- 1Institute of Infrastructure Technology Research and Management (IITRAM), Ahmedabad, India
- 2Department of Electrical Engineering, Qatar University, Doha, Qatar
In recent years, electric unmanned areal vehicles (UAVs) are gaining popularity due to their capabilities in civil and military applications. The rationale is that UAVs can do more complex and risky tasks while maintaining mobility, safety, and cheap cost. UAVs are generally used for inspection and monitoring, search and rescue operation, delivery, precision agriculture, hobbyist applications, and many more. Due to a broad spectrum of applications, UAVs of various sizes and weights have evolved. UAVs have developed with the hybrid architecture of power supply incorporating batteries, fuel cells, solar photovoltaic systems, and supercapacitors for extended endurance and improved performance. The electric propulsion system is the central part of UAVs, which generates thrust to control and hover the UAVs in the air. The propulsion system includes an electric motor, electronic speed controller, power sources, and an energy management system for efficient operation. This paper presents an extensive and critical evaluation of state of the art on classifications, components of the electric propulsion system, the control strategy of electric motor, hybridization of power sources, and energy management system to identify research gaps and give insights exhortations for future research.
1 Introduction
An unmanned aerial vehicle (also called a drone) is a flying robot that can fly considerable distances autonomously or remotely to complete a specific mission (Aasen et al. (2018)), especially when the presence of a human is dangerous, impossible, or impractical (Mukhamediev et al. (2021); Mohan et al. (2021)). Cruise missiles, artillery projectiles, torpedoes, ballistic or semi-ballistic vehicles, and satellites do not come under this category. Smart UAVs have come up in recent times with advancements in artificial intelligence (Lee et al. (2018)) and microprocessor technology. It is operated from the ground station remotely or independently and carries mortar or non-mortar payloads. The development of a wide range of UAVs has become feasible with advancements in navigation, wireless control capabilities, fabrication, electric motors, power electronic converters, and power storage systems. Governments and industrial organizations are undertaking research activities to improve performance and extended usage in civil and military applications. In the past, aerial vehicles found usage in military applications, but significant technological improvements over the years have gained vast opportunities in Civilian applications, including inspection and monitoring of traffic (Outay et al. (2020)), power line (Li et al. (2021); Luque-Vega et al. (2014)), solar photovoltaic panel (Surekha et al. (2020)), infrastructure,environment (Sivakumar and Naga Malleswari 2021) and other significant applications like goods delivery (Benarbia and Kyamakya (2021)), Smart agriculture (Tripicchio et al. (2015)), medical transportation (Rosser et al., 2018), Wireless coverage at a remote location away from cellular network (Al-Hourani and Gomez (2017)), Environmental applications (De Jong 2020), topographic change detection (De Haas et al. (2021)) and more.
The presence and thickness of snow on top of lake ice influences the timing of melt and ice-free situations. The use of UAVs helps recover snow depths with a high spatial resolution for the next generation of ultra-fine hydrological models (De Jong 2020). As renewable energy grows in popularity, researchers confront significant challenges in inspecting and maintaining renewable energy power grids. Elsewhere, an optimization technique for automated inspection of UAVs enhances the efficiency and cost of renewable energy power grid inspection and maintenance (Yao et al. (2021)). Wildlife research, especially in marine mammal inspections, and the negative effect of noise of UAVs on underwater animals are studied (Christiansen et al. (2016)). The UAV remote sensing technology enables precise agricultural parameters and yield estimates. UAV multi-spectral images help predict Chlorophyll content, yield under different water treatments, and vegetation indices (Han et al. (2021)). Drone industries are worth $82 billion in the United States alone, resulting in a significant economic impact with a broad spectrum of applications by creating more than 70, 000 job opportunities in the United States (Jenkins and Vasigh (2013)).
Mission requirements decide the features and configurations of UAVs. Design and classification of various UAVs are available in the literature based on different parameters. Concerning the shape, the varieties of UAVs are fixed-wing, rotary-wing, flapping-wing UAVs, and balloon configurations, and as per size, the classifications are nano, micro, and mini UAVs. The North Atlantic Treaty Organization (NATO) has classified UAVs in three categories based on maximum take-off weight based on altitude and mission (Valavanis and Vachtsevanos (2015)). Watts et al. discussed UAV Platform types for civil and military applications and characteristics such as flight endurance, size, and capabilities Watts et al. (2012), and the regulations affecting UAVs operations. Some of these classifications are HALE (High Altitude, Long Endurance), MALE (Medium Altitude, Long Endurance), LALE (Low Altitude, Long Endurance), VTOL (Vertical Take-Off Landing), NAVs (Nano Air Vehicles), MAVs (Micro or Miniature Air Vehicles).
In the current scenario, electric propulsion system (EPS) based UAVs are more popular because of their key benefits such as high efficiency (Bongermino et al. (2017)), precise control for complex acrobatics in the air, no pollution, high reliability (Jaeger and Adair (2017)), and reduced noise, apart from the depleting fossil fuels and emission of greenhouse gases. UAVs with internal combustion engines (ICE) have higher energy and power densities, thereby providing longer flight time (Bongermino et al. (2017)). However, such machines are not self-starting, and so electric motors, which are complex as far as control is concerned (Sato et al. (2020)), are required. In addition, the acoustic and thermal signatures of ICE are high. Xie et al. and Donateo et al. have combined both types of engines, electric and thermal, to secure the advantages of such hybrid engines (Xie et al. (2019), and Donateo and Spedicato (2017)). Batteries are part of EPS which store and provide power to UAVs. Batteries are more flexible to use, but they have a lower energy density and a long charging period which hinders usage in UAVs for extended flight missions (Gong and Verstraete (2017)). Also, batteries cannot respond to fast power demand by UAVs for some maneuvers due to slower power dynamics. Super capacitors are used as an auxiliary power source to balance battery limitations and assuage related issues (Gong et al. (2018)). One of the major benefits of supercapacitors is the ability to provide power quickly to perform complex maneuvers such as takeoff and sudden changes (Sai et al. (2018)). Aerodynamical optimization at the UAV design stage can extend flight endurance (Panagiotou et al. (2018)). With improvisation in battery technology, lithium-polymer (Li-Po) batteries can extend flight time up to 90 min (Verstraete et al. (2012b)). One option is to increase flight time by using more than one battery, but it is not feasible due to space and weight constraints. Another approach is to use additional power sources to compensate for the limits of the batteries while still meeting the weight and space Constraints. Fuel cells are a suitable alternative because of their high specific energy and quasi-instantaneous recharging (De Wagter et al. (2021)).
Hybrid UAVs (combination of battery and fuel cell) have longer flight times compared to battery-powered UAVs because fuel cells have a higher specific density, nearly five times higher than the battery (Verstraete et al. (2012b)). In some applications of UAVs, the fuel cell is the primary energy source. Also, a super capacitor is a good candidate for power storage devices. A solar cell is one of the renewable power sources generally used in fixed-wing UAVs, for it reduces the consumption of fuel, and so flight time is significantly more (Woźniak and Jessa (2021)). More than one DC power source connected on a common DC bus, known as hybridization of the power system, is the best choice for it combines the benefits of all power sources and balances the limitations of each source. Also, such a concept is handy for a considerably higher endurance of UAVs. The design of the power supply structure is critical and depends on the UAV mission needs and the characteristics of the power sources. For example, in a power supply hybridization system, more than one source supplies power. So for optimal power control, an energy management system (EMS) that splits required power among available sources efficiently and with good performance is needed. Current and voltage sensors help monitor power flow, and power electronic converters control the output power of sources, and a processing unit implements power management strategies (Tao et al. (2019)).
The motor-propeller system is employed in an electric propulsion system of UAVs to generate lift force. Based on the requirements of UAV applications, an appropriate combination of motor propellers helps efficient propulsion system operation. Finding such a combination depends on the modeling of the electric motor and propeller. For motor modeling, there are two main approaches. The equivalent circuit model is one, and the positive polynomial loss model is the other. The first method is straightforward and intuitive but ignores motor losses. The second technique can correctly calculate actual motor efficiency (McDonald (2014)), but some of the parameters are obtainable through experience or experimental tests, which lengthens the design cycle. Generally, the blade element approach is the simplest for propeller modeling. However, it cannot simulate the radial distribution of induced velocity, but Vortex theory can perform this calculation much faster. So in most studies, the vortex theory is used (Tjhai (2013)). In general practice, permanent magnet synchronous motors (PMSM) and BLDC motors find usage in the electric propulsion system. In such motors, the commutation process happens electronically. So electronic speed controller (ESC) is required to control these motors efficiently and as per the need of the flight processing unit. The development of an ESC for drones necessitates high-quality components, particularly those designed for running high-speed motors at full speed. The main features of the ESC include bridge inverter circuitry and microcontroller unit. Various speed control strategies for electric motor speed and torque control in the literature are applicable for UAV applications. The control strategies are implemented in the microcontroller unit and generate PWM signals based on reference signals provided by the flight processing unit and measured data from voltage and current sensors. They also necessitate the collection of motor rotor position data from Hall effect sensors or sensorless techniques (Carey et al. (2018)).
Six-step control is a simple technique that produces a high torque ripple in output (Cabuk (2021)). Field-oriented control and direct torque control are the most popular techniques for PMSM and BLDC motor control applications. Bridge inverter circuit provides commutation to the electric motors. Six switch bridge configuration generally uses such types of electric motors. With recent advances in the field of power electronics, inverter configurations with four switches are also in use for electric motor drive applications (Mani et al. (2022)). The Four switch configuration has high efficiency and is cost-effective, but the control algorithm is more complex (Elkerdany et al. (2020)). Texas Instruments has created the InstaSPIN MCU family, which simplifies the design of three-phase motor control applications. The InstaSPIN-FOC includes a quick software encoder with torque and velocity control for any three-phase motor, making it ideal for sensorless systems. ST-Microelectronics also provides a complete electronic speed controller reference design that uses a sensorless FOC algorithm. Another type of ESC reference design that can drive any three-phase PMSM or BLDC motor driven by battery packs, or any similar DC source, with a peak current of 30 A, is appropriate for entry-level commercial drones.
UAVs have been steadily improving, and they now offer a wide range of uses. As a result, researchers are focusing their efforts on UAVs, which are dealing with issues such as compact design and increased endurance. These issues may be overcome with appropriate EPS design, which improves overall efficiency and flying duration without requiring a modification in the supply system. In this perspective, and because the EPS is the core of a UAV platform, every component of the EPS is extensively examined in this paper. Various control strategies for BLDC motor speed regulation are reviewed in order to assist researchers in selecting the optimum control technique that enhances the UAVs’ overall performance. A comparison of several voltage source inverter typologies is provided, which aids the researcher in selecting the best configuration. To aid researchers in reducing power losses in electronic speed controllers, various wide band gap devices are compared. It also concentrates on the energy aspect of the onboard propulsion system, presenting a comparative and critical state-of-the-art analysis of UAV power-supply systems and appropriate energy/power management strategies. Because of their poor performance under varying operating situations, one source-based UAV power supply system will be severely limited. As a result, while building a UAV electric power system, hybridization of power sources with different characteristics is becoming a common option. The topic of endless endurance for certain missions was also considered in this study. Typical UAV power providing systems, such as swapping, laser-beam in-flight recharging, and UAV tethering, have all been shown and reviewed.
The review paper is organized as follows: Section 2 discusses basic information, including various parts of unmanned aerial systems, classification as per different parameters, and applications. Section 3 presents a comprehensive review of components of the electric propulsion system. UAVs power sources, a combination of various power sources, and the concept of DC microgrid are discussed in Section 4, while Section 5 and Section 6 discuss a critical review of energy management strategy to control DC microgrid, and concluding remarks, respectively.
2 General Information on Unmanned Aerial Systems
With technological growth, there is an endeavor to eliminate human error by autonomous operations (using autonomous vehicles like UAVs), including monitoring and inspections in inaccessible areas for safety considerations. Next, we focus on illustrating the essential components of such UAVs, like the communication modules, sensors, actuators, mission-oriented microcontrollers, and their brief classifications. Some other elements introduced in Figure 1 are elaborated in the subsequent sections of this work.
2.1 Unmanned Aerial System Basic Components
An onboard flight control system is systemic incorporation of various elements, as depicted in Figure 4. Onboard processing units include flight controllers and mission-oriented processing units. The first is required for any UAV and is solely responsible for 1) implementation of guidance, navigation, and control algorithm, 2) data collection, analysis, synthesis, logging, and storage in memory, 3) ground control station communication, and 4) mission planning.
The flight controller analyzes the data of sensors and makes decisions accordingly. Another processing unit is optional, but it typically has increased computational capacity for interacting with mission-oriented sensors and perform high-level activities like advanced sensing, task management, and mission planning. Mission-oriented microcontroller collects data from sensors and supplies command signal to flight controller for necessary actions. The information about different spatial coordinates of UAVs is collected using navigation sensors categorized as 1) Gyroscope, 2) accelerometer, 3) Magnetometer, 4) Global navigation satellite system called as GPS, and 5) Peripheral sensors including a barometer, odometer, airspeed sensor, altitude meter, etc. The Inertial Measurement Unit (IMU) is a device that provides acceleration and angular rate (roll, pitch, and yaw) measurements by combining accelerometers, gyroscopes, and magnetometers positioned along three strictly orthogonal axes with a microprocessor. Mission-oriented sensors work in conjunction with navigation sensors to provide additional information to ground crews for specific missions. Passive sensors and active sensors are two main categories of mission-oriented sensors. The passive sensors include different cameras and image-capture units like low-light-level cameras, thermal imagers, and electro-optical cameras. Communication modules installed at the ground control stations and UAVs enable wireless communication between them. These modules exchange information of the image and in-flight data. Small-scale UAVs and ground control stations can communicate via one of three methods: laser, fiber optics, or radio. Depending on UAV configurations, one or more sources can deliver power to the propulsion system and onboard flight control system.
