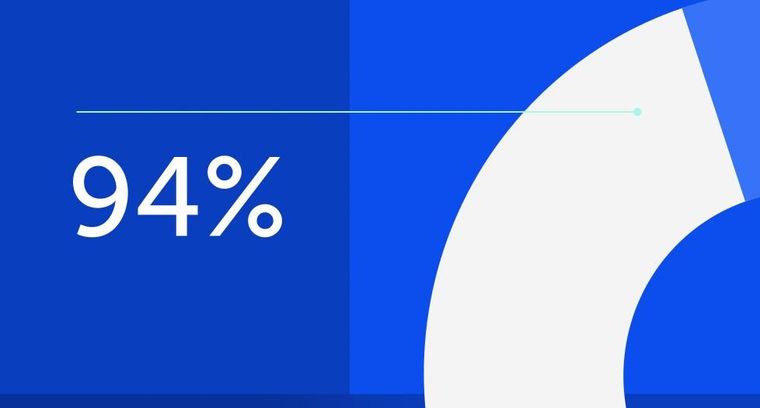
94% of researchers rate our articles as excellent or good
Learn more about the work of our research integrity team to safeguard the quality of each article we publish.
Find out more
REVIEW article
Front. Energy Res., 26 May 2022
Sec. Solar Energy
Volume 10 - 2022 | https://doi.org/10.3389/fenrg.2022.828097
This article is part of the Research TopicForecasting Solar Radiation, Photovoltaic Power and Thermal Energy Production. Applications.View all 9 articles
The measurement of solar radiation and its forecasting at any particular location is a difficult task as it depends on various input parameters. So, intelligent modeling approaches with advanced techniques are always necessary for this challenging activity. Adaptive neuro-fuzzy inference system (
With the rapid increase in global energy demands and depleting fossil fuel reserves, the world is opting for renewable sources of energy. Non-conventional energy sources (Notton et al., 2002) play a great role in mitigating power necessity and have become promising alternatives for the consumers. Among all such energy sources, solar energy plays a leading role because of its widespread availability. Prediction or forecasting of solar energy (Xue, 2017; Almaraashi, 2018a) is extremely important and has to be carried out before the selection of any site for a solar-based power plant. Solar forecasting analysis is necessary for the design and modeling of the solar conversion system. The collection of solar radiation data at a particular location is made possible with the use of designated measuring instruments. Many models have been developed related to the global solar radiation using parameters such as relative humidity, duration of the sunshine, temperature, latitude, and longitude. Basically, it is difficult to deal with systems having uncertain features through conventional mathematical tools and hence advanced controllers are needed to deal with the uncertainties.
Many literature works have repeatedly utilized ANN, fuzzy, and ANFIS-based algorithms to estimate solar radiation forecasting for various applications based on numerous meteorological parameters and outputs. Fuzzy rule–based systems utilize linguistic variables such as the
Many places in India are prone to natural calamities. The four eastern coastal states, West Bengal, Odisha, Andhra Pradesh, and Tamil Nadu, and one western state Gujarat are susceptible to cyclonic events. Solar radiations in these particular localities are more or less haphazard. So for finalizing any project based on renewable energy such as SPV or STWM (solar thermal wind machine), solar data collection becomes crucial. Generally, traditional methodologies are practiced to forecast the solar irradiation in major Indian cities. Moreover, less measuring equipment is utilized in coastal regions due to high wind effects. Some climatic parameters are needed to develop and estimate the global diffuse solar radiation. Several literature works are found describing the use of ANFIS models for many applications. The forecasting of measles cases has been described (Uyar et al., 2019) and greenhouse gas prediction has been mentioned in the article (Ludwig, 2019). In this study (Nguyen and Liao, 2011; Motepe et al., 2018), the author has applied ANFIS for load forecasting to get accurate results. Authors have also discussed its application in the prediction of electricity including forecasting of several renewable energy sources such as PV, wind, and fuel cell (Notton et al., 2002; Gairaa et al., 2016; Singh and Rizwan, 2018a; Yadav et al., 2018a; Campos et al., 2018; Ilmi et al., 2018; Karri et al., 2018; Maitra et al., 2018; Yousefi et al., 2018; Sujil et al., 2019a; Fachini and Lopes, 2019; Perveen et al., 2019; Pourdaryaei et al., 2019).
The proposed review work is arranged in the following manner. Section 2 presents the material and methodology used for the prediction of solar radiation. It also discusses the implementation of intelligent modeling technique such as ANFIS for solar energy forecasting in Eastern Indian cities. Section 3 presents the simulation and modeling of a standalone solar system with ANFIS. Section 4 presents results and discussions. The conclusion has been carried out in Section 5 and Section 6 presents the references.
A total of 6 years data [Appendix], monthly average value of temperature, humidity, and sunshine duration are obtained from the solar radiation handbook and NREL (National renewable energy laboratory) are used for training and the remaining 1 year is used for testing. Recent datasets of 2015–17 have also been prepared with the help of IMD Bhubaneswar center for further studies and experimentation.
As we know that solar energy is unpredictable and uncertain, there is an urgent need to mitigate the uncertain nature of solar radiation. Conventional methods fail to predict the solar irradiation properly because of the uncertain behavior of Sun. So soft computing happens to be an innovative approach with an ability of a human mind. The application of various soft computing tools such as multi-layer perceptron (MLP), ANFIS, RBF, RNN, NARX, GNN, FL, FG, NFG, NG, and SVM are suitably employed to predict and estimate the solar irradiance.
An adaptive neuro-fuzzy inference system has been used to predict the daily global solar radiation of the eastern zone of India. The data on daily solar radiation, sunshine duration, humidity, and temperature for the period of 5 years are collected from the renewable energy source laboratory, NASA, and the solar radiation handbook. A total of 2,190 day (2000–2005) datasets are used in the
The typical structure of
FIGURE 1. (A) ANFIS structure. (B) Detailed
The ANN network is depicted in Figures 1A,B. Possessing lots of nodes joined through the directional linking. In order to minimize the error, couple of basic learning rule–based method has been used in the network such as back-propagation technique. A fuzzy model having rules is as follows:
where
The model (as shown in Figures 2, 3) uses six dissimilar membership type function such as (Gauss mf, triang mf, two side Gaussian mf, Bell mf, Difsig mf, and Trap mf) along with (i.e., linear &constant) membership function. The dataflow has been explained from Eqs 3–8).
Layer 1. Every node i in this layer is an adaptive node with a node function.
Y or X = input node I.
Ai or Bi-2 = linguistic value.
O1, i = membership grade.
Membership grade satisfies the quantifier A.
Layer no 2: Every node in the layer is fixed, where the output symbolizes product of all input signals.
The node output indicates the firing strength. Any other T-norm which performs fuzzy is used as a node function. Layer no three is fixed and is labeled as N. Furthermore, the ith node decides about the ith rule’s firing capacity for the sum of all the rule’s firing capacity.
Layer 4. Every node i in this layer is an adaptive node with function.
where
Layer no 5. Here, in this case; a single node is a fixed node and is given as Σ. This calculates the overall output as sum of all the incoming signals.
Based on the geographical coordinates and following meteorological parameters such as relative humidity and sunshine duration, the isolated places of Nigeria (Ojosu and Komolafe, 1987; Ododo et al., 1995) are studied for forecasting daily global solar radiation using (
Yacef et al. (2012) prepare a comparative study between Bayesian neural network (BNN), classical neural network (CNN), and empirical models for estimating the daily global solar irradiation (DGSR) of AI-Madinah (Saudi Arabia) from 1998 to 2002. A comparative study has also been carried out between the Bayesian network with the classical neural network and the empirical model developed using the Angstrom–Prescott equation. Mellit (2005) and Mellit et al. (2007) applied an ANFIS model for estimating the sequence of monthly mean clearness index (kt) and daily solar radiation data in isolated areas of Algerian location with some geographical coordinates (latitude, longitude, and altitude) and meteorological parameters such as temperature, humidity, and wind speed. The comparison has also been made between ANFIS and ANN by evaluating the RMSE and MAPE.
