Di Gioia et al., 2011). Similarly, vdh deletion in R. jostii RHA1 resulted in 96 mg/L vanillin from wheat straw lignocellulose (Sainsbury et al., 2013). On the other hand, Pseudomonas putida KT2440 strain is well known for its tolerance against xenobiotics compounds, aromatic metabolism (e.g., p-coumarate and ferrulate), and the availability of a wide range of advanced genetic factors tools for pathway engineering (Martínez-García and de Lorenzo, 2019; Lee S. et al., 2020). P. putida KT2440 was engineered to enhance the conversion of non-preferred substrates such as p-coumarate and ferrulate in the presence of preferred substrate glucose (Johnson et al., 2017). Through metabolic modeling and genome editing, Pseudomonas not only can grow in harsh environments but can also co-utilize multiple substrates, which suggests the potential of this organism to convert most of the carbon present in lignocellulosic biomass into advanced bioproducts.
The compounds cis, cis-muconic acid (cis, cis-MA) recently drew significant attention because they are an intermediate for adipic acid production, a captive feedstock in the production of nylon fibers and plastics. Recently, Crc regulation of metabolic pathways for the production of muconate in the engineered strain P. putida KT2440-CJ102 was predicted and confirmed using mass spectrometry (MS)-based proteomics and gene editing (Johnson et al., 2017a). It was demonstrated that deletion of the gene encoding Crc enhances metabolism of both 4-HBA and vanillate, leading to enhanced muconate production from p-coumarate or ferulate when either glucose or acetate are supplied as a source of carbon and energy. In similar work, an engineered Sphingobium sp. SYK6 strain produced muconic acid in the absence of glucose from lignin extracts of Japanese cedar and birch (Sonoki et al., 2018). The deletion of muconate cycloisomerase, combined with further engineering, improved muconic acid production in Amycolatopsis sp., Corynebacterium glutamicum, and P. putida (Barton et al., 2018; Becker et al., 2018; Kohlstedt et al., 2018). Notably, NExT-EMA used a tool that channels elementary flux modes (EFMs) into network-embedded thermodynamic (NET) analysis to analyze E. coli and Saccharomyces cerevisiae metabolic networks. Stoichiometric analysis of 32–99% on glucose and/or palmitate can contribute to the maximum theoretical product carbon yield in routes to adipic acid production. This work highlights the importance of pathway and organism choice to maximize the potential of a biobased process, leading the metabolic engineering community toward highly efficient biotechnical production of adipic acid (Averesch et al., 2018).
Developing synthetic microbial constructs for bioconversion of lignocellulose-based products into industrial chemicals often requires extensive pathway engineering involving designing artificial pathways and optimizing rate-limiting enzymes. While integrating multi-omics data and genome editing tools has opened new metabolic engineering avenues to speed up the combinatorial strain development for lignocellulosic biomass conversion using microbes, engineering a host organism to produce new products still has long development times due to the need for a detailed understanding of the host organism’s metabolic pathways. Recently, researchers have begun to apply machine learning and probabilistic modeling algorithms to predict how cells respond to changes in their DNA and biochemistry and to make recommendations for the next engineering cycle without the need for a detailed understanding of the host organism’s metabolism (Radivojević et al., 2020; Lawson et al., 2021). The development and application of machine learning approaches to systems biology and metabolic engineering promises to greatly reduce development times.
Computational tools and methods are becoming essential to optimizing the various processes involved in converting lignocellulosic biomass to valuable fuels and chemicals, from data-driven optimization of deconstruction to molecular-level understanding and rational engineering of enzymes to discovery and building metabolic pathways for synthesis of final products. The tremendous amount of complex chemical and biological data available for analysis and generated by computational biology and chemistry highlights the considerable power and usefulness of computational sciences to develop lignocellulosic biofuels and products. This review highlights and emphasizes the power of synergistic computational and experimental studies aimed at the full-scale optimization of the conversion of lignocellulosic biomass into valuable products. Through valid approximations to the physical laws, modern algorithms, and supercomputers, computational biology and chemistry tools can simulate systems containing hundreds of millions of atoms, required to study the interactions of solvent systems with biomass components and simulate the interactions of solvent systems and engineer enzymes. Recent developments in machine learning and big data analytics have enabled the discovery of new enzyme systems, microbes for biomass conversion, and the engineering of metabolic pathways to produce desired fuels and chemicals. Continued development and growth in applications of computational approaches to optimize pretreatment of lignocellulosic biomass, rational design of enzymes, and next-generation paradigms of predictive approaches in synthetic and system biology are essential for the fundamental science required for development and optimization of viable lignocellulosic biomass conversion processes.
LTMP, HC, and RG wrote the manuscript. All authors read and approved the final manuscript.
This work was part of the DOE Joint BioEnergy Institute (http://www.jbei.org) and was supported by the U.S. Department of Energy, Office of Science, Office of Biological and Environmental Research, through contract DE-AC02-05CH11231 between Lawrence Berkeley National Laboratory and the U.S. Department of Energy. This work was also supported by the US Department of Energy, Office of Energy Efficiency and Renewable Energy, Bioenergy Technologies Office as part of the Feedstock Conversion Interface Consortium (FCIC). Sandia National Laboratories is a multi-mission laboratory managed and operated by National Technology and Engineering Solutions of Sandia, LLC, a wholly owned subsidiary of Honeywell International, Inc., for the U.S. Department of Energy’s National Nuclear Security Administration under contract DE-NA0003525. The United States Government retains and the publisher, by accepting the article for publication, acknowledges that the United States Government retains a non-exclusive, paid-up, irrevocable, worldwide license to publish or reproduce the published form of this manuscript, or allow others to do so, for United States Government purposes.
The authors declare that the research was conducted in the absence of any commercial or financial relationships that could be construed as a potential conflict of interest.
All claims expressed in this article are solely those of the authors and do not necessarily represent those of their affiliated organizations, or those of the publisher, the editors, and the reviewers. Any product that may be evaluated in this article, or claim that may be made by its manufacturer, is not guaranteed or endorsed by the publisher.
ABTS, 2,2′-azino-bis(3-ethylbenzothiazoline-6-sulfonic acid; BLAST, Basic Local Alignment Search Tool; [C2mim][OAc], 1-ethyl-3-methyl-imidazolium acetate; [C4mim]Cl, 1-butyl-3-methylimidazolium chloride; CBD, carbohydrate-binding domain; CBHs, Cellobiohydrolases; CD, catalytic domain; CEs, carbohydrate esterases; COSMO-RS, Conductor like Screening Model for Real Solvents; DFT, Density Functional Theory; DP, degree of polymerization; EFMs, elementary flux modes; EGs, Endoglucanases; GROMOS, GROningen MOlecular Simulation; HMMs, hidden Markov models; HSP, Hansen solubility parameters; IL, inonic liquid; LiP, lignin peroxidase; LMEs, Lignin-modifying enzymes; LODE, long-distance equivariant; LPMO, lytic polysaccharide monooxygenases; MD, molecular dynamics; ML, Machine learning; MnP, manganese peroxidase; NBO, natural bond orbital; NGS, next-generation sequencing; PCFF, Polymer Consistent Force Field; QC, quantum chemical; QM/MM-FEP, free-energy perturbation based quantum mechanics/molecular mechanics; QTAIM, quantum theory of atoms in molecules; SBS, secondary binding site; SIP, stable isotope probing; VP, versatile peroxidase.
Acebes, S., Fernandez-Fueyo, E., Monza, E., Lucas, M. F., Almendral, D., Ruiz-Dueñas, F. J., et al. (2016). Rational Enzyme Engineering through Biophysical and Biochemical Modeling. ACS Catal. 6 (3), 1624–1629. doi:10.1021/acscatal.6b00028
Acebes, S., Ruiz-Dueñas, F. J., Toubes, M., Sáez-Jiménez, V., Pérez-Boada, M., Lucas, M. F., et al. (2017). Mapping the Long-Range Electron Transfer Route in Ligninolytic Peroxidases. J. Phys. Chem. B 121 (16), 3946–3954. doi:10.1021/acs.jpcb.7b00835
Achinivu, E. C., Mohan, M., Choudhary, H., Das, L., Huang, K., Magurudeniya, H. D., et al. (2021). A Predictive Toolset for the Identification of Effective Lignocellulosic Pretreatment Solvents: a Case Study of Solvents Tailored for Lignin Extraction. Green Chem. 23 (18), 7269–7289. doi:10.1039/D1GC01186C
Akiyama, K., Chikayama, E., Yuasa, H., Shimada, Y., Tohge, T., Shinozaki, K., et al. (2008). PRIMe: a Web Site that Assembles Tools for Metabolomics and Transcriptomics. Silico Biol 8 (3-4), 339–345.
