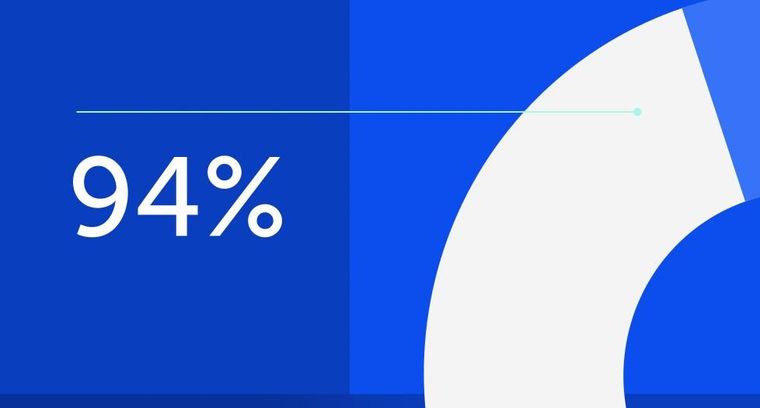
94% of researchers rate our articles as excellent or good
Learn more about the work of our research integrity team to safeguard the quality of each article we publish.
Find out more
ORIGINAL RESEARCH article
Front. Energy Res., 17 January 2023
Sec. Solar Energy
Volume 10 - 2022 | https://doi.org/10.3389/fenrg.2022.1104071
This article is part of the Research TopicPolicy in: Solar EnergyView all 5 articles
The aim of this paper is to investigate the impact of solar energy investment on carbon emissions. The STIRPAT model, a non-parametric additive regression model, and the vector autoregression model are built to investigate the comprehensive effect of solar energy investment on China’s carbon emissions. Solar energy investment and other factors related to carbon emissions are examined. The empirical study shows that it will take about 8 years for the solar energy investment to promote carbon emission reductions. The moderation analysis indicates that technological innovation has a moderating effect in the facilitation of carbon emission reduction by solar energy investment. The finding of this study has some meaningful policy implications. In order to achieve the goal of carbon emission reduction, China should keep solar energy investment continuous and steady and improve technological innovation.
A large number of greenhouse gas emissions from human activities are the main cause of global warming, and climate change concerns everyone in the world. The 26th UN Climate Change Conference (COP26) was held in Glasgow, United Kingdom in November 2021, which is the first climate conference since the implementation of the Paris Accord on climate. The report on the state of global climate in 2021 released at the conference showed that the global average temperature in 2021 was about 1.09°C higher than that from 1850 to 1900. In the summer of 2022, the world encountered another rare high temperature weather again, reflecting the urgency and importance of studying carbon emission reduction. All these indicate the urgency and importance of studying carbon emission reduction. Carbon dioxide covers the largest proportion in greenhouse gases with significant impact on economic growth and it is urgent and important to study carbon emission reduction (Sun et al., 2020).
As a typically large country, China also faces severe carbon emission problems. During recent years, China’s economy has developed rapidly, with its GDP ranking second in the world and less than that of the United States. With economic development, China’s energy consumption and carbon emissions are also growing. China urgently needs to find appropriate new energy sources as gradual substitute for the expensive and increasingly depleted fossil fuels to reduce the heavy dependence on them. The Chinese government is committed to climate protection and has clearly announced that it will strive to reach the peak of carbon dioxide emissions by 2030 and achieve carbon neutrality by 2060. In this context, the development of new energy industry has become one of the key tasks of the 14th Five-Year Plan (2021–2025) for national economic and social development and the long-run goals through the year 2035 in China. The size of solar energy industry, as a relatively mature industry in the new energy field, will be further expanded under the background of carbon emission reduction and the development of solar energy industry is an important guarantee for China to facilitate the successful transformation of energy structure.