An ANFIS model (Mellit, 2004; Mellit et al., 2008) is presented for estimating the mean monthly clearness index (Kt) and total solar radiation data in isolated sites based on geographical coordinates. These data have been collected from 60 locations in Algeria. The magnitude of solar radiation is the most important parameter for sizing photovoltaic (PV) systems. The ANFIS model is trained using MLP based on fuzzy logic (FL) rules. The inputs of the ANFIS model are the latitude, longitude, and altitude, while the outputs are the 12-values of mean monthly clearness index Kt. The results show that the performance of the proposed approach in the prediction of mean monthly clearness index Kt is favorably compared to the measured values. The RMSE between measured and estimated values varies between 0.0215 and 0.0235 and the MAPE is less than 2.2%. Data from 60 locations in Algeria are taken into account, and the performance of the model is found out through the RMSE and mean relative error (MRE) (Angstrom, 1924; Garg and Garg, 1983; Takagi and Sugeno, 1985; Bahel et al., 1987c; Hawlader et al., 2001; Kalogirou, 2001; Iqdour and Zeroual, 2004; López et al., 2005; Tymvios et al., 2005; Bosch et al., 2008; Zounemat-Kermani and Teshnehlab, 2008; Behrang et al., 2010; Tektaş, 2010; Coulson, 2012; Boland et al., 2013; Jafarkazemi et al., 2013; Will et al., 2013; Ramedani et al., 2014b; Choubin et al., 2014; Varzandeh et al., 2014; Mohammadi et al., 2015; Choubin et al., 2016a; Choubin et al., 2016b; Mohammadi et al., 2016b; Despotovic et al., 2016; Kaplanis et al., 2016; Wu and Wang, 2016; Quej et al., 2017; Zou et al., 2017; Almaraashi, 2018b; Halabi et al., 2018; Khosravi et al., 2018; Rafiei-Sardooi et al., 2018). Yadav et al. (2014) have applied the J48 algorithm and WEKA software for selecting significant input parameters such as clearness index, altitude, and longitude for the better prediction of solar radiation in Western Himalayas with ANN (Mani, 2008; Yadav et al., 2014; Yadav and Chandel, 2015). By using the four g-bell input membership function, statistical analysis shows the maximum regression value; R (R = 0.99) in comparison to the other membership function (Bhardwaj et al., 2013a; Ramedani et al., 2013b). M Rizwan et al. (Khan et al., 2008; Rizwan et al., 2012; Chaudhary and Rizwan, 2018; Perveen et al., 2018; Sadhu et al., 2018; Chaudhary and Rizwan, 2019) focused on the GNN model in order to predict global solar energy in India. Parameters used as inputs in this particular model include latitude, longitude, and altitude (Iqbal et al., 2010; Patel and Parekh, 2014; Singh and Rizwan, 2018b; Yadav et al., 2018b; Sujil et al., 2019b; Singh et al., 2019; Vanitha et al., 2019) with temperature ratio, Sunshine/hour whereas the clearness index stands for the output parameter. Solar radiation data set has been prepared for several Indian states for training and performance is evaluated through the mean absolute error. The MAPE during the estimation of global solar energy prediction is found to be nearly 4 percent using GNN but it becomes 6 percent during estimation with the fuzzy logic (Ajil et al., 2010; Chandra et al., 2013; Verma et al., 2019). Joshi (2013) estimated the monthly global solar radiation utilizing the Angstrom model (Angstrom, 1924) forecast solar irradiation with the help of ANN with variables. Thorough comparisons have been carried out between the ANN model and Angstrom model in order to judge the efficiency of the models with mean squared error (MSE) and regression coefficient (R2). From the learning of MSE and R2 values with Angstrom models they come close to (0.1225 and 0.3965) for Ahmedabad, (0.0059 and 0.0149) for Bangalore, (0.1024 and 0.404) for Dehradun, and (0.0625 and 0.0498) for Kolkata whereas the MSE and R2 values for the ANN model as (0.002 and 0.99) for Ahmedabad, (0.006 and 0.98) for Bangalore, (0.01, 0.90) for Dehradun, and (0.006 and 0.99) for Kolkata. The selected ANN model performs better with less RMSE value with maximum regression value than the empirical model. Kadhambari et al. (2012) proposed a recurrent neural network model to estimate the global solar radiation of the Thiruvallur region. Input parameters utilized in this work are all days of month, all day temperature, humidity (relative), pressure of air, and solar azimuth angle. The RNN-based models are trained by the evolutionary swarm optimization–based algorithm. The performances of these algorithms are verified and compared with each other by calculating the RMSE value. The RMSE value of the evolutionary algorithm comes around 0.0667 which is lower in comparison to the RMSE value of the PSO algorithm. Poudyall khem et al. estimate [GSR] depending on the sunshine duration in the Himalayan region. The performance parameters of the model are investigated on the basis of RMSE value, MBE value, MPE value, and correlation coefficient R2 value. Solar radiation data for a span of 3 years of Indian cities have been studied by Katiyar and monthly daily mean clear sky radiation has been estimated.
A thorough comparison on the basis of (RMSE) and (MBE) has been initiated which shows the percentage of MBE with a new constant for each station vary from 0.22 to 2.09% whereas with RMSE it varies from 2.22 to 10.37%. Krishnaiah et al. (2007b) suggest the neural network approach for modeling which suggests the superiority of the neural network–based model compared to conventional regression models. Premalatha and Arasu (2012) estimated the GSR of India utilizing ANN based on the input parameters such as maximum and minimum ambient temperature with least relative humidity.
The monthly global solar radiation in 31 districts of Tamil Nadu, India was predicted by using
Important Eastern stations of India such as Bhubaneswar, Kolkata, Visakhapatnam, Ranchi, Patna, Assam, Lucknow, and Hyderabad with their geographical and meteorological features are presented in Table 1 and Figures 4A,B. The map of Eastern India shows three important stations.
The ANFIS-based PV model predicts the I-V and P-V characteristics of the PV modules in a given environmental setting. For a certain irradiance and temperature combination of the PV cell, the voltage of PV array (from zero to open-circuit voltage) can be determined on the manufacturing datasheet. The corresponding anticipated current set is obtained from the proposed PV estimation model. The equivalent circuit of the PV is described as follows:
where I = output current.
IPh = photo current or generated current under given insolation.
IO = diode reverse saturation current.
η = ideality factor of PV cell.
RS = series loss resistance.
RSh = shunt loss resistance.
Vt = thermal voltage.
Input data = solar irradiance G.
Ambient temp = T.
Operating voltage = V.
Output current = I.
The ANFIS technique is used to find out the impact of all important variables such as
FIGURE 5. (A) PV system and its operating blocks, (B) I-V and P-V based characteristics at 25° with changing radiation.
A computer code for the ANFIS model is developed through MATLAB. The training of the model is continued until it gets the optimum results with a lower MSE and higher regression value (R). After fulfilling the optimal parameters with input and output membership functions, the results are saved and further utilized for training and testing
The regression plot describes the accuracy between the measured and the forecasted value of solar radiation. After splitting the data set, input and output membership functions used in this network are saved and used for training and testing the ANFIS models. Figure 9 describes the training curve after prediction. This curve shows the number of epochs with respect to error, that is, the curve shows how the error varies with respect to the number of epochs. The optimization is performed either by using the hybrid learning algorithm or the back-propagation method for identifying the MF (input and output) parameters. The output membership function (linear or constant) is used for training fuzzy inference system as mentioned in Figure 10.