Andanson, J.-M., Bordes, E., Devémy, J., Leroux, F., Pádua, A. A. H., and Gomes, M. F. C. (2014). Understanding the Role of Co-solvents in the Dissolution of Cellulose in Ionic Liquids. Green Chem. 16 (5), 2528–2538. doi:10.1039/C3GC42244E
Antunes, D. A., Devaurs, D., and Kavraki, L. E. (2015). Understanding the Challenges of Protein Flexibility in Drug Design. Expert Opin. Drug Discov. 10 (12), 1301–1313. doi:10.1517/17460441.2015.1094458
Averesch, N. J. H., Martínez, V. S., Nielsen, L. K., and Krömer, J. O. (2018). Toward Synthetic Biology Strategies for Adipic Acid Production: An In Silico Tool for Combined Thermodynamics and Stoichiometric Analysis of Metabolic Networks. ACS Synth. Biol. 7 (2), 490–509. doi:10.1021/acssynbio.7b00304
Ayuso-Fernández, I., Ruiz-Dueñas, F. J., and Martínez, A. T. (2018). Evolutionary Convergence in Lignin-Degrading Enzymes. Proc. Natl. Acad. Sci. U.S.A. 115 (25), 6428–6433. doi:10.1073/pnas.1802555115
Balaji, C., Banerjee, T., and Goud, V. V. (2012). COSMO-RS Based Predictions for the Extraction of Lignin from Lignocellulosic Biomass Using Ionic Liquids: Effect of Cation and Anion Combination. J. Solut. Chem. 41 (9), 1610–1630. doi:10.1007/s10953-012-9887-3
Balan, V. (2014). Current Challenges in Commercially Producing Biofuels from Lignocellulosic Biomass. ISRN Biotechnol. 2014, 1–31. doi:10.1155/2014/463074
Barbeyron, T., Brillet-Guéguen, L., Carré, W., Carrière, C., Caron, C., Czjzek, M., et al. (2016). Matching the Diversity of Sulfated Biomolecules: Creation of a Classification Database for Sulfatases Reflecting Their Substrate Specificity. PLOS ONE 11 (10), e0164846. doi:10.1371/journal.pone.0164846
Barton, N., Horbal, L., Starck, S., Kohlstedt, M., Luzhetskyy, A., and Wittmann, C. (201839116). Enabling the Valorization of Guaiacol-Based Lignin: Integrated Chemical and Biochemical Production of Cis,cis-Muconic Acid Using Metabolically Engineered Amycolatopsis Sp ATCC 39116. Metab. Eng. 45, 200–210. doi:10.1016/j.ymben.2017.12.001
Bäumgen, M., Dutschei, T., and Bornscheuer, U. T. (2021). Marine Polysaccharides: Occurrence, Enzymatic Degradation and Utilization. ChemBioChem 22 (13), 2247–2256. doi:10.1002/cbic.202100078
Becker, J., Kuhl, M., Kohlstedt, M., Starck, S., and Wittmann, C. (2018). Metabolic Engineering of Corynebacterium Glutamicum for the Production of Cis, Cis-Muconic Acid from Lignin. Microb. Cell. Fact. 17 (1), 115–129. doi:10.1186/s12934-018-0963-2
Berglund, J., Azhar, S., Lawoko, M., Lindström, M., Vilaplana, F., Wohlert, J., et al. (2019). The Structure of Galactoglucomannan Impacts the Degradation under Alkaline Conditions. Cellulose 26 (3), 2155–2175. doi:10.1007/s10570-018-1737-z
Bernini, C., Pogni, R., Basosi, R., and Sinicropi, A. (2014). Prediction of Hydrogen-Bonding Networks Around Tyrosyl Radical inP. Eryngiiversatile Peroxidase W164Y Variants: a QM/MM MD Study. Mol. Simul. 40 (6), 485–490. doi:10.1080/08927022.2013.822967
Bernini, C., Pogni, R., Basosi, R., and Sinicropi, A. (2012). The Nature of Tryptophan Radicals Involved in the Long-Range Electron Transfer of Lignin Peroxidase and Lignin Peroxidase-like Systems: Insights from Quantum Mechanical/molecular Mechanics Simulations. Proteins 80 (5), 1476–1483. doi:10.1002/prot.24046
Bharadwaj, V. S., Knott, B. C., Ståhlberg, J., Beckham, G. T., and Crowley, M. F. (2020). The Hydrolysis Mechanism of a GH45 Cellulase and its Potential Relation to Lytic Transglycosylase and Expansin Function. J. Biol. Chem. 295 (14), 4477–4487. doi:10.1074/jbc.RA119.011406
Bharadwaj, V. S., Schutt, T. C., Ashurst, T. C., and Maupin, C. M. (2015). Elucidating the Conformational Energetics of Glucose and Cellobiose in Ionic Liquids. Phys. Chem. Chem. Phys. 17 (16), 10668–10678. doi:10.1039/c5cp00118h
Bhardwaj, N., Kumar, B., and Verma, P. (2019). A Detailed Overview of Xylanases: an Emerging Biomolecule for Current and Future Prospective. Bioresour. Bioprocess. 6 (1), 40. doi:10.1186/s40643-019-0276-2
Borisova, A. S., Eneyskaya, E. V., Bobrov, K. S., Jana, S., Logachev, A., Polev, D. E., et al. (2015). Sequencing, Biochemical Characterization, Crystal Structure and Molecular Dynamics of Cellobiohydrolase Cel7A from Geotrichum Candidum 3C. Febs J. 282 (23), 4515–4537. doi:10.1111/febs.13509
Borrelli, K. W., Vitalis, A., Alcantara, R., and Guallar, V. (2005). PELE: Protein Energy Landscape Exploration. A Novel Monte Carlo Based Technique. J. Chem. Theory Comput. 1 (6), 1304–1311. doi:10.1021/ct0501811
Bowling, A. J., Amano, Y., Lindstrom, R., and Brown, Jr, R. M. (2001). Rotation of Cellulose Ribbons During Degradation with Fungal Cellulase. Cellulose 8 (1), 91–97. doi:10.1023/A:1016660621440
Briganti, L., Capetti, C., Pellegrini, V. O. A., Ghio, S., Campos, E., Nascimento, A. S., et al. (2021). Structural and molecular dynamics investigations of ligand stabilization via secondary binding site interactions in Paenibacillus xylanivorans GH11 xylanase. Comput. Struct. Biotechnol. J. 19, 1557–1566. doi:10.1016/j.csbj.2021.03.002
Brown, M. E., and Chang, M. C. (2014). Exploring bacterial lignin degradation. Curr. Opin. Chem. Biol. 19, 1–7. doi:10.1016/j.cbpa.2013.11.015
Brunk, E., and Rothlisberger, U. (2015). Mixed Quantum Mechanical/Molecular Mechanical Molecular Dynamics Simulations of Biological Systems in Ground and Electronically Excited States. Chem. Rev. 115 (12), 6217–6263. doi:10.1021/cr500628b
Busse‐Wicher, M., Gomes, T. C. F., Tryfona, T., Nikolovski, N., Stott, K., Grantham, N. J., et al. (2014). The pattern of xylan acetylation suggests xylan may interact with cellulose microfibrils as a twofold helical screw in the secondary plant cell wall of Arabidopsis thaliana. Plant J. 79 (3), 492–506. doi:10.1111/tpj.12575
Casas, A., Omar, S., Palomar, J., Oliet, M., Alonso, M. V., and Rodriguez, F. (2013). Relation between differential solubility of cellulose and lignin in ionic liquids and activity coefficients. RSC Adv. 3 (10), 3453–3460. doi:10.1039/C2RA22800A
Casas, A., Palomar, J., Alonso, M. V., Oliet, M., Omar, S., and Rodriguez, F. (2012). Comparison of lignin and cellulose solubilities in ionic liquids by COSMO-RS analysis and experimental validation. Industrial Crops Prod. 37 (1), 155–163. doi:10.1016/j.indcrop.2011.11.032
Castilla-Archilla, J., O’Flaherty, V., and Lens, P. N. L. (2019). “Biorefineries: Industrial Innovation and Tendencies,” in Biorefinery: Integrated Sustainable Processes for Biomass Conversion to Biomaterials, Biofuels, and Fertilizers. Editors J.-R. Bastidas-Oyanedel, and J. E. Schmidt (Cham: Springer International Publishing), 3–35. doi:10.1007/978-3-030-10961-5_1
Castro, L., Crawford, L. E., Mutengwa, A., Götze, J. P., and Bühl, M. (2016). Insights into structure and redox potential of lignin peroxidase from QM/MM calculations. Org. Biomol. Chem. 14 (8), 2385–2389. doi:10.1039/c6ob00037a
Cerqueira, N. M. F. S. A., Bras, N. F., Fernandes, P. A., and Ramos, M. J. (2009). MADAMM: a multistaged docking with an automated molecular modeling protocol. Proteins 74 (1), 192–206. doi:10.1002/prot.22146
Chen, M., Zeng, G., Tan, Z., Jiang, M., Li, H., Liu, L., et al. (2011). Understanding lignin-degrading reactions of ligninolytic enzymes: binding affinity and interactional profile. PLoS One 6 (9), e25647. doi:10.1371/journal.pone.0025647
Cheng, F., Ouyang, T., Sun, J., Jiang, T., and Luo, J. (2018). Using solubility parameter analysis to understand delignification of poplar and rice straw with catalyzed organosolv fractionation processes. BioRes 14 (1), 486–499. doi:10.15376/biores.14.1.486-499
Cho, H. M., Gross, A. S., and Chu, J.-W. (2011). Dissecting force interactions in cellulose deconstruction reveals the required solvent versatility for overcoming biomass recalcitrance. J. Am. Chem. Soc. 133 (35), 14033–14041. doi:10.1021/ja2046155
