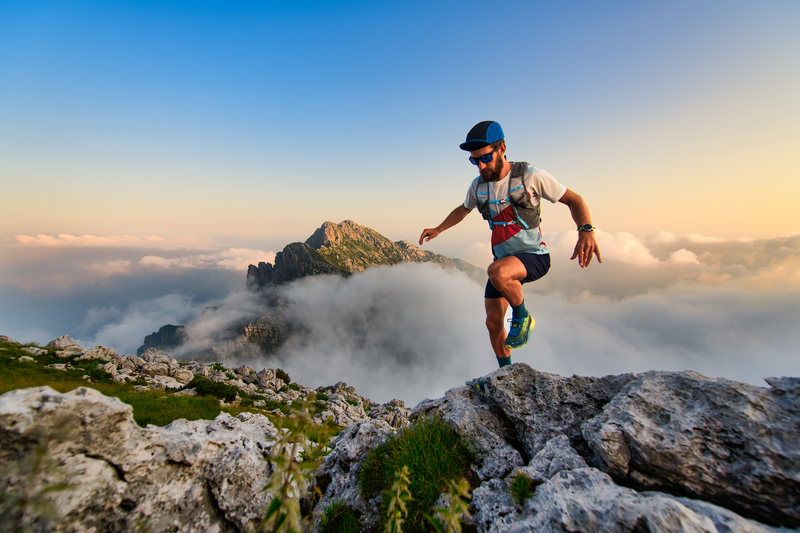
94% of researchers rate our articles as excellent or good
Learn more about the work of our research integrity team to safeguard the quality of each article we publish.
Find out more
ORIGINAL RESEARCH article
Front. Energy Res. , 12 January 2023
Sec. Sustainable Energy Systems
Volume 10 - 2022 | https://doi.org/10.3389/fenrg.2022.1101404
This article is part of the Research Topic Enabling technologies and business models for energy communities View all 15 articles
Introduction: Fossil fuel and electricity-based irrigation practices contribute to greenhouse gases and add substantial costs to water access. Solar-powered irrigation is spreading globally, notably in developing countries, as a solution to the rising energy and climate concerns related to agriculture. This policy perspective devoted to examining the impact of the solar irrigation facilities (SIF) adoption on irrigation cost and return on investment (ROI) based on seven years of panel data seeks to contribute to the efforts to propel solar irrigation toward delivering on the myriad of promises.
Methods: Panel logistic regression was employed to analyze adoption determinants, while adoption impact was evaluated through the propensity score matching with the difference-in-difference (PSM-DID) method. In addition, the time and panel fixed effect DID and doubly robust DID model was also used for robustness check.
Results: The result reveals that SIF adoption significantly increased ROI by 20% to 30% and reduced irrigation costs by 21% to 30%.
Conclusion: The findings call for further research and analysis on evidence-based best practices for solar irrigation solutions at the farm level so that the dissemination of this revolutionary technology, apart from contributing to the advancement of the energy sector, also plays a vital role in driving us towards establishing a more equitable and sustainable world.
The world is confronting a reckoning regarding the energy issue. Despite decades of pleas to minimize dependence on non-renewable energy, nations have intensified the usage of coal, oil, and gas to fuel their economies (WFP, 2022). The consequences of the extensive burning of fossil fuels have intensified the carbon emissions issue and created a globalized world in which food and energy systems are highly concentrated—making them extremely vulnerable to disruption. The world now grapples with consecutive waves (i.e., heat waves, the millennium drought, poverty impacts from COVID-19, and ongoi`ng supply chain challenges due to war) that negatively impact agriculture and have been the instigator of a potentially severe food crisis. These interlocking crises have contributed to global energy and food price spikes and have placed agriculture and irrigation in a precarious position where energy-efficient technology use has become obligatory (UNSDG, 2022; WFP, 2022).