For small-scale UAVs, a man-portable ground control station is typical. A rugged laptop serves as the foundation, and a communication base and antenna supplement the same. The ground control station’s primary duties are.
• Display real time location of UAVs for navigation view
• Monitor the data from UAVs in real time, display as needed, and store in memory as backup
• Send real time commands to UAVs as per mission requirements
• Display images captured by camera installed in UAV
• If necessary, assist in UAV decision-making, mission planning, and specific operations
• Assist ground users and pilots in automated control, mainly in situations like emergency landings
The propulsion system converts electrical energy into mechanical energy using motor propellers to fly UAVs. It includes DC power sources, electronic speed controllers, power electronic converters, energy management systems, and electric motors. The power management strategy distributes power demand among all available sources. Communication between the ground station and UAVs can be possible by radio frequency signals, internet, end-to-end secured communication, and many more communication systems. In the next Section, we describe Electric Propulsion systems used in UAVs in great detail.
2.2 UAVs Classifications
UAVs are classified into different types based on various parameters such as flight range, flying mode, endurance, size, weight, fuselage (fixed, rotary, flapping-wing), and utilization or applications (civil, scientific, and military uses). UAVs are also classifiable according to flight platforms such as fixed-wing UAVs, rotary-wing UAVs, flapping-wing UAVs, Unmanned airships, and parafoil UAVs (Li et al. (2021)). In addition, UAVs are divided into three categories depending on their maximum take-off weight and intended purposes (Brooke-Holland (2012)). Class I has four subcategories as shown in Table 1.
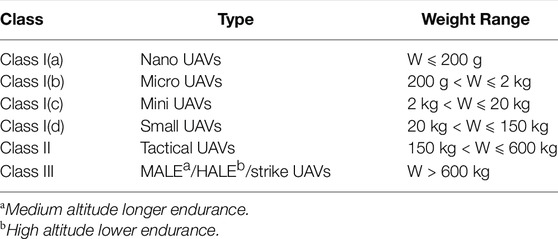
TABLE 1. UAV classification based on weight according to Brooke-Holland (2012).
Classification based on six main characteristics are also proposed (Cai et al. (2014)). In Table 2, UAVs are classified for range of maximum take off, weight less than 25 kg etc. UAVs are classified based on weight, engine type, maximum height above ground level, endurance, wing rotation (Arjomandi et al. (2006)). UAVs are categorized as super heavy, heavy, medium, light, micro which weight ranges from less than 5 kg to more than 2000 kg. UAVs classification based on roles in military missions, MTOW, operational height, midair collision risk and ownership, are also studied (Valavanis and Vachtsevanos (2015)).
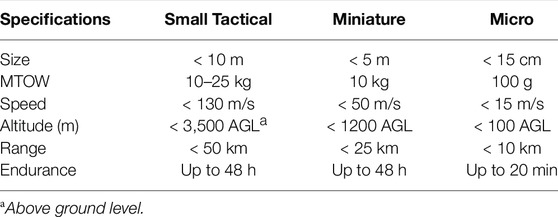
TABLE 2. UAVs classification proposed in Arjomandi et al. (2006).
3 Electric Propulsion System Components
The electric propulsion system (EPS) transforms stored electric power into mechanical power, is a power-consuming component of the UAVs and generates the required thrust by the motor-propeller system to hover the UAV. In addition, it occupies more than half of the volume in the UAVs, so a compact propulsion system is needed. Figure 2 depicts a schematic representation of an electric-powered UAV propulsion system. Unidirectional/bidirectional DC-DC converters help all power sources connect to a common DC bus. An energy management system (EMS) regulates energy consumption from the power sources in the UAV operating region. EMS requires data of voltages and currents from power sources and the common DC buses to decide the power contribution of sources and storage devices at different operating conditions.
DC bus is the junction where all the power sources and storage devices are connected. The capacitor bank is used at the DC bus to stabilize DC bus voltage. One major part of EPS is the electronic speed controller containing bridge inverter circuits and a microprocessor-based speed controller. Speed controller generates pulse width modulation (PWM) signals based on the implemented control strategy, reference generated by flight controller, and precise information from the motor. PWM signals help to trigger power electronic switches of bridge inverter and provide controlled power injection into the motor. A combination of Brushless direct current (BLDC) motors with fixed-pitch propellers are used in small UAVs applications to generate the required thrust. Induction motors have the advantages of low cost and robust structure, but are heavy and provide poor efficiency and so not commonly employed in UAVs.
3.1 Fixed Pitch Propeller
Propeller generates thrust and torque to control UAVs using two or more blades connected by a central hub that attaches the blades to the BLDC motor shaft. Output torque decides the motor efficiency and depends on speed, size, type, and other propeller features. So a suitable combination of propeller and motor is necessary through proper modeling of both the components to ensure that the motor operates with high efficiency to guarantee less consumption of power for the same thrust and increased flight time. Modelling of fixed pitch propeller (Moffitt et al. (2008); Quan (2017)) in terms of thrust F (N) and torque T (Nm) is given by
where CF and CT are thrust coefficient and torque coefficient respectively, and D(m) is diameter of propeller, n is angular velocity of the propeller, ρ is the air density varying with respect to local temperature Tt in (°C) and height h(m) given by
for standard air density ρ0 = 1.293 kg/m3 and atmospheric pressure p (Pa) found from (Cavcar (2000)):
The design and control of UAVs are mainly dependent on motor propeller combinations. For low Reynolds number applications, a mathematical relationship is possible between motor RPM and thrust produced by the propellers (Gupta and Abdallah (2018)). Therefore, it is necessary to estimate aerodynamic loads developed on the propeller by the Blade Element and Momentum Theory (BEMT) theory to estimate thrust generated for a given RPM (Johnson (1980)).
Thrust, torque, and power models of the propeller are available in literature based on steady-state analysis of hover conditions expressed as functions of propeller speed. Still, in actual practice, UAVs perform various activities like ascent or descent, rotation, translation, and many more. For accommodating such conditions, momentum theory helps calculate thrust (Bangura et al. (2014)). Momentum theory is also helpful for calculating the actual aerodynamic power in the airflow for the given thrust. Geometric measurement and vortex theory of air-screw propellers help ascertain propeller performance (Krishnan (2017)). Dimensionless thrust coefficient and power coefficient are also helpful to evaluate propeller performance (Lindahl et al. (2012). The coefficients are modeled as a function of the relative speed of the UAV and advancement ratio.
The motor propeller system has a severe problem of low efficiency, especially for electrically powered UAVs with multiple working states like hovering, cruising, transition, and maximum speed. A High-efficiency motor propeller system for tilt quadrotor is proposed for multi states to overcome this problem (Duan et al. (2020)) by using an improved parallel particle swarm optimization and differential evolution hybrid algorithm - an integrated optimization design method. Goldstein’s vortex theory helps model Variable-speed brushless motor and adjustable-pitch propellers. Improved parallel particle swarm optimization and differential evolution are compared with particle swarm optimization and genetic algorithm to determine effectiveness. A wind tunnel experiment helps validate the rationality of the integrated optimization technique. Other wind tunnel experiments ascertain the performance of small-diameter propellers (Quan (2017)) and show the variation of torque coefficient, thrust coefficient, power coefficient, and efficiency at different Reynolds numbers. Detailed model of fixed-pitch propeller requires the determination of thrust coefficient, torque coefficient, lift coefficient, and drag coefficient from only technical specifications of propellers provided by manufacturers (Shi et al. (2017). The thrust and RPM relationship results are simulated in MATLAB/Simulink and compared with experimental data.
3.2 Brushless Direct Current Motor
The permanent magnet synchronous motor (PMSM) drives and BLDC motors drives are possible options in high-performance applications. PMSM motor is with sinusoidal back electromotive force (EMF) and sinusoidal phase current waveform. BLDC motor is with trapezoidal back-EMF and rectangular phase current waveform due to structural differences between them.
Sensorless BLDC motors have found extensive industrial applications, computer peripheries, electric vehicles, and aerospace applications. Some distinct benefits are high power factor, high operating efficiency, lower noise, increased reliability, higher torque to inertia ratio, smaller size, lighter, long life, higher dynamic response, and easy control mechanisms (Maharajan and Xavier (2018)). Also, these features make BLDC motor a natural choice for the electric propulsion of UAVs. A permanent magnet synchronous motor with trapezoidal back emf known as PM brushless direct current motor finds use in UAVs. The commutation process is performed electronically because of the brushless motor structure. BLDC motor control methods use rotor position information. Hall sensors are employed to get the proper rotor position, but they are not suitable for small motors found in multi rotor aerial vehicles. Various sensorless techniques are available to estimate rotor position without physical sensors.
Figure 3 describes the Stator and Rotor of the outer runner BLDC motor. The Stator of the BLDC motor comprises staked steel lamination with a projected pole to carry three phase windings. Slots of the Stator are cut axially along the periphery while placing the windings connected in a star or delta manner in slots. The design of the Stator is decided based on the rating of the supply voltage. In the BLDC motor, there are no windings on the rotor but permanent magnets on the inner surface of the rotor. The materials which have the property to retain magnetism are known as hard magnets. Nickel, cobalt, and iron are ferromagnetic materials. Generally permanent magnets are made up of samarium-cobalt, ferrites, neodymium boron iron, neodymium ferrite boron and alnico-5. The most popular materials in practice are samarium cobalt and neodymium-type magnets because of their unique magnetic characteristics.
A detailed phase variable model of the BLDC motor assumes equal winding resistance of each phase, equal self-inductance of phase winding, equal mutual inductance between two windings and current induced in rotor neglected (Pillay and Krishnan (1989)), is given as
for an electromagnetic torque (Nm) given by
where Vas, Vbs, Vcs are the motor phase voltages, Ias, Ibs, Ics are the phase currents of motor, eas, ebs, ecs are the back emfs, Rs is the resistance of three phase stator winding, L is the self inductance of stator winding, and M is the mutual inductance of stator winding. Such a model is used to examine the performance of a BLDC servo drive system when fed by a pulse width modulation (PWM) and hysteresis current controller.
A simplified model of BLDC motor represented in state space form (Carey et al. (2018)) is given by
where vab and vbc are line voltages of motor, ia and ib are motor phase currents, eab and ebc are back emf of motor, R is the resistance of stator winding, ωe is the rotor’s electrical velocity, θe is the rotor’s electrical angle, Tl is the load torque, and J is the Moment of inertia. While measuring the phase voltage of a star-connected BLDC motor is challenging, measuring line voltages is simple. As a result, these equations in terms of line voltages help avoid complicated mathematical calculations.
BLDC motor model is combined with a fixed-pitch propeller model to control the aerodynamic thrust of multi-rotor UAVs (Bangura and Mahony (2017)). Based on the modeling of these two components, the thrust controller enhances disturbance rejection significantly and gust tolerance compared with rotor control schemes to show the superiority of the thrust control scheme. Furthermore, as per the theory of electric machinery, the BLDC motor can be modeled as a permanent magnet DC motor because of similarities between them (Toliyat and Kliman (2018)) and is commutated externally by power electronic switches. We assume that the controller estimates the rotor position and commutates the motor efficiently in the steady-state. The steady-state model of the BLDC motor is helpful for motor performance evaluation. A motor manufacturer provides parameters like a no-load nominal motor constant in kilo-volt, nominal no-load current, maximum allowable current in ampere, winding resistance in ohm, a nominal no-load voltage in volt voltage, and motor weight in grams. (Lee (2022)). provides a performance map-based technique for evaluating the combined efficiency of motors, fixed pitch propellers, and airframes under a variety of operating conditions. Designers may get a complete picture of component efficiencies as well as the overall efficiency of the propulsion system. It will also be beneficial for verifying the selection of propulsion components and providing guidelines for locating better-matching components to improve overall system efficiency.
Mathematical equations of BLDC motor are a function of motor parameters given by the manufacturer, and these equations can directly evaluate the performance (Shi et al. (2017)). The modeling process ignores the armature inductance, the transient function due to switching elements, and the windage loss in the motor, thereby possibly not correctly evaluating the performance. The motor modeling aims to obtain equivalent motor voltage Vm and current Im from propeller speed N (RPM), propeller torque M (Nm), and motor parameters are given as.
where KV0 is the nominal no-load motor constant, ImMax is the maximum current, Im0 and Vm0 are the nominal no-load currents and voltages, Rm is the motor resistance, and Gm is the motor weight.
The drag coefficient is determined from parameters provided by the manufacturer (Lindahl et al. (2012)) and is used to establish the model of windage loss. Here windage loss is included in the steady-state model to evaluate a more accurate BLDC motor performance. BLDC motor is modeled with positive polynomial loss function (Duan et al. (2018)), including windage, friction, copper, and iron losses. This motor model is combined with an adjustable pitch propeller model to find the best combination of motor and propeller using the genetic algorithm. (Carev et al. (2021)). discusses a novel multilayer arrangement using three conventional stators with a single rotor body to improve torque even at low speeds. Nine inner windings can use alternative star/delta connections to maximize the BLDC motor’s performance on-demand in this arrangement.