Chu, Y., and He, X. (2019). MoDoop: An Automated Computational Approach for COSMO-RS Prediction of Biopolymer Solubilities in Ionic Liquids. ACS Omega 4 (1), 2337–2343. doi:10.1021/acsomega.8b03255
Ciesielski, P. N., Pecha, M. B., Lattanzi, A. M., Bharadwaj, V. S., Crowley, M. F., Bu, L., et al. (2020). Advances in Multiscale Modeling of Lignocellulosic Biomass. ACS Sustain. Chem. Eng. 8 (9), 3512–3531. doi:10.1021/acssuschemeng.9b07415
Clish, C. B. (2015). Metabolomics: an emerging but powerful tool for precision medicine. Cold Spring Harb. Mol. Case Stud. 1 (1), a000588. doi:10.1101/mcs.a000588
Cohen, A. J., Mori-Sánchez, P., and Yang, W. (2012). Challenges for Density Functional Theory. Chem. Rev. 112 (1), 289–320. doi:10.1021/cr200107z
Cuyvers, S., Dornez, E., Rezaei, M. N., Pollet, A., Delcour, J. A., and Courtin, C. M. (2011). Secondary substrate binding strongly affects activity and binding affinity ofBacillus subtilisandAspergillus nigerGH11 xylanases. Febs J. 278 (7), 1098–1111. doi:10.1111/j.1742-4658.2011.08023.x
Danne, R., Poojari, C., Martinez-Seara, H., Rissanen, S., Lolicato, F., Róg, T., et al. (2017). doGlycans-Tools for Preparing Carbohydrate Structures for Atomistic Simulations of Glycoproteins, Glycolipids, and Carbohydrate Polymers for GROMACS. J. Chem. Inf. Model. 57 (10), 2401–2406. doi:10.1021/acs.jcim.7b00237
Davies, G. J., Gloster, T. M., and Henrissat, B. (2005). Recent structural insights into the expanding world of carbohydrate-active enzymes. Curr. Opin. Struct. Biol. 15 (6), 637–645. doi:10.1016/j.sbi.2005.10.008
Decker, C. H., Visser, J., and Schreier, P. (2001). β-Glucosidase multiplicity from Aspergillus tubingensis CBS 643.92: purification and characterization of four β-glucosidases and their differentiation with respect to substrate specificity, glucose inhibition and acid tolerance. Appl. Microbiol. Biotechnol. 55 (2), 157–163. doi:10.1007/s002530000462
Dellon, L. D., Yanez, A. J., Li, W., Mabon, R., and Broadbelt, L. J. (2017). Computational Generation of Lignin Libraries from Diverse Biomass Sources. Energy fuels. 31 (8), 8263–8274. doi:10.1021/acs.energyfuels.7b01150
Derecskei, B., and Derecskei-Kovacs, A. (2006). Molecular dynamic studies of the compatibility of some cellulose derivatives with selected ionic liquids. Mol. Simul. 32 (2), 109–115. doi:10.1080/08927020600669627
Di Gioia, D., Luziatelli, F., Negroni, A., Ficca, A. G., Fava, F., and Ruzzi, M. (2011). Metabolic engineering of Pseudomonas fluorescens for the production of vanillin from ferulic acid. J. Biotechnol. 156 (4), 309–316. doi:10.1016/j.jbiotec.2011.08.014
Ding, Z.-D., Chi, Z., Gu, W.-X., Gu, S.-M., Liu, J.-H., and Wang, H.-J. (2012). Theoretical and experimental investigation on dissolution and regeneration of cellulose in ionic liquid. Carbohydr. Polym. 89 (1), 7–16. doi:10.1016/j.carbpol.2012.01.080
Dupree, E. J., Jayathirtha, M., Yorkey, H., Mihasan, M., Petre, B. A., and Darie, C. C. (2020). A Critical Review of Bottom-Up Proteomics: The Good, the Bad, and the Future of this Field. Proteomes 8 (3), 14. doi:10.3390/proteomes8030014
Farhat, W., Venditti, R. A., Hubbe, M., Taha, M., Becquart, F., and Ayoub, A. (2017). A Review of Water-Resistant Hemicellulose-Based Materials: Processing and Applications. ChemSusChem 10 (2), 305–323. doi:10.1002/cssc.201601047
Felczak, K., Chen, L., Wilson, D., Williams, J., Vince, R., Petrelli, R., et al. (2011). Cofactor-type inhibitors of inosine monophosphate dehydrogenase via modular approach: targeting the pyrophosphate binding sub-domain. Bioorg. Med. Chem. 19 (5), 1594–1605. doi:10.1016/j.bmc.2011.01.042
Fernández-Fueyo, E., Acebes, S., Ruiz-Dueñas, F. J., Martínez, M. J., Romero, A., Medrano, F. J., et al. (2014). Structural implications of the C-terminal tail in the catalytic and stability properties of manganese peroxidases from ligninolytic fungi. Acta Cryst. D. Biol. Crystallogr. 70 (Pt 12), 3253–3265. doi:10.1107/s1399004714022755
Fernandez-Fueyo, E., Ruiz-Dueñas, F. J., Ferreira, P., Floudas, D., Hibbett, D. S., Canessa, P., et al. (2012). Comparative genomics of Ceriporiopsis subvermispora and Phanerochaete chrysosporium provide insight into selective ligninolysis. Proc. Natl. Acad. Sci. U.S.A. 109 (14), 5458–5463. doi:10.1073/pnas.1119912109
Fernández-Sandoval, M. T., Huerta-Beristain, G., Trujillo-Martinez, B., Bustos, P., González, V., Bolivar, F., et al. (2012). Laboratory metabolic evolution improves acetate tolerance and growth on acetate of ethanologenic Escherichia coli under non-aerated conditions in glucose-mineral medium. Appl. Microbiol. Biotechnol. 96 (5), 1291–1300. doi:10.1007/s00253-012-4177-y
Fleige, C., Hansen, G., Kroll, J., Steinbüchel, A., and microbiology, e. (2013). Investigation of the Amycolatopsis sp. strain ATCC 39116 vanillin dehydrogenase and its impact on the biotechnical production of vanillin. Appl. Environ. Microbiol. 79 (1), 81–90. doi:10.1128/AEM.02358-12
Frenkel, D., and Smit, B. (2002). “Introduction,” in Understanding Molecular Simulation. Editors D. Frenkel, and B. Smit. Second Edition (San Diego: Academic Press), 1–6. doi:10.1016/b978-012267351-1/50003-1
Friesner, R. A., Banks, J. L., Murphy, R. B., Halgren, T. A., Klicic, J. J., Mainz, D. T., et al. (2004). Glide: a new approach for rapid, accurate docking and scoring. 1. Method and assessment of docking accuracy. J. Med. Chem. 47 (7), 1739–1749. doi:10.1021/jm0306430
Gauttam, R., Mukhopadhyay, A., Simmons, B. A., and Singer, S. W. (2021). Development of dual‐inducible duet‐expression vectors for tunable gene expression control and CRISPR interference‐based gene repression in Pseudomonas putida KT2440. Microb. Biotechnol. 14 (6), 2659–2678. doi:10.1111/1751-7915.13832
Gibson, L. J. (2012). The hierarchical structure and mechanics of plant materials. J. R. Soc. Interface. 9 (76), 2749–2766. doi:10.1098/rsif.2012.0341
Gomes, T. C. F., and Skaf, M. S. (2012). Cellulose-builder: a toolkit for building crystalline structures of cellulose. J. Comput. Chem. 33 (14), 1338–1346. doi:10.1002/jcc.22959
Götze, J. P., and Bühl, M. (2016). Laccase Redox Potentials: pH Dependence and Mutants, a QM/MM Study. J. Phys. Chem. B 120 (35), 9265–9276. doi:10.1021/acs.jpcb.6b04978
Grisafi, A., and Ceriotti, M. (2019). Incorporating long-range physics in atomic-scale machine learning. J. Chem. Phys. 151 (20), 204105. doi:10.1063/1.5128375
Gross, A. S., Bell, A. T., and Chu, J.-W. (2012). Entropy of cellulose dissolution in water and in the ionic liquid 1-butyl-3-methylimidazolim chloride. Phys. Chem. Chem. Phys. 14 (23), 8425–8430. doi:10.1039/c2cp40417f
Gross, A. S., Bell, A. T., and Chu, J.-W. (2011). Thermodynamics of cellulose solvation in water and the ionic liquid 1-butyl-3-methylimidazolim chloride. J. Phys. Chem. B 115 (46), 13433–13440. doi:10.1021/jp202415v
Guo, X., An, Y., Chai, C., Sang, J., Jiang, L., Lu, F., et al. (2020). Construction of the R17L mutant of MtC1LPMO for improved lignocellulosic biomass conversion by rational point mutation and investigation of the mechanism by molecular dynamics simulations. Bioresour. Technol. 317, 124024. doi:10.1016/j.biortech.2020.124024
Gupta, K. M., Hu, Z., and Jiang, J. (2011). Mechanistic understanding of interactions between cellulose and ionic liquids: A molecular simulation study. Polymer 52 (25), 5904–5911. doi:10.1016/j.polymer.2011.10.035
Haghighi Mood, S., Hossein Golfeshan, A., Tabatabaei, M., Salehi Jouzani, G., Najafi, G. H., Gholami, M., et al. (2013). Lignocellulosic biomass to bioethanol, a comprehensive review with a focus on pretreatment. Renew. Sustain. Energy Rev. 27 (C), 77–93. doi:10.1016/j.rser.2013.06.033
Hallac, B. B., and Ragauskas, A. J. (2011). Analyzing cellulose degree of polymerization and its relevancy to cellulosic ethanol. Biofuels, Bioprod. Bioref. 5 (2), 215–225. doi:10.1002/bbb.269