It is widely believed that solar energy investment can reduce carbon emissions in a very short time. But this is contrary to the fact that carbon emissions are increasing while solar energy investments are increasing at the beginning years of the investments in China. Why this happens? What role on Earth does solar energy investment play in carbon emission reduction? The aim of this paper is to answer these questions and investigate the impact of solar energy investment on carbon emissions. Therefore, it is very important to study the roles of solar energy investment in the reduction of carbon emissions and China is a good case to be studied. However, there exist few studies on the role of China’s solar energy investment in carbon emissions mainly due to the less availability of the related data. The contribution of this paper is that the scattered solar energy investment data is collated into a consistent one on annual basis to solve the problem of lack of research data, the STIRPAT model is adopted to analyze the impact of solar energy investment, population, GDP per capita and urbanization level on carbon emissions in energy consumption by the ridge regression method and it is found that the inhibition of solar energy investment on carbon emissions has a long lag period (about 8 years), and the level of technological innovation has a moderating effect in the facilitation of carbon emission reduction by solar energy investment. The research results provide some policy implications for the facilitation of carbon emission reduction. China needs to keep solar energy investment continuous and steady and improve technological innovation to achieve the goal of carbon emission reduction. Moreover, this study is widely applicable and is of great significance for other developing countries who aim to reduce emissions to refer to.
This paper is organized as follows. Section 2 provides a literature review. In Section 3, we introduce the research methodology of this paper. Section 4 is the empirical analysis. Finally, Section 5 concludes the paper and offers policy implications.
Carbon emission reduction is the main way to slow down global warming. The studies of carbon emission reduction mainly involve the drivers of emission reduction, how to achieve the goal of emission reduction, and the role of renewable energy in the reduction. The research on the drivers of emission reduction found that technological progress, efficiency improvement and energy structure transformation are the main drivers of carbon emission reduction, but different views exist on the importance of the above three drivers. Many literatures assert that technological innovation and efficiency improvement play a key role in carbon emission reduction (Ang, 2005; Mizobuchi, 2008; Okushima and Tamura, 2010). The establishment of the trading market of carbon emission rights is an important exploration to achieve carbon emission reduction goals through the market mechanism (Wang et al., 2013). Some studies focus on the impact of policy direction on carbon emission reduction at the institutional level. For example, Sun et al. (2022) indicated that institutional quality matters in energy efficiency improvement and countries with good institutional framework has a positive effect on domestic energy efficiency. It is necessary to measure the quantities of emission reduction from different drivers. Kaya identity and Logarithmic Mean Division Index (LMDI) are commonly presented in the literature discussing the influencing factors of carbon emissions (Du et al., 2012).
A tremendous amount of work is about how to achieve carbon emission reduction and the role of renewable energy in emission reduction. Elzen et al. (2016) discussed whether China’s carbon emission reduction goals can be achieved. Umar et al. (2020) believed that all countries in the world need to effectively utilize energy resource endowment and technology for social justice to minimize the adverse effect on nature. However, only by gradually separating economic growth from the use of fossil fuels can global climate change be alleviated. Environmentally sound technology (EST) is considered to be the first concept proposed to cope with carbon emissions (Verhoosel, 1998). With the change of society, economy and environment, the original idea of green has been completely transformed into a new thinking of sustainable development. Economic growth has triggered a simultaneous rise in demand for traditional energy sources such as natural gas, coal and oil (Shah et al., 2020). However, Renewable energy (RE) is regarded as a strategic commodity for sustainable development. Renewable energies such as solar energy, wind energy, waste and biomass are considered cost-effective and eco-friendly, because they can reduce pollution, provide better security for energy, reduce harmful climate change, and ultimately provide low-cost power for remote areas (Tareen et al., 2018; Gielen et al., 2019). Renewable energy reduces the long-term estimated ecological footprint well, and the existence of the environmental Kuznets curve (EKC) was confirmed (Sharif et al., 2020a). Using the advanced quantile model, some scholars tried to analyze the relationship between the use of renewable energy (mainly the solar energy) and carbon emissions from 1990 to 2017 and certified that there is a two-way causal relationship between renewable energy use and carbon emissions (Sharif et al., 2020b).