4 Electronic Speed Controller
Figure 4 shows a conceptual diagram of an electronic speed controller with the flight controller and electronically commutated BLDC motor. The bridge inverter with six power electronic switches is directly connected with the motor to provide proper electronic commutation to the motor. Flight controller generates reference speed signals based on control algorithm as supplied to the microcontroller unit (Mogensen (2016)). The motor control motor strategy implemented in the microcontroller unit generates PWM signals to trigger the six power electronic switches appropriately. It is necessary to isolate control circuits from power circuits to protect the low voltage control circuits. So isolation can be confirmed by gate driver circuits. In addition, speed control algorithms require information related to phase currents provided by current sensors. The main idea of the ESC is to operate the BLDC motor as commanded by the flight controller unit of UAVs.
4.1 Brushless Direct Current Motor Control Strategy
Control of the aerial drone is a complex and challenging task, and so most of the directly adapted traditional control techniques for BLDC motors fail to give an adequate performance. First, the range of controlling rotational speed should be wider to address all the operating modes of multi-rotor aerial vehicles from the rest position to hovering while performing complex acrobatics in the air. Second, control techniques employed in such air vehicles must have a quick response time to perform complex maneuvers and perfect control effectively. In addition, the power consumption of the drive system is directly affected by the quality of implemented control scheme. Finally, proper and efficient control techniques lead to a more significant hovering time for battery-powered aerial vehicles.
Researchers have made significant contributions to traditional sensorless BLDC motor applications, but it is still challenging to choose the appropriate control technique for UAVs. Torque ripple, especially commutation torque ripple, is a well-known issue of BLDC motor drive. A leading cause of torque ripple is the mismatch between stator current and its non sinusoidal back-EMF waveforms, causing oscillation in low inertia motor speed, mechanical stress and vibrations, acoustic noise, and thereby limiting some complex UAV operations. Several works present torque ripple minimization strategies for BLDC motor drives in electric vehicle applications and many more. By analyzing the causes of torque ripples in the BLDC motor, suppression takes place by prediction of phase current based on trapezoidal back EMF without changing the control circuit structure (Xia et al. (2019)). The predictive control eliminates commutation torque ripple by changing the duty cycle of power switches during commutation. Generally, a simple sensorless six-step control strategy used for speed control of BLDC motor (Cabuk (2021)) develops predominant torque ripple due to phase commutation at every 60 electrical degree of rotor position de Castro et al. (2018). On the other hand, the direct torque control (DTC) technique that helps reduce the torque ripple of the BLDC motor (Khazaee et al. (2020)) drives induction machines (Bindal and Kaur (2020)), and subsequently also permanent magnet synchronous motors (PMSM) (Hang et al. (2021)).
The DTC technique as shown in Figure 5, intends to directly control electromagnetic torque and flux linkages by establishing a relationship between the flux, the torque, and optimal switching of the inverter, reducing torque pulsations. As a result, the dynamic response of this technique is better than the conventional vector control and is simpler to implement and robust to parameters variations. The actual electromagnetic torque and flux linkages are estimated from stator voltage, current data and compared with reference value flux linkages and electromagnetic torque. The errors generated are feed to hysteresis bandwidth control, giving one or −1 signal in output depending on the error values. The voltage vector table is a look-up table that generates a switching sequence based on hysteresis bandwidth controller output and sector. A position-sensorless three-phase conduction direct torque control technique for BLDC motor drives (Ozturk and Toliyat (2010)) controls not only torque but also the stator flux indirectly using d-axis current, so flux weakening operation is possible. Furthermore, PI controllers, PWM strategies, inverse park, and inverse Clarke transformation are absent and relatively simple to implement in the proposed method. The direct torque control technique performs better in transient conditions because of faster responses to changes in command speed or load torque. However, in steady-state conditions, vector control or field-oriented control technique performs very well because of lower speed ripple and power consumption. Improved finite control set model-based direct power approach for torque ripple mitigation in BLDC motor without the use of rotor position information or back EMF harmonic estimation (de Castro et al. (2018)) is experimentally compared with conventional field-oriented current control to show the effectiveness of the proposed algorithm. The algorithm is deployed in a 32-bit floating point ARM Cortex M4 processor with input signals of measured current from two phases and the speed information from an incremental encoder. From given input data, the control method returns the appropriate gate signals for the voltage source inverter. However, this method requires the rotor flux estimation, and it has issues of dc offset and initial rotor flux, causing harmonics, detection errors, etc.
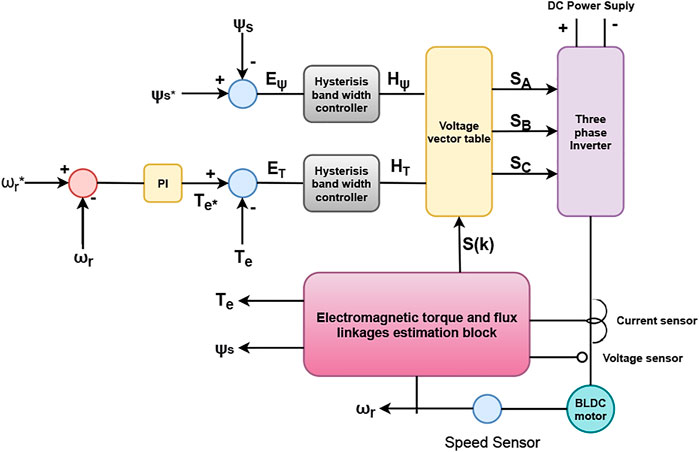
FIGURE 5. Block diagram of direct torque control and flux control (Bose (2020))
The stator winding of the BLDC motor is excited by square, sine, and non-sinusoidal harmonic current, and the influence of various currents on the performance of BLDC motor is investigated (Kshirsagar and Krishnan (2012)). Generalized back EMF expression for all excitation currents is derived to find the effect on torque ripple and harmonic loss of BLDC motor, which is helpful in efficiency evaluation. Experiments prove that a non-sinusoidal harmonic injection scheme is beneficial in speed range until the sum of stator resistive losses and conduction losses of drive exceeds iron losses of motor. When iron losses reach a significant value, the algorithm is modified to sinusoidal current control, maintaining higher efficiency operation. A non-sinusoidal harmonic injection field-oriented sensorless controller achieves this operation.
Another method to mitigate torque ripple is injecting appropriate harmonic components with optimal currents into the motor windings. Due to limited current loop bandwidth, existing techniques may fail to inject optimal currents into motor windings. A generalized vector control strategy helps reduce torque ripple of interior permanent magnet type brushless DC motor (Li et al. (2019)). A novel coordinate frame is derived based on an arbitrary reference frame to decompose the currents of the BLDC motor into two constant components: one linked with the torque and the other one is for the flux linkage. Most of the BLDC motor control techniques minimize commutation torque ripple, but minimizing machine losses is also an essential aspect of improving efficiency. An optimal current torque angle compensation for brushless DC motor helps eliminate machine losses and reduce torque ripple through a combination of conventional rotor field-oriented control and the mechanical energy conversion theory (Zhang et al. (2020)).
4.2 Power Electronic Converter
The BLDC motor, being an electronically commutated machine, is driven by six power switch bridge configurations of the voltage source inverter (Li et al. (2022)). The switching pattern is such that two switches of the same lag do not conduct simultaneously. The reliability of the BLDC motor drive improves by balancing the switching frequency of upper and lower switches of the same lag and reducing the average value of the common-mode voltage of the inverter (Masmoudi et al. (2014)). BLDC motor drive is fed by a four-switch inverter instead of six switch inverters, with one of the inverters lags replaced by two series capacitors Mani et al. (2022)) and (Ayyakrishnan (2022)). Reconfiguration reduces the number of power electronic switches, cost, switching losses, the number of interface circuits to supply logic signals, and complexity (Kivanc and Ozturk (2019); El Badsi et al. (2012); Kadhim et al. (2021); Zhu et al. (2016)). A single current sensor control strategy reduces control complexity, and cost Xia et al. (2009). BLDC motor operated in a constant torque region with four switch inverter configurations (Ozturk et al. (2009)) provides fast torque response by properly switching inverter voltage space vectors at predefined sampling time from a look-up table. In addition, a novel switching pattern helps achieve desired torque response. A current control scheme proposed for a three-phase four-switch inverter with fixed switching frequency reduces current ripple in both the typical conduction region and commutation region (Xia et al. (2016)). An efficient strategy controls an uncontrolled phase in the three-phase four-switch inverter configuration (Naseri et al. (2020)). Model predictive control adjusts the speed of the BLDC motor, and a hysteresis current controller regulates the BLDC motor phase current, reduces torque ripple, and improves transient response.
An experimental method evaluates and supports the development of fault-tolerant machines for aerospace motor drives (de Lillo et al. (2009)). Multi-phase machines, multiphase brushless dc machines specifically, were considered for fault-tolerant aerospace motor drives. Furthermore, different configurations of multiphase two-level voltage source inverters with fault tolerance capabilities provide electric motor design, including GaN-based PECs for high power density applications (Schiestl et al. (2020)). An axial flux permanent magnet machine and a GaN-based power electronic converter can create a modular multiphase drive for a quadrotor drone. In addition, the direct connection of each coil to each module helps reduce cable coupling and associated problems, eliminates losses and voltage drop. Thermal and electrical analysis validate the performance of multiphase PECs. Four three-phase inverters in the PEC provide higher power density and reliability. For high power density and high efficiency, GaN semiconductors are helpful at high switching frequencies. PECs have a significant impact on aircraft operation because they enable proper power management across electrical networks.
The most popular typologies appropriate for electric aircraft are studied for AC/DC, DC/AC, AC/AC, DC/DC, and solid-state power controllers (Dorn-Gomba et al. (2020)). Furthermore, a megawatt-scale power inverter based on a three-level active neutral-point clamped topology is developed for aircraft hybrid propulsion system (Zhang et al. (2019)). The emerging SiC-based MOSFETs help construct switching devices running at the carrier frequency in the power converter. Traditional Si-based IGBTs are used for switching devices operating at the fundamental output frequency. The PWM approach and hardware designs were given, including switching device selection, input EMI filter, and output
The flying capacitor multilevel inverter reduces power loss and weight of inverter circuits due to smaller-sized filters and compact hybrid energy transfer mechanism. Furthermore, it proposes an interleaved GaN-based inverter module for high power density electric aircraft (Pallo et al. (2018)). However, due to the high losses suffered under high temperature and voltage stress, this study emphasizes the need to consider the effect of on-resistance while developing high-performance GaN-based converters. ST microelectronics has formed various bridge inverter configurations with gate driver circuits in a single semiconductor chip, reducing oversized electronic speed controllers. The L6235 device is a DMOS fully integrated 3-phase motor driver with over-current protection. The maximum DC rating and peak current ratings are 2.8 and 5 A, respectively. Another motor driver device (L6230) has a smaller current ratio of 2.8 A, with the facility of current measurement for both six-step and field-oriented control of the BLDC motor. Texas Instruments also has developed a three Phase motor Driver (DRV8305) with Current Shunt Amplifiers and Voltage Regulator that supports a 4.4–45 V voltage supply and up to 15 A RMS (20 A peak) drive current.
More recently, voltage source inverters contain insulated gate bipolar transistors (IGBT) and metal oxide semiconductor field-effect transistors (MOSFET) based on silicon (Si) material. However, several limitations restrict the use of a Si-based inverter in drones. The efficient design of a power electronic converter helps meet desirable requirements in drone applications like less weight, small size, and maximum energy efficiency. Wide bandgap (WBG) semiconductor devices like Silicon Carbide (SiC) and Gallium Nitride (GaN) with higher bandgap energy (3.43 and 3.26 eV) than silicon (Si) (1.12 eV), withstand higher operating temperatures and have low leakage current than Si (Martinez-Heredia et al. (2020)). WBG semiconductors also have high breakdown field than Si, so that they can operate with higher voltages. For the same breakdown voltage, Sic and GaN semiconductor devices have thinner layers than Si.
Moreover, GaN has a maximum breakdown voltage of 3300 VK/cm among these semiconductors. The switching capacity of semiconductor devices depends on the Saturation velocity of semiconductor material. Table 3 provides the saturation velocity of GaN, SiC, and Si. Therefore, WBG semiconductor devices have higher saturation velocity than Si and can operate at a higher switching frequency. The efficiency of operation is mainly affected by the thermal conductivity of semiconductor devices. The temperature rises in the device for lower thermal conductivity and reduces electrons’ mobility and less efficiency. In addition, high temperatures can damage the devices and components around them.
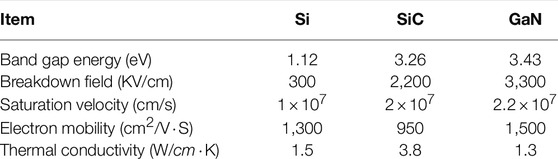
TABLE 3. Material properties of Si, SiC and GaN (Martinez-Heredia et al. (2020)).
Literature review indicates that the six power electronic switches-based bridge configuration of voltage source inverter is valid in UAVs with a six-step control strategy for low-cost drones and a field-oriented control strategy for high-performance drones. Furthermore, the Electronic speed controller controls electric motors as per flight controller requirements and is aided by various power sources, as discussed next.