FIGURE 10. (A) Prediction of solar radiation for the city of Bhubaneswar. (B) Solar radiation forecasting of Kolkata. (C) Prediction of solar radiation for the city of Visakhapatnam.
To validate the accuracy of the developed ANFIS method, its capability has been compared with the artificial neural network (ANN) and support vector machine (SVM). The statistical indicator helps the performance evaluation of the proposed model which indicates lower values of RMSE and MAPE and higher values of R2 during the comparison with ANN and other model (Table 2).
TABLE 2. Relative outcome with ANN, ANFIS, and SVM algorithms. Model comparison of different regions.
The assortment of different parameters remains the most important criteria for forecasting global solar-based radiation of a particular place. So by means of ANFIS methodology, parameters are chosen and dissimilar models are created (Table 3).
TABLE 3. Performance evaluation of Bhubaneswar, Kolkata, and Visakhapatnam with different algorithms.
During the training and testing of the model, only one input is considered. The performance outcome of both training and testing are presented in (Table 4) considering the sunshine input as the most favorable parameter.
The performance evaluation of different models with respect to the number of inputs is described in Table 4.
Here, two input parameters are combined for the purpose of training and testing. In total, six models have been created. The output obtained has been shown in Table 6. The statistical result shows for state Bhubaneswar model 1 with input temperature and sunshine duration produces improved results in comparison to other input combinations. But in the case of Visakhapatnam and Kolkata, model two with inputs (sunshine duration and Relative Humidity) will give a superior outcome compared to other input combinations.
Table 6 shows the statistical analysis with ANFIS models for cities Bhubaneswar, Kolkata, and Visakhapatnam.
Considering three vital input parameters, models have been formulated. Furthermore, all the statistical results have been verified providing better outcome with inputs such as sunshine duration, temperature, and humidity. The outputs after training and testing have been presented in Table 7. Proper knowledge about the inputs helps a lot in forecasting solar radiation at any particular place.
In the past, several initiatives have been taken in India regarding solar radiation data forecasting with conventional empirical models. Three important cities of Eastern India have been taken as case studies to carry out analysis for solar radiation prediction purposes. Furthermore, input parameters are fixed such as proportion of surface air pressure
Figures 13A–C show the surface plot for the optimal combination of inputs of three cities at the time of training and testing. The significant combination of parameters having one, two, and three inputs of three cities of Eastern zone of India during training and testing are shown in Figures 14A–C. The measured average monthly GSR was compared with measured values of Kolkata, Bhubaneswar, and Visakhapatnam (Tables 8, 9). Due to recurrent cyclonic effects, it is essential to devise new computational methods such as soft computing–based algorithms and their applications. ANFIS predicts better outcomes with calculated values (Figure 15).
FIGURE 13. (A–C) Surface plots combining two most important parameters for Bhubaneswar, Kolkata, and Vizag.
FIGURE 14. (A–C)
FIGURE 15. (A–C) Measured and predicted data using different soft computing approaches for Visakhapatnam.
In spite of its efficiency and computational ability, additional inputs are always needed for enhanced accuracy and robustness. More emphasis should be given to the input factors where error in computation of training data is found. Further data fluctuations are taken care of because of ANFIS’s robustness and computational skill. In this regard, a lot of work(Krishnaiah et al., 2007a; Ajil et al., 2010; Kadhambari et al., 2012; Premalatha and Arasu, 2012; Chandra et al., 2013; Joshi, 2013; Citakoglu, 2015; Awasthi and Poudyal, 2018; Verma et al., 2019)has been done in recent days emphasizing the combination of different models and input parameters for better forecasting studies (Table 10).
This research work has been prepared as a review study, which focuses on ANFIS-based solar radiation forecasting in Eastern part of India. Several studies have been undertaken with soft computing techniques. Suitable models have been developed based on several inputs and detailed analysis has been performed to show the minimum MSE and maximum regression (R) values in different places of Eastern India after training and testing. The main idea behind this study is to find out the significance of forecasting in solar radiation data collection and study its applications in agricultural crop production, hydrological, industrial, and ecological studies along the eastern coast of India. The performance of the ANFIS model in comparison with other prediction models has been studied to establish the significance of the proposed model in estimating solar radiation. After several studies, the ANFIS model seems to be computationally efficient and adaptable in managing different parameters. Consequently, the model is engaged in the estimation of the solar radiation–based data with extensively available meteorological information. It also overcomes errors, as it seems highly robust and efficient in dealing with data fluctuations. It may also be fused with additional soft computing approaches to get better network accuracy. The study also surveys similar ANFIS-based work in different areas of India in particular and other important places in the world. Further improvements are expected with several other combinations of meteorological data such as air pressure, humidity, sunshine duration, cloud index, and many more that can be associated with the model for future studies.
All authors contributed to this work. SM and SM have contributed in generating idea, accumulating information, and preparing the manuscript. All authors have read and approved the final manuscript.
The authors declare that the research was conducted in the absence of any commercial or financial relationships that could be construed as a potential conflict of interest.
All claims expressed in this article are solely those of the authors and do not necessarily represent those of their affiliated organizations, or those of the publisher, the editors, and the reviewers. Any product that may be evaluated in this article, or claim that may be made by its manufacturer, is not guaranteed or endorsed by the publisher.
The authors would like to thank the India Meteorological Department, Bhubaneswar, India for providing the required data for this research. The authors also want to appreciate the World Bank sponsored TEQIP-II research facility in Odisha University of Technology and Research (formerly CET Bhubaneswar), India.
Abdo, T., and El-Shimy, M. (2011). “Estimation of Global Solar Radiation (GSR) ER EOVdypt Estimation of Global Solar Radiation (GSR) over Egypt,” in The Future of New and Renewable Energy in Arab World, Assiut, Egypt.
Achour, L., Bouharkat, M., Assas, O., and Behar, O. (2017). Hybrid Model for Estimating Monthly Global Solar Radiation for the Southern of Algeria: (Case Study: Tamanrasset, Algeria). Energy 135, 526–539. doi:10.1016/j.energy.2017.06.155
Aguilar, L., Melin, P., and Castillo, O. (2003). Intelligent Control of a Stepping Motor Drive Using a Hybrid Neuro-Fuzzy ANFIS Approach. Appl. Soft Comput. 3 (3), 209–219. doi:10.1016/s1568-4946(03)00035-8
Ajil, K. S., Thapliyal, P. K., Shukla, M. V., Pal, P. K., Joshi, P. C., and Navalgund, R. R. (2010). A New Technique for Temperature and Humidity Profile Retrieval from Infrared-Sounder Observations Using the Adaptive Neuro-Fuzzy Inference System. IEEE Trans. Geosci. Remote Sens. 48 (4), 1650–1659. doi:10.1109/tgrs.2009.2037314
Al-Mostafa, Z. A., Maghrabi, A. H., and Al-Shehri, S. M. (2014). Sunshine-based Global Radiation Models: A Review and Case Study. Energy Convers. Manag. 84, 209–216. doi:10.1016/j.enconman.2014.04.021
Almaraashi, M. (2018). Investigating the Impact of Feature Selection on the Prediction of Solar Radiation in Different Locations in Saudi Arabia. Appl. Soft Comput. 66, 250–263. doi:10.1016/j.asoc.2018.02.029
Almaraashi, M. (2018). Investigating the Impact of Feature Selection on the Prediction of Solar Radiation in Different Locations in Saudi Arabia. Appl. Soft Comput. 66, 250–263. doi:10.1016/j.asoc.2018.02.029
Angstrom, A. (1924). Solar and Terrestrial Radiation. Report to the International Commission for Solar Research on Actinometric Investigations of Solar and Atmospheric Radiation. Q.J.R. Meteorol. Soc. 50 (210), 121–126. doi:10.1002/qj.49705021008
Asl, S. F. Z., Karami, A., Ashari, G., Behrang, A., Assareh, A., Hedayat, N., et al. (2011). Daily Global Solar Radiation Modelling Using Multi-Layer Perceptron (MLP) Neural Networks. World Acad. Sci. Eng. Technol. 79, 740–742.