The impact is severe in developing countries, especially Asia, where diesel and electric-based irrigation plays a vital role in domestic food security and poverty alleviation (Sunny et al., 2022a). While Myanmar and Pakistan’s daily consumption sits around 2.7 million liters and 3.5 billion liters of diesel, respectively, Nepal’s Eastern Indo-Gangetic Plains only have 20% of its irrigation pumps non-diesel reliant (Qureshi, 2014; Foster et al., 2019; Phillips, 2021). Clearly, the region has learned and adapted to the challenges of inconsistent power generation for rural agricultural work, illustrating flexibility and resilience within the sector. However, reliance on such a non-renewable resource may cause some new challenges in the future. In India, for instance, of the total electrical power generated, 18% goes to agriculture; similarly, 5% of the total diesel in the country is allocated for just irrigational purposes (IRENA, 2016). Though not sounding too dire, one must consider what concentrations of which demographic find themselves heavily reliant on diesel as a substitute for the lack of national electrical grid energy supplies. This is a profound question, especially since 1.6 billion people live without electricity in developing countries—most in Sub-Saharan Africa and South Asia (The World Bank, 2018). The struggle to meet energy demands leads to load shedding that disrupts planning and resource management and, more specifically, interrupts the irrigation process (Hoque et al., 2016).
Energy security is one of the major global concerns, as energy deficiencies and resulting economic factors may generate socio-political issues. To ensure food security, enhance energy security, prevent local pollution, and increase climate benefits, the policy manifesto for most developing countries with similar issues has the impetus for adopting reliable, cost-effective, and clean energy irrigation technologies (Schwanitz et al., 2014; Rentschler and Bazilian, 2016; Sarker and Ghosh, 2017).
Like other developing economies, the agriculture sector is regarded as one of the critical drivers of Bangladesh’s economy. As a catalyst for sustainable growth of the country, the sector accounts for 12.92% of the gross domestic product (GDP) and 38 percent of the labor force (The World Bank, 2021; The World Bank, 2022). Around 70% of Bangladesh’s population’s livelihood depends on agricultural activities (Imdad, 2021). The country’s natural inheritance of favorable soil, climate, and groundwater availability has alleviated farmers’ opportunities to grow tropical and temperate crops on over two-thirds of cultivatable land twice or more annually. Rice (Oryza sativa) is the staple food that accounts for approximately 75 percent of the total harvested area and contributes around 95 percent of the total food grain (Shew et al., 2019; Alam et al., 2021). Irrigation is a fundamental operation unit in rice production and is essential for the agriculture life cycle system (Ali, 2018). Even though insufficient rainfall in the dry season and scarcity of surface water has hampered rice productivity in many parts of Bangladesh, especially the northern regions, groundwater utilization has played a vital role in ameliorating agricultural development (Biswas and Hossain, 2013; Hasnat et al., 2014). The development of groundwater policies has resulted in the expansion of Low Lift Pumps (LLP), Shallow Tube Wells (STW), and Deep Tube Wells (DTW) usage in Bangladesh (BGEF, 2016). The first two systems run on diesel, while the latter runs on electricity from the national grid. The maximum capacities of the LLP are 7.5, SWT is 12.5, and DWT is 55 horsepower (hp) (Hossain et al., 2015). These water extraction technologies have greatly aided Bangladesh in attaining near self-sufficiency in rice production. The downside, however, has been the massive energy demand increase (Sunny et al., 2022a; Sunny et al., 2022b). Presently, approximately 1.6 million diesel pumps consume at least one million tons per year to satisfy irrigation needs (Prothom Alo, 2021). When this is estimated in monetary terms, the sum reaches a conservative total of $900 million (Ershadullah, 2021). Between the transportation, added pollution through transportation of the fuel and the use of it at its endpoint, and the potential calamities that could occur environmentally along the production chain - a second thought should be given to diesel as a primary fuel source for extracting ‘clean renewables’ like water. Recent estimates indicate that even though irrigation consumes 4.58% of the total electricity generated in the nation (ADB, 2018), the electricity demand in the forthcoming irrigation season is projected to rise from 14,097 MW to 15,500 MW (The Business Standard, 2022). The national grid can not ensure regular power without frequent outages, voltage flickering, and constantly increased tariffs and rates (Doby, 2018). The result is major disruptions in irrigation activities and, thus, revenue streams. In some situations, farmers have been forced to adapt by irrigating during low-peak hours (at night) when the power is more stable (Odarno, 2017). Other farmers have chosen to take control of their power provision by investing in diesel pumps, which carry their deficiencies. These pumps are at the mercy of fuel prices, technical defects, service gaps, and mismanaged usage and can sometimes be more problematic than the national grid (Energypedia, 2020; Mirta et al., 2021).