5 Power Supply System in UAV
UAVs with internal combustion engines use hydrogen, gasoline, and methane as fuel (Ge et al. (2021)). The power density of fossil fuels is more than batteries and thus provides a longer endurance time and extensive payload range. Still, the efficiency of internal combustion engines is low (Austin (2011); Sharaf and Orhan (2014)) due to the multi-step process of energy production, and stability problems may arise due to fuel usage in micro air vehicles. Gas turbine engines also find use in aircraft propulsion systems because of their long endurance time and high power to weight ratio (El-Sayed (2017)). However, they perform better in higher power ranges, especially when the power demand is more than 100Hp (Austin (2011)). Because of their low efficiency, increased noise, and low fuel economy, they are not suitable for small-size UAVs (El-Sayed (2017)). On the other hand, in most of the applications, an electric motor-based propulsion system is incorporated because of several critical features like self-starting, low cast, easy automatic control, higher reliability, thermal and acoustic signatures.
It is easy to integrate an embedded structural UAV health monitoring system with an electric propulsion system, resulting in increased system reliability and lesser UAV crashes (Brown et al. (2015)). A complex sensor network is required to collect data from various parts of UAV systems about the health of those parts. Some authors have discussed hybrid propulsion system in which both thermal engines and electric motors combine key features of thermal and electric engines and balance the limitations of them (Bongermino et al. (2017); Sato et al. (2020)). Elsewhere, the hybrid design of the parallel power train finds mention (Xie et al. (2019)).
5.1 Battery Based Supplying Techniques
To supply power to the propulsion system, battery is one of the maximum usable source for UAVs application due to operating flexibility and circuit simplicity. Although, battery has less storage capacity. So some techniques are required to recharge delayed batteries through direct contact or wireless charging. Main techniques for battery charging are battery swapping, dumping, charging through laser beam technology and tethered UAVs.
5.1.1 Battery Powered UAVs
In small UAVs as well as in a medium aerial vehicle, the battery is the primary source of power (Khofiyah et al. (2018)). The use of batteries in the propulsion system improves the flexibility and simplicity of the propulsion system. UAVs can fly up to 90 min using LiPo batteries. However, battery-powered UAVs have lower endurance because of the weight of the battery pack (Verstraete et al. (2012b)). For small UAVs, lithium batteries are the best choice as a power source because of their low weight and high specific energy (Hassanalian et al. (2014)). LiPo batteries used in almost 90% of small UAVs does not exceed 2 kg weight and 100 cm length. Table 4 compares the features of four distinct battery technologies to determine the best technology for UAV applications. Comparison helps determine the best battery technology for a specific application. In (Donateo et al. (2017)) Correct calculation of flight, endurance is estimated based on battery state of charge (SoC). The battery primary power source is the best choice for low-energy applications. Reliable operation and extension of flight time are possible through optimal use of the battery by estimating the SoC in run time (Kim et al. (2020)).
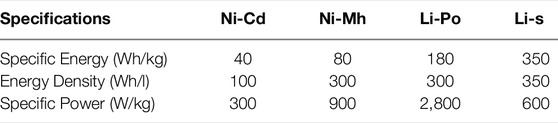
TABLE 4. Comparison of different batteries specification: specific energy, energy density, and specific power (Hassanalian et al. (2014))
Power consumption and endurance estimation models for LiPo battery-powered UAVs and experiments on commercial ones reveal specific energy models and run time (Abdilla et al., 2015). The battery model is combined with the rotor craft power model to estimate endurance. (Traub 2011). derived mathematical equations to estimate endurance based on battery discharge conditions, but they are not validated. The parameters affecting the performance of battery-powered UAVs are known. Reduced autonomy of battery-powered UAVs is a major challenge. Extensive research in battery characteristics has not yet satisfactorily extended the flight time of UAVs, thereby limiting usage in certain types of applications. In addition, improvement in energy density affects safety and stability measures (Mike 2018). As a result, batteries as the primary power source are not a viable solution for all UAVs. Different solutions balance the limitations of battery-powered UAVs. The fuel cell looks to be a powerful alternate candidate because of higher specific energy. UAVs used in long-duration missions come with multiple energy sources like batteries, solar cells, fuel cells, and supercapacitors, known as hybridized power supply systems.
5.1.2 Battery Swapping
Recharging of batteries is necessary for longer-duration missions of UAVs performed by charging or swapping the batteries. Swapping is an autonomous or human-operated method to recharge depleted batteries by the ground station during the mission. There are two main swapping techniques: “Cold” swapping and “hot” swapping (De Silva et al. (2022)). As shown in Figure 6, in “Cold” swapping, the onboard electronics of the UAV are entirely shut down during swapping, which delays the mission, and may result in loss of state information and onboard data. These issues lead to “Hot” battery swapping in which a depleted battery gets replenished with a fully charged battery while the UAV remains powered. Instead of a single UAV in operation, a swarm of UAVs is more convenient by using and controlling the cooperation such that a vehicle hands over the allotted task to a different one for continuous service to a specified mission area (Galkin et al. (2019)). Three aspects affect Swapping operation: 1) Ground station for charging/discharging batteries, 2) UAV swarm for continuous operation, 3) Operating mechanism to control UAV swarm. Automated battery swapping machines in the drone delivery network extend flight range (Cokyasar et al. (2021)) and minimize long-term delivery cost and time through linear optimization.
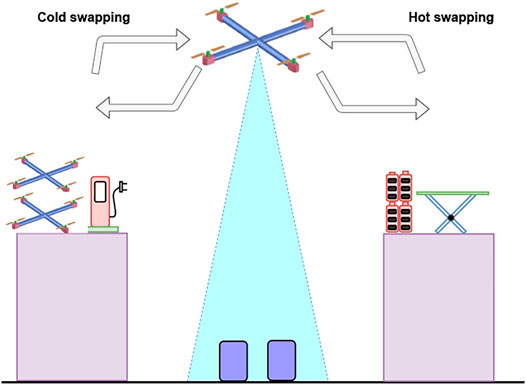
FIGURE 6. Hot swapping vs. Cold Swapping (Galkin et al. (2019)).
The battery swapping technique is useful where longer endurance is required, such as drone-based goods delivery (Cokyasar et al. (2021); Cokyasar (2021)) and a quadcopter-based prototype for long-duration missions while monitoring battery state of charge (Williams and Yakimenko (2018)). The idea is to keep one of three quadcopters always in working condition. When a battery’s state of charge drops below the threshold, the second quadcopter takes over the given task. The quadcopter with a depleted battery comes to the ground station to charge and get ready for its subsequent use. This cycle gets repeated until the mission gets completed. The number of batteries required depends on the discharging and charging time of the batteries and does not depend on the number of vehicles. The implemented system for charging batteries on the ground station requires human intervention.
Short battery life limits the uses of UAVs and has proven to be a complex problem to solve. An autonomous drone charging station with a wireless charging facility is one of the many possible solutions to this problem (Choi et al. (2016)). Figure 7 shows a conceptual representation of the ground charging station in which the DC power supply directly connects with the ground station. Ground stations for battery swapping include several components such as onboard circuits, ground electronics, battery carriage, battery receiver, landing frame, and contact mechanisms (De Silva et al. (2022); Ucgun et al. (2021)). The location of ground stations in cities must be at high-rise places such as on streetlights, cellular towers, high rise buildings, rooftops, power line poles (Hassanalian et al. (2020); Gentry et al. (2016)). A ground recharge station (Ucgun et al. (2021)) implements an algorithm to reduce battery charging duration using an autonomous charging process for a swarm of UAVs with safer electrical contact and balancer.
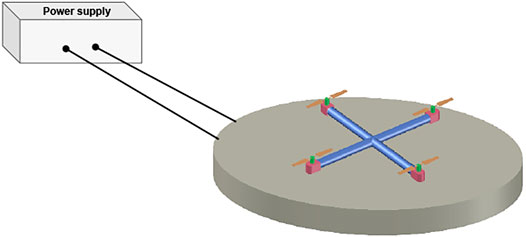
FIGURE 7. Charging stations for UAVs (Choi et al. (2016); Galimov et al. (2020)).
5.1.3 Laser-Beam Technology for Recharging
There are several limitations of the swapping technique. First, a ground station is necessary for battery charging. Second, each time UAVs have to descend to charge the batteries, it impacts mission time and system efficiency, especially at high altitudes. Due to these limitations, apart from direct contact, wireless charging (inductive coupling) (Campi et al. (2017)) is an ideal option (Galkin et al. (2019); Lu et al. (2018)) to power the UAVs. Laser beam in-flight recharging is a convenient wireless charging technique in which the beam generated at the ground station provides the necessary power. Figure 8 shows a laser beam emitted by a transmitter and directed to a photovoltaic receiver situated under UAVs (Yang et al. (2021)).
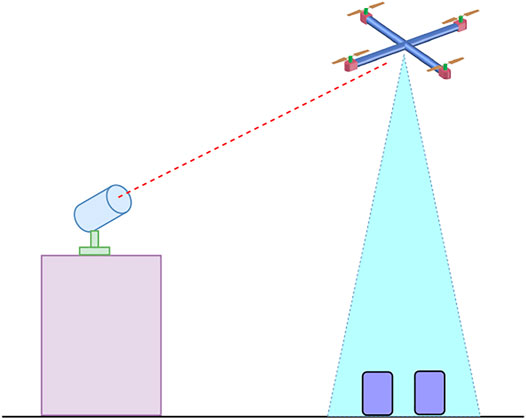
FIGURE 8. Wireless recharging of UAVs by laser beam technology (Galkin et al. (2019)).
Laser beam technology can provide significant power to UAVs for flight time of up to 12 h (do not need to turn off even at night), leading to unlimited flight endurance to overcome limitations of UAVs for specific missions (Achtelik et al. (2011)). Though it limits the range of UAVs, it is a good choice for micro rotary-wing UAVs whose range is less than 5 km anyways. However, another limitation of laser beam technology is that it can charge only one UAV simultaneously since the transmitter has to focus the laser beam towards the UAV mechanically, thus limiting the number of laser-powered UAVs in a specific mission. Low power laser source (below 1 KW) recharge UAV batteries to extend endurance time through battery level estimation (Jaafar and Yanikomeroglu (2020)) and an electrical model of motor and battery. The use of graph theory provides an optimal solution when compared with benchmark trajectory techniques.
5.1.4 Tethered UAVs
There is no need to recharge the batteries in tethered UAVs, either in the air or at the ground station, and can fly for days together. Directly connected to the ground power supply through the power line, as shown in Figure 9, such UAVs have significant autonomy. Typically, power lines are copper wires, but high-intensity and eight times lighter fiber optic cables can transmit power to UAVs in Kilowatts (Technologies (2020)) and are also effective in high altitudes, data collection applications (Xu (2021)), inspection of nuclear power plants (Gu et al. (2016)), and anti-terror drills. For example, tethered UAVs (Jiao et al. (2019)), connected to the ship, detect oil fall on the water surface, and are also helpful in air quality measurements and surveillance (Walendziuk et al. (2020)). However, such UAVs can only cover a limited area from the ground station, so the primary power sources must sometimes be transported in vehicles to cover more significant regions, although this is not a solution for many applications. In addition, the physical connection of the UAV limits the aerial vehicle from flying far away from the ground station.
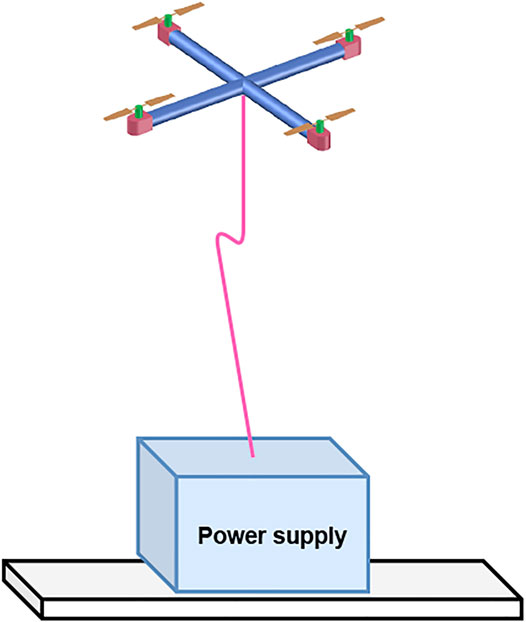
FIGURE 9. A tethered UAV (Zikou et al. (2015)).
5.2 Fuel Cell Powered UAVs
Micro fuel cells are under development and can be a good source of power in micro drones (Depcik et al. (2020)). There are various fuel cell technologies available in the market: electric storage, phosphoric acid, solid acid, proton exchange membrane, high temperature, and alkaline fuel cells. The Proton exchange membrane fuel cell (PEMFC) operation is similar to battery operation as there are two electrodes: cathode, and anode, connected through the electrolyte and separated by a membrane. A Phosphoric acid fuel cell uses hydrogen is used as fuel, and the electrolyte is phosphoric acid (Ganguly et al. (2012)). Some drawbacks of this fuel cell include absorption of phosphate ions on catalyst surface leading to difficulty in the chemical reaction, acid loss at high temperature, and corrosion at high voltage (Kundu and Dutta (2018)). The Alkaline fuel cell is one of the first developed fuel cells that use liquid potassium hydroxide as electrolyte (Thanomjit et al. (2012)). This fuel cell offers various advantages, including reduced cost, higher efficiency, and a simpler structure. Still, it also has some disadvantages like limited operational life, the need for pure oxygen, and high intolerance to carbon dioxide. (Thanomjit et al. (2012)). Statistical analysis of flight mission patterns facilitates a framework created to guide the design of fuel cell systems using a Monte Carlo-based design method (Kadyk et al. (2019)).