Awasthi, J., and Poudyal, K. N. (2018). Estimation of Global Solar Radiation Using Empirical Model on Meteorological Parameters at Simara Airport, Bara, Nepal. J. Inst. Eng. 14 (1), 143–150. doi:10.3126/jie.v14i1.20078
Bahel, V., Bakhsh, H., and Srinivasan, R. (1987). A Correlation for Estimation of Global Solar Radiation. Energy 12 (2), 131–135. doi:10.1016/0360-5442(87)90117-4
Bahel, V., Bakhsh, H., and Srinivasan, R. (1987). A Correlation for Estimation of Global Solar Radiation. Energy 12 (2), 131–135. doi:10.1016/0360-5442(87)90117-4
Bahel, V., Bakhsh, H., and Srinivasan, R. (1987). A Correlation for Estimation of Global Solar Radiation. Energy 12 (2), 131–135. doi:10.1016/0360-5442(87)90117-4
Behrang, M. A., Assareh, E., Ghanbarzadeh, A., and Noghrehabadi, A. R. (2010). The Potential of Different Artificial Neural Network (ANN) Techniques in Daily Global Solar Radiation Modeling Based on Meteorological Data. Sol. Energy 84 (8), 1468–1480. doi:10.1016/j.solener.2010.05.009
Bhardwaj, S., Sharma, V., Srivastava, S., Sastry, O. S., Bandyopadhyay, B., Chandel, S. S., et al. (2013). Estimation of Solar Radiation Using a Combination of Hidden Markov Model and Generalized Fuzzy Model. Sol. Energy 93, 43–54. doi:10.1016/j.solener.2013.03.020
Bhardwaj, S., Sharma, V., Srivastava, S., Sastry, O. S., Bandyopadhyay, B., Chandel, S. S., et al. (2013). Estimation of Solar Radiation Using a Combination of Hidden Markov Model and Generalized Fuzzy Model. Sol. Energy 93, 43–54. doi:10.1016/j.solener.2013.03.020
Boland, J., Huang, J., and Ridley, B. (2013). Decomposing Global Solar Radiation into its Direct and Diffuse Components. Renew. Sustain. Energy Rev. 28, 749–756. doi:10.1016/j.rser.2013.08.023
Bosch, J. L., López, G., and Batlles, F. J. (2008). Daily Solar Irradiation Estimation over a Mountainous Area Using Artificial Neural Networks. Renew. Energy 33 (7), 1622–1628. doi:10.1016/j.renene.2007.09.012
Campos, V., Osório, G., Shafie-khah, M., Lotfi, M., and Catalão, J. P. (2018). “Short-Term Hybrid Probabilistic Forecasting Model for Electricity Market Prices,” in 2018 Twentieth International Middle East Power Systems Conference (MEPCON), Dec 18 (Cairo, Egypt: IEEE), 962–967. doi:10.1109/mepcon.2018.8635145
Chandra, D. R., Kumari, M. S., and Sydulu, M. (2013). “A Detailed Literature Review on Wind Forecasting,” in 2013 International Conference on Power, Energy and Control (ICPEC), Feb 6 (Dindigul, India: IEEE), 630–634. doi:10.1109/icpec.2013.6527734
Chaudhary, P., and Rizwan, M. (2018). Energy Management Supporting High Penetration of Solar Photovoltaic Generation for Smart Grid Using Solar Forecasts and Pumped Hydro Storage System. Renew. Energy 118, 928–946. doi:10.1016/j.renene.2017.10.113
Chaudhary, P., and Rizwan, M. (2019). Short Term Solar Energy Forecasting Using GNN Integrated Wavelet-Based Approach. Ijret 10 (3), 229–246. doi:10.1504/ijret.2019.101729
Choubin, B., Darabi, H., Rahmati, O., Sajedi-Hosseini, F., and Kløve, B. (2018a). River Suspended Sediment Modelling Using the CART Model: A Comparative Study of Machine Learning Techniques. Sci. Total Environ. 615, 272–281. doi:10.1016/j.scitotenv.2017.09.293
Choubin, B., Khalighi-Sigaroodi, S., Malekian, A., Ahmad, S., and Attarod, P. (2014). Drought Forecasting in a Semi-arid Watershed Using Climate Signals: a Neuro-Fuzzy Modeling Approach. J. Mt. Sci. 11 (6), 1593–1605. doi:10.1007/s11629-014-3020-6
Choubin, B., Khalighi-Sigaroodi, S., Malekian, A., and Kişi, Ö. (2016). Multiple Linear Regression, Multi-Layer Perceptron Network and Adaptive Neuro-Fuzzy Inference System for Forecasting Precipitation Based on Large-Scale Climate Signals. Hydrological Sci. J. 61 (6), 1001–1009. doi:10.1080/02626667.2014.966721
Choubin, B., Malekian, A., and Golshan, M. (2016). Application of Several Data-Driven Techniques to Predict a Standardized Precipitation Index. Atmósfera 29 (2), 121–128. doi:10.20937/atm.2016.29.02.02
Choubin, B., Malekian, A., Samadi, S., Khalighi-Sigaroodi, S., and Sajedi-Hosseini, F. (2017). An Ensemble Forecast of Semi-arid Rainfall Using Large-Scale Climate Predictors. Metall. Apps 24 (3), 376–386. doi:10.1002/met.1635
Choubin, B., Zehtabian, G., Azareh, A., Rafiei-Sardooi, E., Sajedi-Hosseini, F., and Kişi, Ö. (2018b). Precipitation Forecasting Using Classification and Regression Trees (CART) Model: a Comparative Study of Different Approaches. Environ. Earth Sci. 77 (8), 314. doi:10.1007/s12665-018-7498-z
Citakoglu, H. (2015). Comparison of Artificial Intelligence Techniques via Empirical Equations for Prediction of Solar Radiation. Comput. Electron. Agric. 118, 28–37. doi:10.1016/j.compag.2015.08.020
Coulson, K. (2012). Solar and Terrestrial Radiation: Methods and Measurements. Amsterdam, Netherlands: Elsevier. Dec 2.