Concerns for alternatives have been raised regarding agricultural sustainability in defying these challenges. Hence, like other emergent nations,’ Bangladesh has also embraced the idea of sustainable agriculture practices alongside the overarching concept of sustainable development. Sustainable agriculture advocates adopting measures to conserve the natural environment and resources through technically appropriate, economically viable, and socially accepted approaches (FAO, 1989). It also integrates the ideology of enhancing resilience to shocks and stresses over more prolonged periods and addresses more comprehensive economic, social and environmental outcomes from the local to the global level (Pretty, 2008). Sustainable agriculture is central to attaining many sustainable development goals (United Nations, 2015; FAO, 2019). The key to achieving agricultural sustainability is by ameliorating productivity through adopting technology and practices that remediate the environment from poor agricultural practices abuse and drive the welfare of the food producers (Zilberman et al., 1997; Pretty, 2008). Therefore, besides improving strategic and operational farm management, the government has highlighted up-scaling renewable energy-based irrigation systems. If current climate change estimates were to add sufficient motivation for action, then the proposition holds to establish 50,000 solar irrigation pumps by 2027. The results would be an estimated reduction in greenhouse gas (GHG) emissions by up to 15% by 2030 (Mirta et al., 2021). Apart from that, 10% of conventional energy would be replaced, and fossil fuel reserve depletion would rapidly regress while ensuring sustainable water management in agriculture sectors (Kanojia, 2019; Sajid, 2019; Rana et al., 2021a). Despite the significant potential, solar irrigation technologies promotion has been sluggish, and the penetration of solar pumps faces the challenge of competing against other conventional systems (SREDA, 2015; Rana et al., 2021a). Given this context, this work attempts to answer the following research questions.
• What key determinants influence our study area farmers to adopt SIF?
• How do SIF adoption impact farmers’ irrigation cost and ROI?
• What are the associated challenges to the sustainability of SIFs and the measures to overcome the challenges?
Several studies have documented the advantage of solar-based irrigation system adoption over conventional systems. For instance: the performance and reliability test of different types of solar-powered water pumping systems in the United States and Spain revealed that these systems are cheaper alternatives for rural, with high performance, ensure customer satisfaction, and are an environmentally-viable energy source for pumping in irrigation networks (Chowdhury et al., 1993; García et al., 2019). A study conducted in northern Benin revealed that compared to non-adopters, commercial-scale solar-powered drip irrigation systems adopters were able to significantly increase production (Alaofè et al., 2016). Likewise, the adoption of solar-based water pumping systems in china has resulted in ameliorating forage productivity, meeting local demand, and minimizing carbon emissions (Campana et al., 2017). Besides, SIF adoption impact analysis in the Philippines revealed that the adoption not only aided in GHG emissions reduction by up to 26.5 tons CO2eq/ha/year but also contributed to the energy sector by savings between 11.4 and 378.5 L/ha of diesel per year with an average of 315% returns on investment (Guno and Agaton, 2022). Furthermore, SIF adoption in Pakistan has significantly contributed to reducing operational costs, increased farmers’ income, reduced 17,622 tons of CO2 emissions per year, and saved 41% of water usage (Raza et al., 2022). In addition, apart from irrigation purpose usage and meeting electricity needs, SIFs adoption contributes to facilitating drinking water requirements in water-scarce regions and contributes toward gender empowerment by alleviating the burden of labor-intensive diesel system operation and allowing women to utilize their time for productive purposes (IRENA, 2016; Agrawal and Jain, 2018).