PEM fuel cells, direct methanol fuel cells, and solid oxide fuel cells are generally in usage in UAV applications (Gong and Verstraete (2017)). Hydrogen-based fuel cells with compressed hydrogen tanks also find usage in UAVs due to low operating temperature and rapid response to load changes, apart from high power density and lightweight, making them ideal for any electric vehicle application. Moreover, the refueling process of the fuel cell is instant compared to batteries which take a much longer time to charge Verstraete et al. (2012b). It is also important to note that specific energy of fuel cell with hydrogen tank can reach up to 1,000 wh/kg and LiPo batteries reach up to 300 wh/kg. So battery-powered vehicles hover for few minutes (Pan et al. (2019)), but fuel cell-powered vehicles can do so for few hours. For instance, fuel cell-powered UAVs are used in Mobile crane inspection (Belmonte et al. (2018)). Critical analysis of PEM fuel cells carried out from an economic and life cycle assessment viewpoint reveals higher specific energy but lower efficiency (60%) than lithium-ion batteries (90%) (Hwang et al. (2013)), thereby limiting the use of fuel cells. Auxiliary equipment is necessary for stack operation of a fuel cell which reduces fuel cell efficiency while the hydrogen generating system onboard increases system complexity. The transient characteristics of the fuel cell-powered UAV propulsion system depend on the model parameters (Zhou and Prasad (2014)). A hardware in loop (HIL) simulation with a 200 W PEM fuel cell hybrid-electric propulsion system for small-scale UAVs (Verstraete et al. (2012a)) analyzes the impact of fuel cell dynamics on mission performance and determines the behavior of different power sources in diverse operating conditions. Fuel cells with beneficial characteristics like low operating temperature, long life, high power density, no direct pollution, lightweight, and no-sound are power supplying candidates for UAVs (Gong and Verstraete (2017); Lapeña-Rey et al. (2017)). The drone design employs a hollow structure of its body to store hydrogen rather than air, eliminating the weight added by traditional hydrogen storage (Townsend et al. (2020)).
5.3 UAVs Hybrid Power Sources
A combination of two or more power sources supplies power to the electric propulsion system of UAVs. Fuel cells, batteries, solar cells, and supercapacitors may be involved.
5.3.1 Fuel Cell and Battery
Technological advancement and improvement in fuel cell performance make them an excellent power supply source. Still, there exist certain downsides when used as an independent source of power in UAVs. Auxiliary equipment of fuel cells is compressors, pumps, and valves needed for supplying fuel. All of them are mechanical devices that result in a high fuel cell time constant. Furthermore, flow delay, pump characteristics, thermodynamic behavior, and the effect of capacitance lead to slower response of fuel cell (Ou et al. (2015)). Electric propulsion is required to respond to significant variations in current during some complex maneuvers; however, fuel starvation can occur due to a considerable time constant, affecting the fuel cell’s efficiency, reliability, and life (Ou et al. (2018)). So, to avoid these limitations, battery and fuel cell are combined (Belmonte et al. (2018); Ozbek et al. (2021)). The performance of fuel cell-based hybrid propulsion systems under different UAV mission scenarios is studied (Gong et al. (2016)). Power scheduling is necessary for a hybrid supply system to improve the propulsion system performance. For example, when a UAV performs complex maneuvers such as take-off, climbing, and landing, it demands picking power from sources. In that condition, the battery acts as a primary source due to faster response, high power density, and higher efficiency than a fuel cell. Afterward, in the cruise period of UAVs, fuel cell becomes the primary source of energy.
5.3.2 Solar Cells and Supercapacitors as Auxiliary Power Sources
Batteries and many other sources cannot provide power to UAVs, especially in long endurance applications. Therefore, integrating solar energy has been a topic of interest and has received increased attention in the recent past. Solar Photovoltaic (PV) generation system is a powerful energy source candidate at high altitudes and long endurance. PV system is generally fitted on a larger wing to extract the maximum energy from sunlight and supplies power to the electric propulsion system and batteries, where the batteries provide power in night conditions or when sunlight is unavailable (Zhang et al. (2021)). A small-scale UAV with a solar-powered airframe design deployed in a mission lasts longer than a day achieving efficient flight (Morton et al. (2015)). Furthermore, different experimental tests prove that solar energy is enough to carry additional payloads and achieve longer endurance. In another instance, a solar-powered UAV saves fuel up to 59% and also reduces the weight (Harvey et al. (2012)). Furthermore, mono-crystalline silicon PV cell-based solar-powered UAV designed for environmental monitoring application flies continuously up to 6 h (Thipyopas et al. (2019)), and the effect of altitude and payload on the size and design of such UAVs are studied (Jashnani et al. (2013)). The maximum power point tracking (MPPT) technique is generally employed using DC/DC converter, current and voltage sensors, and microcontroller-based system to extract maximum power from solar irradiation (Peng et al. (2018)).
Super-capacitors are useful as auxiliary power sources of UAVs due to unique benefits such as large power density, longer life cycle, and faster charging/discharging. In addition, supercapacitors have a more comprehensive operating range of temperature, overcharging tolerance, low maintenance, almost unlimited recharge cycle, and reasonable cost (Ruan et al. (2017)). Power density refers to the amount of power that the source at a particular instance can supply. Energy density refers to the amount of energy stored by the source and how long power is deliverable. Super-capacitors have a larger power density (80 − 75,000 W/kg) but a small energy density (0.09 −0.10 Wh/kg) (Aravindan et al. (2014)), that is, the ability to supply a large amount of power for a shorter duration of time during pick power requirements. Due to lower energy density, supercapacitors are useful as auxiliary power sources in a hybrid propulsion system. A HIL-based hybrid propulsion system incorporating a battery, fuel cell, and super-capacitor outperforms a battery/fuel cell-based system (Gong et al. (2018)). The investigation of supercapacitors and fuel cells connected to a common DC bus reveals a smooth load profile and improved dynamic response. After the literature review, it is clear that supercapacitors have a predominant contribution in transient conditions and absorbing load fluctuation. However, the use of supercapacitors in UAVs is still in the early stages of development.
5.4 Power Management Strategy
Hybridization (several sources connected to a common DC bus) is an appropriate architecture for the UAV propulsion system that combines the benefits of all power sources and balances the limitations of each source by optimally splitting power among all sources to achieve high performance. Therefore, an appropriate power management strategy to distribute power demand among all energy sources with high efficiency, flight condition, batter dynamic response, and efficient fuel consumption is essential. There are two primary power management strategies: 1) Active power management strategy and 2) Passive power management strategy. Figure 10 depicts power management techniques, with the additional dotted blocks (subsystems) used only during an active power management strategy deployment. The energy management system controls the power flow of available resources through DC/DC converter and includes different sensors and microcontrollers. Passive power management strategy is in wide usage due to a simple design and ease of operation (Boukoberine et al. (2019)). According to their characteristics, power sources directly connect to a common DC bus without DC/DC converters supplying power. Furthermore, this method eliminates the need for microcontrollers, and reduces weight, cost, system complexity, and power losses.
In another instance, active and passive power management for hybrid systems, including solar cells, battery, and fuel cells, are investigated for the benefits and drawbacks through power simulation and flight test results (Lee et al. (2014)), resulting in 4.7% reduced power losses with active power management. Table 5 shows a comparison between active and passive power management strategies.
The active power management system helps split the power among available sources with predefined conditions and comes with simplicity, reliability, and meager computational cost enabling ease of implementation. Power among all available sources is assigned by considering data of battery SoC and required power. The solar PV system is regarded as the primary energy source since it does not require onboard fuel. The power management and battery management systems work together to prevent the battery from overcharging by continuously monitoring SoC. There are two DC/DC converters in power system architecture: 1) bidirectional converter with battery for charging and discharging purpose, and 2) another one operates along with fuel cell. In addition, two PI controllers are deployed to control power and voltages as per reference values. The authors have performed experiments to validate proposed power management strategy with different battery SoC initial values, but it was not tested with real UAVs. In another instance, power management strategy is employed for long endurance solar PV and battery based hybrid propulsion system (Gao et al., 2013). The whole power management strategy divides into three phases. In the first phase, the total energy produced by the PV system was distributed into three parts: 1) supplied to battery for charging, 2) motors of UAV, and stored in gravitational potential (by achieving high altitude) for usage in the second phase. In the second phase, solar irradiation decreases, and so the UAV releases gravitational potential by gliding. Finally, in the third phase, the UAV uses the battery as a power source and safely lands on the ground. The power management strategy considered the effect of wind also. Compared to the present EMS, the suggested EMS leaves around 23.5% energy in the batteries after a single day-night cycle. These types of UAVs strongly depend on solar power. Multiple batteries or a hybrid power supply system with a battery, supercapacitor and fuel cell can serve as power sources. Wang et al., 2021 propose power management as well as Soc-based droop coefficient stability analysis for distributed generation, for implementation in UAV stability and power management across all sources.
6 Challenges of Electric Propulsion System
This section discusses the challenges of electric propulsion systems for UAVs.
• Choosing control techniques for an electric motor is a challenging job because traditional methods result in excessive torque ripple, resulting in low efficiency and endurance.
• At high switching frequencies, traditional power electronic switches used in voltage source inverters produce larger losses. As a result, WBG devices are employed, but they have high design complexity.
• Lithium batteries, for example, are expensive components of electric propulsion systems. Because electric propulsion technology is still in an early stage of development, and use is limited due to the expensive development costs. The existing lithium battery’s energy density is very low, resulting in a battery weight that is too heavy to fulfill the power demand of electric propulsion UAVs. LiPo battery wireless or physical charging is also difficult. Improper charging procedures shorten the battery’s life and could cause irreparable harm.
• Battery management is a complicated task that includes planning, scheduling, and replacing a battery to complete a given mission. A precise energy management plan is needed to connect more than one power source to a common DC bus to ensure that energy is used efficiently.
• It’s tough to get UAVs to function appropriately in adverse weather. The electric propulsion system’s performance will be decreased to some extent in a complicated electromagnetic environment.
7 Conclusion
Intensive ongoing research exists in the area of UAV technologies due to a broad spectrum of UAV applications. For example, aerial vehicles used in civil and military operations require accurate and efficient control to perform the specified duty quickly and effectively. In addition, UAV designs seek longer endurance and compact size, and so we undertake comprehensive classifications of UAVs in this review paper. The appropriate design and control of an electric propulsion system can help tackle these challenging tasks. We evaluate electric motor control strategies for conventional BLDC motors in UAVs with various propellers and combination types for conventional BLDC motors. In addition, two different configurations of bridge inverter are studied with benefits and limitations to select the best configuration for UAVs. A new concept in semiconductor technology: wide-bandgap devices like GaN and SiC make electronic speed controllers more efficient and compact due to their small size, high efficiency, high switching frequency, and high reliability. Comparing different battery technologies reveals that LiPo batteries are the best choice for UAVs due to their high power and energy density, high efficiency, and longer life. However, battery-powered UAVs have lower endurance, limiting their use in some specific missions. The study of the various battery supplying techniques like swapping, laser beam in-flight charging, and tethered UAVs identifies the benefits and limitations of each. Fuel cell-powered UAVs are addressed, along with their benefits and downsides, for deployment in specific missions. A hybrid of two or more sources such as battery and fuel cell may provide longer endurance and reliable UAVs. On the other hand, supercapacitors serve as a great power source, especially in peak power demand and drastic load variations in hybrid propulsion systems. When more than two DC power sources connect to a common bus, a power management strategy must ensure efficient and reliable operation. Active and passive power management strategies help to select a powerful technique for specific UAV missions. Review of components and different control strategies of the electric propulsion system indicate possible research gaps and recommendations for feature research.
Electric propulsion systems can increase UAV performance, indicating some future scope in this field. Furthermore, the propulsion system’s electric motor can be designed for high power density and efficiency. Researchers can focus their efforts on finding the ideal motor propeller combination through detailed mathematical models of the electric motor and the propeller. Power losses in electric propulsion systems can reduce by making appropriate decisions about voltage source inverter configuration and the use of recently designed power electronic switches. Researchers can create or choose a sensorless control strategy for electric motor control that enhances propulsion system efficiency and leads to extended endurance with given energy sources. Machine learning and deep learning algorithms have recently gained tremendous popularity in various UAV-related applications such as battery scheduling. Low recharge cycles and low energy density in batteries limit the flying time of UAVs. These challenges need to be addressed in the future. Novel energy-delivery methods, such as multi-antenna energy beam-forming and distributed multi-point wireless power transfer, can be incorporated to improve charging efficiency.
Author Contributions
DJ: Investigation, Data curation, writing review, Writing-original draft. DD: planned the outlay of the review, editing, Writing-original draft. SM: supervised the development of topics and edited the final manuscript.
Conflict of Interest
The authors declare that the research was conducted in the absence of any commercial or financial relationships that could be construed as a potential conflict of interest.
Publisher’s Note
All claims expressed in this article are solely those of the authors and do not necessarily represent those of their affiliated organizations, or those of the publisher, the editors and the reviewers. Any product that may be evaluated in this article, or claim that may be made by its manufacturer, is not guaranteed or endorsed by the publisher.
