- 1Department of International Affairs, College of Arts and Sciences, Qatar University, Doha, Qatar
- 2Department of Industrial and Systems Engineering, Rutgers University, Piscataway, NJ, United States
- 3Department of Humanities, College of Arts and Sciences, Qatar University, Doha, Qatar
Urban communities differ in their social, economic, and environmental characteristics, as well as in the approach to energy use. Dynamic energy use and available on-site resources allow interaction with the surroundings and contribute to the key performance indicators of smart cities. This study aimed at proposing systematically a strategic framework for smart cities development by gradually transforming urban communities into smart-energy systems. This framework is based on multidisciplinary practices regarding the staged planning of smart communities and develops smart transformation concepts to enhance capacities toward the preservation, revitalization, livability, and sustainability of a community. In this study, we focused on the concept of smart and zero-carbon communities by using technology and infrastructure. We also considered the premise of the “community” and the related social, technological, and economic aspects. The decision constructs are explained from the perspective of a bottom-up approach ranging from preliminary inspections to economic investment planning. The study proposed a set of decision constructs aimed at allowing planners, engineers, and investors to have different alternatives at their disposal and select a feasible set of practical solutions for smart transformations accordingly.
1 Introduction
The energy sector has undergone a technology-driven transformation aimed at achieving smart and zero-carbon ecosystems initiated by the governments through intensive investments in Information and Communication Technologies (ICT)-based infrastructures. The end goal is to develop smart cities using efficient solutions and to reshape urban development through megaprojects and centralized master planning. This global and modernist approach is, however, controversial, which can be seen in the literature. In the last decades, the concept of a smart city has gained popularity owing to an emerging critical role of technology in cities’ urban sustainability plans, especially following the smart growth movement of the late 1990s (Susanti et al., 2016) (Harrison and Donnelly, 2011). The smart city concept refers to the implementation of cutting-edge technologies aimed at social development and economic growth. Cities use intelligent technologies and innovative design to offer high-quality services to citizens and reconstruct urban spaces to enhance life quality. Currently, more than 1,000 smart cities have been developed worldwide, mostly in Asia, Europe, and North America (Zheng et al., 2020). Yet, the definition of this concept in both academia and industry is lacking.
The performance metrics of smart cities consist of the following pillars: smart people, smart economy, smart environment, smart mobility, smart living, and smart governance (Zheng et al., 2020)– (Elessawy and Zaidan, 2014). Accordingly, traditional urban development theories are connected with modernization in sustainable development to provide a comprehensive definition of a smart city (Giffinger et al., 2007). This definition focuses on actors, technologies, and outcomes aimed at improving the city’s quality (Cocchia et al., 2014). The inclusion of those pillars of a smart city contributes to the effectiveness and efficiency of necessary and useful city activities, processes, and services. A city represents a smart system when investments are in line with human and social capital, advanced energy and mobility, and ICT infrastructure. Accordingly, participatory governance and the careful management of natural resources contribute to the sustainable development of the economy and enhance life quality.
There are three main approaches toward the concept of smart cities. The first is a techno-centered approach that assigns a critical role to ICT and it focuses on advanced technologies, hardware, and digital infrastructure. For example, Harrison et al. (Harrison, 2010) defined smart cities as a concept that integrates real data in real-time into a computing platform to offer services by implementing practices such as modeling, visualization, information processing, and optimization. Hancke et al. (Hancke and Silva, 2012) argue that a smart city operates in a sustainable and intelligent environment based on intelligent devices for monitoring and control. Moreover, its infrastructure and citizen services are cohesively integrated. Today, smart city technologies are used to improve urban life quality on daily basis in different fields such as society and economy (i.e., cultural heritage management, human capital management, digital education, innovation, and entrepreneurship), government (i.e., transparency, e-democracy, and e-governance), daily life (i.e., management of public spaces, culture, welfare and social inclusion, health, public security, pollution control, hospitality, and entertainment), smart buildings (i.e., housing quality, construction services, and facilities management), transport and mobility (i.e., services related to district information models, citizens mobility, mobility information, and city logistics), and natural resources and energy (i.e., food and agriculture, waste management, renewable energies, smart street lighting, and smart grids) [ (Sousa et al., 2012; Barthel and Isendahl, 2013; Gomes et al., 2014; Perera et al., 2014; Kingston et al., 2015; Chamoso and De La Prieta, 2016; De Paz et al., 2016; González-Briones et al., 2018; Abulibdeh, 2020a)]. Digital broadband technologies contribute to intra-community and inter-community interactions and they can enhance social inclusion, local prosperity, and competitiveness in the urban context. Moreover, these technologies can provide a communication platform for informed citizens to enhance further the development potential of the community (Albert et al., 2009). Numerous urban environments focused on providing sufficient digital infrastructure development for local stakeholders and citizens considering that digital broadband technology can transform current interaction patterns and communications increase efficiency in social, economic, and environmental urban processes.
The second is a human-centered approach and it emphasizes the role of social and human capital in the smart city. Different authors highlight the role of human capital and education in urban development and sustainability. The definition of the smart community proposed by Coe et al. (Coe et al., 2001) emphasizes the importance of social and environmental capital in urban development. Therefore, participation in community affairs, social inclusion, and decision-making processes are critical for reaching social and environmental objectives. Some studies investigated, the relation between the educated labor force and smart cities (Berry and Glaeser, 2005). This relation is critical for employment growth, economic development, and technology (Eger, 2009). However, intense debates are focused on social innovation and the role of the human factors and communities. The communities are regarded as key elements of the regeneration of urban areas using innovation. Accordingly, the concept of a smart community is based on the synergy using technology and infrastructure that is technically feasible and relevant for any region. Nevertheless, when the concept of the “community” is discussed, it is necessary to consider its critical aspects such as affordability, acceptability, privacy, and coherence. Moreover, the smart community is the concept encompassing advanced technologies and infrastructures aimed at sustainable development. Consequently, smart communities allow governance and infrastructural and technological drivers to generate social innovation. In turn, those innovations can engage local actors such as associations, businesses, and citizens, and accordingly respond to the challenges related to life quality, inclusion, and growth. Networking and information technology research and development (NITRD) offered a framework for smart and connected communities defined as “communities in all settings and at all scales that have access to advanced cyber-physical systems/Internet of Things [IoT] technologies and services to enhance the sustainability and quality of life and improve health and safety and economic prosperity for their residents” (Sun et al., 2014). The generation of smart communities is aimed at their preservation, revitalization, livability, and sustainability.
The third is an integrated approach arguing that human and social capital and technology create jointly adequate conditions for a continual process of growth and innovation (Bencardino and Greco, 2014). A smart city integrates human capabilities, knowledge-intensive activities, institutional mechanisms for social cooperation toward knowledge, and innovation development and digital infrastructure (i.e., ICT infrastructure, tools, and applications). Accordingly, the smart city represents an environment that can combine sustainability and competitiveness by integrating different dimensions of development (i.e., social, economic, environment, and living, and governance, people, and mobility). The focus is on ICT drivers and how they influence urban development. All definitions of smart communities, share three common concepts: the process (networking of various actors); communication means (ICT, technology, and network infrastructure); and the goal pursued (public involvement or other).
The Climate Change Performance Index (CCPI) (‘Climate Change Performan, 2022a) is one of the significant metrics that can evaluate the commitment of governments supporting the transformation toward a zero-carbon energy system and technological developments. The CCPI is calculated using 14 indicators classified into four categories (‘Climate Change Performan, 2022b): 1) Greenhouse gas emissions 2) Renewable energy sources 3) Energy consumption 6) Climate policy. Based on Climate Change Performance Index 2022 results (Jan et al., 2022), countries can be classified the into three main clusters, “Alpha”, “Beta”, and “Gamma”, as displayed in Table 1.
- “Alpha” or “High commitment to smart and zero-carbon transition, GHG Emissions, renewable energy and climate policy”. This cluster is primarily composed of northern European nations that able to perform in the CCPI. They are all targeting a zero-carbon future, reducing GHG emissions, and contributing to the Green Climate Fund (‘Green Climate Fund’.2022, 2022), while also participating actively and constructively in climate legislation. Sweden exemplifies the hypothesis that the more industrialized countries are more environmentally friendly (Yesiloglu et al., 2019), having committed to producing 100 percent renewable electricity by 2040 and imposing the world’s highest carbon tax (delclima, 2019). Despite these encouraging findings, there is still room for improvement in terms of energy efficiency (Sweden and Norway) (‘Nordic Energy Technology, 2013) or carbon reduction in the transportation, building, and agriculture sectors (Denmark) ((2022). Denmark: foc, 2022). As a result, none of them achieves the maximum CCPI pillar score.
- “Beta” or “Medium commitment to Smart and zero-carbon transition”. This group is primarily formed of eastern European states, but also includes nine other nations from different continents. These are countries whose commitment to combating climate change should be bolstered by more effective measures targeted at lowering GHG emissions and increasing the use of renewable energy; in other words, they require stronger environmental legislation. Romania and Bulgaria, in particular, lack a strategy for coal phase-out and rely significantly on fossil fuels for energy (Zlateva et al., 2020). Typically, these are countries with significant economic resources to accelerate the shift to cleaner technology. Nonetheless, there is a need for increased awareness and action on the part of the competent agencies.
- “Gamma” or “Low commitment to smart and zero-carbon transition, GHG emissions, renewable energy and energy use”: This category is consisting mostly of eight Asian countries, United States, Australia and Canada, all of which are ranked at the bottom of the CCPI. Globally, the poor climate performance is a result of high per capita GHG emissions, particularly in Canada, which ranks first among the most active emitters (Davis et al., 2018), a lack of commitment to renewable energy implementation, and a lack of long-term energy planning. Specifically, Hungary and Poland’s lackluster activities are impeding the EU’s 2030 Climate Target Plan (Poland (2022). Hungary th, 2022). Saudi Arabia is particularly deserving of consideration. This country is highly reliant on fossil fuels and has substantial challenges in transitioning to renewable energy. Similarly, approximately 80% of Iran’s electricity producing sector’s energy demand is satisfied by fossil fuels (Shahsavari and Akbari, 2018).
The concept of smart communities has been developed as a result of competitiveness gains and community development aimed towards new network opportunities. Revolutionary network developments and technologies offer access to knowledge, resources, and tools for connection, and accordingly, they have a considerable impact on the interactions of individuals, local governments, institutions, and businesses. Smart communities are, thus, clusters that can establish alliances and partnerships via electronic networks and the internet, leading to novel economic and social values (anadian Federal Gove, 2002). In particular, it is critical to invest in human and social capital along with the network deployment (transport and ICTs) to reach objectives of the sustainable community and enhance life. To achieve this, social participation, as well as user-specific technologies and community-building applications, should be fostered (Komninos, 2009).
2. Knowledge Gaps
Despite improvements in smart cities and communities, certain gaps regarding the integration of the multi-dimensional domain knowledge of this transition to create a meaningful roadmap remain. This integration is highly complex considering that smart transition involves human, technological, economic, policy, and environmental factors. The integration is a prerequisite for complex large-scale projects because such projects must respond to and incorporate gradual, but constant changes of communities. However, it is still not investigated how this incorporation can occur. Moreover, up to date, the convergence of regional projects aimed at achieving high-level sustainability objectives has not been explored.
Accordingly, further research is needed to develop a multistage and multidisciplinary transition plan that will incorporate all aforementioned factors into the transformation of an existing urban community into a smart system to achieve high-level sustainability goals. As argued by Israilidis et al. (Israilidis et al., 2021) studies conducted on smart city projects so far have not been systematic enough to conduct efficiently future smart city policies and practices. The reason is that both academic studies and policies have been primarily focused on addressing solely technical issues and accordingly, further research is urgently needed to address non-technical aspects. Our study intends to overcome this gap. Accordingly, the transition plan must take into account social, economic, environmental, and technological factors and constraints based on both local and regional contexts. We particularly focus on local and regional context, considering that efficient engagement and cooperation of different stakeholders (e.g., private, public, and civic) is critical. Furthermore, Walker et al. (Walker and Devine-Wright, 2008) proposed the assessment of energy and environmental projects at the neighborhood level, as opposed to the approach focused on single buildings. However, we assume that the convergence of neighborhood policies is needed to achieve sustainability policies at the higher levels, following the argument by Kim et al. that energy management at the city level remains unsatisfactory. However, drawing upon Walker et al. (Walker and Devine-Wright, 2008) and Kim et al. (Kim et al., 2021), we devised a bottom-up approach to energy transition of smart communities and their convergence by particularly focusing on non-technical aspects. Thus, the purpose of this study is to propose a strategic knowledge-based framework based using a systems approach that incorporates all of these factors emphasizing social and energy dimensions. The objective is to develop a set of simple-to-use decision constructs for investors, engineers, and planners to allow them exploring various alternatives and identifying a feasible set of practical solutions for a smart transformation.
3 The Proposed Framework
3.1 From Connected Communities to a Connected World
The transition from a community’s targets and plans to those at the city level is highly challenging considering an exponential increase in complexities, particularly owing to the inevitable inclusion of social and policy factors at the greater scale. Thus, the transition at the city level should be conducted in two ways—distributed and incremental to achieve the transformation of a connected network of smart communities into large-scale smart cities. The communities assumed as capable of a smart and sustainable transformation have the following common characteristics: public participation, asset ownership and management, available resources, heterogeneous and flexible energy demands, and scale. Consequently, it is possible to establish a knowledgeable resource-driven system that includes individuals residing in it. The transition involving underprivileged communities is an excellent showcase of economic and social benefits resulting from the transition at the community and city levels. Furthermore, certain areas have a considerable footprint and are highly vulnerable in an environmental sense, namely major commercial areas, transportation hubs, and ports.