Hamid, M., and Khalil-ur-Rehman, R. (2009). Potential applications of peroxidases. Food Chem. 115 (4), 1177–1186. doi:10.1016/j.foodchem.2009.02.035
Hanley, S. J., Revol, J.-F., Godbout, L., and Gray, D. G. (1997). Atomic force microscopy and transmission electron microscopy of cellulose from Micrasterias denticulata; evidence for a chiral helical microfibril twist. Cellulose 4 (3), 209–220. doi:10.1023/A:1018483722417
Hansen, C. M. (2007). “Hansen Solubility Parameters,” in Hansen Solubility Parameters: A User's Handbook. 2nd Edn (Boca Raton, FL: CRC Press). doi:10.1201/9781420006834
Hernández-de-Diego, R., Tarazona, S., Martínez-Mira, C., Balzano-Nogueira, L., Furió-Tarí, P., Pappas, G. J., et al. (2018). PaintOmics 3: a web resource for the pathway analysis and visualization of multi-omics data. Nucleic Acids Res. 46 (W1), W503–w509. doi:10.1093/nar/gky466
Higuchi, T. (1997). “Structure and Functions of Wood,” in Biochemistry and Molecular Biology of Wood. Editor T. Higuchi (Berlin, Heidelberg: Springer Berlin Heidelberg), 1–42. doi:10.1007/978-3-642-60469-0_1
Hong, G., Ivnitski, D. M., Johnson, G. R., Atanassov, P., and Pachter, R. (2011). Design parameters for tuning the type 1 Cu multicopper oxidase redox potential: insight from a combination of first principles and empirical molecular dynamics simulations. J. Am. Chem. Soc. 133 (13), 4802–4809. doi:10.1021/ja105586q
Hu, N., Xu, D., Fang, J., Li, H., Mo, J., Zhou, M., et al. (2020). Intracellular recording of cardiomyocyte action potentials by nanobranched microelectrode array. Biosens. Bioelectron. 169, 112588. doi:10.1016/j.bios.2020.112588
Huang, K., Mohan, M., George, A., Simmons, B. A., Xu, Y., and Gladden, J. M. (2021). Integration of acetic acid catalysis with one-pot protic ionic liquid configuration to achieve high-efficient biorefinery of poplar biomass. Green Chem. 23 (16), 6036–6049. doi:10.1039/D1GC01727F
Huffman, F. G. (2003). “URONIC ACIDS,” in Encyclopedia of Food Sciences and Nutrition. Editor B. Caballero. Second Edition (Oxford: Academic Press), 5890–5896. doi:10.1016/b0-12-227055-x/01221-9
Huo, F., Liu, Z., and Wang, W. (2013). Cosolvent or antisolvent? A molecular view of the interface between ionic liquids and cellulose upon addition of another molecular solvent. J. Phys. Chem. B 117 (39), 11780–11792. doi:10.1021/jp407480b
Iglesias-Fernández, J., Hancock, S. M., Lee, S. S., Khan, M., Kirkpatrick, J., Oldham, N. J., et al. (2017). A front-face 'SNi synthase' engineered from a retaining 'double-SN2' hydrolase. Nat. Chem. Biol. 13 (8), 874–881. doi:10.1038/nchembio.2394
Iqbal, J., Muhammad, N., Rahim, A., Khan, A. S., Ullah, Z., Gonfa, G., et al. (2019). COSMO-RS predictions, hydrogen bond basicity values and experimental evaluation of amino acid-based ionic liquids for lignocellulosic biomass dissolution. J. Mol. Liq. 273, 215–221. doi:10.1016/j.molliq.2018.10.044
Janesko, B. G. (2011). Modeling interactions between lignocellulose and ionic liquids using DFT-D. Phys. Chem. Chem. Phys. 13 (23), 11393–11401. doi:10.1039/C1CP20072K
Jarin, Z., and Pfaendtner, J. (2014). Ionic Liquids Can Selectively Change the Conformational Free-Energy Landscape of Sugar Rings. J. Chem. Theory Comput. 10 (2), 507–510. doi:10.1021/ct4010036
Ji, H., and Lv, P. (2020). Mechanistic insights into the lignin dissolution behaviors of a recyclable acid hydrotrope, deep eutectic solvent (DES), and ionic liquid (IL). Green Chem. 22 (4), 1378–1387. doi:10.1039/C9GC02760B
Johnson, C. W., Abraham, P. E., Linger, J. G., Khanna, P., Hettich, R. L., and Beckham, G. T. (2017a). Eliminating a global regulator of carbon catabolite repression enhances the conversion of aromatic lignin monomers to muconate in Pseudomonas putida KT2440. Metab. Eng. Commun. 5, 19–25. doi:10.1016/j.meteno.2017.05.002
Kahlen, J., Masuch, K., and Leonhard, K. (2010). Modelling cellulose solubilities in ionic liquids using COSMO-RS. Green Chem. 12 (12), 2172–2181. doi:10.1039/C0GC00200C
Kameda, Y., Maeda, S., Amo, Y., Usuki, T., Ikeda, K., and Otomo, T. (2018). Neutron Diffraction Study on the Structure of Hydrated Li+ in Dilute Aqueous Solutions. J. Phys. Chem. B 122 (5), 1695–1701. doi:10.1021/acs.jpcb.7b12218
Kearsley, S. K., Underwood, D. J., Sheridan, R. P., and Miller, M. D. (1994). Flexibases: A way to enhance the use of molecular docking methods. J. Computer-Aided Mol. Des. 8 (5), 565–582. doi:10.1007/BF00123666
Khan, S., Farooq, U., and Kurnikova, M. (2016). Exploring Protein Stability by Comparative Molecular Dynamics Simulations of Homologous Hyperthermophilic, Mesophilic, and Psychrophilic Proteins. J. Chem. Inf. Model. 56 (11), 2129–2139. doi:10.1021/acs.jcim.6b00305
Khoury, M. J., Feero, W. G., Reyes, M., Citrin, T., Freedman, A., Leonard, D., et al. (2009). The genomic applications in practice and prevention network. Genet. Med. 11 (7), 488–494. doi:10.1097/GIM.0b013e3181a551cc
Kirk, T. K., Tien, M., Kersten, P. J., Mozuch, M. D., and Kalyanaraman, B. (1986). Ligninase of Phanerochaete chrysosporium. Mechanism of its degradation of the non-phenolic arylglycerol β-aryl ether substructure of lignin. Biochem. J. 236 (1), 279–287. doi:10.1042/bj2360279
Kitano, H. (2002). Systems biology: a brief overview. Science 295 (5560), 1662–1664. doi:10.1126/science.1069492
Knott, B. C., Crowley, M. F., Himmel, M. E., Ståhlberg, J., and Beckham, G. T. (2014). Carbohydrate-protein interactions that drive processive polysaccharide translocation in enzymes revealed from a computational study of cellobiohydrolase processivity. J. Am. Chem. Soc. 136 (24), 8810–8819. doi:10.1021/ja504074g
Kohler, A. C., Simmons, B. A., and Sale, K. L. (2018). Structure-based Engineering of a Plant-Fungal Hybrid Peroxidase for Enhanced Temperature and pH Tolerance. Cell. Chem. Biol. 25 (8), 974–983. e973. doi:10.1016/j.chembiol.2018.04.014
Kohlstedt, M., Starck, S., Barton, N., Stolzenberger, J., Selzer, M., Mehlmann, K., et al. (2018). From lignin to nylon: cascaded chemical and biochemical conversion using metabolically engineered Pseudomonas putida. Metab. Eng. 47, 279–293. doi:10.1016/j.ymben.2018.03.003
Krämer, A., Green, J., Pollard, J., and Tugendreich, S. (2014). Causal analysis approaches in Ingenuity Pathway Analysis. Bioinformatics 30 (4), 523–530. doi:10.1093/bioinformatics/btt703
Kumar, A., and Chandra, R. (2020). Ligninolytic enzymes and its mechanisms for degradation of lignocellulosic waste in environment. Heliyon 6 (2), e03170. doi:10.1016/j.heliyon.2020.e03170
Kumar, P., and Kim, B. S. (2018). Valorization of polyhydroxyalkanoates production process by co-synthesis of value-added products. Bioresour. Technol. 269, 544–556. doi:10.1016/j.biortech.2018.08.120
Kuo, T.-C., Tian, T.-F., and Tseng, Y. J. (2013). 3Omics: a web-based systems biology tool for analysis, integration and visualization of human transcriptomic, proteomic and metabolomic data. BMC Syst. Biol. 7 (1), 64. doi:10.1186/1752-0509-7-64
Lawson, C. E., Martí, J. M., Radivojevic, T., Jonnalagadda, S. V. R., Gentz, R., Hillson, N. J., et al. (2021). Machine learning for metabolic engineering: A review. Metab. Eng. 63, 34–60. doi:10.1016/j.ymben.2020.10.005
Lê Cao, K.-A., González, I., and Déjean, S. (2009). integrOmics: an R package to unravel relationships between two omics datasets. Bioinformatics 25 (21), 2855–2856. doi:10.1093/bioinformatics/btp515
Lee, N., Hwang, S., Kim, J., Cho, S., Palsson, B., Cho, B.-K., et al. (2020a). Mini review: genome mining approaches for the identification of secondary metabolite biosynthetic gene clusters in Streptomyces. Comput. Struct. Biotechnol. J. 18, 1548–1556. doi:10.1016/j.csbj.2020.06.024
Lee, S., Sohn, J.-H., Bae, J.-H., Kim, S. C., Sung, B. H. J. B., and Engineering, B. (2020b). Current Status of Pseudomonas Putida Engineering for Lignin Valorization, 1–10.