References
Aasen, H., Honkavaara, E., Lucieer, A., and Zarco-Tejada, P. (2018). Quantitative Remote Sensing at Ultra-high Resolution with Uav Spectroscopy: a Review of Sensor Technology, Measurement Procedures, and Data Correction Workflows. Remote Sens. 10, 1091. doi:10.3390/rs10071091
Abdilla, A., Richards, A., and Burrow, S. (2015). “Power and Endurance Modelling of Battery-Powered Rotorcraft,” in Proceeding of the 2015 IEEE/RSJ International Conference on Intelligent Robots and Systems (IROS) (IEEE), 675–680. doi:10.1109/iros.2015.7353445
Achtelik, M. C., Stumpf, J., Gurdan, D., and Doth, K.-M. (2011). Design of a Flexible High Performance Quadcopter Platform Breaking the Mav Endurance Record with Laser Power Beaming in Proceeding of the 2011 IEEE/RSJ International Conference on Intelligent Robots and Systems (IEEE), 5166–5172.
Al-Hourani, A., and Gomez, K. (2017). Modeling Cellular-To-Uav Path-Loss for Suburban Environments. IEEE Wirel. Commun. Lett. 7, 82–85. doi:10.1109/LWC.2017.2755643
Aravindan, V., Gnanaraj, J., Lee, Y.-S., and Madhavi, S. (2014). Insertion-type Electrodes for Nonaqueous Li-Ion Capacitors. Chem. Rev. 114, 11619–11635. doi:10.1021/cr5000915
Arjomandi, M., Agostino, S., Mammone, M., Nelson, M., and Zhou, T. (2006). “Classification of Unmanned Aerial Vehicles,” in Report for Mechanical Engineering Class (Adelaide, Australia: University of Adelaide).
Austin, R. (2011). Unmanned Aircraft Systems: UAVS Design, Development and Deployment, 54. John Wiley & Sons.
Ayyakrishnan, M. (2022). Fuzzy Control Based Double Switching Fault Tolerant Control on Four Switch Voltage Source Inverters. J. Electr. Eng. Technol. 17, 1031–1038. doi:10.1007/s42835-021-00905-3
Bangura, M., Lim, H., Kim, H. J., and Mahony, R. (2014). “Aerodynamic Power Control for Multirotor Aerial Vehicles,” in Proceeding of the 2014 IEEE International Conference on Robotics and Automation (ICRA) (IEEE), 529–536. doi:10.1109/icra.2014.6906906
Bangura, M., and Mahony, R. (2017). Thrust Control for Multirotor Aerial Vehicles. IEEE Trans. Robot. 33, 390–405. doi:10.1109/tro.2016.2633562
Belmonte, N., Staulo, S., Fiorot, S., Luetto, C., Rizzi, P., and Baricco, M. (2018). Fuel Cell Powered Octocopter for Inspection of Mobile Cranes: Design, Cost Analysis and Environmental Impacts. Appl. energy 215, 556–565. doi:10.1016/j.apenergy.2018.02.072
Benarbia, T., and Kyamakya, K. (2021). A Literature Review of Drone-Based Package Delivery Logistics Systems and Their Implementation Feasibility. Sustainability 14, 360. doi:10.3390/su14010360
Bindal, R. K., and Kaur, I. (2020). Torque Ripple Reduction of Induction Motor Using Dynamic Fuzzy Prediction Direct Torque Control. ISA Trans. 99, 322–338. doi:10.1016/j.isatra.2019.09.012
Bongermino, E., Mastrorocco, F., Tomaselli, M., Monopoli, V. G., and Naso, D. (2017). “Model and Energy Management System for a Parallel Hybrid Electric Unmanned Aerial Vehicle,” in Proceeding of the 2017 IEEE 26th International Symposium on Industrial Electronics (ISIE) (IEEE), 1868–1873. doi:10.1109/isie.2017.8001534
Boukoberine, M. N., Zhou, Z., and Benbouzid, M. (2019). A Critical Review on Unmanned Aerial Vehicles Power Supply and Energy Management: Solutions, Strategies, and Prospects. Appl. Energy 255, 113823. doi:10.1016/j.apenergy.2019.113823
Brooke-Holland, L. (2012). Unmanned Aerial Vehicles (Drones): An Introduction. London, UK: House of Commons Library.
Brown, J. M., Coffey, J. A., Harvey, D., and Thayer, J. M. (2015). “Characterization and Prognosis of Multirotor Failures,” in Structural Health Monitoring and Damage Detection (Springer), 7, 157–173. doi:10.1007/978-3-319-15230-1_15
Çabuk, A. S. (2021). Sensorless Control of Outer Rotor Brushless Dc Motor with Back-Emf Observer for Drone. Balkan J. Electr. Comput. Eng. 9, 379–385. doi:10.17694/bajece.958760
Cai, G., Dias, J., and Seneviratne, L. (2014). A Survey of Small-Scale Unmanned Aerial Vehicles: Recent Advances and Future Development Trends. Un. Sys. 02, 175–199. doi:10.1142/s2301385014300017
Campi, T., Cruciani, S., Feliziani, M., and Maradei, F. (2017). “High Efficiency and Lightweight Wireless Charging System for Drone Batteries,” in Proceeding of the 2017 AEIT International Annual Conference (IEEE), 1–6. doi:10.23919/aeit.2017.8240539
Carev, V., Roháč, J., Šipoš, M., and Schmirler, M. (2021). A Multilayer Brushless Dc Motor for Heavy Lift Drones. Energies 14, 2504. doi:10.3390/en14092504
Carey, K. D., Zimmerman, N., and Ababei, C. (2018). Hybrid Field Oriented and Direct Torque Control for Sensorless Bldc Motors Used in Aerial Drones. IET Power Electron. 12, 438–449. doi:10.1049/iet-pel.2018.5231
Cavcar, M. (2000). The International Standard Atmosphere (Isa), 30. Turkey: Anadolu University, 1–6.
Choi, C. H., Jang, H. J., Lim, S. G., Lim, H. C., Cho, S. H., and Gaponov, I. (2016). “Automatic Wireless Drone Charging Station Creating Essential Environment for Continuous Drone Operation,” in Proceeding of the 2016 International Conference on Control, Automation and Information Sciences (ICCAIS) (IEEE), 132–136. doi:10.1109/iccais.2016.7822448
Christiansen, F., Rojano-Doñate, L., Madsen, P. T., and Bejder, L. (2016). Noise Levels of Multi-Rotor Unmanned Aerial Vehicles with Implications for Potential Underwater Impacts on Marine Mammals. Front. Mar. Sci. 3, 277. doi:10.3389/fmars.2016.00277
Cokyasar, T., Dong, W., Jin, M., and Verbas, İ. Ö. (2021). Designing a Drone Delivery Network with Automated Battery Swapping Machines. Comput. Operations Res. 129, 105177. doi:10.1016/j.cor.2020.105177
Cokyasar, T. (2021). Optimization of Battery Swapping Infrastructure for E-Commerce Drone Delivery. Comput. Commun. 168, 146–154. doi:10.1016/j.comcom.2020.12.015
de Castro, A. G., Pereira, W. C. A., de Almeida, T. E. P., de Oliveira, C. M. R., Roberto Boffino de Almeida Monteiro, J., and de Oliveira, A. A. (2018). Improved Finite Control-Set Model-Based Direct Power Control of Bldc Motor with Reduced Torque Ripple. IEEE Trans. Ind. Appl. 54, 4476–4484. doi:10.1109/tia.2018.2835394
De Haas, T., Nijland, W., McArdell, B. W., and Kalthof, M. W. (2021). Case Report: Optimization of Topographic Change Detection with Uav Structure-From-Motion Photogrammetry through Survey Co-alignment. Front. Remote Sens. 2, 5. doi:10.3389/frsen.2021.626810
De Jong, S. M. (2020). Grand Challenges in Earth Observation Using Unpiloted Airborne Systems. Front. Remote Sens. 1, 1. doi:10.3389/frsen.2020.601737
de Lillo, L., Empringham, L., Wheeler, P. W., Khwan-On, S., Gerada, C., Othman, M. N., et al. (2009). Multiphase Power Converter Drive for Fault-Tolerant Machine Development in Aerospace Applications. IEEE Trans. Industrial Electron. 57, 575–583. doi:10.1109/TIE.2009.2036026
De Silva, S. C., Phlernjai, M., Rianmora, S., and Ratsamee, P. (2022). Inverted Docking Station: A Conceptual Design for a Battery-Swapping Platform for Quadrotor Uavs. Drones 6, 56. doi:10.3390/drones6030056
De Wagter, C., Remes, B., Smeur, E., van Tienen, F., Ruijsink, R., van Hecke, K., et al. (2021). The Nederdrone: A Hybrid Lift, Hybrid Energy Hydrogen Uav. Int. J. hydrogen energy 46, 16003–16018. doi:10.1016/j.ijhydene.2021.02.053
Depcik, C., Cassady, T., Collicott, B., Burugupally, S. P., Li, X., Alam, S. S., et al. (2020). Comparison of Lithium Ion Batteries, Hydrogen Fueled Combustion Engines, and a Hydrogen Fuel Cell in Powering a Small Unmanned Aerial Vehicle. Energy Convers. Manag. 207, 112514. doi:10.1016/j.enconman.2020.112514
Donateo, T., Ficarella, A., Spedicato, L., Arista, A., and Ferraro, M. (2017). A New Approach to Calculating Endurance in Electric Flight and Comparing Fuel Cells and Batteries. Appl. energy 187, 807–819. doi:10.1016/j.apenergy.2016.11.100
Donateo, T., and Spedicato, L. (2017). Fuel Economy of Hybrid Electric Flight. Appl. energy 206, 723–738. doi:10.1016/j.apenergy.2017.08.229
Dorn-Gomba, L., Ramoul, J., Reimers, J., and Emadi, A. (2020). Power Electronic Converters in Electric Aircraft: Current Status, Challenges, and Emerging Technologies. IEEE Trans. Transp. Electrification 6, 1648–1664. doi:10.1109/tte.2020.3006045
Duan, D., Wang, Z., Wang, Q., and Li, J. (2020). Research on Integrated Optimization Design Method of High-Efficiency Motor Propeller System for Uavs with Multi-States. IEEE Access 8, 165432–165443. doi:10.1109/access.2020.3014411
Duan, D., Zhao, H., Peng, M., and Li, J. (2018). “Research on Optimal Design Method of Tilt-Rotor Electric Propulsion System,” in Asia-Pacific International Symposium on Aerospace Technology (Springer), 1108–1119.
El Badsi, B., Bouzidi, B., and Masmoudi, A. (2012). Dtc Scheme for a Four-Switch Inverter-Fed Induction Motor Emulating the Six-Switch Inverter Operation. IEEE Trans. power Electron. 28, 3528–3538. doi:10.1109/TPEL.2012.2225449
El-Sayed, A. F. (2017). “Turbofan Engines,” in Aircraft Propulsion and Gas Turbine Engines (Boca Raton: CRC Press), 289–424.
Elkerdany, M. S., Safwat, I. M., Yossef, A. M. M., and Elkhatib, M. M. (2020). “A Comparative Study on Using Brushless Dc Motor Six-Switch and Four-Switch Inverter for Uav Propulsion System,” in Proceeding of the 2020 12th International Conference on Electrical Engineering (ICEENG) (IEEE), 58–61. doi:10.1109/iceeng45378.2020.9171757
Galimov, M., Fedorenko, R., and Klimchik, A. (2020). Uav Positioning Mechanisms in Landing Stations: Classification and Engineering Design Review. Sensors 20, 3648. doi:10.3390/s20133648
Galkin, B., Kibilda, J., and DaSilva, L. A. (2019). Uavs as Mobile Infrastructure: Addressing Battery Lifetime. IEEE Commun. Mag. 57, 132–137. doi:10.1109/mcom.2019.1800545
Ganguly, S., Das, S., Kargupta, K., and Bannerjee, D. (2012). Optimization of Performance of Phosphoric Acid Fuel Cell (Pafc) Stack Using Reduced Order Model with Integrated Space Marching and Electrolyte Concentration Inferencing. Comput. Aided Chem. Eng. 31, 1010–1014. doi:10.1016/b978-0-444-59506-5.50033-x
Gao, X.-Z., Hou, Z.-X., Guo, Z., Liu, J.-X., and Chen, X.-Q. (2013). Energy Management Strategy for Solar-Powered High-Altitude Long-Endurance Aircraft. Energy Convers. Manag. 70, 20–30. doi:10.1016/j.enconman.2013.01.007
Ge, J., Liu, L., Dong, X., and He, Y. (2021). A Trajectory Optimization Method for Reducing Magnetic Disturbance of an Internal Combustion Engine Powered Unmanned Aerial Vehicle. Aerosp. Sci. Technol. 116, 106885. doi:10.1016/j.ast.2021.106885
Gentry, N. K., Hsieh, R., and Nguyen, L. K. (2016). Multi-use Uav Docking Station Systems and Methods. Seattle, WA, United States. US Patent.