From an energy perspective, the continual advancement of smart technologies regarding energy systems, advanced automation, IoT, and ICT has enabled the connection of a distributed network of smart communities. Energy consumers, such as assets, buildings, and mobility, and energy providers within the community should operate in synchronization to achieve optimization in terms of energy conservation and energy efficiency. Smart technologies and data (e.g., waste, water, and electricity tracking, energy use optimization, and air quality monitoring) can be used to considerably enhance life quality for the citizens, increase their opportunities, and ensure a sustainable future. The use of these technologies will lead to considerable saving of water and less solid waste per person annually, interception of pollutants, and fewer GHG emissions. However, to meet the objectives of a smart city, it is necessary to combine community- and facility-level actions. The incremental transition takes the form of the establishment of systems-aware community clusters to achieve the broader impacts of all sub-systems in high-level logistics. Accordingly, smart cities are reinforced, and a smart economy, smart society, and smart governance are enhanced. Such a holistic system is accessible and inclusive, and consequently, the life quality, in general, is improved. Furthermore, it is important to note that environmental impacts are not limited to GHG emissions. Moreover, the synergy should be established within a connected system. This connected architecture allows the communities to operate in a connected world and exchange their resources in an energy transition roadmap. More precisely, the transactive energy exchange between communities can be envisioned although costs and benefits have not been specified yet. Furthermore, community-to-community offerings could have particular financial parameters. This connected network will allow optimal management to expand sustainability and efficiency. Various measures can be used to define communities and collaborating entities in a connected energy world. Concepts that can serve as a baseline for the incremental transition to a smart city are nanogrids (NG), microgrids (MG), and community energy.
3.1.1 Community Energy and Zero-Carbon Communities
Community energy (CE) is a potential concept to be applied to a multistage transition to a connected energy world (‘Climate Change Performan, 2022a). Through CE, communities can be transformed into zero-carbon energy systems by prioritizing the participation of local community members. Worldwide, this strategy has attracted considerable attention, particularly in Denmark, the United Kingdom, Germany, Australia, and the United States (MacArthur, 2017). CE can provide alternative energy initiatives aimed at local people and conducted by local people, in contrast to traditional energy systems (Walker and Devine-Wright, 2008), (Hoffman and High-Pippert, 2005), (Abulibdeh, 2021a). Led by people residing in the community, those communities can significantly benefit through CE projects (Rogers et al., 2008). Various assumptions on the processes such as increased local support for renewable energy, socio-economic regeneration, energy literacy and environmental lifestyles, knowledge and skill development, community empowerment, improved social capital, access to affordable energy, ownership, participation, and decision-making are critical for the outcomes of CE (Berka and Creamer, 2018)– (Abulibdeh, 2021b). However, there is no strong evidence supporting the theoretical assumptions of benefits to the local community (Berka and Creamer, 2018). Accordingly, it is necessary to investigate those benefits systematically, comparatively, and empirically (Wyse and Hoicka, 2019). The studies should explore technological, economic, and social factors that impact the sustainable transition to zero-carbon, in addition to the factors impacting uptake and successful implementation.
CE is primarily defined by the way communities participate in energy developments. Through CE, citizens actively participate in policy perceptions, and energy production, delivery, and consumption, in contrast to traditional energy systems (Ceglia et al., 2020) (Wyse and Hoicka, 2019). CE consists of open participatory practices based on the participation of community members. This allows gathering comprehensive evidence-based data based on a variety of local perspectives (Abulibdeh, 2021a), (Wyse and Hoicka, 2019), (Groves et al., 2013), (Hoffman et al., 2013). The new approaches and practices of CE systems primarily focus on community participation and resemble bottom-up approaches (citizen involvement and a variety of community actors) in opposition to top-down approaches (institutionally-driven traditional systems) (Wyse and Hoicka, 2019), (Groves et al., 2013)– (Devine-Wright and Wiersma, 2013). Therefore, community members’ participation is critical for the efficient adoption of non-carbon energy sources and emission reduction. Accordingly, it is necessary to investigate and determine related socio-economic factors. As a result, residents participate in the process impacting their lives. Moreover, a high level of place attachment and related positive emotional bonds between people and the environment can have a considerable impact on either opposing or supporting the transformation toward a zero-carbon energy system and technological developments (Wyse and Hoicka, 2019). The opposition or support depend on the perceptions of new technologies, which can be regarded as a threat or an opportunity to the local community. Thus, the concept of a “community” is useful for investigating how new technologies and new technological activities such as the transformation toward zero-carbon energy systems and technologies and their associated risks are related to people’s feelings about their community (Jin, 2015).
3.1.2 Microgrid and Nanogrid Concepts
Distributed energy resources (DERs) have been widely adopted in power systems, and their use is continually increasing. Therefore, it is necessary to revise current business models and infrastructure behind the grid operations to make them more environmentally friendly and reliable. This is a critical component of the energy transition, which is a prerequisite for achieving smart cities. Among viable solutions, the micro-grid (MG) connects DERs in local areas into a mini-grid that has a controllable aggregated node from the utility-grid side. In the relevant literature, MG is defined in different ways formally. According to the US Department of Energy, the MG represents “a group of interconnected loads and distributed energy resources within clearly defined electrical boundaries that act as a single controllable entity with respect to the grid” (Madushan and Ralalage, 2020) (Ton and Smith, 2012). Furthermore, the International Council on Large Electric Systems (CIGRÉ) defines the micro-grid as “a group of interconnected loads and distributed energy resources within clearly defined electrical boundaries that act as a single controllable entity with respect to the grid. An MG can connect and disconnect from the grid to enable it to operate in both grid-connected or island mode” (Nordman, 2009).
Drawing upon these definitions, the MG consists of the following primary components: controllers, a set of sensors, AC-DC converters, wires/pipes creating electrical/thermal networks, supply-demand nodes, and switches operating as the MG’s central information processing that ensures smart energy management (Karki and Chanana, 2016). MGs represent locally controlled systems; thus, they operate in both grid-connected or islanded modes while meeting network constraints such as nodal voltages and feeder power flow in electric-only MGs. The islanded mode allows MGs to operate all/parts of their critical loads during a crisis such as power outages and storms, whereas the upstream grid is jeopardized in such situations (Shahsavari and Akbari, 2018). Hence, MGs have advantages such as more reliable and resilient service (Ortmeyer et al., 2016); congestion relief and T&D investment deferral through reduced peak loads (Jin, 2015); ancillary services as a result of co-optimization of the DERs’ dispatch (CONTRERAS et al., 2019); and environmentally friendly operations owing to increased renewable energy integration enabled by electric/thermal storage capabilities (Abu-Elzait and Parkin, 2019).
Following the design of the MG, a meshed-grid layout with multiple loops is typically selected to improve its reliability and security. The meshed-grid layout incorporates a set of controllable switches, which can ensure the continuity of the service (Ortiz et al., 2020) (Aluko et al., 2020). Furthermore, a critical feature of an MG is optimal selection of the point of common coupling, or more precisely, a static switching node allowing both grid-connected and islanded operation modes. In general, an MG requires a plus-one distributed generation (DG) unit. This one can be in the form of renewable energy sources (RESs), which includes biomass-fueled DGs, fuel cells, wind turbines, and solar panels, fossil-fuel-based dispatchable DGs (DDGs), including gas-fired and diesel-fueled DDGs, or hybrid DGs such as a combined heat and power (CHP) system to maintain operating the critical loads once the MG is islanded. In addition to hybrid DSs, RESs, and DDGs, demand response (DR) resources and energy storage systems (ESSs) and demand response (DR) resources are also classified as DERs when designing an MG. Therefore, DERs act as the prime mover of any MGs whose portfolio is optimized in regard to siting and sizing. The choice of the technology is based on the MG operator’s purposes and goals, thermal energy needs, fuel accessibility, and operational parameters. Fundamental for the efficient operation of an MG is to ensure a full state-aware energy management system using metering that can take prompt control actions. The role of the energy management systems is to perform the coordinated operation of DERs within the MG’s boundary and continually optimize the supply-demand balance. It also needs to incorporate DR resources as a set of flexible options to respond to changes in demand. The MG and NG, referred to as fractal grids as well, are similar, but the NG represents a smaller scale system. Accordingly, they conduct operations for only one facility or customer. They can function under both DC and AC modes. The most widely used NGs are joint solar-storage systems. Importantly, NGs can be connected to the utility grid through a meter, implemented within MGs, and connected with other NGs (Nordman, 2009).
Considering a technical perspective point of view, the MG and NG concepts are communities capable of supporting the multistage planning of developing smart cities in the smart energy transition (Antonysamy et al., 2020). Such communities, using the concept of community energy, can enhance resiliency and service quality in energy systems, enhance citizens’ well-being, and facilitate smart transformation (PriyaDharshini et al., 2022).
3.2 Social Factors and Smart Transformation
Community smart energy transformation can be hindered by social and behavioral elements such as a lack of willingness, acceptance, and awareness, preventing the deployment and expansion of technology alternatives. Therefore, smart transformation must be advocated and implemented through a participatory strategy involving social actors in all horizontal and vertical processes. Human and socio-economic factors ought to be included at all decision-making stages of the smart transformation. Importantly, it is critical to highlight that such factors are case-specific; thus, it is not possible to provide general conclusions. Such factors can be categorized into policy, structural and demographic factors, cultural barriers, and psychological drivers.
For example, demographic factors impact individuals’ decisions regarding pro-environmental strategies (Niamir et al., 2018), (De Silva and Pownall, 2014). To illustrate, the studies of Mills and Schleich (Mills and Schleich, 2009) and Michelsen and Madlener (Michelsen and Madlener, 2012) demonstrated that German households consisting of individuals with higher levels of education are more inclined to use solar water heating. Furthermore, Mills and Schleich (Mills and Schleich, 2012) investigated households in European countries and confirmed that those with a university degree are more likely to use energy-efficient technologies. Comparably, Sardianou and Genoudi (Sardianou and Genoudi, 2013) showed that educated Greek households are more inclined to adopt renewable energy resources. In addition, Niamir et al. (Niamir et al., 2020) found that educated households in two European regions invest in housing insulation and solar panels more than those less educated and are more likely to choose a green energy provider. In addition, gender is a critical factor in correlation with the adoption of pro-environmental strategies. Several studies have demonstrated that women are more inclined to adopt energy conservation policies. According to Zelezny et al. (Zelezny et al., 2000), most studies have confirmed that women are more likely to engage in environmental protection. However, studies have also reported that men are inclined to install solar panels (Niamir et al., 2020). Furthermore, age is also a critical factor because older individuals are more skeptical regarding the return on investments in energy solutions (Nair et al., 2010). In contrast, some studies have reported that the older population is more likely to use than younger homeowners [ (Long, 1993; Barr et al., 2005; Ghofrani et al., 2021)]. Research on the relationship between income and attitudes toward technology adoption has demonstrated that the inclination to adopt renewable energy technologies is in correlation with monthly income (Sardianou and Genoudi, 2013). Similarly, homeowners with high incomes are more inclined to invest in solar hot water technology (Sidiras and Koukios, 2004).
On the other hand, psychological drivers, primarily personal and social norms, influence smart transformation decision-making. Social norms represent “perceived social pressures from significant others and/or beliefs about how significant others expect one to act in a given situation that impacts the individuals’ degree of reliance on policies” (Chen et al., 2017). Furthermore, Allcott (Allcott, 2011) confirmed that social norms influence energy efficiency in the US. These norms provide information about residential end-users, which allows comparing their consumption patterns with the community and envisioning tips and recommendations on energy conservation practices. In addition, in a study conducted in China, Wang et al. (Wang et al., 2011) demonstrated that social norms are related to increased household electricity saving. Also in the Chinese context, Chen et al. (Chen et al., 2016) demonstrated that social norms positively affect the intention of people to adopt vehicles that use non-fossil fuel energy and solar water heating systems. Moreover, Han et al. (Han et al., 2010) revealed that because of perceived social norms, customers choose environmentally friendly hotels. Furthermore, personal norms represent the perception of having a moral obligation to adopt a new behavior (Huijts et al., 2012). According to Werff et al. (van der Werff et al., 2019), in the Netherlands, households with stronger personal norms are more inclined to exhibit an energy-saving attitude. A study conducted in Spain and the Netherlands by Niamir et al. (Niamir et al., 2018) demonstrated that personal norms positively impact the decision to invest in appliances that help save energy, solar panels, and housing insulation, solar panels. Moreover, cultural barriers such as thermal comfort, vandalism, social exclusion, social status, ethnic bias, and masculinity, can negatively impact (Sovacool and Griffiths, 2020). For instance, social status represents a main cultural barrier to shared transportation in GCC countries because car ownership is a crucial status of wealth (Abulibdeh, 2020b), (Abulibdeh and Zaidan, 2018). Similarly, in Australia and the US, masculinity and machismo are the primary factors behind aggressive driving and speeding (Schmid Mast et al., 2008), resulting in high CO2 emissions.
Another important social determinants that critically impact the transition is the structural factors, which refer to the characteristics of buildings (e.g., size, age, and type). To illustrate, Mills et al. found that older houses are less energy efficient (Mills and Schleich, 2009). Besides, Niamir et al. (Niamir et al., 2018) argued that homeowners are more inclined to invest in green energy than apartment owners, because owners of new buildings are more likely to invest in solar panels. In addition, there is a positive correlation between the size of the house and the investment in solar panels and housing insulation. It is assumed that the owners of larger houses have a higher income and are able to invest in green energy. However, larger houses also have larger rooftop spaces that can host a solar panel system.