Demirhan, H., and Kayhan Atilgan, Y. (2015). New Horizontal Global Solar Radiation Estimation Models for Turkey Based on Robust Coplot Supported Genetic Programming Technique. Energy Convers. Manag. 106, 1013–1023. doi:10.1016/j.enconman.2015.10.038
Demirhan, H. (2014). The Problem of Multicollinearity in Horizontal Solar Radiation Estimation Models and a New Model for Turkey. Energy Convers. Manag. 84, 334–345. doi:10.1016/j.enconman.2014.04.035
Despotovic, M., Nedic, V., Despotovic, D., and Cvetanovic, S. (2016). Evaluation of Empirical Models for Predicting Monthly Mean Horizontal Diffuse Solar Radiation. Renew. Sustain. Energy Rev. 56, 246–260. doi:10.1016/j.rser.2015.11.058
Fachini, F., and Lopes, B. I. (2019). “Critical Bus Voltage Mapping Using ANFIS with Regards to Max Reactive Power in PV Buses,” in 2019 IEEE Milan Power Tech (Milan, Italy: IEEE), 1–6. doi:10.1109/ptc.2019.8810562
Gairaa, K., Khellaf, A., Messlem, Y., and Chellali, F. (2016). Estimation of the Daily Global Solar Radiation Based on Box-Jenkins and ANN Models: A Combined Approach. Renew. Sustain. Energy Rev. 57, 238–249. doi:10.1016/j.rser.2015.12.111
Garg, H. P., and Garg, S. N. (1983). Prediction of Global Solar Radiation from Bright Sunshine Hours and Other Meteorological Data. Energy Convers. Manag. 23 (2), 113–118. doi:10.1016/0196-8904(83)90070-5
Halabi, L. M., Mekhilef, S., and Hossain, M. (2018). Performance Evaluation of Hybrid Adaptive Neuro-Fuzzy Inference System Models for Predicting Monthly Global Solar Radiation. Appl. energy 213, 247–261. doi:10.1016/j.apenergy.2018.01.035
Hassan, M. A., Khalil, A., Kaseb, S., and Kassem, M. A. (2018). Independent Models for Estimation of Daily Global Solar Radiation: A Review and a Case Study. Renew. Sustain. Energy Rev. 82, 1565–1575. doi:10.1016/j.rser.2017.07.002
Hassan, M. A., Khalil, A., Kaseb, S., and Kassem, M. A. (2017). Potential of Four Different Machine-Learning Algorithms in Modeling Daily Global Solar Radiation. Renew. Energy 111, 52–62. doi:10.1016/j.renene.2017.03.083
Hawlader, M. N. A., Chou, S. K., and Ullah, M. Z. (2001). The Performance of a Solar Assisted Heat Pump Water Heating System. Appl. Therm. Eng. 21 (10), 1049–1065. doi:10.1016/s1359-4311(00)00105-8
Ibrahim, I. A., and Khatib, T. (2017). A Novel Hybrid Model for Hourly Global Solar Radiation Prediction Using Random Forests Technique and Firefly Algorithm. Energy Convers. Manag. 138, 413–425. doi:10.1016/j.enconman.2017.02.006
Ilmi, S. H., Handayani, A. N., and Wibawa, A. P. (2018). “Water Production Forecasting Using Adaptive Neuro-Fuzzy Inference System,” in 2018 2nd East Indonesia Conference on Computer and Information Technology (EIConCIT) (Makassar, Indonesia: IEEE), 24–28. doi:10.1109/eiconcit.2018.8878549
Iqbal, A., Abu-Rub, H., and Ahmed, S. M. (2010). “Adaptive Neuro-Fuzzy Inference System Based Maximum Power Point Tracking of a Solar PV Module,” in 2010 IEEE International Energy Conference (Manama, Bahrain: IEEE), 51–56. doi:10.1109/energycon.2010.5771737
Iqdour, R. (2006). A Rule Based Fuzzy for Prediction of Solar Radiation. Rev. Des. energies renouvelables 9 (2), 113–120.
Iqdour, R., and Zeroual, A. (2004). “Modelling Solar Data Using the Takagi-Sugeno Fuzzy Systems,” in International Conference on Modelling & Simulation-MS (Vol. 4).
Jafarkazemi, F., Moadel, M., Khademi, M., and Razeghi, A. (2013). Performance Prediction of Flat-Plate Solar Collectors Using MLP and ANFIS. J. Basic Appl. Sci. Res. 3 (2), 196–200.
Joshi, J. (2013). A Research Study on Developing Solar Potential Map Using GIS. Int. J. Emerg. Trends Technol. Comput. Sci. 2.
Kadhambari, T., Yokesh, V., and Selva, T. (2012). Global Solar Radiation Prediction Using Recurrent Neural Networks. Int. J. Curr. Res. 7, 11492–11496.
Kalogirou, S. A. (2001). Artificial Neural Networks in Renewable Energy Systems Applications: a Review. Renew. Sustain. energy Rev. 5 (4), 373–401. doi:10.1016/s1364-0321(01)00006-5
Kaplanis, S., Kumar, J., and Kaplani, E. (2016). On a Universal Model for the Prediction of the Daily Global Solar Radiation. Renew. Energy 91, 178–188. doi:10.1016/j.renene.2016.01.037
Karri, C., Durgam, R., and Raghuram, K. (2018). “Electricity Price Forecasting in Deregulated Power Markets Using Wavelet-ANFIS-KHA,” in 2018 International Conference on Computing, Power and Communication Technologies (GUCON)Sep 28 (Greater Noida, India: IEEE), 982–987. doi:10.1109/gucon.2018.8674980
Keshtegar, B., Mert, C., and Kisi, O. (2018). Comparison of Four Heuristic Regression Techniques in Solar Radiation Modeling: Kriging Method vs RSM, MARS and M5 Model Tree. Renew. Sustain. energy Rev. 81, 330–341. doi:10.1016/j.rser.2017.07.054
Khan, M. R., Iqbal, A., and Ashraf, I. (2008). Artificial Neural Network Model of a 25 kW (Peak) Grid Connected Solar Photovoltaic Power Plant. JEE 1 (3), 19–24. doi:10.26634/jee.1.3.415
Khosravi, A., Koury, R. N. N., Machado, L., and Pabon, J. J. G. (2018). Prediction of Hourly Solar Radiation in Abu Musa Island Using Machine Learning Algorithms. J. Clean. Prod. 176, 63–75. doi:10.1016/j.jclepro.2017.12.065
Koca, A., Oztop, H. F., Varol, Y., and Koca, G. O. (2011). Estimation of Solar Radiation Using Artificial Neural Networks with Different Input Parameters for Mediterranean Region of Anatolia in Turkey. Expert Syst. Appl. 38 (7), 8756–8762. doi:10.1016/j.eswa.2011.01.085
Krishnaiah, T., Rao, S. S., Madhumurthy, K., and Reddy, K. S. (2007). Neural Network Approach for Modelling Global Solar Radiation. J. Appl. Sci. Res. 3 (10), 1105–1111.
Krishnaiah, T., SrinivasaRao, S., and Madhumurthy, K. (2007). Neural Network Approach for Modelling Global Solar Radiation. JApplSciRes 3, 1105–1111.
López, G., Batlles, F. J., and Tovar-Pescador, J. (2005). Selection of Input Parameters to Model Direct Solar Irradiance by Using Artificial Neural Networks. Energy 30 (9), 1675–1684. doi:10.1016/j.energy.2004.04.035
Ludwig, S. A. (2019). “Comparison of Time Series Approaches Applied to Greenhouse Gas Analysis: ANFIS, RNN, and LSTM,” in 2019 IEEE International Conference on Fuzzy Systems (FUZZ-IEEE), Jun 23 (New Orleans, LA, USA: IEEE), 1–6. doi:10.1109/fuzz-ieee.2019.8859013
Maitra, S., Madan, S., and Mahajan, P. (2018). “An Adaptive Neural Fuzzy Inference System for Prediction of Student Performance in Higher Education,” in 2018 International Conference on Advances in Computing, Communication Control and Networking (ICACCCN), Oct 12 (Greater Noida, India: IEEE), 1158–1163. doi:10.1109/icacccn.2018.8748869
Meenal, R., and Selvakumar, A. I. (2018). Assessment of SVM, Empirical and ANN Based Solar Radiation Prediction Models with Most Influencing Input Parameters. Renew. Energy 121, 324–343. doi:10.1016/j.renene.2017.12.005
Melin, P., and Castillo, O. (2005). Intelligent Control of a Stepping Motor Drive Using an Adaptive Neuro-Fuzzy Inference System. Inf. Sci. 170 (2-4), 133–151. doi:10.1016/j.ins.2004.02.015
Melin, P., Soto, J., Castillo, O., and Soria, J. (2012). A New Approach for Time Series Prediction Using Ensembles of ANFIS Models. Expert Syst. Appl. 39 (3), 3494–3506. doi:10.1016/j.eswa.2011.09.040
Mellit, A. (2005). An Adaptive Artificial Neural Network for Modelling and Simulation of a Stand-Alone Photovoltaic Power System. Proc. IEEE, SSD’05 4, 258.