Gong, A., MacNeill, R., Verstraete, D., and Palmer, J. L. (2018). “Analysis of a Fuel-Cell/battery/supercapacitor Hybrid Propulsion System for a Uav Using a Hardware-In-The-Loop Flight Simulator,” in Proceeding of the 2018 AIAA/IEEE Electric Aircraft Technologies Symposium (EATS) (IEEE), 1–17. doi:10.2514/6.2018-5017
Gong, A., Palmer, J. L., Brian, G., Harvey, J. R., and Verstraete, D. (2016). Performance of a Hybrid, Fuel-Cell-Based Power System during Simulated Small Unmanned Aircraft Missions. Int. J. hydrogen energy 41, 11418–11426. doi:10.1016/j.ijhydene.2016.04.044
Gong, A., and Verstraete, D. (2017). Fuel Cell Propulsion in Small Fixed-Wing Unmanned Aerial Vehicles: Current Status and Research Needs. Int. J. hydrogen energy 42, 21311–21333. doi:10.1016/j.ijhydene.2017.06.148
Gu, B. W., Choi, S. Y., Choi, Y. S., Cai, G., Seneviratne, L., and Rim, C. T. (2016). Novel Roaming and Stationary Tethered Aerial Robots for Continuous Mobile Missions in Nuclear Power Plants. Nucl. Eng. Technol. 48, 982–996. doi:10.1016/j.net.2016.02.014
Gupta, G., and Abdallah, S. (2018). Propeller Force-Constant Modeling for Multirotor Uavs from Experimental Estimation of Inflow Velocity. Int. J. Aerosp. Eng. 2018, 9632942. doi:10.1155/2018/9632942
Han, X., Wei, Z., Chen, H., Zhang, B., Li, Y., and Du, T. (2021). Inversion of Winter Wheat Growth Parameters and Yield under Different Water Treatments Based on Uav Multispectral Remote Sensing. Front. plant Sci. 12, 639. doi:10.3389/fpls.2021.609876
Hang, J., Ding, S., Ren, X., Hu, Q., Huang, Y., Hua, W., et al. (2021). Integration of Interturn Fault Diagnosis and Torque Ripple Minimization Control for Direct-Torque-Controlled Spmsm Drive System. IEEE Trans. Power Electron. 36, 11124–11134. doi:10.1109/tpel.2021.3073774
Harvey, J. R., Jarvis, R. A., Verstraete, D., Bagg, R. L., Honnery, D., and Palmer, J. L. (2012). “Development of a Hybrid-Electric Power-System Model for a Small Surveillance Aircraft,” in 28th International Congress of the Aeronautical Sciences.
Hassanalian, M., Mirzaeinia, A., and Lee, K. (2020). “Smart Cities and Organizing the Drones’ Applications in Urban Areas: Ne St (Networking, Efficient, Strategies),” in Proceeding of the AIAA Scitech 2020 Forum. 1944.
Hassanalian, M., Radmanesh, M., and Sedaghat, A. (2014). Increasing Flight Endurance of Mavs Using Multiple Quantum Well Solar Cells. Int. J. Aeronautical Space Sci. 15, 212–217. doi:10.5139/ijass.2014.15.2.212
Hwang, J.-J., Kuo, J.-K., Wu, W., Chang, W.-R., Lin, C.-H., and Wang, S.-E. (2013). Lifecycle Performance Assessment of Fuel Cell/battery Electric Vehicles. Int. J. hydrogen energy 38, 3433–3446. doi:10.1016/j.ijhydene.2012.12.148
Jaafar, W., and Yanikomeroglu, H. (2020). Dynamics of Laser-Charged Uavs: A Battery Perspective. IEEE Internet Things J. 8, 10573–10582. doi:10.1109/JIOT.2020.3048087
Jaeger, M., and Adair, D. (2017). Conceptual Design of a High-Endurance Hybrid Electric Unmanned Aerial Vehicle. Mater. Today Proc. 4, 4458–4468. doi:10.1016/j.matpr.2017.04.018
Jashnani, S., Nada, T., Ishfaq, M., Khamker, A., and Shaholia, P. (2013). Sizing and Preliminary Hardware Testing of Solar Powered Uav. Egypt. J. Remote Sens. Space Sci. 16, 189–198. doi:10.1016/j.ejrs.2013.05.002
Jenkins, D., and Vasigh, B. (2013). The Economic Impact of Unmanned Aircraft Systems Integration in the United States (Association for Unmanned Vehicle Systems International (AUVSI)).
Jiao, Z., Jia, G., and Cai, Y. (2019). A New Approach to Oil Spill Detection that Combines Deep Learning with Unmanned Aerial Vehicles. Comput. Industrial Eng. 135, 1300–1311. doi:10.1016/j.cie.2018.11.008
Johnson, W. (1980). Helicopter Theory. Princeton, New Jersey, USA: Helicopter theoryprinceton university press.
Kadhim, Q. S., Abbas, A. H., and Ezzaldean, M. M. (2021). Optimized Sliding Mode Control of Three-phase Four-Switch Inverter Bldc Motor Drive Using Lfd Algorithm. Iraqi J. Electr. Electron. Eng. 17. doi:10.37917/ijeee.17.2.15
Kadyk, T., Schenkendorf, R., Hawner, S., Yildiz, B., and Römer, U. (2019). Design of Fuel Cell Systems for Aviation: Representative Mission Profiles and Sensitivity Analyses. Front. Energy Res. 7, 35. doi:10.3389/fenrg.2019.00035
Khazaee, A., Zarchi, H. A., Markadeh, G. A., and Hesar, H. M. (2020). Mtpa Strategy for Direct Torque Control of Brushless Dc Motor Drive. IEEE Trans. Industrial Electron. 68, 6692–6700. doi:10.1109/TIE.2020.3009576
Khofiyah, N. A., Maret, S., Sutopo, W., and Nugroho, B. D. A. (2018). “Goldsmith’s Commercialization Model for Feasibility Study of Technology Lithium Battery Pack Drone,” in Proceeding of the 2018 5th International Conference on Electric Vehicular Technology (ICEVT) (IEEE), 147–151.
Kim, J., Choi, Y., Jeon, S., Kang, J., and Cha, H. (2020). Optrone: Maximizing Performance and Energy Resources of Drone Batteries. IEEE Trans. Computer-Aided Des. Integr. Circuits Syst. 39, 3931–3943. doi:10.1109/tcad.2020.3012790
Kivanc, O. C., and Ozturk, S. B. (2019). Low-cost Position Sensorless Speed Control of Pmsm Drive Using Four-Switch Inverter. Energies 12, 741. doi:10.3390/en12040741
Krishnan, R. (2017). Permanent Magnet Synchronous and Brushless DC Motor Drives. Boca Raton: CRC Press.
Kshirsagar, P., and Krishnan, R. (2012). High-efficiency Current Excitation Strategy for Variable-Speed Nonsinusoidal Back-Emf Pmsm Machines. IEEE Trans. Industry Appl. 48, 1875–1889. doi:10.1109/tia.2012.2225814
Lapeña-Rey, N., Blanco, J., Ferreyra, E., Lemus, J., Pereira, S., and Serrot, E. (2017). A Fuel Cell Powered Unmanned Aerial Vehicle for Low Altitude Surveillance Missions. Int. J. Hydrogen Energy 42, 6926–6940. doi:10.1016/j.ijhydene.2017.01.137
Lee, B., Kwon, S., Park, P., and Kim, K. (2014). Active Power Management System for an Unmanned Aerial Vehicle Powered by Solar Cells, a Fuel Cell, and Batteries. IEEE Trans. Aerosp. Electron. Syst. 50, 3167–3177. doi:10.1109/taes.2014.130468
Lee, D., La, W. G., and Kim, H. (2018). “Drone Detection and Identification System Using Artificial Intelligence,” in Proceeding of the 2018 International Conference on Information and Communication Technology Convergence (ICTC) (IEEE), 1131–1133. doi:10.1109/ictc.2018.8539442
Lee, H.-t. (2022). “A Technique for Matching Propeller, Motor, and Airframe of an Electric Powered Aircraft Based on Efficiency Maps,” in Proceeding of the AIAA SCITECH 2022 Forum. 0885. doi:10.2514/6.2022-0885
Li, X., Li, Z., Wang, H., and Li, W. (2021). Unmanned Aerial Vehicle for Transmission Line Inspection: Status, Standardization, and Perspectives. Front. Energy Res. 9, 336. doi:10.3389/fenrg.2021.713634
Li, X., Yuan, H., Chen, W., Yu, L., and Gu, X. (2022). Commutation Torque Ripple Reduction Strategy of Brushless Dc Motor Drives Based on Boosting Voltage of Dc-Link Small Capacitor. Micromachines 13, 226. doi:10.3390/mi13020226
Li, Z., Wang, J., Zhou, L., Liu, X., and Jiang, F. (2019). Enhanced Generalized Vector Control Strategy for Torque Ripple Mitigation of Ipm-type Brushless Dc Motors. IEEE Trans. Power Electron. 34, 12038–12049. doi:10.1109/tpel.2019.2906247
Lindahl, P., Moog, E., and Shaw, S. R. (2012). Simulation, Design, and Validation of an Uav Sofc Propulsion System. IEEE Trans. Aerosp. Electron. Syst. 48, 2582–2593. doi:10.1109/TAES.2012.6237610
Lu, M., Bagheri, M., James, A. P., and Phung, T. (2018). Wireless Charging Techniques for Uavs: A Review, Reconceptualization, and Extension. IEEE Access 6, 29865–29884. doi:10.1109/access.2018.2841376
Luque-Vega, L. F., Castillo-Toledo, B., Loukianov, A., and Gonzalez-Jimenez, L. E. (2014). “Power Line Inspection via an Unmanned Aerial System Based on the Quadrotor Helicopter,” in MELECON 2014-2014 17th IEEE Mediterranean Electrotechnical Conference (IEEE), 393–397. doi:10.1109/melcon.2014.6820566
Maharajan, M. P., and Xavier, S. A. E. (2018). Design of Speed Control and Reduction of Torque Ripple Factor in Bldc Motor Using Spider Based Controller. IEEE Trans. Power Electron. 34, 7826–7837. doi:10.1109/TPEL.2018.2880916
Mani, P., Mahadevan, S. K., Johnson, A. R., and Kullan, M. (2022). An Optimized Design Modelling of Pv Integrated Sepic-Based Four-Switch Inverter for Sensorless Pmbldc Motor Control. Automatika 63, 90–101. doi:10.1080/00051144.2021.2008621
Martinez-Heredia, J. M., Colodro, F., Mora-Jiménez, J. L., Remujo, A., Soriano, J., and Esteban, S. (2020). Development of gan Technology-Based Dc/dc Converter for Hybrid Uav. IEEE Access 8, 88014–88025. doi:10.1109/access.2020.2992913
Masmoudi, M., El Badsi, B., and Masmoudi, A. (2014). Direct Torque Control of Brushless Dc Motor Drives with Improved Reliability. IEEE Trans. Industry Appl. 50, 3744–3753. doi:10.1109/tia.2014.2313700
McDonald, R. A. (2014). “Electric Propulsion Modeling for Conceptual Aircraft Design,” in 52nd Aerospace Sciences Meeting. 0536. doi:10.2514/6.2014-0536
Mike, R. (2018). Whitepaper: Fuel Cell-Based Energy Systems for Commercial Uavs. UK: Unmanned Systems Technology.
Moffitt, B., Bradley, T., Parekh, D., and Mavris, D. (2008). “Validation of Vortex Propeller Theory for Uav Design with Uncertainty Analysis,” in Proceeding of the 46th AIAA Aerospace Sciences Meeting and Exhibit, 406. doi:10.2514/6.2008-406
Mogensen, K. N. (2016). Motor-Control Considerations for Electronic Speed Control in Drones. Analog Applications Journal.
Mohan, M., Richardson, G., Gopan, G., Aghai, M. M., Bajaj, S., Galgamuwa, G., et al. (2021). Uav-supported Forest Regeneration: Current Trends, Challenges and Implications. Remote Sens. 13, 2596. doi:10.3390/rs13132596
Morton, S., D’Sa, R., and Papanikolopoulos, N. (2015). “Solar Powered Uav: Design and Experiments,” in Proceeding of the 2015 IEEE/RSJ international conference on intelligent robots and systems (IROS) (IEEE), 2460–2466. doi:10.1109/iros.2015.7353711
Mukhamediev, R. I., Symagulov, A., Kuchin, Y., Zaitseva, E., Bekbotayeva, A., Yakunin, K., et al. (2021). Review of Some Applications of Unmanned Aerial Vehicles Technology in the Resource-Rich Country. Appl. Sci. 11, 10171. doi:10.3390/app112110171
Naseri, F., Farjah, E., Schaltz, E., Lu, K., and Tashakor, N. (2020). “Predictive Control of Low-Cost Three-phase Four-Switch Inverter-Fed Drives for Brushless Dc Motor Applications,” in IEEE Transactions on Circuits and Systems I: Regular Papers.