Finally, financial policy factors such as the cost of carbon-neutral products and zero-carbon transition expenses also considerably impact the smart transformation (Zhang et al., 2011), (Cheng et al., 2019). Credits for consumers to purchase energy-efficient cooling and heating systems (e.g., Bulgaria) and the reduction of added-value tax for technology-saving, appliances/technologies that save energy contribute to the energy transition. According to Sardianou et al., subsidies and tax deductions positively influence the adoption of renewable energy resources by households (Sardianou and Genoudi, 2013). Furthermore, in the Chinese context, Li et al. (Li et al., 2016) demonstrated that preferential tax policies and government subsidies help consumers choose new energy solutions. A survey by Wang et al. (Wang et al., 2011) revealed that Beijing would purchase clean energy solutions if subsidized by the government. In addition, higher fossil fuel energy taxes would motivate individuals to opt for renewable energy. As Sardianou et al. (Sardianou and Genoudi, 2013) found, people are more likely to invest in green energy when prices of fossil fuel energy are higher. Drawing upon the literature, various types of incentives (financial, economic, and social) should be investigated to determine particular behavioral factors and evaluate how people adapt to new technologies to facilitate the energy transition process. Importantly, behavioral factors are dependent on social, temporal, and spatial factors. Thus, decision-makers must be informed about these factors to take appropriate actions to raise community awareness and acceptance, as well as to foster adequate norms and attitudes in the smart energy transition of communities.
3.3 Smart Transformation Roadmap
We define a smart community as a dynamic complex system with a hierarchical architecture that evolves over time. Besides, the bidirectional interactions and externalities crossing the system’s boundaries and the uncertainties created by factors such as human behavior and weather conditions present even more complexities in the planning and operation of smart communities. The smart transformation of an existing community consists of multiple phases in order to create a hierarchical architecture of information and asset layers that facilitate achieving the energy transition’s goals by adopting new technologies and establishing synergy to enable the ubiquity of devices and information fusion. The system should be designed based on an ICT infrastructure that is equipped with local automation systems, sensors and measurement tools, data hubs, database management systems, and central processing modules. For a smart energy transformation, technologies such as DERs, ESSs, district heating/cooling devices, and technologies that enable transportation electrification are inevitable. The end-use loads associated with built environments and mobility can be dynamically managed and reshaped based on the real-time and projected availability of resources and demand. As a result of the synergy within the system, a smart community can be regarded as a self-aware, resource-driven, and connected community that also has bidirectional interactions with its surroundings and the connected world (Figure 1).
The goal of this system is to achieve sustainability while having targets concerning cost savings, resiliency, quality of service, human life, system performance, responsiveness, and contributions to municipalities and the surrounding communities. In this framework, a smart community can achieve zero-carbon emissions and ensure the possibility of negative carbon emission by focusing on natural and artificial carbon capture approaches. It should be ensured that the design and information/asset layers are in accordance with environmental measures to achieve a zero-carbon state. Toward this smart and zero-carbon transition, the economic, technological, and social behavior impediments require comprehensive scrutiny. Aside from emissions reduction and performance targets, the impact of involving human factors such as willingness, productivity, and comfort in a smart transformation must be involved in the architecture. Through the use of DERs and shared district assets (e.g., heating and cooling, thermal storage, community solar, etc.), a smart community can improve its system resiliency to even contribute to its surrounding communities in normal operations and catastrophe events. A smart community can receive various signals from the connected world and combine these inputs with real-time information within its boundary to orchestrate its operation with municipalities based on different circumstances. Communication and IoT infrastructure of smart communities can create a real-time and fast-response system that is aware of single internal variations and external signals to enhance the system’s flexibility and responsiveness in planning and operations.
Nevertheless, considering continual technological changes in automation, ESSs, energy resources, computing power, innovative artificial intelligence (AI) solutions, and ICT, the potentials are constrained. AI solutions can be developed further owing to increased data sources with higher resolutions, resulting in comprehensive planning schemes and more reliable and precise predictive modeling, which includes the state of all participating nodes in the system. This is enabled by big data analysis and database management system platforms that are able to analyze enormous amounts of data. In addition, novel IoT architectures facilitate data transmission within the community and enable prompt response decision-making and system monitoring. Considering an increase in technology efficiency and decreased costs, clean power generation and storage systems have been established as economically justifiable. Furthermore, innovative universal gateways and protocols allow for the integration of more technologies as a network, thus increasing the potentials further. However, in order for the communities to comprehend their options and use them in the best way implies a necessity for establishing a scientific framework and a set of decision-making tools. More specifically, it is necessary that these decisions are based on massive amounts of dynamic and static data to develop a feasible solution for the smart transition. The smart transition, accordingly, is based on technological and managerial options and alternatives but constrained by economic, regulatory, and social factors. Figure 2 shows the decision-making stages required for establishing a transformation plan. The first stage is preliminary assessments, followed by setting targets, policy evaluation, virtual/physical prototyping, and economic analysis. The established targets should be checked by a decision-maker at each stage of the decision-making process. Furthermore, feasibility studies and technical/economic assessments should be considered to revise the transformation plan accordingly.
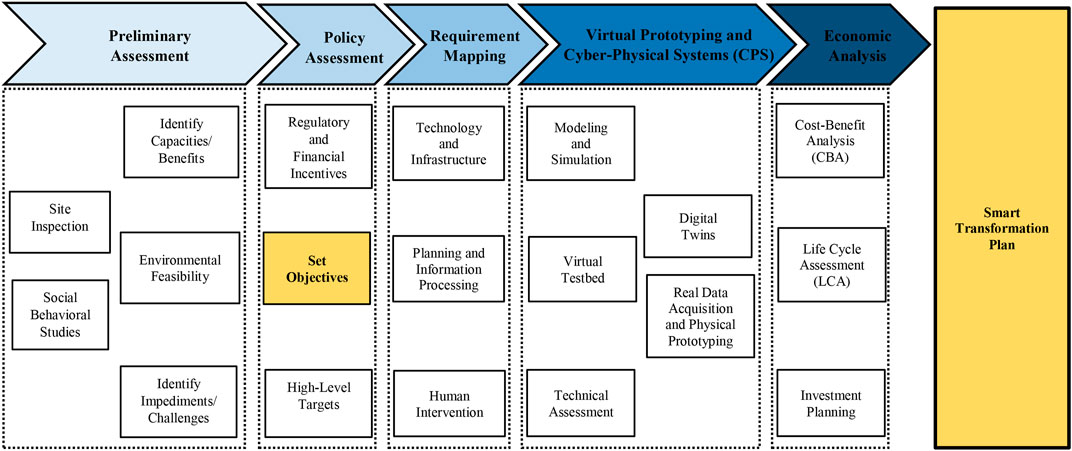
FIGURE 2. Strategic framework and multiple decision-making stages to establish a smart transformation plan.
To test and validate our approach scientifically, we applied the method inspired from (Chang et al., 2018) (Yigitcanlar et al., 2018) (Joshi et al., 2016) (Gondokusuma et al., 2019) including the followings steps to explore the research problem. The concept of smart community and estimated smart transition plans form the observation of our underlying scientific approach, followed by the question of the factors impacting the smart transition and the necessary phases of the smart transition. Next, we hypothesize in line with our observations that smart communities are highly dynamic and complex systems that continually evolve and engage in the interaction between the components within the boundaries of the smart community and with the surrounding beyond the boundaries, where multiple factors have an impact on it.
We conducted virtual prototyping to perform validation and evaluation prior to actual phases and physical implementations. As it was shown, virtual testbed enables for the co-simulation of various components such as data-driven modules, optimization modules, simulation programs, and mathematical modules into a computer-aided software environment, which can model information and physical layers and interaction within the smart community boundaries but also beyond them to evaluate various scenarios and contexts impacting a smart transformation. More precisely, we developed a virtual prototype to analyze the dynamics and behavior of a smart community. The virtual prototype allows decision-makers to carry out dynamic modeling on using analytical tools on the performance targets and measures of a smart community and the impact of investment on advanced technologies under various scenarios and circumstances. In particular, we focused on the buildings being a critical element of the smart community. More precisely, buildings represent the most complex systems and primary consumers in a smart community. To illustrate, we conducted both deterministic and probabilistic modeling of input to analyze the state of the community and the state of the surrounding using a local data hub. We defined the inputs (e.g., weather conditions, occupancy patterns, electric equipment use, gas equipment use, and device failure). The following controlled variables were selected: temperature setpoints, mechanical plant setpoints, mechanical plant operation, zone equipment control, air system control, and lighting control. Furthermore, the decision-making engine, which conveys the signals to the smart community, comprises dynamic planning optimization, static planning optimization, adaptive planning optimization, and forecast modeling. In addition, we determined the building outputs to be measured, namely electricity consumption, gas consumption, plant sensor data, zone sensor data, and equipment condition, as shown in Figure 6. Furthermore, we developed the decision-making engine of the virtual testbed aimed at obtaining the state of the smart community. This decision-making engine conducts planning optimization, static planning optimization, adaptive planning optimization, and forecast modeling as well. In addition, it aims at ensuring optimal battery dispatch by controlling and analyzing power, total current, total voltage, and discharge; optimal EV charging schedule by analyzing EV SOC, charger, and availability, and district heating/cooling operation schedule by considering cooling energy and heating energy. These local data hubs consist of an analytical layer, exemplified by the decision layer, control signals to simulation, and energy asset simulation layer (Figure 7).
In the subsequent step, in order to test the prediction, we designed case scenarios to perform technical feasibility assessments and to determine the primary value propositions of the smart community. We conducted co-simulation of a small community in a virtual testbed (Lawrence Berkeley BCVTB environment) comprising centrally controlled and integrated EVs, a fast-charging station, small-scale wind turbine, solar panels, battery storage, and two buildings. Through this simulation, we estimated power generation using a possible loss of loads, imported power from the grid, the role of energy storage in supporting intermittent renewable power generation, and transportation demand, building, and renewable energy resources to provide insights about surplus power generation and resource contribution to the surroundings, the air quality of the built environment, cost savings, and carbon emission reduction. The technical assessments show how optimization and data-driven frameworks increase performance through fast response, predictive decisions as a dynamic integrated system. Such simulation can lead to results that can serve as a basis for forming new predictions and hypotheses.
3.3.1 Preliminary Assessment
As demonstrated before, communities represent complex systems comprising different geographic and demographic characteristics, which are primary enablers providing capacities and benefits for sustainability targets and establishing the roadmap for the energy transition of a given community. Because renewable energy primarily depends on the presence or abundance of resources, the priority is a geological site assessment to assess available renewable options. In addition, demographics and social must be included in planning an energy transition. To identify plausible capacities of the site and the expected benefits from the transition, it is necessary to determine first the demographic and geological constraints by inspecting deficiency and abundance of resources and environmental limitations. The challenges to the plan implementation challenges should be taken into account as well.
3.3.1.1 Site Inspections
All communities have distinctive characteristics in terms of resources and current infrastructure and technologies. Typically, communities have on disposal smart lighting, building management system platforms, and district heating/cooling. Thus, an early-stage site inspection is needed to provide information about integrable assets and the necessity to upgrade or retrofit integration in a smart transition. The early-stage inspection includes assessing protocols, safety codes, risks, and asset ownership. In addition, to identify viable technologies and conduct feasibility studies, resource and land availability should be explored. In addition to the given community, it is needed to assess the surrounding and neighboring communities to identify particular limitations, needs, and infrastructure flexibility.
3.3.1.2 Social and Behavioral Studies
The main factors behind the transition to a zero-carbon city are social and human dimensions; therefore, visionary policies and full citizen participation are critical for achieving sustainable, tangible solutions. However, the correlation between human factors and smart transformation has not been clearly established due to a variety of those factors. Accordingly, the social and behavioral characteristics of the given community must be determined to identify the constraints, driving forces, and changing interactions between technology deployment and people throughout the transition. Whereas some behavioral and social attitudes contribute to the implementation of novel technologies during the smart transformation, others can hinder it. To summarize, contextual, economic, psychological, and personal factors together impact public acceptance and must be carefully investigated. In addition, it is necessary to define precisely the indicators for measure social adaptation and acceptance. In particular, it is necessary to include the socio-economic background of community members (e.g., gender, age, education, and employment), social and personal norms, attitudes and behaviors, position toward renewable energy, culture, time, system reliability, financial policy factors, knowledge, intentions to use, perceived enjoyment and sociability, anxiety, and costs. These indicators allow testing individuals’ acceptability of a new system of energy technology and willingness to participate in the transition to a zero-carbon energy system. Such investigation will provide significant insights into the public’s acceptance and adaptation toward new technologies and the transformation to a zero-carbon energy system. In addition, investigation of historical trends provides important insights into the adaptation of people to new technologies, which leads to their participation in the community transformation process. The transformation acceptance rates can considerably vary among the community members, depending on socio-economic factors, including gender, age, ownership, income, education, and willingness to purchase clean energy technologies. The awareness and acceptability of renewable energy systems and technologies are critical as well. Such insights are necessary to devise steps required for ensuring that new energy sources and technologies are accepted. More precisely, successful implementation depends on a proper understanding of social dimensions of flexibility for private and commercial elements of the community is pertinent to successful implementation.
3.3.1.3 Identifying Capacities and Benefits
The objectives of the system depend on its capacity, the capacities should be determined as well as expanded by a decision-maker in the pre-actional phase. Moreover, this phase should include identifying the communal resources and benefits expecting to result from the smart transformation. In addition, a smart community can operate as a responsive system embedded in future smart and sustainable cities. Importantly, the community has bidirectional interconnections with neighboring communities such as government bases, important facilities, and underprivileged communities. Accordingly, the benefits obtained by the smart community expand beyond its boundaries and therefore, are not limited to it. The benefits result from public welfare, infrastructure, resiliency, revenue streams, cost savings, resources, and the environment.