Mellit, A. (2003). Modelling of Sizing the Photovoltaic System Parameters Using Artificial Neural Network. Proc. IEEE, CCA 1, 353–357.
Mellit, A. (2004). “Prediction and Modeling Signals from the Monitoring of Stand-Alone Photovoltaic Systems Using an Adaptive Neural Network Model,” in Proceedings of the 5th ISES European solar conference, 224–230.
Mellit, A., Benghanem, M., and Kalogirou, S. A. (2007). Modeling and Simulation of a Stand-Alone Photovoltaic System Using an Adaptive Artificial Neural Network: Proposition for a New Sizing Procedure. Renew. energy 32 (2), 285–313. doi:10.1016/j.renene.2006.01.002
Mellit, A., Kalogirou, S. A., Shaari, S., Salhi, H., and Hadj Arab, A. (2008). Methodology for Predicting Sequences of Mean Monthly Clearness Index and Daily Solar Radiation Data in Remote Areas: Application for Sizing a Stand-Alone PV System. Renew. Energy 33 (7), 1570–1590. doi:10.1016/j.renene.2007.08.006
Mohammad Hossein, M., Omid, R., Majid, V., Kaamran, R., and Fahime, H. (2014). “Wavelet Neural Network and ANFIS Algorithms for Short-Term Prediction of Solar Radiation and Wind Velocities,” in World Sustainability Forum Conference Proceedings.
Mohammadi, K., Shamshirband, S., Kamsin, A., Lai, P. C., and Mansor, Z. (2016). Identifying the Most Significant Input Parameters for Predicting Global Solar Radiation Using an ANFIS Selection Procedure. Renew. Sustain. Energy Rev. 63, 423–434. doi:10.1016/j.rser.2016.05.065
Mohammadi, K., Shamshirband, S., Petković, D., and Khorasanizadeh, H. (2016). Determining the Most Important Variables for Diffuse Solar Radiation Prediction Using Adaptive Neuro-Fuzzy Methodology; Case Study: City of Kerman, Iran. Renew. Sustain. Energy Rev. 53, 1570–1579. doi:10.1016/j.rser.2015.09.028
Mohammadi, K., Shamshirband, S., Tong, C. W., Arif, M., Petković, D., and Ch, S. (2015). A New Hybrid Support Vector Machine-Wavelet Transform Approach for Estimation of Horizontal Global Solar Radiation. Energy Convers. Manag. 92, 162–171. doi:10.1016/j.enconman.2014.12.050
Mohanty, A., Viswavandya, M., Mishra, D., Ray, P., and Mohanty, S. P. (2016). “ANFIS Based Sliding Mode Controller for Reactive Power Compensation in Fuel Cell Based Hybrid Power System,” in 2016 International Conference on Signal Processing, Communication, Power and Embedded System (SCOPES) (Paralakhemundi, India: IEEE), 851–855. doi:10.1109/scopes.2016.7955562
Mohanty, A., Viswavandya, M., Mohanty, S., and Paramita, P. (2016). “ANFIS-based Controller for DFIG-Based Tidal Current Turbine to Improve System Stability,” in Proceedings of the International Conference on Signal, Networks, Computing, and Systems (New Delhi: Springer), 115–122. doi:10.1007/978-81-322-3589-7_12
Mohanty, P. P., Mohapatra, D., Mohanty, A., and Nayak, S. (2020). “ANFIS-based Modeling for Prediction of Surface Roughness in Powder Mixed Electric Discharge Machining,” in Computational Intelligence in Data Mining (Singapore: Springer), 151–159. doi:10.1007/978-981-13-8676-3_14
Mohanty, S., Rout, A., Patra, P. K., and Sahoo, S. S. (2017). ANFIS Based Solar Radiation Data Forecasting for Energy and Economic Study of Solar Water Heaters in Eastern India. Int. J. Control Theory Appl. 10, 179–190.
Mohanty, S., Patra, P. K., Sahoo, S. S., and Mohanty, A. (2017). Forecasting of Solar Energy with Application for a Growing Economy like India: Survey and Implication. Renew. Sustain. Energy Rev. 78, 539–553. doi:10.1016/j.rser.2017.04.107
Motepe, S., Hassan, A. N., and Stopforth, R. (2018). “South African Distribution Networks Load Forecasting Using ANFIS,” in 2018 IEEE International conference on power electronics, drives and energy system (PEDES), Dec 18 (Chennai, India: IEEE), 1–6. doi:10.1109/pedes.2018.8707876
Nguyen, T., and Liao, Y. (2011). Short-Term Load Forecasting Based on Adaptive Neuro-Fuzzy Inference System. J. Comput. 6 (11), 2267–2271. doi:10.4304/jcp.6.11.2267-2271
Notton, G., Cristofari, C., Poggi, P., and Muselli, M. (2002). Calculation of Solar Irradiance Profiles from Hourly Data to Simulate Energy Systems Behaviour. Renew. Energy 27 (1), 123–142. doi:10.1016/s0960-1481(01)00166-5
Ododo, J. C., Sulaiman, A. T., Aidan, J., Yuguda, M. M., and Ogbu, F. A. (1995). The Importance of Maximum Air Temperature in the Parameterisation of Solar Radiation in Nigeria. Renew. Energy 6 (7), 751–763. doi:10.1016/0960-1481(94)00097-p
Ojosu, J. O., and Komolafe, L. K. (1987). Models for Estimating Solar Radiation Availability in South Western Nigeria. Niger. J. Sol. Energy 7, 69–77.
Olatomiwa, L., Mekhilef, S., Shamshirband, S., Mohammadi, K., Petković, D., and Sudheer, C. (2015). A Support Vector Machine-Firefly Algorithm-Based Model for Global Solar Radiation Prediction. Sol. Energy 115, 632–644. doi:10.1016/j.solener.2015.03.015
Olatomiwa, L., Mekhilef, S., Shamshirband, S., Mohammadi, K., Petković, D., and Sudheer, C. (2015). A Support Vector Machine-Firefly Algorithm-Based Model for Global Solar Radiation Prediction. Sol. energy 115, 632–644. doi:10.1016/j.solener.2015.03.015
Olatomiwa, L., Mekhilef, S., Shamshirband, S., and Petković, D. (2015). Adaptive Neuro-Fuzzy Approach for Solar Radiation Prediction in Nigeria. Renew. Sustain. Energy Rev. 51, 1784–1791. doi:10.1016/j.rser.2015.05.068
Olatomiwa, L., Mekhilef, S., Shamshirband, S., and Petkovic, D. (2015). RETRACTED ARTICLE: Potential of Support Vector Regression for Solar Radiation Prediction in Nigeria. Nat. Hazards 77 (2), 1055–1068. doi:10.1007/s11069-015-1641-x
Patel, J., and Parekh, F. (2014). Forecasting Rainfall Using Adaptive Neuro-Fuzzy Inference System (ANFIS). Int. J. Appl. or Innovation Eng. Manag. 3 (6), 262–269.