Ou, K., Wang, Y.-X., Li, Z.-Z., Shen, Y.-D., and Xuan, D.-J. (2015). Feedforward Fuzzy-Pid Control for Air Flow Regulation of Pem Fuel Cell System. Int. J. hydrogen energy 40, 11686–11695. doi:10.1016/j.ijhydene.2015.04.080
Ou, K., Yuan, W.-W., Choi, M., Yang, S., Jung, S., and Kim, Y.-B. (2018). Optimized Power Management Based on Adaptive-Pmp Algorithm for a Stationary Pem Fuel Cell/battery Hybrid System. Int. J. hydrogen energy 43, 15433–15444. doi:10.1016/j.ijhydene.2018.06.072
Outay, F., Mengash, H. A., and Adnan, M. (2020). Applications of Unmanned Aerial Vehicle (Uav) in Road Safety, Traffic and Highway Infrastructure Management: Recent Advances and Challenges. Transp. Res. part A policy Pract. 141, 116–129. doi:10.1016/j.tra.2020.09.018
Ozbek, E., Yalin, G., Karaoglan, M. U., Ekici, S., Colpan, C. O., and Karakoc, T. H. (2021). Architecture Design and Performance Analysis of a Hybrid Hydrogen Fuel Cell System for Unmanned Aerial Vehicle. Int. J. Hydrogen Energy 46, 16453–16464. doi:10.1016/j.ijhydene.2020.12.216
Ozturk, S. B., Alexander, W. C., and Toliyat, H. A. (2009). Direct Torque Control of Four-Switch Brushless Dc Motor with Non-sinusoidal Back Emf. IEEE Trans. Power Electron. 25, 263–271. doi:10.1109/TPEL.2009.2028888
Ozturk, S. B., and Toliyat, H. A. (2010). Direct Torque and Indirect Flux Control of Brushless Dc Motor. IEEE/ASME Trans. Mechatronics 16, 351–360. doi:10.1109/TMECH.2010.2043742
Pallo, N., Foulkes, T., Modeer, T., Coday, S., and Pilawa-Podgurski, R. (2018). “Power-dense Multilevel Inverter Module Using Interleaved gan-based Phases for Electric Aircraft Propulsion,” in Proceeding of the 2018 IEEE Applied Power Electronics Conference and Exposition (APEC) (IEEE), 1656–1661. doi:10.1109/apec.2018.8341239
Pan, Z., An, L., and Wen, C. (2019). Recent Advances in Fuel Cells Based Propulsion Systems for Unmanned Aerial Vehicles. Appl. Energy 240, 473–485. doi:10.1016/j.apenergy.2019.02.079
Panagiotou, P., Fotiadis-Karras, S., and Yakinthos, K. (2018). Conceptual Design of a Blended Wing Body Male Uav. Aerosp. Sci. Technol. 73, 32–47. doi:10.1016/j.ast.2017.11.032
Peng, L., Zheng, S., Chai, X., and Li, L. (2018). A Novel Tangent Error Maximum Power Point Tracking Algorithm for Photovoltaic System under Fast Multi-Changing Solar Irradiances. Appl. energy 210, 303–316. doi:10.1016/j.apenergy.2017.11.017
Pillay, P., and Krishnan, R. (1989). Modeling, Simulation, and Analysis of Permanent-Magnet Motor Drives. Ii. The Brushless Dc Motor Drive. IEEE Trans. Industry Appl. 25, 274–279. doi:10.1109/28.25542
Rosser, J. C, , Vignesh, V., Terwilliger, B. A., and Parker, B. C. (2018). Surgical and Medical Applications of Drones: A Comprehensive Review. JSLS J. Soc. Laparoendosc. Surg. 22. doi:10.4293/JSLS.2018.00018
Ruan, J., Walker, P. D., Zhang, N., and Wu, J. (2017). An Investigation of Hybrid Energy Storage System in Multi-Speed Electric Vehicle. Energy 140, 291–306. doi:10.1016/j.energy.2017.08.119
Sai, P. G., Rani, C. S., and Nelakuditi, U. R. (2018). Implementation of Power Optimization Technique for Uavs. Mater. Today Proc. 5, 132–137. doi:10.1016/j.matpr.2017.11.063
Sato, M., Nirei, M., Yamanaka, Y., Suzuki, T., Bu, Y., and Mizuno, T. (2020). Increasing the Efficiency of a Drone Motor by Arranging Magnetic Sheets to Windings. Energy Rep. 6, 439–446. doi:10.1016/j.egyr.2019.11.100
Schiestl, M., Marcolini, F., Incurvati, M., Capponi, F. G., Stärz, R., Caricchi, F., et al. (2020). Development of a High Power Density Drive System for Unmanned Aerial Vehicles. IEEE Trans. Power Electron. 36, 3159–3171. doi:10.1109/TPEL.2020.3013899
Sharaf, O. Z., and Orhan, M. F. (2014). An Overview of Fuel Cell Technology: Fundamentals and Applications. Renew. Sustain. energy Rev. 32, 810–853. doi:10.1016/j.rser.2014.01.012
Shi, D., Dai, X., Zhang, X., and Quan, Q. (2017). A Practical Performance Evaluation Method for Electric Multicopters. IEEE/ASME Trans. Mechatronics 22, 1337–1348. doi:10.1109/tmech.2017.2675913
Sivakumar, M., and Naga Malleswari, T. Y. J. (2021). A Literature Survey of Unmanned Aerial Vehicle Usage for Civil Applications. J. Aerosp. Technol. Manag. 13. doi:10.1590/jatm.v13.1233
Surekha, P., Venu, N., Shetty, A. N., and Sachan, O. (2020). “An Automatic Drone to Survey Orchards Using Image Processing and Solar Energy,” in Proceeding of the 2020 IEEE 17th India Council International Conference (INDICON) (IEEE), 1–7.
Tao, L., Zhou, Y., Zicun, L., and Zhang, X. (2019). State of Art on Energy Management Strategy for Hybrid-Powered Unmanned Aerial Vehicle. Chin. J. Aeronautics 32, 1488–1503. doi:10.1016/j.cja.2019.03.013
Technologies, P. L. (2020). Tethered Uavs. Available at: https://powerlighttech.com/power-over-fiber-case-study-aerial-applications (Accessed 04 06 2021)
Thanomjit, C., Patcharavorachot, Y., and Arpornwichanop, A. (2012). Design and Thermal Analysis of a Solid Oxide Fuel Cell System Integrated with Ethanol Steam Reforming. Comput. Aided Chem. Eng. 30, 287–291. doi:10.1016/b978-0-444-59519-5.50058-7
Thipyopas, C., Sripawadkul, V., and Warin, N. (2019). “Design and Development of a Small Solar-Powered Uav for Environmental Monitoring Application,” in Proceeding of the 2019 IEEE Eurasia Conference on IOT, Communication and Engineering (ECICE) (IEEE), 316–319. doi:10.1109/ecice47484.2019.8942777
Tjhai, C. (2013). Developing Stochastic Model of Thrust and Flight Dynamics for Small UAVs. Minnetonka: University of Minnesota. Ph.D. thesis.
Townsend, A., Jiya, I. N., Martinson, C., Bessarabov, D., and Gouws, R. (2020). A Comprehensive Review of Energy Sources for Unmanned Aerial Vehicles, Their Shortfalls and Opportunities for Improvements. Heliyon 6, e05285. doi:10.1016/j.heliyon.2020.e05285
Traub, L. W. (2011). Range and Endurance Estimates for Battery-Powered Aircraft. J. Aircr. 48, 703–707. doi:10.2514/1.c031027
Tripicchio, P., Satler, M., Dabisias, G., Ruffaldi, E., and Avizzano, C. A. (2015). “Towards Smart Farming and Sustainable Agriculture with Drones,” in Proceeding of the 2015 International Conference on Intelligent Environments (IEEE), 140–143. doi:10.1109/ie.2015.29
Ucgun, H., Yuzgec, U., and Bayilmis, C. (2021). A Review on Applications of Rotary-Wing Unmanned Aerial Vehicle Charging Stations. Int. J. Adv. Robotic Syst. 18, 17298814211015863. doi:10.1177/17298814211015863
Valavanis, K. P., and Vachtsevanos, G. J. (2015). Handbook of Unmanned Aerial Vehicles, 2077. Springer.
Verstraete, D., Harvey, J. R., and Palmer, J. L. (2012a). “Hardware-in-the-loop Simulation of Fuel-Cell-Based Hybrid-Electrical Uav Propulsion,” in Proceedings of the 28th Congress of the International Council of the Aeronautical Sciences, 4, 2662–2674.
Verstraete, D., Lehmkuehler, K., and Wong, K. (2012b). “Design of a Fuel Cell Powered Blended Wing Body Uav,” in ASME International Mechanical Engineering Congress and Exposition (American Society of Mechanical Engineers (ASME)), 45172, 621–629. doi:10.1115/imece2012-88871
Walendziuk, W., Falkowski, P., and Kulikowski, K. (2020). “The Analysis of Power Supply Topologies for Tethered Drone Applications,” in Multidisciplinary Digital (Bialystok, Poland: Publishing Institute Proceedings), 51, 25. doi:10.3390/proceedings2020051025
Wang, R., Sun, Q., Hu, W., Li, Y., Ma, D., and Wang, P. (2021). Soc-based Droop Coefficients Stability Region Analysis of the Battery for Stand-Alone Supply Systems with Constant Power Loads. IEEE Trans. Power Electron. 36, 7866–7879. doi:10.1109/tpel.2021.3049241
Watts, A. C., Ambrosia, V. G., and Hinkley, E. A. (2012). Unmanned Aircraft Systems in Remote Sensing and Scientific Research: Classification and Considerations of Use. Remote Sens. 4, 1671–1692. doi:10.3390/rs4061671
Williams, A., and Yakimenko, O. (2018). “Persistent Mobile Aerial Surveillance Platform Using Intelligent Battery Health Management and Drone Swapping,” in Proceeding of the 2018 4th International Conference on Control, Automation and Robotics (ICCAR) (IEEE), 237–246. doi:10.1109/iccar.2018.8384677
Woźniak, W., and Jessa, M. (2021). Selection of Solar Powered Unmanned Aerial Vehicles for a Long Range Data Acquisition Chain. Sensors 21, 2772. doi:10.3390/s21082772
Xia, C., Li, Z., and Shi, T. (2009). A Control Strategy for Four-Switch Three-phase Brushless Dc Motor Using Single Current Sensor. IEEE Trans. Industrial Electron. 56, 2058–2066. doi:10.1109/tie.2009.2014307
Xia, C., Wu, D., Shi, T., and Chen, W. (2016). A Current Control Scheme of Brushless Dc Motors Driven by Four-Switch Three-phase Inverters. IEEE J. Emerg. Sel. Top. Power Electron. 5, 547–558. doi:10.1109/JESTPE.2016.2637383
Xia, K., Ye, Y., Ni, J., Wang, Y., and Xu, P. (2019). Model Predictive Control Method of Torque Ripple Reduction for Bldc Motor. IEEE Trans. Magnetics 56, 1–6. doi:10.1109/TMAG.2019.2950953
Xie, Y., Savvaris, A., and Tsourdos, A. (2019). Fuzzy Logic Based Equivalent Consumption Optimization of a Hybrid Electric Propulsion System for Unmanned Aerial Vehicles. Aerosp. Sci. Technol. 85, 13–23. doi:10.1016/j.ast.2018.12.001
Xu, Z. (2021). Application Research of Tethered Uav Platform in Marine Emergency Communication Network. J. Web Eng. 20, 491–512. doi:10.13052/jwe1540-9589.20212
Yang, J., Zhu, K., Zhu, X., and Wang, J. (2021). “Learning-based Aerial Charging Scheduling for Uav-Based Data Collection,” in International Conference on Wireless Algorithms, Systems, and Applications (Springer), 600–611. doi:10.1007/978-3-030-86130-8_47
Yao, Y., Jun-hua, C., Yi, G., Zhun, F., An-Min, Z., Biao, X., et al. (2021). Autonomous Control Method of Rotor Uavs for Power Inspection with Renewable Energy Based on Swarm Intelligence. Front. Energy Res. 9, 229. doi:10.3389/fenrg.2021.697054
Zhang, D., He, J., and Pan, D. (2019). A megawatt-scale medium-voltage high-efficiency high power density “sic+ si” hybrid three-level anpc inverter for aircraft hybrid-electric propulsion systems. IEEE Trans. Industry Appl. 55, 5971–5980. doi:10.1109/tia.2019.2933513
Zhang, Z., Ji, R., Wang, Y., Chang, M., Ma, X., Sha, J., et al. (2021). An Improved Energy Management Strategy for the Solar Powered Unmanned Aerial Vehicle at the Extreme Condition. J. Energy Storage 43, 103114. doi:10.1016/j.est.2021.103114
Zhang, Z., Shen, A., Li, P., and Luo, X. (2020). “Generalized Vector Control Strategy for Torque Angle Compensation of Ipm-type Brushless Dc Motors,” in Proceeding of the IEEE 9th International Power Electronics and Motion Control Conference (IPEMC2020-ECCE Asia) (IEEE), 2254–2260. doi:10.1109/ipemc-ecceasia48364.2020.9368067
Zhou, M., and Prasad, J. (2014). Transient Characteristics of a Fuel Cell Powered Uav Propulsion System. J. Intelligent Robotic Syst. 74, 209–220. doi:10.1007/s10846-013-9903-x
Zhu, C., Zeng, Z., and Zhao, R. (2016). Adaptive Suppression Method for Dc-Link Voltage Offset in Three-phase Four-Switch Inverter-Fed Pmsm Drives. Electron. Lett. 52, 1442–1444. doi:10.1049/el.2016.2048
Keywords: unmanned aerial vehicles, drones, electric propulsion system, field oriented control, direct torque control, brushless direct current (BLDC) motor
Citation: Joshi D, Deb D and Muyeen SM (2022) Comprehensive Review on Electric Propulsion System of Unmanned Aerial Vehicles. Front. Energy Res. 10:752012. doi: 10.3389/fenrg.2022.752012
Received: 02 August 2021; Accepted: 05 May 2022;
Published: 14 May 2022.
Edited by:
Haris M. Khalid, Higher Colleges of Technology, United Arab EmiratesReviewed by:
Rui Wang, Northeastern University, ChinaZimran Rafique, Higher Colleges of Technology, United Arab Emirates
Copyright © 2022 Joshi, Deb and Muyeen. This is an open-access article distributed under the terms of the Creative Commons Attribution License (CC BY). The use, distribution or reproduction in other forums is permitted, provided the original author(s) and the copyright owner(s) are credited and that the original publication in this journal is cited, in accordance with accepted academic practice. No use, distribution or reproduction is permitted which does not comply with these terms.
*Correspondence: S. M Muyeen, sm.muyeen@qu.edu.qa