Moreover, there are a lot of surveys on renewable energy, mainly involving the diffusion models of renewable energy technologies (Rao and Kishore, 2010; Chen and Lin, 2020), renewable energy structure (Burandt et al., 2019; Wang et al., 2019), cost-benefit analysis (Snyder and Kaiser, 2009; Johansson and Kriström, 2019; Huang et al., 2020) and economic performance on investment (Menegaki, 2008; Edenhofer et al., 2013; Zhao and Xie, 2019; Rasoulinezhad, 2022). These studies are mainly related to bioenergy, hydropower, wind energy and other types of renewable energy (Huang et al., 2017; Nian et al., 2019).
The number of studies on solar energy, one of the renewable energies, is increasing. Solar energy is considered to be one of the best options to facilitate carbon emission reduction. The installation cost of solar photovoltaic (PV) has dropped significantly by more than 80% since 2010 (Ilas et al., 2018). Therefore, the global solar energy investment has increased significantly, and the total installed capacity in the world had reached 399 GW by 2017 (Dudley, 2018). Nugent and Sovacool (2014) found that the life cycle of solar greenhouse gas emissions is far less than that of fossil energy. However, there is very little literature on China’s solar energy investment and its role in carbon emission reduction, which is inconsistent with the fact that China is currently the largest solar energy consumption market in the world (Xu et al., 2020). Therefore, this paper attempts to find out the role of China’s solar energy investment in carbon emission reduction.
Various macro-econometric models are used for empirical study of carbon emissions. Kalmaz and Kirikkaleli (2019) confirmed the long-term equilibrium relationship between carbon emissions, energy consumption and other macroeconomic variables by using the ARDL model. Some early studies used the Impact-Population-Affluence-Technology (IPAT) model to find that variables such as population, income and technology can also affect carbon emissions (Raskin, 1995; York et al., 2002). The theoretical foundation for the IPAT model is the relationship among income, population, technology and environmental impact. With further development, the IPAT model is extended to a random version, the STIRPAT model (York et al., 2003). The recent study which uses the STIRPAT model to examine the relationship between CO2 emissions, energy efficiency, green energy index (GEI), and green finance in the top ten economies is Rasoulinezhad and Taghizadeh-Hesary (2022). Hence the STIRPAT model is adopted in this paper to empirically analyze the impact of various factors on carbon emissions, especially the role of solar investment in carbon emission reduction. Due to the fact that there exist few studies on the role of solar energy investment in carbon emissions in China, this study will bridge the gap.
The conceptual framework to show the methodological procedure is in Figure 1.
The STIRPAT model is used to examine the impact of population, GDP per capita, solar energy investment and the level of urbanization on carbon emissions generated by energy consumption. The formula of the STIRPAT model is
where
In line with the reality of China, model (2) can be extended to the following model
where
The vector autoregression (VAR) model is a widely used econometric model and it is in the form of simultaneous multiple equations. The VAR model is a model for two or more time series where each variable is modeled as a linear function of past values of all variables, plus stochastic disturbances that have zero means given all past values of the observed variables. The mathematical expression for the VAR(p) model for k variables of time series is
where
The time series data of each variable in this paper is from two sources, Statistical Yearbook of the Chinese Investment in Fixed Assets (2003–2011) and China Electric Power Yearbook (2012–2020). The total carbon emissions, population, GDP per capita, solar energy investment and urbanization level were processed with the method of total sum normalization. The data shows that during the sample period, the amount of solar energy investment grows continually, while the carbon emissions also rises year by year.
Let K = 0.240, and the results of ridge regression analysis of model (3) are illustrated in Table 1. The coefficient of determination is 0.923 which shows that solar energy investment, population, GDP per capita and urbanization level can explain 92.3% of the changes of total carbon emission in China. The model passes the test of significance and the equation after estimation will be
All the coefficients of the regression are positive, which implies that the impacts of solar energy investment, population, GDP per capita and urbanization level are significantly positive.
Furthermore, another variable,
All the coefficients of the regression are positive, which implies that the impacts of solar energy investment, population, GDP per capita, urbanization level and technological innovation level are significantly positive.