Pérez, E. C., Algredo-Badillo, I., and Rodríguez, V. H. (2012). Performance Analysis of ANFIS in Short Term Wind Speed Prediction. arXiv Prepr. Arxiv1212. 2671. Dec 11.
Perveen, G., Rizwan, M., and Goel, N. (2019). Comparison of Intelligent Modelling Techniques for Forecasting Solar Energy and its Application in Solar PV Based Energy System. IET Energy Syst. Integr. 1 (1), 34–51. doi:10.1049/iet-esi.2018.0011
Perveen, G., Rizwan, M., and Goel, N. (2018). Intelligent Model for Solar Energy Forecasting and its Implementation for Solar Photovoltaic Applications. J. Renew. Sustain. Energy 10 (6), 063702. doi:10.1063/1.5027824
Pourdaryaei, A., Mokhlis, H., Illias, H. A., Kaboli, S. H. A., and Ahmad, S. (2019). Short-term Electricity Price Forecasting via Hybrid Backtracking Search Algorithm and ANFIS Approach. IEEE Access 7, 77674–77691. doi:10.1109/access.2019.2922420
Pousinho, H. M. I., Mendes, V. M. F., and Catalão, J. P. S. (2011). Application of Adaptive Neuro-Fuzzy Inference for Wind Power Short-Term Forecasting. IEEJ Trans. Elec Electron Eng. 6 (6), 571–576. doi:10.1002/tee.20697
Premalatha, N., and Arasu, A. V. (2012). Estimation of Global Solar Radiation in India Using Artificial Neural Network. Int. J. Eng. Sci. Adv. Technol. 2, 1715–1721.
Prescott, J. A. (1940). Evaporation from a Water Surface in Relation to Solar Radiation. Trans. Roy. Soc. S. Aust. 46, 114–118.
Quej, V. H., Almorox, J., Ibrakhimov, M., and Saito, L. (2016). Empirical Models for Estimating Daily Global Solar Radiation in Yucatán Peninsula, Mexico. Energy Convers. management’ 110, 448–456.
Quej, V. H., Almorox, J., Arnaldo, J. A., and Saito, L. (2017). ANFIS, SVM and ANN Soft-Computing Techniques to Estimate Daily Global Solar Radiation in a Warm Sub-humid Environment. J. Atmos. Solar-Terrestrial Phys. 155, 62–70. doi:10.1016/j.jastp.2017.02.002
Rafiei-Sardooi, E., Mohseni-Saravi, M., Barkhori, S., Azareh, A., Choubin, B., and Jafari-Shalamzar, M. (2018). Drought Modeling: a Comparative Study between Time Series and Neuro-Fuzzy Approaches. Arab. J. Geosci. 11 (17), 487. doi:10.1007/s12517-018-3835-5
Rahimikhoob, A. (2010). Estimating Global Solar Radiation Using Artificial Neural Network and Air Temperature Data in a Semi-arid Environment. Renew. Energy 35 (9), 2131–2135. doi:10.1016/j.renene.2010.01.029
Rahoma, W. A. (2011). Application of Neuro-Fuzzy Techniques for Solar Radiation. J. Comput. Sci. 7, 1605–1611. doi:10.3844/jcssp.2011.1605.1611
Ramedani, Z., Omid, M., Keyhani, A., Shamshirband, S., and Khoshnevisan, B. (2014). Potential of Radial Basis Function Based Support Vector Regression for Global Solar Radiation Prediction. Renew. Sustain. Energy Rev. 39, 1005–1011.
Ramedani, Z., Omid, M., Keyhani, A., Khoshnevisan, B., and Saboohi, H. (2014). A Comparative Study between Fuzzy Linear Regression and Support Vector Regression for Global Solar Radiation Prediction in Iran. Sol. Energy 109, 135–143. doi:10.1016/j.solener.2014.08.023
Ramedani, Z., Omid, M., and Keyhani, A. (2013). Modeling Solar Energy Potential in a Tehran Province Using Artificial Neural Networks. Int. J. Green Energy 10 (4), 427–441. doi:10.1080/15435075.2011.647172
Ramedani, Z., Omid, M., and Keyhani, A. (2013). Modeling Solar Energy Potential in a Tehran Province Using Artificial Neural Networks. Int. J. Green Energy 10 (4), 427–441. doi:10.1080/15435075.2011.647172
Rizwan, M., Jamil, M., and Kothari, D. P. (2012). Generalized Neural Network Approach for Global Solar Energy Estimation in India. IEEE Trans. Sustain. Energy 3 (3), 576–584. doi:10.1109/tste.2012.2193907
Robaa, S. M. (2009). Validation of the Existing Models for Estimating Global Solar Radiation over Egypt. Energy Convers. Manag. 50 (1), 184–193. doi:10.1016/j.enconman.2008.07.005
Sadhu, M., Goswami, U., Das, N., Sadhu, P. K., and Goswami, A. (2018). Improvement of Energy Forecasting Model to Safeguard Energy Security in India. J. Renew. Sustain. Energy 10 (6), 065907. doi:10.1063/1.5053109
Singh, P. K., Singh, N., and Negi, R. (2019). “Short-Term Price Forecasting with Renewable Integration in Smart Grids Using ARIMA-ANN-ANFIS Hybrid Model,” in 2019 International Conference on Electrical, Electronics and Computer Engineering (UPCON) (Aligarh, India: IEEE), 1–6. doi:10.1109/upcon47278.2019.8980042
Singh, U., and Rizwan, M. (2018). “Analysis of Fuzzy Logic, ANN and ANFIS Based Models for the Forecasting of Wind Power,” in 2018 2nd IEEE International Conference on Power Electronics, Intelligent Control and Energy Systems (ICPEICES) (Delhi, India: IEEE), 1–7. doi:10.1109/icpeices.2018.8897445
Singh, U., and Rizwan, M. (2018). “Analysis of Fuzzy Logic, ANN and ANFIS Based Models for the Forecasting of Wind Power,” in 2018 2nd IEEE International Conference on Power Electronics, Intelligent Control and Energy Systems (ICPEICES) (Delhi, India: IEEE), 1–7. doi:10.1109/icpeices.2018.8897445
Sobri, S., Koohi-Kamali, S., and Rahim, N. A. (2018). Solar Photovoltaic Generation Forecasting Methods: A Review. Energy Convers. Manag. 156, 459–497. doi:10.1016/j.enconman.2017.11.019
Sujil, A., Kumar, R., and Bansal, R. C. (2019). FCM Clustering‐ANFIS‐based PV and Wind Generation Forecasting Agent for Energy Management in a Smart Microgrid. J. Eng. 2019 (18), 4852–4857. doi:10.1049/joe.2018.9323
Sujil, A., Kumar, R., and Bansal, R. C. (2019). FCM Clustering‐ANFIS‐based PV and Wind Generation Forecasting Agent for Energy Management in a Smart Microgrid. J. Eng. 2019 (18), 4852–4857. doi:10.1049/joe.2018.9323
Takagi, T., and Sugeno, M. (1985). Fuzzy Identification of Systems and its Applications to Modeling and Control. IEEE Trans. Syst. Man. Cybern. SMC-15 (1), 116–132. doi:10.1109/tsmc.1985.6313399
Teke, A., and Yıldırım, H. B. (2014). Estimating the Monthly Global Solar Radiation for Eastern Mediterranean Region. Energy Convers. Manag. 87, 628–635. doi:10.1016/j.enconman.2014.07.052
Tektaş, M. (2010). Weather Forecasting Using ANFIS and ARIMA Models. Environ. Res. Eng. Manag. 51 (1), 5–10.