A strategic energy transition plan uses clean energy resources and energy-efficient solutions to achieve short- and long-term benefits regarding air pollution, ecological factors, climate change, and CO2 emissions. Communities with adequate infrastructure and resources in addition to efficient management can create benefits beyond the community boundaries. The paramount is resource sharing. To illustrate, district heating and cooling systems can be used to provide hot and cold water. Similarly, renewable energy investment opportunities are critical when there is available land within the community. Dynamic management increases capacities and enables the use of devices and information fusion, which, in turn, results in enhanced system performance. Consequently, the improved performance leads to cost-saving and more efficacy. Human comfort and well-being are improved whereas energy is saved via improved operation of the built environment and mobility. ESSs and DERs improve the capacities of the community by increasing its resiliency and preparedness against grid outages. In addition, the resilience of the surrounding is improved as well. Furthermore, during the normal grid operation, the community can be involved in the electricity market through frequency regulation and arbitrage to improve the reliability and operation of the grid and generate revenue streams. The smart community can contribute to a power system by preventing transmission and distribution line upgrades and consequently saving opportunities at a high level. The adoption of clean energy enhances human well-being and life quality and consequently enhances other capacities, which, in turn, result in numerous direct and indirect benefits.
3.3.1.4 Environment Feasibility
Furthermore, even in the case of economically viable and abundant resources, some strategic plans may not be feasible because of environmental constraints such as human health concerns, ecosystem conservation, and the protection of wildlife. Governmental environmental protection regulations also present limitations and prohibit certain practices. To illustrate, offshore wind technologies can endanger sea organisms and marine life. The development of hydropower and other renewable energies can damage the ecosystem, which is opposite to the objectives of the smart energy transition in communities. Therefore, the decision-making process has to take into account tradeoffs and penalizing factors in cost-benefit analysis.
3.3.1.5 Identifying Impediments/Challenges
Following the determination of the transition capacities and preliminary assessments, it is necessary to incorporate the challenges related to economic, social, and environmental dimensions in the transition targets. Any strategy must identify and include the main stakeholders in effective dialogue. The evaluation of deployment and implementation barriers is needed to determine the techno-economic challenges. Similarly, the assessment of challenges related to securing and preserving community goals in the long term is needed, as well as of challenges in effective operational control practices aimed at improving system performance and integrating human factors in decision-making and planning. Some examples are: How to approach equity issues to ensure that savings are properly trickled down among the main stakeholders? What are the social and economic drivers for people residing or working in the community to participate in energy efficiency and zero-carbon communities? What measures need to be taken to create a cost-effective smart community and to quantify the value streams? What are the implications for underprivileged communities close to the smart community? How can a smart community improve its surrounding municipalities socially, environmentally, and economically? What are the options for the last mile and integration of multi-modal transportation? How can electric vehicles (EVs) become a part of a smart community? Challenges related to privacy, system protection, and cyber security must be also considered when setting and mitigated by reliability measures at the asset level and precautionary security measures in ICT infrastructure at the information level. Privacy must be ensured by protecting user information using data protection methods such as encryption and anonymization.
Furthermore, the large-scale penetration of E-buses, E-trucks, and EVs can result in a considerable increase in electricity demand and a highly variable load profile for the community. Thus, it is necessary to envision solutions for this problem of conflicting energy reduction and mobility to minimize conflict. The strategy should look at load impact risks of EVs related to an increase in peak demand, uncertain demand changes to the distribution network, and economic and cost challenges. Researchers estimate a net increase in the overall miles traveled as a consequence of the growing adoption of commercial mobility solutions and autonomous vehicles. A drastic rise in EV and autonomous vehicle loads and its non-deterministic nature is likely to considerably influence the overall load profile of the host distribution network, resulting in transformation and substation failures. A functioning zero-carbon community should respond to these problems.
3.3.2 Policy Assessment
Policy and regulations are among the main drivers in an energy transition. Most of the time, investments and planning for smart energy transitions are not economically viable, or the payback periods are beyond an acceptable range and need government incentives and rebates. Besides, decision-makers put forth specific priorities that might contradict a viable transition plan in an existing community. Therefore, such political factors should be considered at both the early stages of decision-making and before setting the goals of a transition.
3.3.2.1 Regulations, Incentives
At the commercial level, there are financial constructs such as carbon credit or carbon tax, while for individuals, there are various types of economic incentives. Decision-making toward a transition should consider the flexibility to be offered either individually or at the community level through the collaboration of its constituents. The affordability of energy systems among buildings is often low, preventing investments that increase costs for the owners or tenants. Further, many business and technological solutions are developed and result in a lack of cost-effective solutions.
3.3.2.2 Master Plans and High-Level Targets
Governments set different sustainability priorities and targets. Communities are dynamic participating actors within smart cities, and, therefore, should be based on priority or high-level targets to achieve them. Master plans include technological priorities on the basis of factors initiated by legislation or government officials. The governmental master plans and priorities are aimed at unlocking government incentives and creating momentum to achieve sustainability goals and smart city targets (Zaidan and Abulibdeh, 2020).
3.3.3 Setting the Objectives
Considering a lack of a comprehensive definition of a smart system in the context of smart cities and communities, it is necessary to conduct preliminary assessments to set goals within a smart energy transition framework as a multidisciplinary practice. It is recommended that a plan includes measurable financial, social, physical, and virtual boundaries and attributes (e.g., environmental footprint, reliability, economics, efficiency, performance, and capacity). Having initial options that can be further developed is a prerequisite for a community to plan and assess the transition process. Planners and investors must follow a wide range of mixed or individual objectives at the pre-decision stage. Considering the importance of emission and monetary factors, they should be sub-categorized. The factors that ensure direction to transition targets are security, welfare contribution to the smart city, human productivity, resiliency, reliability, responsiveness, and service quality. A weighted sum of a set of targets should be a basis of the transition plan of a given community. Nevertheless, it is a challenge to quantify those targets and assign the weight vector.
A critical measure for assessing the goals of a smart community toward transformation into a zero-carbon community is the rate of carbon emissions and other hazardous gases (e.g., NOx). Building/community demand to measure emissions, power import from the grid, emergency backup generators, transportation, and clean on-site resources serve for smart community’s continual monitoring of power generation. Using the monitoring system also allows the assessment of the possible loss of load for resiliency metrics, system stability, power quality, cooling/heating demands, and the balance between the supply and buildings’ electricity demands. The effectiveness of a smart community can be also assessed via measures such as responsiveness and interoperability of the system, which are based on failures within the network, error rates, data traffic, and the control and device response time. The amount of cooling and heating energy and electricity exported to the surrounding is another measure used to evaluate the system’s contribution to the grid. Analyses of human productivity and performance and survey studies serve to monitor human impact dimensions.
3.3.4 Requirement Mapping
Meeting smart transition targets requires appropriate means and infrastructures along with effective management and interventions. Before framing the roadmap and architecture of a smart community, targets and expectations from the transition should be mapped using a set of effective tools. The tools are not limited to physical assets but also include logistic and management plans. There are enablers that can be leveraged to achieve the aforementioned goals. A robust technology plan and its implementation as supported by a life-long commitment are essential in the transition. Different power generation resources, ESSs, energy recovery systems, district heating cooling systems, smart lighting systems, HVAC technologies, and EV fast chargers are among the enablers that are in accordance with the transition targets. These assets can be individualized or shared at the community level, or a combination of both. Besides, one may decide on the use of advanced technology options with regard to automation and ICT such as IoT infrastructure, cloud services, edge technologies, advanced monitoring systems, distributed and adaptive building controls, transactive demand reduction, metering, and sensor devices, and so on. In addition, management and operation policies are necessary to implement the assets and infrastructure in an effective way to improve the overall system’s performance. Some of the policies that pave the way toward the community goals include advanced control logic in a built environment’s control, optimal scheduling for EV chargers, optimal dispatch planning for energy storage, and prognosis maintenance planning. Finally, the role of human factors in a transition should not be omitted. Most of the time, intervention strategies to shape and establish behavioral factors are necessary for positive motivational, coercive, and incentives approaches.
3.3.4.1 Technology and Infrastructure Requirements
Considering technology, numerous forms of constructs and enablers have been developed in energy and transportation. Following the transition goals, the next step is to compile a high-level matrix of technologies and relate them to the targets. Individual enablers contribute to reaching multiple targets with particular physical and financial constraints. It is possible to categorize the enablers of a smart energy transition into ICT infrastructure, automation, transportation electrification, ESSs, and energy and power generation resources. Technology and infrastructure are aimed at ensuring information fusion for real-time and optimal decision-making and the ubiquity of devices. The responses to the system state should be considered while managing and reshaping the end-use loads related to built environments and mobility. Communication and automation are used to create synergy within the community. To do so, it is necessary to employ decision-making platforms, computation modules, monitoring systems, IoT infrastructure, data aggregation, and sensor device, as well as measurement.
To establish a responsive and resilient energy system that can mitigate emissions and cost, ESSs and DERs should be implemented in smart communities. In the communities with available on-site resources and land availability, DERs can be used. Renewable energy resources such as ocean thermal energy conversion (OTEC), water waves and tidal energy, geothermal energy, offshore and onshore wind generation, and photovoltaic systems, and low and zero-carbon technologies such as CHPs and fuel cells can contribute to zero emissions. ESSs such as flywheels, hydrogen energy storage, and batteries can be integrated with to generate a dispatchable system and prevent an intermittent power supply. Technologies such as natural gas generators and diesel can be used for catastrophe preparedness and in the cases of blackouts as reliable, fast-response emergency backup systems to enhance system resiliency. To integrate these technologies, it is necessary to employ electronic devices such as voltage control across the power network, transformers, and inverters. When integrated with the grid, the system can import and export electricity on the basis of the balance between the supply and the demand on both sides.
District steam, cooling, and heating systems can be established in some communities. Chilled water is generated by chillers, whereas low, medium, and high-temperature water is generated by large-scale boilers. Thermal storage and the heating network combined can control the optimal dispatch of hot water generation. Ice storage units and district chillers combined can enhance their efficacy and displace their demand from peak demand periods to low demand periods. In the case of low cooling and heating demands in a community, the interconnection of steam networks and the hot and chilled water with the surroundings benefit the aggregate system.
Different communities are equipped with various types of light, medium, and heavy-duty vehicles, such as facility service fleets, freight transportation, university transit systems, and personal vehicles. Transportation electrification alternatives to be used in smart communities are battery and plug-in hybrid EVs. There is considerable potential of transportation for electrification and consequently, carbon footprint reduction. A system of distributed batteries can be used to implement EVsto stabilize and balance supply and demand within a power distribution network. EVs have become trustworthy and reliable for users owing to the advancement of fast chargers. Nevertheless, economic and cost challenges and load impact risks related to uncertain demand changes to the distribution network and peak demand increase are associated with fast chargers and EVs. Figure 3 shows that it is possible to differentiate controlled and uncontrolled EV-charging topologies can be differentiated. Smart communities and controlled topologies can be easily integrated to achieve the building demand through the grid-to-vehicle (G2V) and vehicle-to-grid (V2G) principles and for load balancing. The optimal dispatch control over the state of charge of the batteries contributes to emissions reductions and uses EVs in an economically viable way. V2G can be used efficiently during times of high peak demands and in both parking facilities. Vehicle-to-home (V2H) can also contribute to transacting load flexibility and reducing peak demand.
ICT systems based on a centralized web-based architecture allowing data accessibility across the smart community unlock the information fusion. The architecture is divided into a data sources layer, application layer, and network layer. The demand containing EV charging, occupancy patterns, building meter data and multiple sensor data points is the main data source. Other data segments consist of the state of charge of the ESSs and the time-series data for power supply. Measurement services and sensor points need particular communication protocols and devices with resolutions based on the service application and various granularity levels. Protocols such as BACnet for building management systems serve to ensure information integration and exchange at the local level. On the basis of the gateway protocol architectures of the IoT, as shown in Figure 4, they can communicate with the high-level network. Backend servers such as web interfaces for real-time decision-making and control and DBSM and other applications such as failures and anomaly detection the information and data flow. Technologies such as cloud computing, as well as fog and edge computing at an advanced level, are employed to ensure system performance due to the need for fast and responsive control signals and a high rate of data accumulation in a system. To ensure a responsive and infallible computing system, a suitable design for data preprocessing and database structures is needed as well.
The cloud-based decision-making platform acquires an enormous amount of information regarding the state of the sub-systems and processes the input to detect anomalies within a system’s components and propose an optimal operation plan for the network. Subsequently, the optimal control signals are implemented using field equipment such as DCS, DDD, and PLC, conveyed to the local facility level processes, and interpreted with the Fieldbus protocols, applications, and supervisory networks.
Smart communities also represent adaptive and dynamic connected systems, creating synergy within the system and beyond its boundaries. Synergy occurs via real-time data exchange and considerable data fusion owing to ICT. Accordingly, an open and expandable ICT infrastructure is required during the smart energy transition to achieve efficiency and connectedness. Thus, ICT is regarded as enabling technology that integrating the human factor into decision-making, serves as a reliable information exchange, measures, assesses and controls to enhance efficiency. Thus, by creating synergy, ICT allows the system’s participation outside of its boundaries and in the smart city and connected world. The smart communities consist of the components that are data sources. Accordingly, their state influences the entire framework as a dynamic system. Most of the exchanged information is based on the massive amounts of data flow to be handled by big data manipulation and processing.