Due to the role of exogenous technological factors and renewable energy in carbon dioxide emission reduction (Edziah et al., 2022), the interaction term,
We can understand the role of the product term lnT×lnR&D in Equation 7 from two perspectives. From the statistical perspective, the influence of the independent variable lnT on the dependent variable lnI will be moderated by another variable lnR&D, that is, lnR&D will affect the sign and the strength of the correlation between lnI and lnT. While from the perspective of economic implications, the level of technological innovation will affect both the direction and the intensity of the influence of solar energy investment on carbon emissions. It can be seen from the above empirical results that the coefficient of the interaction term is negative and technological innovation plays a moderating role in the process of solar energy investment affecting carbon emissions. This means that solar energy investment can play a role in curbing carbon emissions by improving the level of technological innovation.
In order to further explore the impact of solar energy investment on carbon emissions, a VAR model for impulse response analysis is built. First, the ADF unit root test and the maximum lag order test are performed. The natural logarithms of all the data show stationarity and are significant at the level of 5%, indicating that the variables are stationary. Similarly, according to the test results of the maximum lag order in Table 4, the maximum lag order of the VAR model should be 3.
The results of Granger causality test in Table 5 show that there is a one-way Granger causality between solar energy investment and carbon emissions and carbon emissions are Granger caused by solar energy investment.
Then the unit root test of VAR model is carried out and the results are displayed in Table 6. It can be seen that all the moduli of the inverse roots of the characteristic polynomial are less than 1. The VAR model passes the unit root test.
The impulse response in Figure 2 shows that the impact of solar energy investment on carbon emissions will gradually have an adverse effect after the eighth phase, indicating that it will take at least eight years to promote the carbon emission reduction solely by the development of solar energy investment.
To sum up, the empirical analysis of this paper illustrates that carbon emissions will be restrained long after the investment made to solar energy. It is necessary to make the moderating effect of technological innovation work to facilitate solar energy investment to reduce carbon emissions. To ensure the validation of empirical findings, we use the carbon emissions per capita in China to replace the total carbon emissions of China to conduct the robustness check. The empirical results are almost the same. Hence the empirical analysis in this study is reliable.
Carbon emission reduction is a key measure to promote global climate governance and realize sustainable development. Solar energy, as a renewable energy, should play an important role in achieving carbon emission reduction goals. In order to study the effect of solar energy investment on carbon emission reduction, the STIRPAT model and the VAR model are employed for empirical analysis. It is found that the inhibition of solar energy investment on carbon emissions has a long lag period (about 8 years), and the level of technological innovation has a moderating effect in the facilitation of carbon emission reduction by solar energy investment. The finding of this study is somewhat like that of Saboori et al. (2022) on the effect of renewable energy consumption and economic growth on unemployment rate and is shown in Figure 3.
In the light of the conclusion, we make some policy implications to give full play to the positive role of solar energy investment in facilitating carbon emission reduction.
Investment in solar energy is required to continue and increase. As a renewable and clean energy, solar energy is an effective alternative to fossil fuels. It will take a long time (about eight years) for solar energy investment to form production capacity and play an alternative role to traditional energy. Therefore, it is necessary to continuously increase the investment in solar energy, increase the proportion of solar energy in energy consumption, and optimize the energy structure. To provide sufficient funds for the investment, diversifying channels of financing instead of just relying on bank loans is recommended. There exist some Green Financing solutions, such as green bonds, are more favorable (Taghizadeh-Hesary et al., 2022). In addition, FDI is also an effective source for investment funds and it is found that FDI can promote green growth in a country (Phung et al., 2022).
The level of technological innovation, especially the level of that in the field of solar energy should be constantly improved. The empirical analysis of this paper finds that technological innovation has a moderating effect on the promotion of solar energy investment to carbon emission reduction. Therefore, while increasing the investment in solar energy, it is also needed to constantly accelerate technological innovation and improve the level of technological innovation. In this way, twice as much can be accomplished with half the effort, the proportion of fossil fuels will be gradually reduced, the energy structure will be optimized, and the goal of carbon emission reduction is finally achieved.
There are some limitations to this study. First, the methodology of this paper cannot capture the overall effects of the solar energy investment. In the future, it is necessary to use the general equilibrium framework to evaluate impacts of solar energy investments on carbon emissions from the macroeconomic perspective (Yoshino et al., 2022). Second, the shock of COVID-19 pandemic on the relationship between solar energy investments and carbon emissions are not examined and it is a hot issue to be studied in the future.