Lee, S. Y., Sekhon, S. S., Ban, Y.-H., Ahn, J.-Y., Ko, J. H., Lee, L., et al. (2016). Proteomic analysis of polycyclic aromatic hydrocarbons (PAHs) degradation and detoxification in Sphingobium chungbukense DJ77. J. Microbiol. Biotechnol. 26 (11), 1943–1950. doi:10.4014/jmb.1606.06005
Levitt, M. (1982). Protein conformation, dynamics, and folding by computer simulation. Annu. Rev. Biophys. Bioeng. 11, 251–271. doi:10.1146/annurev.bb.11.060182.001343
Levitt, M., and Warshel, A. (1975). Computer simulation of protein folding. Nature 253 (5494), 694–698. doi:10.1038/253694a0
Li, J., Du, L., and Wang, L. (2010). Glycosidic-bond hydrolysis mechanism catalyzed by cellulase Cel7A from Trichoderma reesei: a comprehensive theoretical study by performing MD, QM, and QM/MM calculations. J. Phys. Chem. B 114 (46), 15261–15268. doi:10.1021/jp1064177
Li, J., Farrokhnia, M., Rulíšek, L., and Ryde, U. (2015a). Catalytic Cycle of Multicopper Oxidases Studied by Combined Quantum- and Molecular-Mechanical Free-Energy Perturbation Methods. J. Phys. Chem. B 119 (26), 8268–8284. doi:10.1021/acs.jpcb.5b02864
Li, Q., Sun, B., Xiong, K., Teng, C., Xu, Y., Li, L., et al. (2017). Improving special hydrolysis characterization into Talaromyces thermophilus F1208 xylanase by engineering of N-terminal extension and site-directed mutagenesis in C-terminal. Int. J. Biol. Macromol. 96, 451–458. doi:10.1016/10.1016/j.ijbiomac.2016.12.050
Li, Y., Liu, X., Zhang, S., Yao, Y., Yao, X., Xu, J., et al. (2015b). Dissolving process of a cellulose bunch in ionic liquids: a molecular dynamics study. Phys. Chem. Chem. Phys. 17 (27), 17894–17905. doi:10.1039/C5CP02009C
Libbrecht, M. W., and Noble, W. S. (2015). Machine learning applications in genetics and genomics. Nat. Rev. Genet. 16, 321–332. doi:10.1038/nrg3920
Liu, B., Kognole, A. A., Wu, M., Westereng, B., Crowley, M. F., Kim, S., et al. (2018). Structural and molecular dynamics studies of a C1‐oxidizing lytic polysaccharide monooxygenase from Heterobasidion irregulare reveal amino acids important for substrate recognition. Febs J. 285 (12), 2225–2242. doi:10.1111/febs.14472
Liu, H., Cheng, G., Kent, M., Stavila, V., Simmons, B. A., Sale, K. L., et al. (2012). Simulations Reveal Conformational Changes of Methylhydroxyl Groups during Dissolution of Cellulose Iβ in Ionic Liquid 1-Ethyl-3-methylimidazolium Acetate. J. Phys. Chem. B 116 (28), 8131–8138. doi:10.1021/jp301673h
Liu, H., Sale, K. L., Holmes, B. M., Simmons, B. A., and Singh, S. (2010a). Understanding the Interactions of Cellulose with Ionic Liquids: A Molecular Dynamics Study. J. Phys. Chem. B 114 (12), 4293–4301. doi:10.1021/jp9117437
Liu, J., Wang, X., and Xu, D. (2010b). QM/MM study on the catalytic mechanism of cellulose hydrolysis catalyzed by cellulase Cel5A from Acidothermus cellulolyticus. J. Phys. Chem. B 114 (3), 1462–1470. doi:10.1021/jp909177e
Liu, Y.-R., Thomsen, K., Nie, Y., Zhang, S.-J., and Meyer, A. S. (2016). Predictive screening of ionic liquids for dissolving cellulose and experimental verification. Green Chem. 18 (23), 6246–6254. doi:10.1039/C6GC01827K
Ludwiczek, M. L., Heller, M., Kantner, T., and McIntosh, L. P. (2007). A secondary xylan-binding site enhances the catalytic activity of a single-domain family 11 glycoside hydrolase. J. Mol. Biol. 373 (2), 337–354. doi:10.1016/j.jmb.2007.07.057
Martí, S., Roca, M., Andrés, J., Moliner, V., Silla, E., Tuñón, I., et al. (2004). Theoretical insights in enzyme catalysis. Chem. Soc. Rev. 33 (2), 98–107. doi:10.1039/B301875J
Martínez-Abad, A., Berglund, J., Toriz, G., Gatenholm, P., Henriksson, G., Lindström, M., et al. (2017). Regular Motifs in Xylan Modulate Molecular Flexibility and Interactions with Cellulose Surfaces. Plant Physiol. 175 (4), 1579–1592. doi:10.1104/pp.17.01184
Martínez-García, E., and de Lorenzo, V. (2019). Pseudomonas putida in the quest of programmable chemistry. Curr. Opin. Biotechnol. 59, 111–121. doi:10.1016/j.copbio.2019.03.012
Matthews, J. F., Beckham, G. T., Bergenstråhle-Wohlert, M., Brady, J. W., Himmel, M. E., and Crowley, M. F. (2012). Comparison of Cellulose Iβ Simulations with Three Carbohydrate Force Fields. J. Chem. Theory Comput. 8 (2), 735–748. doi:10.1021/ct2007692
Matthews, J. F., Bergenstråhle, M., Beckham, G. T., Himmel, M. E., Nimlos, M. R., Brady, J. W., et al. (2011). High-Temperature Behavior of Cellulose I. J. Phys. Chem. B 115 (10), 2155–2166. doi:10.1021/jp1106839
Mazeau, K., and Heux, L. (2003). Molecular Dynamics Simulations of Bulk Native Crystalline and Amorphous Structures of Cellulose. J. Phys. Chem. B 107 (10), 2394–2403. doi:10.1021/jp0219395
Medve, J., Karlsson, J., Lee, D., and Tjerneld, F. (1998). Hydrolysis of microcrystalline cellulose by cellobiohydrolase I and endoglucanase II fromTrichoderma reesei: Adsorption, sugar production pattern, and synergism of the enzymes. Biotechnol. Bioeng. 59 (5), 621–634. doi:10.1002/(sici)1097-0290(19980905)59:5<621::aid-bit13>3.0.co;2-c
Miki, K., Renganathan, V., and Gold, M. H. (1986). Mechanism of .beta.-aryl ether dimeric lignin model compound oxidation by lignin peroxidase by Phanerochaete chrysosporium. Biochemistry 25 (17), 4790–4796. doi:10.1021/bi00365a011
Miki, Y., Pogni, R., Acebes, S., Lucas, F., Fernández-Fueyo, E., Baratto, M. C., et al. (2013). Formation of a tyrosine adduct involved in lignin degradation by Trametopsis cervina lignin peroxidase: a novel peroxidase activation mechanism. Biochem. J. 452 (3), 575–584. doi:10.1042/bj20130251
Min, S., Lee, B., and Yoon, S. (2016). Deep learning in bioinformatics. Brief. Bioinform 18, 851–869. doi:10.1093/bib/bbw068
Moal, I. H., and Bates, P. A. (2012). Kinetic rate constant prediction supports the conformational selection mechanism of protein binding. PLoS Comput. Biol. 8 (1), e1002351. doi:10.1371/journal.pcbi.1002351
Mohan, M., Choudhary, H., George, A., Simmons, B. A., Sale, K., and Gladden, J. M. (2021). Towards understanding of delignification of grassy and woody biomass in cholinium-based ionic liquids. Green Chem. 23 (16), 6020–6035. doi:10.1039/D1GC01622A
Mohan, M., Huang, K., Pidatala, V. R., Simmons, B. A., Singh, S., Sale, K. L., et al. (2022). Prediction of solubility parameters of lignin and ionic liquids using multi-resolution simulation approaches. Green Chem. 24 (3), 1165–1176. doi:10.1039/D1GC03798F
Momeni, M. H., Payne, C. M., Hansson, H., Mikkelsen, N. E., Svedberg, J., Engström, Å., et al. (2013). Structural, Biochemical, and Computational Characterization of the Glycoside Hydrolase Family 7 Cellobiohydrolase of the Tree-killing Fungus Heterobasidion irregulare*. J. Biol. Chem. 288 (8), 5861–5872. doi:10.1074/jbc.M112.440891