Owing to expected responsiveness, real-time system, and scale ICT architecture in the smart community represents an intricate system. Therefore, it is necessary to investigate objectives to set goals for management, data queries, and data acquisition, interconnections, processes, and components. It is needed to classify data sources to evaluate the system’s requirements and determine proper granularity levels. Consequently, the connected system can be orchestrated instantly. Thus, a conceptual design should identify the functional requirements, components, consolidation processes, attributes, and entity relationships to build a logical data model before implementing the ICT. Preliminary assessment of performance and technical feasibility of various topologies for data collection and transmission is needed to establish an uninterrupted, continuous system. Centralized control, and flexible connectivity methods for edge device integration, centralized control, lowered installation and configuration periods of costs, reliable and scalable IoT cloud infrastructures, and user-friendly interfaces can lead to connectivity cost reductions. It is required to predefine data collection and protection and incorporate them into a system’s architecture.
Metering endpoints and sensors in automation and management systems follow standard industrial communication protocols such as BACnet, analog/digital I/O, Mbus, and Modbus RTU, and identified for the physical layers of the network. In addition, legacy and existing devices unmatched by the architecture can be integrated with interface modules and protocol converters. Wireless network technologies, cloud services, IoT applications, asset tracking applications, gateways, physical and communication layers, hybrid communication strategies, edge devices, and actuators and field sensors should be explored on the basis of different options regarding the coverage and exchange rate to establish a reliable communication system. Anonymization and encryption and other network and application layer security measures such as encryption and anonymization protect user privacy and system’s operations.
3.3.4.2 Planning and Information Processing Requirements
Adaptive and dynamic decision-making in the context of advanced technologies and extensive data fusion practices can considerably influence the performance and operation of communities regarding economic, social, and environmental measures, as well as mobility and energy. In addition, these technologies and practices impact the interaction of the smart community with the surroundings because it is a sub-system of smart cities and societies. Decision-making processes are based on methodologies related to data-driven approaches (e.g., reinforcement learning, deep learning, and ensemble learning) and advanced optimization (e.g., adaptive, dynamic, and static) to gain from the data. The transition is highly dependent on such tools in the pre-actional phase and subsequently during the entire system’s lifetime.
Short-term and long-term decision-making tools can be used for resiliency preparedness, surrounding interconnection, ancillary services, EV charge station planning, energy storage dispatch, resource planning, optimal power flow, building automation, maintenance planning, system upgrade planning, and investment planning. To illustrate, building operation schedules following optimal plans aim at cost reduction and energy efficiency consider factors such as on-demand planning and control, pre/post-occupancy, environment quality monitoring, temperature and humidity setpoint assignments, equipment control, load shift, resource availability to carry out actions such as smart lighting system control, asset conditions, occupancy, air quality, and weather conditions. The optimal actions stem from advanced optimization models processing input variables to determine a feasible solution by taking into account system constraints and objective functions. Those tools are selected during the pre-actional phase following community needs. When selecting the tools, it is necessary to specify state variables, control variables, technology parameters, and cost elements together with relevant projections and data repositories. At this state, the selection of algorithms and solvers and adequate dynamic/static and deterministic/stochastic is performed. Component behavior and relevant distribution functions are chosen and assessed if simulation tasks are needed.
In addition to the decision-making tools, AI applications and data-driven models serve to support system monitoring and predictive planning, carry out classifications and clustering, determine anomalies, and predict trends. Applications needed in the transition are fault and failure detection of assets, occupancy patterns, EV charge station usage, and prediction of building demands. The data-driven tools can process diverse and massive data sets to conduct predictive modeling, business intelligence, data visualization, and statistical analysis, and implement state-of-the-art AI applications.
3.3.4.3 Human Intervention Requirements
As discussed before, social and human factors impact the decision-making process and smart transformations. Before the implementation, it is necessary to analyze demographic data and conduct surveys on the target society to determine the amount of acceptance of new technologies and human participation in smart transition practices. If social or psychological factors hinder the smart transition implementation process, it is necessary to conduct intervention measures and the establishment of new norms before the actional phase. It is recommended to establish new behavioral factors such as skills, trust, emotions, attitudes, personal norms, and social norms to increase communal participation and achieve transition targets. In addition, intervention strategies should encompass coercive, motivational, and positive incentives within the community.
3.3.5 Virtual Prototyping and Cyber Physical Systems
Smart communities and smart transformations represent non-deterministic and complex systems; accordingly, virtual prototyping is required to perform validation and evaluation prior to actual phases and physical implementations. It is aimed at establishing a virtual testbed for the co-simulation of various components such as data-driven modules, optimization modules, simulation programs, and mathematical modules into a computer-aided software environment, which can model information and physical layers and interaction within the smart community boundaries but also beyond them to evaluate various scenarios and contexts impacting a smart transformation. The integration and communication between the component blocks during co-simulation development are highly challenging. More precisely, data flow and integration refer to the integration of the components of the smart community. Thus, stochastic and probabilistic modeling of input variables on the basis of validated and real data aimed at ensuring more representative and realistic interactions of the components. As shown in Figure 5, the actual representation of a system’s dynamics and system’s component models allow the development of a virtual prototype for decision-makers to carry out technical evaluations of various technology combinations (see Figure 5). The virtual prototype allows decision-makers to carry out dynamic modeling using analytical tools on the performance targets and measures of a smart community and the impact of investment on advanced technologies under various scenarios and circumstances.
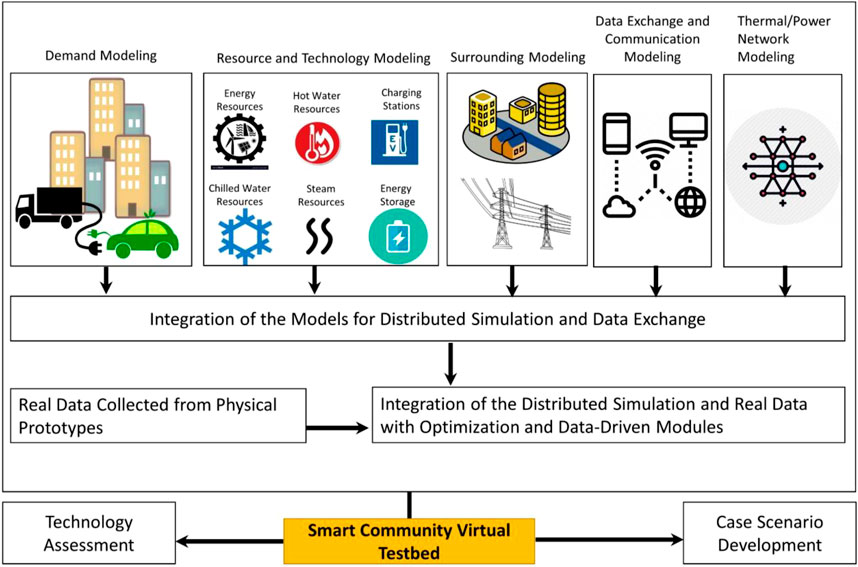
FIGURE 5. Development of a virtual prototype to analyze the dynamics and behavior of a smart community.
3.3.5.1 Mathematical Modeling and Simulation Tools
The behavior and dynamics of components of the system of the smart community are subject to change under various circumstances because they are not deterministic. Transportation systems, cooling/heating plants, storage systems, power and energy resources, and buildings have to be modeled before actional phases during a smart energy transition. It is necessary to integrate those components into simulation testbeds and co-simulations for feasibility assessments and technical analysis of various portfolios. Commercial tools for built environments and community assets used to simulate complex systems are Dymola, Trynsis, and EnergyPlus. Typically, simplified mathematical models are used to perform technical feasibility studies. Also, in decision-making and planning, they can be integrated into optimization models. In this transition assessment phase, decision-makers decide on adequate computer-aided engineering tools for the modeling process and the complexity level.
The most complex systems and primary consumers in a smart community are buildings (Jafari et al., 2020). Mathematical modeling and obtaining precise results of such systems is challenging; however, TRYNSIS and EnergyPlus can be used to conduct reliable analysis. Under different circumstances, simulations offer different parameters (e.g., indoor air quality) and outputs (e.g., load profiles). For instance, simulations can identify buildings’ saving capacities and opportunities. The simulation of a real building is costly and challenging; however, it is possible to calibrate reference models for various functionalities on the basis of weather conditions and actual buildings. Furthermore, DERs are critical components of the smart community aimed at achieving targets regarding interaction with the surrounding communities, cost reductions, resiliency, and emission reductions. Simulation tools and theoretical models investigate the dynamics of such systems within a smart community regarding degradation, asset failure, physical and operational constraints, design parameters, and input and output variables. Those models are processed in planning and optimization models, simplified and linearized forms, and as complex models operating in virtual testbeds and co-simulations. Thermal and power connections and networks are investigating in the modeling process in system simulations and planning optimization to perform a reliable analysis of the dynamics of the system in a smart community. The following factors are including in power flow modeling: loads in decision-making, storage, the interconnection of power generation, active/reactive power, power quality, voltage constraints, and power loss. In addition, piping systems and thermal networks need to be modeled to represent thermal losses and the connection between resources such as physical constraints in the system, heating/cooling demands, heat recovery systems, CHP units, boilers, and electric/absorption chillers. Communication and data exchange within a smart community must also be modeled to generate a realistic representation of the following factors: network delay, failure, noise, and data traffic. These assumptions enhance the transition analysis by encompassing factors in dynamic and real-time decision-making to achieve synergy within the system. Transportation systems, particularly charging stations and EVs, can be included in the modeling process to show the behavior of end-users in the system. Modeling the interconnection of a community with the dynamic behavior of the surrounding communities regarding thermal and power demand enhances the transition assessment. The surrounding community behavior is assessed on valid assumptions and historical data to evaluate the demand beyond the system’s boundary and the interactions between the available community’s resources. Factors such as social dimensions, climate patterns, energy market behavior, contingencies, and power outage events are modeled via historical data.
3.3.5.2 Virtual Testbeds
A virtual testbed is aimed at unlocking the co-simulation of advanced simulations such as TRNSYS and EnergyPlus, decision-making and optimization tools, and possible mathematical models, optimization and decision-making tools, data-driven and predictive models, and advanced simulations such as EnergyPlus and TRNSYS in one software environment to represent a system’s components, including surrounding communities, human behavior, data communication, EVs, ESSs, power generation technologies, and buildings. Inter-process communication tools such as Berkeley sockets embedded in building virtual control testbeds (BCVTB) proposed by Lawrence Berkeley Labs allow input/output streams of data among all the components. To illustrate, other variables should be processed in addition to incidental solar radiation and outdoor temperature to simulate a building’s physics over time. Controllable variables are related to the operational signals and control generated by decision-making tools embedded in a dynamic simulation to compute the optimal signals, analyze the state of the system, and obtain the state of each simulation block. This structure enables the user to perform various optimization practices regarding adaptive, dynamic, and stating planning and to virtually compare their influences on performance measures and targets of the community. Lastly, the output stream evaluates each component’s state, including state of charge of batteries, solar generation, building’s indoor temperature, and building demands. Owning to streams of data and information, dynamic simulation stores and tracks all the interactions within a system.
As discussed previously, stochasticity inputs are embedded into the virtual prototype of a smart community to provide a more reliable and realistic assessment. It is possible to use data repositories or on-site real data to assess the model parameters of the stochastic inputs. To illustrate, instead of using deterministic assumptions, stochastic inputs to model occupancy patterns can be constructed using the occupancy data for buildings. Moreover, it is possible to model asset failure using equipment failure data to assess a system’s responsiveness and reliability. In addition, the resiliency of the system can be estimated accurately using historical power outages. Examples of a dynamic simulation using virtual prototyping for a set of community-level technologies and building on the basis of the integration of optimization modules and stochastic input models in a system are shown in Figures 6, 7. Figure 6 shows how model definition inputs such as occupancy and weather patterns are generated on the basis of deterministic and stochastic and passed over a building’s simulation. Data streams can be simultaneously transferred to a decision-making engine to compute control signals, such as thermostat setpoints and a sequence of operation of equipment and the database of a virtual testbed by considering the state of a community and its surroundings.
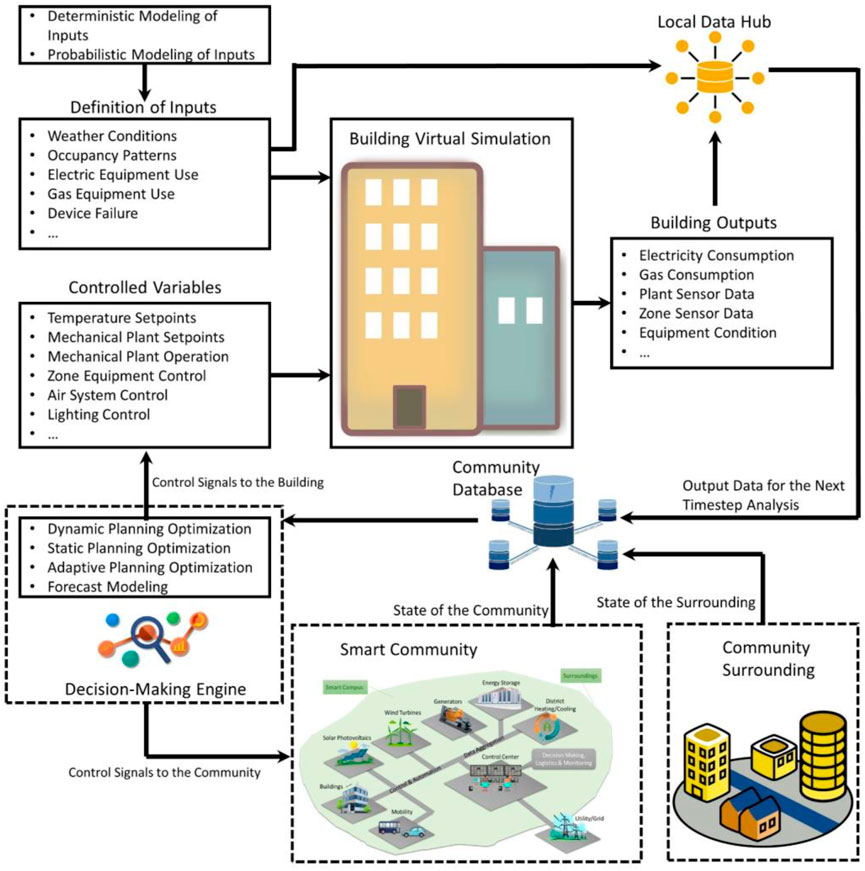
FIGURE 6. Example of input/output streams of data for a building as a component of a connected ecosystem.