Publicly available datasets were analyzed in this study. This data can be found here: Statistical Yearbook of the Chinese Investment in Fixed Assets (2003–2011) and China Electric Power Yearbook (2012–2020).
LZ: supervision, conceptualization, methodology, writing—original draft, and writing—reviewing and editing, validation. RC: software, data, visualization, writing—original draft. YW: methodology, data, and writing—reviewing and editing.
The authors declare that the research was conducted in the absence of any commercial or financial relationships that could be construed as a potential conflict of interest.
All claims expressed in this article are solely those of the authors and do not necessarily represent those of their affiliated organizations, or those of the publisher, the editors and the reviewers. Any product that may be evaluated in this article, or claim that may be made by its manufacturer, is not guaranteed or endorsed by the publisher.
Ang, J. (2005). CO2 emissions, research and technology transfer in China. Ecol. Econ. 68 (10), 2658–2665. doi:10.1016/J.ECOLECON.2009.05.002
Burandt, T., Xiong, B., Löffler, K., and Oei, P-Y. (2019). Decarbonizing China’s energy system – modeling the transformation of the electricity, transportation, heat, and industrial sectors. Appl. Energy 255, 113820. doi:10.1016/j.apenergy.2019.113820
Chen, Y., and Lin, B. (2020). Slow diffusion of renewable energy technologies in China: An empirical analysis from the perspective of innovation system. J. Clean. Prod. 261, 121186. doi:10.1016/j.jclepro.2020.121186
Du, G., Sun, Z., and Miao, J. (2012). Research on human ADL data real-time transmission optimization method based on bayesian network. Econ. Perspect. 508 (4), 88–91. doi:10.4028/www.scientific.net/amr.508.88
Edenhofer, O., Hirth, L., Knopf, B., Pahle, M., Schlömer, S., Schmid, E., et al. (2013). On the economics of renewable energy sources. Energy Econ. 40, S12–S23. doi:10.1016/j.eneco.2013.09.015
Edziah, B., Sun, H., Adom, P., Wang, F., and Agyemang, A. (2022). The role of exogenous technological factors and renewable energy in carbon dioxide emission reduction in Sub-Saharan Africa. Renew. Energy 196, 1418–1428. doi:10.1016/j.renene.2022.06.130
Elzen, M., Fekete, H., Höhne, N., Admiraal, A., Forsell, N., Hof, A. F., et al. (2016). Greenhouse gas emissions from current and enhanced policies of China until 2030: Can emissions peak before 2030? Energy Policy 89, 224–236. doi:10.1016/j.enpol.2015.11.030
Gielen, D., Boshell, F., Saygin, D., Bazilian, M. D., Wagner, N., and Gorini, R. (2019). The role of renewable energy in the global energy transformation. Energy Strateg. Rev. 24, 38–50. doi:10.1016/j.esr.2019.01.006
Huang, J., Li, W., Guo, L., Hu, X., and Hall, J. (2020). Renewable energy and household economy in rural China. Renew. Energy 155, 669–676. doi:10.1016/j.renene.2020.03.151
Huang, J., Li, W., Huang, X., and Guo, L. (2017). Analysis of the relative sustainability of land devoted to bioenergy: Comparing land-use alternatives in China. Sustainability 9, 801. doi:10.3390/su9050801
Ilas, A., Ralon, P., Rodriguez, A., and Taylor, M. (2018). Renewable power generation costs in 2017. Abu Dhabi: International Renewable Energy Agency.