Mostofian, B., Cheng, X., and Smith, J. C. (2014a). Replica-exchange molecular dynamics simulations of cellulose solvated in water and in the ionic liquid 1-butyl-3-methylimidazolium chloride. J. Phys. Chem. B 118 (38), 11037–11049. doi:10.1021/jp502889c
Mostofian, B., Smith, J. C., and Cheng, X. (2014b). Simulation of a cellulose fiber in ionic liquid suggests a synergistic approach to dissolution. Cellulose 21 (2), 983–997. doi:10.1007/s10570-013-0018-0
Mostofian, B., Smith, J. C., and Cheng, X. (2011). The solvation structures of cellulose microfibrils in ionic liquids. Interdiscip. Sci. Comput. Life Sci. 3 (4), 308–320. doi:10.1007/s12539-011-0111-8
Mulholland, A. J. (2005). Modelling enzyme reaction mechanisms, specificity and catalysis. Drug Discov. Today 10 (20), 1393–1402. doi:10.1016/s1359-6446(05)03611-1
Ngenyoung, A., Muhammad, A., Rattanarojpong, T., Sutthibutpong, T., and Khunrae, P. (2021). Effect of N-terminal modification on the mode of action between the Xyn11A and Xylotetraose. Int. J. Biol. Macromol. 170, 240–247. doi:10.1016/10.1016/j.ijbiomac.2020.12.154
Nguyen, L. T., Phan, D.-P., Sarwar, A., Tran, M. H., Lee, O. K., Lee, E. Y., et al. (2021). Valorization of industrial lignin to value-added chemicals by chemical depolymerization and biological conversion. Industrial Crops Prod. 161, 113219. doi:10.1016/j.indcrop.2020.113219
Novoselov, N. P., Sashina, E. S., Petrenko, V. E., and Zaborsky, M. (2007). Study of dissolution of cellulose in ionic liquids by computer modeling. Fibre Chem. 39 (2), 153–158. doi:10.1007/s10692-007-0030-y
Nys, K., Furtmüller, P. G., Obinger, C., Van Doorslaer, S., and Pfanzagl, V. (2021). On the Track of Long-Range Electron Transfer in B-Type Dye-Decolorizing Peroxidases: Identification of a Tyrosyl Radical by Computational Prediction and Electron Paramagnetic Resonance Spectroscopy. Biochemistry 60 (15), 1226–1241. doi:10.1021/acs.biochem.1c00129
Ogunmolu, F. E., Jagadeesha, N. B. K., Kumar, R., Kumar, P., Gupta, D., and Yazdani, S. S. (2017). Comparative insights into the saccharification potentials of a relatively unexplored but robust Penicillium funiculosum glycoside hydrolase 7 cellobiohydrolase. Biotechnol. Biofuels 10 (1), 71. doi:10.1186/s13068-017-0752-x
Orella, M. J., Gani, T. Z. H., Vermaas, J. V., Stone, M. L., Anderson, E. M., Beckham, G. T., et al. (2019). Lignin-KMC: A Toolkit for Simulating Lignin Biosynthesis. ACS Sustain. Chem. Eng. 7 (22), 18313–18322. doi:10.1021/acssuschemeng.9b03534
Pang, Z., Chong, J., Zhou, G., de Lima Morais, D. A., Chang, L., Barrette, M., et al. (2021). MetaboAnalyst 5.0: narrowing the gap between raw spectra and functional insights. Nucleic Acids Res. 49 (W1), W388–W396. doi:10.1093/nar/gkab382
Parra-Ramírez, D., Martinez, A., and Cardona, C. A. (2018). Technical and economic potential evaluation of the strain Escherichia coli MS04 in the ethanol production from glucose and xylose. Biochem. Eng. J. 140, 123–129. doi:10.1016/j.bej.2018.09.015
Parthasarathi, R., Balamurugan, K., Shi, J., Subramanian, V., Simmons, B. A., and Singh, S. (2015). Theoretical Insights into the Role of Water in the Dissolution of Cellulose Using IL/Water Mixed Solvent Systems. J. Phys. Chem. B 119 (45), 14339–14349. doi:10.1021/acs.jpcb.5b02680
Payne, C. M., Resch, M. G., Chen, L., Crowley, M. F., Himmel, M. E., Taylor, L. E., et al. (2013). Glycosylated linkers in multimodular lignocellulose-degrading enzymes dynamically bind to cellulose. Proc. Natl. Acad. Sci. U.S.A. 110 (36), 14646–14651. doi:10.1073/pnas.1309106110
Pereira, C. S., Silveira, R. L., and Skaf, M. S. (2021). QM/MM Simulations of Enzymatic Hydrolysis of Cellulose: Probing the Viability of an Endocyclic Mechanism for an Inverting Cellulase. J. Chem. Inf. Model. 61 (4), 1902–1912. doi:10.1021/acs.jcim.0c01380
Pham, L. T. M., Deng, K., Northen, T. R., Singer, S. W., Adams, P. D., Simmons, B. A., et al. (2021). Experimental and theoretical insights into the effects of pH on catalysis of bond-cleavage by the lignin peroxidase isozyme H8 from Phanerochaete chrysosporium. Biotechnol. Biofuels 14 (1), 108. doi:10.1186/s13068-021-01953-7
Pham, L. T. M., Kim, S. J., and Kim, Y. H. (2016). Improvement of catalytic performance of lignin peroxidase for the enhanced degradation of lignocellulose biomass based on the imbedded electron-relay in long-range electron transfer route. Biotechnol. Biofuels 9 (1), 247. doi:10.1186/s13068-016-0664-1
Piétu, G., Mariage-Samson, R., Fayein, N.-A., Matingou, C., Eveno, E., Houlgatte, R., et al. (1999). The Genexpress IMAGE knowledge base of the human brain transcriptome: a prototype integrated resource for functional and computational genomics. Genome Res. 9 (2), 195–209. doi:10.1101/gr.9.2.195
Pogni, R., Baratto, M. C., Teutloff, C., Giansanti, S., Ruiz-Dueñas, F. J., Choinowski, T., et al. (2006). A Tryptophan Neutral Radical in the Oxidized State of Versatile Peroxidase from Pleurotus eryngii. J. Biol. Chem. 281 (14), 9517–9526. doi:10.1074/jbc.M510424200
Pollegioni, L., Tonin, F., and Rosini, E. (2015). Lignin-degrading enzymes. Febs J. 282 (7), 1190–1213. doi:10.1111/febs.13224
Pollet, A., Lagaert, S., Eneyskaya, E., Kulminskaya, A., Delcour, J. A., and Courtin, C. M. (2010). Mutagenesis and subsite mapping underpin the importance for substrate specificity of the aglycon subsites of glycoside hydrolase family 11 xylanases. Biochimica Biophysica Acta (BBA) - Proteins Proteomics 1804 (4), 977–985. doi:10.1016/j.bbapap.2010.01.009
Quesada-Medina, J., López-Cremades, F. J., and Olivares-Carrillo, P. (2010). Organosolv extraction of lignin from hydrolyzed almond shells and application of the δ-value theory. Bioresour. Technol. 101 (21), 8252–8260. doi:10.1016/j.biortech.2010.06.011
Rabideau, B. D., Agarwal, A., and Ismail, A. E. (2013). Observed Mechanism for the Breakup of Small Bundles of Cellulose Iα and Iβ in Ionic Liquids from Molecular Dynamics Simulations. J. Phys. Chem. B 117 (13), 3469–3479. doi:10.1021/jp310225t
Rabideau, B. D., Agarwal, A., and Ismail, A. E. (2014). The role of the cation in the solvation of cellulose by imidazolium-based ionic liquids. J. Phys. Chem. B 118 (6), 1621–1629. doi:10.1021/jp4115755
Rabideau, B. D., and Ismail, A. E. (2015). Mechanisms of hydrogen bond formation between ionic liquids and cellulose and the influence of water content. Phys. Chem. Chem. Phys. 17 (8), 5767–5775. doi:10.1039/C4CP04060K
Radivojević, T., Costello, Z., Workman, K., and Garcia Martin, H. (2020). A machine learning Automated Recommendation Tool for synthetic biology. Nat. Commun. 11 (1), 4879. doi:10.1038/s41467-020-18008-4
Rapaport, D. C., Blumberg, R. L., McKay, S. R., and Christian, W. (1996). The Art of Molecular Dynamics Simulation. Comput. Phys. 10 (5), 456. doi:10.1063/1.4822471
Rashid, T., Kait, C. F., and Murugesan, T. (2016). A “Fourier Transformed Infrared” Compound Study of Lignin Recovered from a Formic Acid Process. Procedia Eng. 148, 1312–1319. doi:10.1016/j10.1016/j.proeng.2016.06.547