Figure 7 shows a scenario in which the decision-making engine of the virtual testbed obtains the state of the smart community such as occupancy profiles, weather conditions, and its demand/supply patterns, weather conditions, occupancy profiles to establish optimal operation schedules for a district heating/cooling plant, EV charging station, and battery storage. The deployment and integration of the optimization and analytical modules require the engagement of the management of large data streams of data into a system and must be investigated during the construction phase of a virtual testbed.
3.3.5.3 Technical Assessment
Following the construction and integration of a virtual testbed and testing the system following the smart community’s architecture, the next step is to design case scenarios to perform technical feasibility studies and to determine the primary value propositions of the smart community. Figure 8 shows a co-simulation of a small community in a virtual testbed (Lawrence Berkeley BCVTB environment) comprising centrally controlled and integrated EVs, a fast-charging station, small-scale wind turbine, solar panels, battery storage, and two buildings.
This example shows the simulation of the system for planning externalities such as EV demand and weather conditions and time resolutions and horizons. Such a case scenario estimates power generation using a possible loss of loads, imported power from the grid, the role of energy storage in supporting intermittent renewable power generation, and transportation demand, building, and renewable energy resources to provide insights about surplus power generation and resource contribution to the surroundings, the air quality of the built environment, cost savings, and carbon emission reduction. The model can leverage various control policies in the operation planning of battery storage systems, EV fast chargers, and buildings in terms of the real-time and predicted state of the system. The analysis outcome should be compared with base case scenarios without smart architecture. Various combinations of portfolios and technologies regarding communication systems, control, transportation, buildings, and energy and power resources must be investigated to identify how components of the system contribute to achieving the targets of a smart community. The role of base case scenarios is to show how the smart transition and transformation to a connected system equipped with advanced technologies enhance a system’s performance and contributes to sustainability targets. The technical assessments show how optimization and data-driven frameworks increase performance through fast response, predictive decisions as a dynamic integrated system.
3.3.5.4 Digital Twins
To generate digital twin applications, a virtual testbed is implemented at the decision-making stage and throughout the system’s entire lifecycle. Digital twins were proposed several decades ago; however, owing to the exceptional advancement of the IoT, they are now regarded as future tools (Jafari et al., 2020). Importantly, digital twins can integrate machine learning and AI to combine contexts, algorithms, and data. Consequently, organizations can monitor items remotely, predict problems, and test new concepts. Previously, digital twins were aimed at enhancing the single asset performance (e.g., engine). Currently, they are used in the systems of assets at the organizational level. Thus, it is possible to implement the virtual testbed as a digital twin engine to include various types of process-based, statistics-based, and physics-based models, or their combinations.
3.3.5.5 Real Data Acquisition and Physical Prototyping
Although virtual testbeds can provide insights toward the impact analysis of smart transitions and technological feasibility, the acquisition of real data samples from the community and physical prototypes can enhance the reliability of the decision-making process. This phase can be regarded as a small-scale field experiment that enables the decision-maker to partially validate the virtual evaluations by real data. An IoT prototype can ensure expectations and system requirements. For instance, the range or data rate can be checked regarding a system’s responsiveness. Further, a small-scale PV-battery system or EV charge station can be used for both system assessment and data acquisition. Human interaction with technological prototypes can be tested and selected accordingly. Physical prototypes can even be integrated with a virtual software environment to assess the impact of planning and optimization modules on actual asset performance.
3.3.6 Economic Analysis
The evaluation of the smart community transformation is paramount to determine if it is worth modifying the lifestyle and the infrastructure to incorporate smart features such as optimal resource allocation, grid resiliency and responsiveness, less GHG emissions, energy-saving, and smart transportation. Thus, the assessment should answer if the transformation would enhance the well-being of the residents. The indirect and direct costs of services and technologies must be assessed in order to estimate the economic justification of the project and included in the planning and decision-making process. Moreover, it is necessary to quantify benefits related to system responsiveness, resiliency, environmental factors, energy efficiency, and performance. Government incentives, transfer payments, subsidies, and tax rebates should be analyzed in this phase.
On the other hand, in the phase of the transition’s economic analysis, business models and financial concepts should be adopted taking into consideration technological requirements, advancement, pricing, and availability to enable deployment. The smart energy transition must incorporate economic viability and financial sustainability factors. However, many options are not economically viable due to high technology prices, implying that new non-conventional, innovative solutions are needed to ensure financial benefits for individuals and commercial entities benefit from the transition. The justification of the economic viability of the transformation should be based on the quantification of its potentials and benefits of it. The potentials and benefits depend on particular demographics and other characteristics of specific societies.
The analysis should include, base case, time frame, goals, and project alternatives. In the second stage, non-monetary, indirect (intangible), and direct (tangible) impacts are determined. In the third stage, the costs (e.g., operation, maintenance, and capitals costs) and benefits (e.g., tons of carbon saving and kWh energy saving) are determined. In the fourth stage, quantified costs and benefits are combined under common terms. Importantly, other valuation techniques are used to estimate indirect costs, particularly in the cases of market imperfections, including fiscal policies distorting the markets, externalities, and market power. Following the monetization of all factors, their present value is determined in the fifth stage by discounting. Finally, the comparison of costs and benefits is conducted in the last stage by considering different interest rate scenarios.
3.3.6.1 Cost-Benefit Analysis
Owing to its design and objectives, a smart community can significantly benefit its surrounding, and it also must be included in the quantification for the CBA of the project. These benefits can be social, environmental, non-monetary (cost reductions and savings), and monetary. All these factors should be assessed when developing alternatives of smart transformation to estimate their feasibility. More precisely, it is necessary to quantify all the benefits and convert them to monetary values to allow comprehensive economic analysis. Thus, it is necessary to develop performance metrics to assess the benefits and value propositions in regard to targets, including service quality, responsiveness, smartness, resiliency, sustainability, and cost savings. Consideration of detailed economic impacts and investment assessments of technology portfolios is needed to perform CBA.
The critical drivers behind the design and functioning of a smart community are economic factors and costs. The deployment of on-site resources such as thermal and electric storage systems, cooling plants, district heating, and renewable or clean power generation serve to achieve revenue streams and cost reductions. Moreover, this can improve the performance of other communities as well. There is no need to import electricity from the grid in the case of on-site and distributed power generation; moreover, this form of power generation also enables the export of the surplus and participation in the electricity market for ancillary services and operating reserves. Accordingly, revenue streams are created and the operation and reliability of the grid are improved. In addition, on-site power generation is likely to reduce the costs related to the use of electric fleets and create opportunities such as V2G. Furthermore, the dispatch of power generation resources for optimal operation of increased cost-effectiveness can be achieved through energy storage technologies. In smart communities, district heating and cooling technologies can be combined with thermal and ice storage plants to provide additional hot and chilled water resources, leading to cost reductions and revenue generation. Furthermore, indirect cost benefits are created via DERs for a smart community by eliminating expensive new peak power plants and a necessity for distribution/transmission upgrades and new peak power plants, which are generally costly.
Transportation electrification, the energy efficiency of the buildings and the use of renewable and clean energy resources lead to environmental benefits. Furthermore, it is assumed that the interconnection of smart communities at a larger scale is likely to replace fossil fuel-sourced power with clean electrons or to eliminate current power plants. It is necessary to include both the supply and demand sides when evaluating the environmental impacts of smart transformations can be. The demand side benefits are owing to consumer behavior and energy efficiency (e.g., reduction in particles and air pollution) and the supply-side benefits occur because of the increased use of green energies.
Moreover, owing to the service quality and responsiveness of the system, smart communities also improve social and human dimensions. To illustrate, DERs can prevent power outages by evaluated the value of load loss. However, it should be noted that resiliency metrics vary in value depending on the type of the applications (e.g., critical facilities). In addition, with proactive strategies aimed at failure avoidance and data-driven prognosis, it is possible to considerably enhance asset maintaining using asset condition monitoring. Consequently, it enables significant cost savings. Using sensors and automation systems to improve measures such as life quality, productivity, and health, a more comfortable environment is created for building occupants. Reliable EV fast chargers and smart traffic controls lead to improved experience and service quality. The human factor should be included in control loops and decision-making to account for preferences and habits. Smart communities can also contribute to the generation of a new supply chain system to advance further business development and job creation. Smart transformation is also likely to result in additional human involvement. It is also recommended to use beneficiary perspectives such as multi-criteria analysis (MCA), social cost-benefit analysis (SCBA), and private cost-benefit analysis (PCBA) to assess CBA.
PCBA takes into account the costs and benefits from alternatives imposed on or accrued to private sectors (e.g., companies or individuals). More precisely, it takes into consideration the transfer payments such as taxes and subsidies that private entities pay to the administration or receive from it. The financial appraisal is the term used to refer to this variant of CBA. In SCBA, environmental impacts can be assessed or omitted.
SCBA deals with the costs and benefits accrued to society in general. Social costs and benefits are typically not the same as private ones due to current market imperfections, such as government intervention in the market (e.g., price regulations, subsidies, and taxes, imperfect competition in the market (e.g., monopoly power), and externalities and public goods. Thus, MCA represents a decision support method for assessing and comparing different alternatives such as concrete cases of applied instruments or different policy options. To compare the alternatives, it is necessary to use the performance of a selected set of evaluation criteria in the form of a consequence table or performance matrix. In the matrix, each row shows the performance of the alternative against each criterion, whereas each column represents an alternative (case). MCA begins with defining the aims, the decision-makers, and the other stakeholders. In the second step, alternatives are identified, whereas criteria are defined in the third step. In the fourth step, the performance of each alternative is described against the criteria in the performance matrix, and the score matrix is determined. In the fifth step, each criterion receives weight to set the relative importance. The overall values are calculated in the sixth step, whereas the results are analyzed in the final step.
3.3.6.2 Life Cycle Assessment
Smart developments in transportation, resource management, and energy will lead to reducing the damage to the environment. Nevertheless, it is important to note that indirect damage to the environment might increase during the production and implementation stage of smart solutions. Therefore, it is necessary to consider the indirect environmental impact in the smart transformation process. Thus, LCA is an instrument aimed at determining the trade between the ecosphere and the technosphere during the life cycle phase of a system by assessing possible environmental effects and resources allocated during the life cycle (e.g., raw materials, extraction, waste treatment) of the product or service. It is a trustworthy and reliable, reproducible method based on user guidelines published by the European Commission and the ISO standardization (ISO 2006a and ISO 2006b). LCA comprises four stages according to the ISO 14040:2006 guidelines: 1) definition of the goals and scope of the assessment, 2) inventory analysis, 3) impact assessment, and 4) interpretation of the life cycle. The purpose of LCA is to determine the best available life cycles with minimum adverse environmental impacts to choose significant indicants of an organization’s environmental behavior and to assist decision-makers with strategic planning and system design.
3.3.6.3 Investment Planning and Economic Feasibility
Following the determination of direct and indirect benefits and cash flows of the transition project, it is possible to implement different capital budgeting approaches to assess the financial viability of the plan. It is recommended to incorporate risk factors and adopt a real-options approach and incorporating risk factors during the investment and planning stages of the project. The project planning at this stage should involve sensitivity analysis, scenario development, cost estimations, and the identification of feasible alternatives. If there is no cost-effective alternative, government incentives and available technologies and government can lead to viable portfolios.
4 Discussion
As outlined previously, this study was aimed at addressing the gaps in knowledge regarding the integration of the multi-dimensional domain knowledge of this transition. The purpose was to create a meaningful roadmap. The objective was to elaborate on human, technological, economic, policy, and environmental factors and to propose a multistage and multidisciplinary transition plan that will incorporate all aforementioned factors into the transformation. More precisely, it was necessary to address non-technical aspects of the transformation. Accordingly, the proposed connected network will allow optimal management to expand sustainability and efficiency. We introduced innovative concepts needed to achieve the transition such as community energy and zero-carbon communities and microgrids and nanogrids and defined their essential components. Subsequently, we addressed the role of the social factors in smart transformation and highlighted the necessity to incorporate them in both vertical and horizontal processes. We precisely identified different factors to be included in the transition plan. More specifically, demographics, psychological drivers (primarily personal and social norms), and cultural barriers all critically impact the transition. In addition, structural factors and financial policy factors are intertwined with more individual factors.
Subsequently, we proposed a smart transformation roadmap. This smart transformation roadmap consists of a strategic framework and multiple decision-making stages to establish a smart transformation plan include preliminary assessment (identification of capacities/benefits, site inspections, environment feasibility, social-behavioral studies, and identification of impediments/challenges), policy assessment (regulatory incentives/rebates, setting of objectives and high-level targets), requirement mapping (technology and infrastructure, planning and information processing, and human intervention), virtual prototyping and CPS (modeling and simulation, digital twins, virtual testbeds, real data acquisition, and physical prototyping, and technical assessment), and economic analysis (CBA, LCA, and investment planning), explained in detail in the manuscript.