Johansson, P-O., and Kriström, B. (2019). Welfare evaluation of subsidies to renewable energy in general equilibrium: Theory and application. Energy Econ. 83, 144–155. doi:10.1016/j.eneco.2019.06.024
Kalmaz, D., and Kirikkaleli, D. (2019). Modeling CO2 emissions in an emerging market: Empirical finding from ARDL-based bounds and wavelet coherence approaches. Environ. Sci. Pollut. Res. 26, 5210–5220. doi:10.1007/s11356-018-3920-z
Menegaki, A. (2008). Valuation for renewable energy: A comparative review. Renew. Sust. Energy Rev. 12, 2422–2437. doi:10.1016/j.rser.2007.06.003
Mizobuchi, K. (2008). An empirical study on the rebound effect considering capital costs. Energy Econ. 30 (5), 2486–2516. doi:10.1016/j.eneco.2008.01.001
Nian, V., Liu, Y., and Zhong, S. (2019). Life cycle cost-benefit analysis of offshore wind energy under the climatic conditions in Southeast Asia – setting the bottom-line for deployment. Appl. Energy 233, 1003–1014. doi:10.1016/j.apenergy.2018.10.042
Nugent, D., and Sovacool, B. (2014). Assessing the lifecycle greenhouse gas emissions from solar PV and wind energy: A critical meta-survey. Energy Policy 65, 229–244. doi:10.1016/j.enpol.2013.10.048
Okushima, S., and Tamura, M. (2010). What causes the change in energy demand in the economy? The role of technological change. Energy Econ. 32, S41–S46. doi:10.1016/j.eneco.2009.03.011
Phung, T., Rasoulinezhad, E., and Luong Thi Thu, H. (2022). How are FDI and green recovery related in Southeast Asian economies? Econ. Change Restruct. doi:10.1007/s10644-022-09398-0
Rao, K., and Kishore, V. (2010). A review of technology diffusion models with special reference to renewable energy technologies. Renew. Sust. Energy Rev. 14, 1070–1078. doi:10.1016/j.rser.2009.11.007
Raskin, P. (1995). Methods for estimating the population contribution to environmental change. Ecol. Econ. 15, 225–233. doi:10.1016/0921-8009(95)00047-X
Rasoulinezhad, E. (2022). Environmental impact assessment analysis in the kahak’s wind farm. J. Environ. Assess. Policy Manage. 22, 2250006. doi:10.1142/S1464333222500065
Rasoulinezhad, E., and Taghizadeh-Hesary, F. (2022). Role of green finance in improving energy efficiency and renewable energy development. Energy Effic. 15, 14. doi:10.1007/s12053-022-10021-4
Saboori, B., Gholipour, H., Rasoulinezhad, E., and Ranjbar, O. (2022). Renewable energy sources and unemployment rate: Evidence from the US states. Energy Policy 168, 113155. doi:10.1016/j.enpol.2022.113155
Shah, S., Chughtai, S., and Simonetti, B. (2020). Renewable energy, institutional stability, environment and economic growth nexus of D-8 countries. Energy Strateg. Rev. 29, 100484. doi:10.1016/j.esr.2020.100484
Shahbaz, M., Loganathan, N., Sbia, R., and Afza, T. (2015). The effect of urbanization, affluence and trade openness on energy consumption: A time series analysis in Malaysia. Renew. Sust. Energy Rev. 47, 683–693. doi:10.1016/j.rser.2015.03.044
Sharif, A., Baris-Tuzemen, O., Uzuner, G., Ozturk, I., and Sinha, A. (2020a). Revisiting the role of renewable and non-renewable energy consumption on Turkey’s ecological footprint: Evidence from quantile ARDL approach. Sustain. Cities Soc. 57, 102138. doi:10.1016/j.scs.2020.102138
Sharif, A., Mishra, S., Sinha, A., Jiao, Z., Shahbaz, M., and Afshan, S. (2020b). The renewable energy consumption-environmental degradation nexus in Top-10 polluted countries: Fresh insights from quantile-on-quantile regression approach. Renew. Energy 150, 670–690. doi:10.1016/j.renene.2019.12.149
Snyder, B., and Kaiser, M. (2009). Ecological and economic cost-benefit analysis of offshore wind energy. Renew. Energy 34, 1567–1578. doi:10.1016/j.renene.2008.11.015
Sun, H., Edziah, B., Sun, C., and Kporsu, A. (2022). Institutional quality and its spatial spillover effects on energy efficiency. Socio-Econ. Plan. Sci. 83, 101023. doi:10.1016/j.seps.2021.101023