Ray, A. E., Williams, C. L., Hoover, A. N., Li, C., Sale, K. L., Emerson, R. M., et al. (2020). Multiscale Characterization of Lignocellulosic Biomass Variability and Its Implications to Preprocessing and Conversion: a Case Study for Corn Stover. ACS Sustain. Chem. Eng. 8 (8), 3218–3230. doi:10.1021/acssuschemeng.9b06763
Ren, H., Shi, C., and Zhao, H. (2020). Computational tools for discovering and engineering natural product biosynthetic pathways. iScience 23 (1), 100795. doi:10.1016/j.isci.2019.100795
Riccardi, D., Schaefer, P., YangYu, H., Yu, H., Ghosh, N., Prat-Resina, X., et al. (2006). Development of Effective Quantum Mechanical/Molecular Mechanical (QM/MM) Methods for Complex Biological Processes. J. Phys. Chem. B 110 (13), 6458–6469. doi:10.1021/jp056361o
Riou, C., Salmon, J.-M., Vallier, M.-J., Günata, Z., and Barre, P. (1998). Purification, Characterization, and Substrate Specificity of a Novel Highly Glucose-Tolerant β-Glucosidase from Aspergillus oryzae. Appl. Environ. Microbiol. 64 (10), 3607–3614. doi:10.1128/aem.64.10.3607-3614.1998
Romero, J. O., Fernández-Fueyo, E., Avila-Salas, F., Recabarren, R., Alzate-Morales, J., and Martínez, A. T. (2019). Binding and Catalytic Mechanisms of Veratryl Alcohol Oxidation by Lignin Peroxidase: A Theoretical and Experimental Study. Comput. Struct. Biotechnol. J. 17, 1066–1074. doi:10.1016/j.csbj.2019.07.002
Ruiz-Dueñas, F. J., and Martínez, Á. T. (2009). Microbial degradation of lignin: how a bulky recalcitrant polymer is efficiently recycled in nature and how we can take advantage of this. Microb. Biotechnol. 2 (2), 164–177. doi:10.1111/j.1751-7915.2008.00078.x
Sainsbury, P. D., Hardiman, E. M., Ahmad, M., Otani, H., Seghezzi, N., Eltis, L. D., et al. (2013). Breaking Down Lignin to High-Value Chemicals: The Conversion of Lignocellulose to Vanillin in a Gene Deletion Mutant of Rhodococcus jostii RHA1. ACS Chem. Biol. 8 (10), 2151–2156. doi:10.1021/cb400505a
Senn, H. M., and Thiel, W. (2009). QM/MM methods for biomolecular systems. Angew. Chem. Int. Ed. 48 (7), 1198–1229. doi:10.1002/anie.200802019
Sethupathy, S., Morales, G. M., Li, Y., Wang, Y., Jiang, J., Sun, J., et al. (2021). Harnessing microbial wealth for lignocellulose biomass valorization through secretomics: a review. Biotechnol. Biofuels Bioproducts14 (1), 1–31. doi:10.1186/s13068-021-02006-9
Shen, R., Olshen, A. B., and Ladanyi, M. (2009). Integrative clustering of multiple genomic data types using a joint latent variable model with application to breast and lung cancer subtype analysis. Bioinformatics 25 (22), 2906–2912. doi:10.1093/bioinformatics/btp543
Shen, T., and Gnanakaran, S. (2009). The stability of cellulose: a statistical perspective from a coarse-grained model of hydrogen-bond networks. Biophysical J. 96 (8), 3032–3040. doi:10.1016/j.bpj.2008.12.3953
Shi, J., Balamurugan, K., Parthasarathi, R., Sathitsuksanoh, N., Zhang, S., Stavila, V., et al. (2014). Understanding the role of water during ionic liquid pretreatment of lignocellulose: co-solvent or anti-solvent? Green Chem. 16 (8), 3830–3840. doi:10.1039/C4GC00373J
Singh, A. K., Katari, S. K., Umamaheswari, A., and Raj, A. (2021). In Silico exploration of lignin peroxidase for unraveling the degradation mechanism employing lignin model compounds. RSC Adv. 11 (24), 14632–14653. doi:10.1039/D0RA10840E
Smith, A. T., Doyle, W. A., Dorlet, P., and Ivancich, A. (2009). Spectroscopic evidence for an engineered, catalytically active Trp radical that creates the unique reactivity of lignin peroxidase. Proc. Natl. Acad. Sci. U.S.A. 106 (38), 16084–16089. doi:10.1073/pnas.0904535106
Sonoki, T., Takahashi, K., Sugita, H., Hatamura, M., Azuma, Y., Sato, T., et al. (2018). Glucose-Free cis,cis-Muconic Acid Production via New Metabolic Designs Corresponding to the Heterogeneity of Lignin. ACS Sustain. Chem. Eng. 6 (1), 1256–1264. doi:10.1021/acssuschemeng.7b03597
Teeri, T. T. (1997). Crystalline cellulose degradation: new insight into the function of cellobiohydrolases. Trends Biotechnol. 15 (5), 160–167. doi:10.1016/S0167-7799(97)01032-9
Thimm, O., Bläsing, O., Gibon, Y., Nagel, A., Meyer, S., Krüger, P., et al. (2004). MAPMAN: a user-driven tool to display genomics data sets onto diagrams of metabolic pathways and other biological processes. Plant J. 37 (6), 914–939. doi:10.1111/j.1365-313x.2004.02016.x
Tilay, A., Bule, M., Annapure, U., and chemistry, f. (2010). Production of Biovanillin by One-Step Biotransformation Using Fungus Pycnoporous cinnabarinus. J. Agric. Food Chem. 58 (7), 4401–4405. doi:10.1021/jf904141u
Totrov, M., and Abagyan, R. (2008). Flexible ligand docking to multiple receptor conformations: a practical alternative. Curr. Opin. Struct. Biol. 18 (2), 178–184. doi:10.1016/j.sbi.2008.01.004
Tu, W.-C., and Hallett, J. P. (2019). Recent advances in the pretreatment of lignocellulosic biomass. Curr. Opin. Green Sustain. Chem. 20, 11–17. doi:10.1016/j.cogsc.2019.07.004
Tuncbag, N., McCallum, S., Huang, S.-s. C., and Fraenkel, E. (2012). SteinerNet: a web server for integrating 'omic' data to discover hidden components of response pathways. Nucleic Acids Res. 40, W505–W509. Web Server issue). doi:10.1093/nar/gks445
Valdés, G., Mendonça, R. T., and Aggelis, G. (2020). Lignocellulosic biomass as a substrate for oleaginous microorganisms: a review. Appl. Sci. 10 (21), 7698. doi:10.3390/app10217698
Vandera, E., Samiotaki, M., Parapouli, M., Panayotou, G., and Koukkou, A. I. (2015). Comparative proteomic analysis of Arthrobacter phenanthrenivorans Sphe3 on phenanthrene, phthalate and glucose. J. Proteomics 113, 73–89. doi:10.1016/j.jprot.2014.08.018
Vandermarliere, E., Bourgois, T. M., Rombouts, S., van Campenhout, S., Volckaert, G., Strelkov, S. V., et al. (2008). Crystallographic analysis shows substrate binding at the −3 to +1 active-site subsites and at the surface of glycoside hydrolase family 11 endo-1,4-β-xylanases. Biochem. J. 410 (1), 71–79. doi:10.1042/bj20071128
Vázquez-Lima, H., Guadarrama, P., and Martínez-Anaya, C. (2012). Geometric distortions on a three-coordinated T1 Cu site model as a potential strategy to modulate redox potential. A theoretical study. J. Mol. Model. 18 (2), 455–466. doi:10.1007/s00894-011-1063-y
Vermaas, J. V., Dellon, L. D., Broadbelt, L. J., Beckham, G. T., and Crowley, M. F. (2018). Automated Transformation of Lignin Topologies into Atomic Structures with LigninBuilder. ACS Sustain. Chem. Eng. 7 (3), 3443–3453. doi:10.1021/acssuschemeng.8b05665
Wang, G., Zhao, Z., Ke, J., Engel, Y., Shi, Y.-M., Robinson, D., et al. (2019). CRAGE enables rapid activation of biosynthetic gene clusters in undomesticated bacteria. Nat. Microbiol. 4 (12), 2498–2510. doi:10.1038/s41564-019-0573-8