The structural approach to the energy transition is highly required considering the complexity of connected communities. More precisely, because energy transition is highly complex, it is not possible to explain it or to model it using a small number of factors or focusing only on technical aspects. Accordingly, it is necessary, as shown in the paper, to devise energy system models using the aforementioned energy system modeling tools. Only using the structural approach, it is possible to ensure the long-term sustainability of societies that were previously highly dependent on fossil fuels.
Furthermore, the structural approach overcomes the limitations of the development of a single model. Single models are typically based on distinctive methodologies and assumptions. Thus, simply linking different models is not recommended. Thus, in this study, we discussed AI applications and data-driven models that serve to support system monitoring and predictive planning, carry out classifications and clustering, determine anomalies, and predict trends. As highlighted in the study, the actual representation of a system’s dynamics and system’s component models allow the development of a virtual prototype for decision-makers to carry out technical evaluations of various technology combinations. The structural approach regards the energy transition of communities as multi-stakeholder processes.
5 Conclusion
The study showed that smart communities can significantly contribute to achieving economic, social, and environmental goals. In addition, we proposed a decision-making framework for the transition of current communities into smart energy systems. Drawing upon the concept from different disciplines, this framework includes economic analysis, physical and virtual prototyping, technology and technological feasibility assessment, objective definitions, policy evaluation, and preliminary assessment of the project. Furthermore, each phase of the planning process must incorporate feedbacks and multiple iterations to make the transition smooth using the full capabilities of the community. Such capacities should be enhanced owing to revolutionary technological changes using automation, ESSs, renewable energy resources, computing power, novel AI solutions, big data analysis, and ICT. Moreover, prompt technological changes require regular revision and updating of logistics, tools, and goals during the lifecycle of the smart community project. For instance, novel IoT architectures play a vital role in facilitating and accelerating the data transmission and share in the community. Consequently, this enables prompt response decision-making and system monitoring. In addition, enormous amounts of data can be managed efficiently using database management system platforms and big data analysis. AI solutions based on increased data sources with higher resolutions lead to be more reliable and precise predictive modeling. Moreover, it can result in comprehensive planning schemes comprising all participating nodes in the system. Technological efficiency is related to the increased productivity of on-site and clean power generation and storage systems. The development of technology will also reduce error rates of controllers, measurement tools, and sensor devices. Furthermore, we assume additional integration of technologies owing to innovative universal gateways and protocols. More precisely, this allows smart communities to obtain signals from their surroundings and combine these inputs with real-time information within the network. The operation of the municipalities based on this information will lead to a sustainable environment enhanced life quality, smart economy, smart society, and smart governance. In the future, we plan to investigate how to allocate efficiently resources and budgeting for improving the capacities of the smart communities. Moreover, it is necessary to validate the proposed framework and tools via a real-world case study.
Data Availability Statement
The original contributions presented in the study are included in the article/Supplementary Material, further inquiries can be directed to the corresponding author.
Author Contributions
EZ, AG, AA, and MJ contributed to the design of the research, to the analysis of the results and to the writing of the manuscript, with EZ leading this research project.
Funding
This publication was made possible by an NPRP awards (NPRP11S-1228-170,142 and NPRP13S-0206-200272) from the Qatar National Research Fund (a member of the Qatar Foundation). The statements made herein are the sole responsibility of the authors. The publication of this article was funded by the Qatar National Library.
Conflict of Interest
The authors declare that the research was conducted in the absence of any commercial or financial relationships that could be construed as a potential conflict of interest.
Publisher’s Note
All claims expressed in this article are solely those of the authors and do not necessarily represent those of their affiliated organizations, or those of the publisher, the editors and the reviewers. Any product that may be evaluated in this article, or claim that may be made by its manufacturer, is not guaranteed or endorsed by the publisher.
References
Abu-Elzait, S., and Parkin, R. (2019). “Economic and Environmental Advantages of Renewable-Based Microgrids over Conventional Microgrids,” in 2019 IEEE Green Technologies Conference(GreenTech), 1–4. doi:10.1109/GreenTech.2019.8767146
Abulibdeh, A. (2020). Can COVID ‐19 Mitigation Measures Promote Telework Practices? J. Labor Soc. 23 (4), 551–576. doi:10.1111/wusa.12498
Abulibdeh, A. (2021). Modeling Electricity Consumption Patterns during the COVID-19 Pandemic across Six Socioeconomic Sectors in the State of Qatar. Energy Strateg. Rev. 38, 100733. doi:10.1016/J.ESR.2021.100733
Abulibdeh, A. (2020). Planning for Congestion Pricing Policies in the Middle East: Public Acceptability and Revenue Distribution. Transp. Lett. doi:10.1080/19427867.2020.1857908
Abulibdeh, A. (2021). Spatiotemporal Analysis of Water-Electricity Consumption in the Context of the COVID-19 Pandemic across Six Socioeconomic Sectors in Doha City, Qatar. Appl. Energ. 304, 117864. doi:10.1016/J.APENERGY.2021.117864
Abulibdeh, A., and Zaidan, E. (2018). Analysis of Factors Affecting Willingness to Pay for High-Occupancy-Toll Lanes: Results from Stated-Preference Survey of Travelers. J. Transp. Geogr. 66, 91–105. doi:10.1016/j.jtrangeo.2017.11.015
Albert, S., Flournoy, D., and LeBrasseur, R. (2009). Networked Communities: Strategies for Digital collaborationNo Title. New York: Information Science Reference, Hershey.
Allcott, H. (2011). Social Norms and Energy Conservation. J. Public Econ. 95 (9), 1082–1095. doi:10.1016/j.jpubeco.2011.03.003
Aluko, O. E., Onibonoje, M. O., and Dada, J. O. (2020). A Review of the Control System Roles in Integrating Renewable Energy into the National Grid. 2020 IEEE PES/IAS PowerAfrica, PowerAfrica. doi:10.1109/POWERAFRICA49420.2020.9219971
Antonysamy, S., Devi, S., Murugasan, D., and Simon, E. S. (2020). Future Nano-Grid Technologies and its Implementation Challenges for Smart Cities. IOP Conf. Ser. Mater. Sci. Eng. 955 (1), 012002. doi:10.1088/1757-899X/955/1/012002
Barr, S., Gilg, A. W., and Ford, N. (2005). The Household Energy gap: Examining the divide between Habitual- and purchase-related Conservation Behaviours. Energy Policy 33 (11), 1425–1444. doi:10.1016/j.enpol.2003.12.016
Barthel, S., and Isendahl, C. (2013). Urban Gardens, Agriculture, and Water Management: Sources of Resilience for Long-Term Food Security in Cities. Ecol. Econ. 86, 224–234. doi:10.1016/j.ecolecon.2012.06.018
Bencardino, M., and Greco, I. (2014). Smart Communities. Social Innovation at the Service of the Smart Cities. Tema - J. L. Use, Mobil. Environ. doi:10.6092/1970-9870/2533
Berka, A. L., and Creamer, E. (2018). Taking Stock of the Local Impacts of Community Owned Renewable Energy: A Review and Research Agenda. Renew. Sustain. Energ. Rev. 82, 3400–3419. doi:10.1016/j.rser.2017.10.050
Berry, C. R., and Glaeser, E. L. (2005). The Divergence of Human Capital Levels across Cities. Natl. Bur. Econ. Res. Work. Pap. Ser. 11617. doi:10.3386/w11617
Ceglia, F., Esposito, P., Marrasso, E., and Sasso, M. (2020). From Smart Energy Community to Smart Energy Municipalities: Literature Review, Agendas and Pathways. J. Clean. Prod. 254, 120118. doi:10.1016/J.JCLEPRO.2020.120118
CFG Canadian Federal Government (2002). Fostering Innovation and Use. Industry Canada. Availableat: http://broadband.gc.ca/Broadband-document/english/chapter5.htm.
Chamoso, P., and De La Prieta, F. (2016). Smart Cities Simulation Environment for Intelligent Algorithms Evaluation. ADCAIJ Adv. Distrib. Comput. Artif. Intell. J. 4, 87. doi:10.14201/ADCAIJ2015438796
Chang, D. L., Sabatini-Marques, J., da Costa, E. M., Selig, P. M., and Yigitcanlar, T. (2018). Knowledge-based, Smart and Sustainable Cities: a Provocation for a Conceptual Framework. J. Open Innov. Technol. Mark. Complex. 414 (1), 1–17. doi:10.1186/S40852-018-0087-2
Chen, C., Xu, X., and Day, J. K. (2017). Thermal comfort or Money Saving? Exploring Intentions to Conserve Energy Among Low-Income Households in the United States. Energy Res. Soc. Sci. 26, 61–71. doi:10.1016/j.erss.2017.01.009
Chen, C., Xu, X., and Frey, S. (2016). Who Wants Solar Water Heaters and Alternative Fuel Vehicles? Assessing Social–Psychological Predictors of Adoption Intention and Policy Support in China. Energ. Res. Soc. Sci. 15, 1–11. doi:10.1016/j.erss.2016.02.006
Cheng, X., Long, R., Chen, H., and Yang, J. (2019). Does Social Interaction Have an Impact on Residents’ Sustainable Lifestyle Decisions? A Multi-Agent Stimulation Based on Regret and Game Theory. Appl. Energ. 251, 113366. doi:10.1016/j.apenergy.2019.113366
Climate Change Performance Index 2022: Background and Methodology | Climate Change Performance Index’. 2022.
Cocchia, A. (2014). “Smart and Digital City: A Systematic Literature Review BT - Smart City,” in How to Create Public and Economic Value with High Technology in Urban Space. Editors R. P. Dameri,, and C. Rosenthal-Sabroux (Cham: Springer International Publishing), 13–43. doi:10.1007/978-3-319-06160-3_2
Coe, A., Paquet, G., and Roy, J. (2001). E-governance and Smart Communities: A Social Learning Challenge. Soc. Sci. Comput. Rev. - SOC. SCI. Comput. REV. 19, 80–93. doi:10.1177/089443930101900107
Contreras, S. F., Cortes, C. A., and Myrzik, J. M. A. (2019). Optimal Microgrid Planning for Enhancing Ancillary Service Provision. J. Mod. Power Syst. Clean. Energ. 7 (4), 862–875. doi:10.1007/s40565-019-0528-3
Davis, M., Ahiduzzaman, M., and Kumar, A. (2018). How Will Canada’s Greenhouse Gas Emissions Change by 2050? A Disaggregated Analysis of Past and Future Greenhouse Gas Emissions Using Bottom-Up Energy Modelling and Sankey Diagrams. Appl. Energ. 220, 754–786. doi:10.1016/J.APENERGY.2018.03.064
De Paz, J. F., Bajo, J., Rodríguez, S., Villarrubia, G., and Corchado, J. M. (2016). Intelligent System for Lighting Control in Smart Cities. Inf. Sci. (Ny) 372, 241–255. doi:10.1016/j.ins.2016.08.045
De Silva, D. G., and Pownall, R. A. J. (2014). Going green: Does it Depend on Education, Gender or Income? Appl. Econ. 46 (5), 573–586. doi:10.1080/00036846.2013.857003
Devine-Wright, P., and Wiersma, B. (2013). Opening up the “Local” to Analysis: Exploring the Spatiality of UK Urban Decentralised Energy Initiatives. Local Environ. 18 (10), 1099–1116. doi:10.1080/13549839.2012.754742
Eger, J. (2009). Smart Growth, Smart Cities, and the Crisis at the Pump A Worldwide Phenomenon. I-ways - J. E-government Pol. Regul. 32, 47–53. doi:10.3233/iwa-2009-0164
Elessawy, F., and Zaidan, E. (2014). Living in the Move: Impact of Guest Workers on Population Characteristics of the United Arab Emirates (UAE). Arab World Geogr. 17 (1), 2–23. doi:10.5555/ARWG.17.1.04502312V8G83U76
Ghofrani, A., Zaidan, E., and Abulibdeh, A. (2021). Simulation and Impact Analysis of Behavioral and Socioeconomic Dimensions of Energy Consumption. Energy, 122502. doi:10.1016/J.ENERGY.2021.122502
Giffinger, R., Fertner, C., Meijers, E., and Kramar, H. (2007). City-ranking of European Medium-Sized Cities. Eur. Smart Cities.
Gomes, L., Faria, P., Morais, H., Vale, Z., and Ramos, C. (2014). Distributed, Agent-Based Intelligent System for Demand Response Program Simulation in Smart Grids. IEEE Intell. Syst. 29 (1), 56–65. doi:10.1109/MIS.2013.2
Gondokusuma, M. I. C., Kitagawa, Y., and Shimoda, Y. (2019). Smart Community Guideline: Case Study on the Development Process of Smart Communities in Japan. IOP Conf. Ser. Earth Environ. Sci. 294 (1), 012017. doi:10.1088/1755-1315/294/1/012017
González-Briones, A., Prieto, J., De La Prieta, F., Herrera-Viedma, E., and Corchado, J. M. (2018). Energy Optimization Using a Case-Based Reasoning Strategy. Sensors (Basel). 18 (3), 865. doi:10.3390/s18030865
Groves, C., Munday, M., and Yakovleva, N. (2013). Fighting the Pipe: Neo-liberal Governance and Barriers to Effective Community Participation in Energy Infrastructure Planning. Environ. Plan. C Gov. Pol., 340–356. doi:10.1068/c11331r
Han, H., JHsu, L.-T., and Sheu, C. (2010). Application of the Theory of Planned Behavior to green Hotel Choice: Testing the Effect of Environmental Friendly Activities. Tour. Manag. 31 (3), 325–334. doi:10.1016/j.tourman.2009.03.013
Hancke, G., and Silva, B. (2012). The Role of Advanced Sensing in Smart Cities. Sensors (Basel). 13, 393–425. doi:10.3390/s130100393
Harrison, C., and Donnelly, I. A. (2011). “A Theory of Smart Cities,” in Proc. 55th Annu. Meet. ISSS - 2011, Hull, UK.55.