Sun, H., Samuel, C., Amissah, J., Taghizadeh-Hesary, F., and Mensah, I. (2020). Non-linear nexus between CO2 emissions and economic growth: A comparison of oecd and B&R countries. Energy 212, 118637. doi:10.1016/j.energy.2020.118637
Taghizadeh-Hesary, F., Li, Y., Rasoulinezhad, E., Mortha, A., Long, Y., Lan, Y., et al. (2022). Green finance and the economic feasibility of hydrogen projects. Int. J. Hydrog. Energy 47, 24511–24522. doi:10.1016/j.ijhydene.2022.01.111
Tareen, W., Anjum, Z., Yasin, N., Siddiqui, L., Farhat, I., Malik, S. A., et al. (2018). The prospective non-conventional alternate and renewable energy sources in Pakistan—A focus on biomass energy for power generation, transportation, and industrial fuel. Energies 11, 2431. doi:10.3390/en11092431
Umar, M., Ji, X., Kirikkaleli, D., Shahbaz, M., and Zhou, X. (2020). Environmental cost of natural resources utilization and economic growth: Can China shift some burden through globalization for sustainable development? Sustain. Dev. 28, 1678–1688. doi:10.1002/sd.2116
Verhoosel, G. (1998). Beyond the unsustainable rhetoric of sustainable development: Transferring environmentally sound technologies. Geo. Int. Environ. L. Rev. 11, 49. 1998-1999.
Wang, F., Feng, G., and Wu, L. (2013). Regional contribution to the decline of national carbon intensity in China’s economic growth. Econ. Res. J. (8), 143–155.
Wang, F., Xie, Y., and Xu, J. (2019). Reliable-economical equilibrium based short-term scheduling towards hybrid hydro-photovoltaic generation systems: Case study from China. Appl. Energy 253, 113559. doi:10.1016/j.apenergy.2019.113559
Xu, M., Xie, P., and Xie, B-C. (2020). Study of China’s optimal solar photovoltaic power development path to 2050. Resour. Policy 65, 101541. doi:10.1016/j.resourpol.2019.101541
York, R., Rosa, E., and Dietz, T. (2002). Bridging environmental science with environmental policy: Plasticity of population, affluence, and technology. Soc. Sci. Q. 83, 18–34. doi:10.1111/1540-6237.00068
York, R., Rosa, E., and Dietz, T. (2003). STIRPAT, IPAT and ImPACT: Analytic tools for unpacking the driving forces of environmental impacts. Ecol. Econ. 46 (3), 351–365. doi:10.1016/S0921-8009(03)00188-5
Yoshino, N., Rasoulinezhad, E., and Taghizadeh-Hesary, F. (2022). Economic impacts of carbon tax in a general equilibrium framework: Empirical study of Japan. J. Environ. Assess. Policy Manage. 23, 2250014. doi:10.1142/S1464333222500144
Keywords: solar energy investment, carbon emission reduction, STIRPAT model, VAR model, moderation analysis
Citation: Zhao L, Cao R and Wang Y (2023) Solar energy investment, technological innovation and carbon emission reduction: Evidence from China. Front. Energy Res. 10:1104071. doi: 10.3389/fenrg.2022.1104071
Received: 21 November 2022; Accepted: 09 December 2022;
Published: 17 January 2023.
Edited by:
Huaping Sun, Jiangsu University, ChinaReviewed by:
Haixiang Yao, Guangdong University of Foreign Studies, ChinaCopyright © 2023 Zhao, Cao and Wang. This is an open-access article distributed under the terms of the Creative Commons Attribution License (CC BY). The use, distribution or reproduction in other forums is permitted, provided the original author(s) and the copyright owner(s) are credited and that the original publication in this journal is cited, in accordance with accepted academic practice. No use, distribution or reproduction is permitted which does not comply with these terms.
*Correspondence: Linhai Zhao, bG9uZ21lbjE2OEAxMjYuY29t
Disclaimer: All claims expressed in this article are solely those of the authors and do not necessarily represent those of their affiliated organizations, or those of the publisher, the editors and the reviewers. Any product that may be evaluated in this article or claim that may be made by its manufacturer is not guaranteed or endorsed by the publisher.
Research integrity at Frontiers
Learn more about the work of our research integrity team to safeguard the quality of each article we publish.