Wang, J., Hou, Q., Dong, L., Liu, Y., and Liu, C. (2011). QM/MM studies on the glycosylation mechanism of rice BGlu1 β-glucosidase. J. Mol. Graph. Model. 30, 148–152. doi:10.1016/j.jmgm.2011.06.012
Wang, Q., and Pang, Y.-P. (2007). Preference of small molecules for local minimum conformations when binding to proteins. PLoS One 2 (9), e820. doi:10.1371/journal.pone.0000820
Wang, Y., Song, X., Zhang, S., Li, J., Shu, Z., He, C., et al. (2016). Improving the activity ofTrichoderma reeseicel7B through stabilizing the transition state. Biotechnol. Bioeng. 113 (6), 1171–1177. doi:10.1002/bit.25887
Warshel, A. (1976). Bicycle-pedal model for the first step in the vision process. Nature 260 (5553), 679–683. doi:10.1038/260679a0
Warshel, A., and Levitt, M. (1976). Theoretical studies of enzymic reactions: dielectric, electrostatic and steric stabilization of the carbonium ion in the reaction of lysozyme. J. Mol. Biol. 103 (2), 227–249. doi:10.1016/0022-2836(76)90311-9
Warshel, A. (2014). Multiscale Modeling of Biological Functions: From Enzymes to Molecular Machines (Nobel Lecture). Angew. Chem. Int. Ed. 53 (38), 10020–10031. doi:10.1002/anie.201403689
Wilhelm, R. C., Singh, R., Eltis, L. D., and Mohn, W. W. (2019). Bacterial contributions to delignification and lignocellulose degradation in forest soils with metagenomic and quantitative stable isotope probing. Isme J. 13 (2), 413–429. doi:10.1038/s41396-018-0279-6
Wong, D. W. S. (2009). Structure and action mechanism of ligninolytic enzymes. Appl. Biochem. Biotechnol. 157 (2), 174–209. doi:10.1007/s12010-008-8279-z
Xu, H., Pan, W., Wang, R., Zhang, D., and Liu, C. (2012). Understanding the mechanism of cellulose dissolution in 1-butyl-3-methylimidazolium chloride ionic liquid via quantum chemistry calculations and molecular dynamics simulations. J. Comput. Aided Mol. Des. 26 (3), 329–337. doi:10.1007/s10822-012-9559-9
Yaegashi, J., Kirby, J., Ito, M., Sun, J., Dutta, T., Mirsiaghi, M., et al. (2017). Rhodosporidium toruloides: a new platform organism for conversion of lignocellulose into terpene biofuels and bioproducts. Biotechnol. Biofuels 10 (1), 241. doi:10.1186/s13068-017-0927-5
Yang, H., Watts, H. D., Gibilterra, V., Weiss, T. B., Petridis, L., Cosgrove, D. J., et al. (2019). Quantum Calculations on Plant Cell Wall Component Interactions. Interdiscip. Sci. Comput. Life Sci. 11 (3), 485–495. doi:10.1007/s12539-018-0293-4
Yao, A., Choudhary, H., Mohan, M., Rodriguez, A., Magurudeniya, H., Pelton, J. G., et al. (2021). Can Multiple Ions in an Ionic Liquid Improve the Biomass Pretreatment Efficacy? ACS Sustain. Chem. Eng. 9 (12), 4371–4376. doi:10.1021/acssuschemeng.0c09330
Yoo, C. G., Pu, Y., Li, M., and Ragauskas, A. J. (2016). Elucidating Structural Characteristics of Biomass using Solution‐State 2 D NMR with a Mixture of Deuterated Dimethylsulfoxide and Hexamethylphosphoramide. ChemSusChem 9 (10), 1090–1095. doi:10.1002/cssc.201600135
You, X., Wang, X., Liang, C., Liu, X., and Wang, S. (2019). Purification of hemicellulose from sugarcane bagasse alkaline hydrolysate using an aromatic-selective adsorption resin. Carbohydr. Polym. 225, 115216. doi:10.1016/j.carbpol.2019.115216
Youngs, T. G. A., Holbrey, J. D., Deetlefs, M., Nieuwenhuyzen, M., Costa Gomes, M. F., and Hardacre, C. (2006). A molecular dynamics study of glucose solvation in the ionic liquid 1,3-dimethylimidazolium chloride. Chemphyschem 7 (11), 2279–2281. doi:10.1002/cphc.200600569
Yuryev, A., Kotelnikova, E., and Daraselia, N. (2009). Ariadne's ChemEffect and Pathway Studio knowledge base. Expert Opin. Drug Discov. 4 (12), 1307–1318. doi:10.1517/17460440903413488
Zhang, X., Yang, W., and Blasiak, W. (2011). Modeling Study of Woody Biomass: Interactions of Cellulose, Hemicellulose, and Lignin. Energy fuels. 25 (10), 4786–4795. doi:10.1021/ef201097d
Zhang, Y., He, H., Dong, K., Fan, M., and Zhang, S. (2017). A DFT study on lignin dissolution in imidazolium-based ionic liquids. RSC Adv. 7 (21), 12670–12681. doi:10.1039/C6RA27059J
Zhang, Z., Liu, B., and Zhao, Z. (2012). Efficient acid-catalyzed hydrolysis of cellulose in organic electrolyte solutions. Polym. Degrad. Stab. 97 (4), 573–577. doi:10.1016/j.polymdegradstab.2012.01.010
Zhao, Y., Liu, X., Wang, J., and Zhang, S. (2013a). Effects of anionic structure on the dissolution of cellulose in ionic liquids revealed by molecular simulation. Carbohydr. Polym. 94 (2), 723–730. doi:10.1016/j.carbpol.2013.02.011
Zhao, Y., Liu, X., Wang, J., and Zhang, S. (2013b). Insight into the cosolvent effect of cellulose dissolution in imidazolium-based ionic liquid systems. J. Phys. Chem. B 117 (30), 9042–9049. doi:10.1021/jp4038039
Zong, Z., Li, Q., Hong, Z., Fu, H., Cai, W., Chipot, C., et al. (2019). Lysine Mutation of the Claw-Arm-Like Loop Accelerates Catalysis by Cellobiohydrolases. J. Am. Chem. Soc. 141 (36), 14451–14459. doi:10.1021/jacs.9b08477
Keywords: multi-omic analyses, lignin peroxidase, cellulase, predictive biology, ionic liquid, lignocellulosic biomass, computational biology, and chemistry
Citation: Pham LTM, Choudhary H, Gauttam R, Singer SW, Gladden JM, Simmons BA, Singh S and Sale KL (2022) Revisiting Theoretical Tools and Approaches for the Valorization of Recalcitrant Lignocellulosic Biomass to Value-Added Chemicals. Front. Energy Res. 10:863153. doi: 10.3389/fenrg.2022.863153
Received: 26 January 2022; Accepted: 17 June 2022;
Published: 15 July 2022.
Edited by:
Allison E. Ray, Idaho National Laboratory (DOE), United StatesReviewed by:
Antonio D. Moreno, Medioambientales y Tecnológicas, SpainCopyright © 2022 Pham, Choudhary, Gauttam, Singer, Gladden, Simmons, Singh and Sale. This is an open-access article distributed under the terms of the Creative Commons Attribution License (CC BY). The use, distribution or reproduction in other forums is permitted, provided the original author(s) and the copyright owner(s) are credited and that the original publication in this journal is cited, in accordance with accepted academic practice. No use, distribution or reproduction is permitted which does not comply with these terms.
*Correspondence: Seema Singh, c3NpbmdoQGxibC5nb3Y=; Kenneth L. Sale, a2xzYWxlQGxibC5nb3Y=
†These authors have contributed equally to this work
Disclaimer: All claims expressed in this article are solely those of the authors and do not necessarily represent those of their affiliated organizations, or those of the publisher, the editors and the reviewers. Any product that may be evaluated in this article or claim that may be made by its manufacturer is not guaranteed or endorsed by the publisher.
Research integrity at Frontiers
Learn more about the work of our research integrity team to safeguard the quality of each article we publish.