Tian, Y. Q., Davies-Colley, R. J., Gong, P., and Thorrold, B. W. (2001). Estimating Solar Radiation on Slopes of Arbitrary Aspect. Agric. For. Meteorology 109 (1), 67–74. doi:10.1016/s0168-1923(01)00245-3
Tymvios, F. S., Jacovides, C. P., Michaelides, S. C., and Scouteli, C. (2005). Comparative Study of Ångström's and Artificial Neural Networks' Methodologies in Estimating Global Solar Radiation. Sol. energy 78 (6), 752–762. doi:10.1016/j.solener.2004.09.007
Uyar, K., Ilhan, U., Iseri, E. I., and Ilhan, A. (2019). “Forecasting Measles Cases in Ethiopia Using Neuro-Fuzzy Systems,” in 2019 3rd International Symposium on Multidisciplinary Studies and Innovative Technologies (ISMSIT) (Ankara, Turkey: IEEE), 1–5. doi:10.1109/ismsit.2019.8932882
Vanitha, V., Raphel, D., and Resmi, R. (2019). “Forecasting of Wind Power Using Variational Mode Decomposition-Adaptive Neuro Fuzzy Inference System,” in 2019 Innovations in Power and Advanced Computing Technologies (i-PACT) (Vellore, India: IEEE), 1–4. doi:10.1109/i-pact44901.2019.8960017
Varzandeh, M. H., Rahbari, O., Vafaeipour, M., Raahemifar, K., and Heidarzade, F. (2014). “Performance of Wavelet Neural Network and ANFIS Algorithm for Short Term Prediction,” in The 4th world sustainability forum.
Verma, N., Singh, P. K., Singh, N., and Negi, R. (2019). “Short Term Wind Power Forecasting Using Optimized WT-ANFIS Hybrid Model,” in 2019 IEEE Students Conference on Engineering and Systems (SCES), May 29 (Allahabad, India: IEEE), 1–6. doi:10.1109/sces46477.2019.8977217
Viswavandya, M., and Mohanty, A. (2017). Fuzzy Logic and ANFIS Based Short Term Solar Energy Forecasting. Int. J. Future Revolut. Comput. Sci. Commun. Eng. 78, 539–553.
Viswavandya, M., Sarangi, B., Mohanty, S., and Mohanty, A. (2020). “Short Term Solar Energy Forecasting by Using Fuzzy Logic and ANFIS,” in Computational Intelligence in Data Mining (Singapore: Springer), 751–765. doi:10.1007/978-981-13-8676-3_63
Will, A., Bustos, J., Bocco, M., Gotay, J., and Lamelas, C. (2013). On the Use of Niching Genetic Algorithms for Variable Selection in Solar Radiation Estimation. Renew. energy 50, 168–176. doi:10.1016/j.renene.2012.06.039
Wu, Y., and Wang, J. (2016). A Novel Hybrid Model Based on Artificial Neural Networks for Solar Radiation Prediction. Renew. Energy 89, 268–284. doi:10.1016/j.renene.2015.11.070
Xue, X. (2017). Prediction of Daily Diffuse Solar Radiation Using ANN. Int. J. hydrogen energy 42 (47), 28214–28221.
Yacef, R., Benghanem, M., and Mellit, A. (2012). Prediction of Daily Global Solar Irradiation Data Using Bayesian Neural Network: A Comparative Study. Renew. energy 48, 146–154. doi:10.1016/j.renene.2012.04.036
Yadav, A. K., and Chandel, S. S. (2015). Solar Energy Potential Assessment of Western Himalayan Indian State of Himachal Pradesh Using J48 Algorithm of WEKA in ANN Based Prediction Model. Renew. Energy 75, 675–693. doi:10.1016/j.renene.2014.10.046
Yadav, A. K., Malik, H., and Chandel, S. S. (2014). Selection of Most Relevant Input Parameters Using WEKA for Artificial Neural Network Based Solar Radiation Prediction Models. Renew. Sustain. Energy Rev. 31, 509–519. doi:10.1016/j.rser.2013.12.008
Yadav, H. K., Pal, Y., and Tripathi, M. M. (2018). “Short-Term PV Power Forecasting Using Adaptive Neuro-Fuzzy Inference System,” in 2018 IEEE 8th Power India International Conference (PIICON) (Kurukshetra, India: IEEE), 1–6. doi:10.1109/poweri.2018.8704445
Yadav, H. K., Pal, Y., and Tripathi, M. M. (2018). “Short-Term PV Power Forecasting Using Adaptive Neuro-Fuzzy Inference System,” in 2018 IEEE 8th Power India International Conference (PIICON) (Kurukshetra, India: IEEE), 1–6. doi:10.1109/poweri.2018.8704445
Yıldırım, H. B., Çelik, Ö., Teke, A., and Barutçu, B. (2018). Estimating Daily Global Solar Radiation with Graphical User Interface in Eastern Mediterranean Region of Turkey. Renew. Sustain. Energy Rev. 82, 1528–1537.
Yohanna, J. K., Itodo, I. N., and Umogbai, V. I. (2011). A Model for Determining the Global Solar Radiation for Makurdi, Nigeria. Renew. Energy 36 (7), 1989–1992. doi:10.1016/j.renene.2010.12.028
Yousefi, M., Kianpoor, N., Hajizadeh, A., and Soltani, M. (2018). “ANFIS Based Approach for Stochastic Modeling of Smart Home,” in 2018 2nd European Conference on Electrical Engineering and Computer Science (EECS), Dec 20 (Bern, Switzerland: IEEE), 458–463. doi:10.1109/eecs.2018.00091
Zou, L., Wang, L., Xia, L., Lin, A., Hu, B., and Zhu, H. (2017). Prediction and Comparison of Solar Radiation Using Improved Empirical Models and Adaptive Neuro-Fuzzy Inference Systems. Renew. Energy 106, 343–353. doi:10.1016/j.renene.2017.01.042
Keywords: solar radiation, forecasting, neural network, fuzzy logic, adaptive neuro-fuzzy, ANFIS
Citation: Mohanty S, Patra PK, Mohanty A, Harrag A and Rezk H (2022) Adaptive Neuro-Fuzzy Approach for Solar Radiation Forecasting in Cyclone Ravaged Indian Cities: A Review. Front. Energy Res. 10:828097. doi: 10.3389/fenrg.2022.828097
Received: 02 December 2021; Accepted: 12 April 2022;
Published: 26 May 2022.
Edited by:
Petru Adrian Cotfas, Transilvania University of Brașov, RomaniaReviewed by:
Godwin Norense Osarumwense Asemota, University of Rwanda, RwandaCopyright © 2022 Mohanty, Patra, Mohanty, Harrag and Rezk. This is an open-access article distributed under the terms of the Creative Commons Attribution License (CC BY). The use, distribution or reproduction in other forums is permitted, provided the original author(s) and the copyright owner(s) are credited and that the original publication in this journal is cited, in accordance with accepted academic practice. No use, distribution or reproduction is permitted which does not comply with these terms.
*Correspondence: A. Harrag, YS5oYXJyYWdAdW5pdi1zZXRpZi5keg==
Disclaimer: All claims expressed in this article are solely those of the authors and do not necessarily represent those of their affiliated organizations, or those of the publisher, the editors and the reviewers. Any product that may be evaluated in this article or claim that may be made by its manufacturer is not guaranteed or endorsed by the publisher.
Research integrity at Frontiers
Learn more about the work of our research integrity team to safeguard the quality of each article we publish.