Harrison, C. (2010). Foundations for Smarter Cities, IBM J. Res. Dev., 54, 1–16. doi:10.1147/JRD.2010.2048257
Hoffman, S., Fudge, S., Pawlisch, L., High-Pippert, A., Peters, M., and Haskard, J. (2013). Public Values and Community Energy: Lessons from the US and UK. Sustainability 5, 1747–1763. doi:10.3390/su5041747
Hoffman, S. M., and High-Pippert, A. (2005). Community Energy: A Social Architecture for an Alternative Energy Future. Bull. Sci. Technol. Soc. 25 (5), 387–401. doi:10.1177/0270467605278880
Huijts, N. M. A., Molin, E. J. E., and Steg, L. (2012). Psychological Factors Influencing Sustainable Energy Technology Acceptance: A Review-Based Comprehensive Framework. Renew. Sustain. Energ. Rev. 16 (1), 525–531. doi:10.1016/j.rser.2011.08.018
Israilidis, J., Odusanya, K., and Mazhar, M. U. (2021). Exploring Knowledge Management Perspectives in Smart City Research: A Review and Future Research Agenda. Int. J. Inf. Manage. 56, 101989. doi:10.1016/J.IJINFOMGT.2019.07.015
Jafari, M. A., Zaidan, E., Ghofrani, A., Mahani, K., and Farzan, F. (2020). Improving Building Energy Footprint and Asset Performance Using Digital Twin Technology. IFAC-PapersOnLine 53 (3), 386–391. doi:10.1016/J.IFACOL.2020.11.062
Jan, B., Uhlich, T., Bals, C., Höhne, N., and Nascimento, L. (2022). RESULTS - Climate Change Performance Index.
Jin, X. (2015). “Analysis of Microgrid Comprehensive Benefits and Evaluation of its Economy,” in 10th International Conference on Advances in Power System Control, Operation & Management (Hong Kong, China: APSCOM 2015), 1–4. doi:10.1049/ic.2015.0279
Joshi, S., Saxena, S., Godbole, T., and Shreya, (2016). Developing Smart Cities: An Integrated Framework. Proced. Comput. Sci. 93, 902–909. doi:10.1016/J.PROCS.2016.07.258
Karki, R. S., and Chanana, S. (2016). “Simulation of Energy Management System for Local Energy Market in Microgrids,” in 2016 IEEE Students’ Conference on Electrical, Electronics and Computer Science (SCEECS), 1–6. doi:10.1109/SCEECS.2016.7509334
Kim, H., Choi, H., Kang, H., An, J., Yeom, S., and Hong, T. (2021). A Systematic Review of the Smart Energy Conservation System: From Smart Homes to Sustainable Smart Cities. Renew. Sustain. Energ. Rev. 140, 110755. doi:10.1016/J.RSER.2021.110755
Kingston, R., Cauvain, J., and Viitanen, nee. (2015). Smart Cities and green Growth: Outsourcing Democratic and Environmental Resilience to the Global Technology Sector. Environ. Plan. A. 46, 803–819. doi:10.1068/a46242
Komninos, N. (2009). Intelligent Cities: Towards Interactive and Global Innovation Environments. Int. J. Innov. Reg. Dev. - Int. J. Innov. Reg. Dev. 1 (Jan). doi:10.1504/IJIRD.2009.022726
Li, W., Long, R., and Chen, H. (2016). Consumers’ Evaluation of National New Energy Vehicle Policy in China: An Analysis Based on a Four Paradigm Model. Energy Policy 99, 33–41. doi:10.1016/j.enpol.2016.09.050
Long, J. E. (1993). An Econometric Analysis of Residential Expenditures on Energy Conservation and Renewable Energy Sources. Energy Econ 15 (4), 232–238. doi:10.1016/0140-9883(93)90012-G
MacArthur, J. (2017). Trade, Tarsands and Treaties: The Political Economy Context of Community Energy in Canada. Sustainability 9, 464. doi:10.3390/su9030464
Madushan, A., and Ralalage, D. (2020). Optimal Energy Management and Control of Microgrids in Modern Electrical Power Systems. Availableat: https://hdl.handle.net/11244/325497.
Michelsen, C. C., and Madlener, R. (2012). Homeowners’ Preferences for Adopting Innovative Residential Heating Systems: A Discrete Choice Analysis for Germany. Energ. Econ 34 (5), 1271–1283. doi:10.1016/j.eneco.2012.06.009
Mills, B. F., and Schleich, J. (2009). Profits or Preferences? Assessing the Adoption of Residential Solar thermal Technologies. Energy Policy 37 (10), 4145–4154. doi:10.1016/j.enpol.2009.05.014
Mills, B., and Schleich, J. (2012). Residential Energy-Efficient Technology Adoption, Energy Conservation, Knowledge, and Attitudes: An Analysis of European Countries. Energy Policy 49, 616–628. doi:10.1016/j.enpol.2012.07.008
Nair, G., Gustavsson, L., and Mahapatra, K. (2010). Factors Influencing Energy Efficiency Investments in Existing Swedish Residential Buildings. Energy Policy 38 (6), 2956–2963. doi:10.1016/j.enpol.2010.01.033
Niamir, L., Filatova, T., Voinov, A., and Bressers, H., (2018). ‘Transition to Low-Carbon Economy: Assessing Cumulative Impacts of Individual Behavioral Changes’, Energy Policy, 118, 325–345. doi:10.1016/j.enpol.2018.03.045
Niamir, L., Ivanova, O., Filatova, T., Voinov, A., and Bressers, H. (2020). Demand-side Solutions for Climate Mitigation: Bottom-Up Drivers of Household Energy Behavior Change in the Netherlands and Spain. Energ. Res. Soc. Sci. 62, 101356. doi:10.1016/j.erss.2019.101356
Nordman, B. (2009). “Nanogrids: Evolving Our Electricity Systems from the Bottom up,” in Darnell Green Power Forum.
OECD (2022). Denmark: Focus on Climate Policy and Labour Market Inclusion for a strong and Sustainable Recovery, Says OECD.
Ortiz, L., González, J. W., Gutierrez, L. B., and Llanes-Santiago, O. (2020). A Review on Control and Fault-Tolerant Control Systems of AC/DC Microgrids. Heliyon 6 (8), e04799. doi:10.1016/J.HELIYON.2020.E04799
Ortmeyer, T., Wu, L., and Li, J. (2016). “Planning and Design Goals for Resilient Microgrids,” in 2016 IEEE Power & Energy Society Innovative Smart Grid Technologies Conference (ISGT), 1–5. doi:10.1109/ISGT.2016.7781248
Perera, C., Zaslavsky, A., Christen, P., and Georgakopoulos, D. (2014). Sensing as a Service Model for Smart Cities Supported by Internet of Things. Eur. Trans. Telecommun. doi:10.1002/ett.2704
Priya Dharshini, K., Gopalakrishnan, D., Shankar, C. K., and Ramya, R. (2022). A Survey on IoT Applications in Smart Cities. EAI/Springer Innov. Commun. Comput., 179–204. doi:10.1007/978-3-030-66607-1_9
Rogers, J. C., Simmons, E. A., Convery, I., and Weatherall, A. (2008). Public Perceptions of Opportunities for Community-Based Renewable Energy Projects. Energy Policy 36 (11), 4217–4226. doi:10.1016/J.ENPOL.2008.07.028
Sardianou, E., and Genoudi, P. (2013). Which Factors Affect the Willingness of Consumers to Adopt Renewable Energies? Renew. Energ. 57, 1–4. doi:10.1016/j.renene.2013.01.031
Schmid Mast, M., Sieverding, M., Esslen, M., Graber, K., and Jäncke, L. (2008). Masculinity Causes Speeding in Young Men. Accid. Anal. Prev. 40 (2), 840–842. doi:10.1016/J.AAP.2007.09.028
Shahsavari, A., and Akbari, M. (2018). Potential of Solar Energy in Developing Countries for Reducing Energy-Related Emissions. Renew. Sustain. Energ. Rev. 90, 275–291. doi:10.1016/J.RSER.2018.03.065
Sidiras, D. K., and Koukios, E. G. (2004). Solar Systems Diffusion in Local Markets. Energy Policy 32 (18), 2007–2018. doi:10.1016/S0301-4215(03)00173-3
Sousa, T., Morais, H., Soares, J., and Vale, Z. (2012). Day-ahead Resource Scheduling in Smart Grids Considering Vehicle-To-Grid and Network Constraints. Appl. Energ. 96, 183–193. doi:10.1016/j.apenergy.2012.01.053
Sovacool, B. K., and Griffiths, S. (2020). The Cultural Barriers to a Low-Carbon Future: A Review of Six Mobility and Energy Transitions across 28 Countries. Renew. Sustain. Energ. Rev. 119, 109569. doi:10.1016/j.rser.2019.109569
Sun, Y., Xia, Y., Song, H., and Bie, R. (2014). “Internet of Things Services for Small Towns,” in 2014 International Conference on Identification, Information and Knowledge in the Internet of Things, 92–95. doi:10.1109/IIKI.2014.27
Susanti, R., Soetomo, S., Buchori, I., and Brotosunaryo, P. M. (2016). Smart Growth, Smart City and Density: In Search of the Appropriate Indicator for Residential Density in Indonesia. Proced. - Soc. Behav. Sci. 227, 194–201. doi:10.1016/j.sbspro.2016.06.062
Ton, D. T., and Smith, M. A. (2012). The U.S. Department of Energy’s Microgrid Initiative. Electr. J. 25 (8), 84–94. doi:10.1016/j.tej.2012.09.013
van der Werff, E., Taufik, D., and Venhoeven, L. (2019). Pull the Plug: How Private Commitment Strategies Can Strengthen Personal Norms and Promote Energy-Saving in the Netherlands. Energ. Res. Soc. Sci. 54, 26–33. doi:10.1016/j.erss.2019.03.002
Walker, G., and Devine-Wright, P. (2008). Community Renewable Energy: What Should it Mean? Energy Policy 36 (2), 497–500. doi:10.1016/j.enpol.2007.10.019
Wang, Z., Zhang, B., Yin, J., and Zhang, Y. (2011). Determinants and Policy Implications for Household Electricity-Saving Behaviour: Evidence from Beijing, China. Energy Policy 39 (6), 3550–3557. doi:10.1016/j.enpol.2011.03.055
Wyse, S., and Hoicka, C. (2019). ‘“By and for Local People”: Assessing the Connection between Local Energy Plans and Community Energy’. Local Environ., 1–18. doi:10.1080/13549839.2019.1652802
Yesiloglu, S., Lapacz, A., and Miladinova, Y. (2019). Human Values and newsâ€TM Impact on Climate Change Beliefs: A Comparative Study on Millennials in Sweden and Russia. J. Promot. Commun. 7 (2).
Yigitcanlar, T., Kamruzzaman, M., Buysb, L., Ioppoloc, J., Sabatini-Marquesd, J., Yune, J., et al. (2018). Understanding “Smart Cities”: Intertwining Development Drivers with Desired Outcomes in a Multidimensional Framework. Cities 81, 145–160. doi:10.1016/J.CITIES.2018.04.003
Zaidan, E., and Abulibdeh, A. (2020). Master Planning and the Evolving Urban Model in the Gulf Cities: Principles, Policies, and Practices for the Transition to Sustainable Urbanism. Plan. Pract. Res. doi:10.1080/02697459.2020.1829278
Zelezny, L. C., Chua, P.-P., and Aldrich, C. (2000). Elaborating on Gender Differences in Environmentalism. J. Soc. Issues 56 (3), 443–457. doi:10.1111/0022-4537.00177
Zhang, X., Platten, A., and Shen, L. (2011). Green Property Development Practice in China: Costs and Barriers. Build. Environ. 46 (11), 2153–2160. doi:10.1016/j.buildenv.2011.04.031
Zheng, C., Yuan, J., Zhu, L., Zhang, Y., and Shao, Q. (2020). From Digital to Sustainable: A Scientometric Review of Smart City Literature between 1990 and 2019. J. Clean. Prod. 258, 120689. doi:10.1016/j.jclepro.2020.120689
Keywords: smart cites, community synergy, zero-carbon transition, strategic planning, smart communities, decision-making
Citation: Zaidan E, Ghofrani A, Abulibdeh A and Jafari M (2022) Accelerating the Change to Smart Societies- a Strategic Knowledge-Based Framework for Smart Energy Transition of Urban Communities. Front. Energy Res. 10:852092. doi: 10.3389/fenrg.2022.852092
Received: 10 January 2022; Accepted: 02 February 2022;
Published: 02 March 2022.
Edited by:
Michal Jasinski, Wrocław University of Science and Technology, PolandReviewed by:
İrfan Kalaycı, İnönü University, TurkeyHasim Altan, Arkin University of Creative Arts and Design (ARUCAD), Cyprus
Hannah Jacobs Wiseman, The Pennsylvania State University, United States
Copyright © 2022 Zaidan, Ghofrani, Abulibdeh and Jafari. This is an open-access article distributed under the terms of the Creative Commons Attribution License (CC BY). The use, distribution or reproduction in other forums is permitted, provided the original author(s) and the copyright owner(s) are credited and that the original publication in this journal is cited, in accordance with accepted academic practice. No use, distribution or reproduction is permitted which does not comply with these terms.
*Correspondence: Esmat Zaidan, ZXphaWRhbkBxdS5lZHUucWE=