The literature on SIF adoption analysis in the context of Bangladesh revealed that if the economic return is considered based on the internal rate of return (IRR), then the most profitable option would be establishing small-sized SIF (20%), followed by large-sized (10%). On the other hand, the net environmental benefit per kilowatt peak (kWp) is highest (86,000) for the small SIFs, followed by medium SIFs (67,184 kWp) and large SIFs (65,392 kWp) (Islam and Hossain, 2022). Other research findings suggested that SIF adopters could reduce irrigation costs by a maximum of 2.22%, obtain 4.48%–8.16% higher ROI, and reduce nearly 1% of total production cost compared to non-adopters (Sunny et al., 2022a). Another study stated that even though the initial investment cost of SIF was found to be higher than a diesel-powered system, the low maintenance and zero fuel costs make it a cheaper option in the long run (Rana et al., 2021b).
This study compares to others contributing to literature in several ways. Firstly, this study used panel data to assess the impact of SIF adoption on irrigation cost and return on investment (ROI). As the return on investment variable considers the gross revenue of farm production and the production costs, it can better reflect the efficiency of farm performance (Kleemann et al., 2014; Zheng and Ma, 2021). Secondly, we employed propensity score matching (PSM) with the difference in difference (DID or DD) models to estimate adoption impact and address the selection bias issue, which also differs from other related studies (Barreto and Bell, 1994; Coady, 1995; Duflo et al., 2011; Dong et al., 2012; Fanus et al., 2012; Martey et al., 2013; Zhou and Abdullah, 2017; Kumar et al., 2019; Kumar et al., 2020; Sanap et al., 2020). We also used fixed effect DID and doubly robust DID for robustness checking. Finally, examining the role of solar irrigation technology on welfare outcomes is of great significance to policy formulation to tackle future climate vulnerability while enhancing farm productivity, food security, and poverty reduction.
This study focuses on the drought-prone area of the northern region of Bangladesh that receives merely 372 mm of rain from November to May, compared to 546 mm during the same time in the whole country. The average annual rainfall of this region is 21.83% lower than the country’s average annual rainfall. Inadequate rainfall and limited surface water have created high dependence on groundwater for irrigation in these areas (Hossain et al., 2021; Rahman et al., 2022). Nearly 1.6 million diesel pumps (Prothom Alo, 2021) and 3.20 lakh electricity pumps (Ershadullah, 2021) that are operating in the country, a significant proportion is operating in the northern region (Hossain et al., 2021).
For this study, multistage sampling techniques were employed. At first, the Dinajpur district was selected for several reasons. Dinajpur is the largest district among all sixteen districts situated in the northern part, and according to the international ‘Köppen climate classification,’ the district has a tropical wet-dry climate. The annual average temperature is 25 °C. The average precipitation from November to March is below 20 mm, April and October are below 100 mm, and the remaining 5 months are over 200 mm (Encyclopedia, 2018). Due to the low precipitation rate, the district is considered one of the top drought-prone areas of Bangladesh (Afrin et al., 2019; Islam et al., 2022a; Rahman et al., 2022), where the food insecurity and poverty rate are high (BBS and WFP, 2020). This district is also one of the top districts where more solar irrigation pumps are installed (SREDA, 2022). We used a simple random sampling method to select 3 of 13 sub-districts from the Dinajpur district in the second stage. The randomly chosen three sub-districts were Birganj, Khanshama, and Kaharol. The combined population of these three sub-districts is 643,431 (Population and BBS, 2011).
We then used Krejcie and Morgans’ (Krejcie and Morgan, 1970) table to determine the optimal sample size. A sample of 384 farmers was determined based on the population size. However, a 5% additional sample was collected to avoid unexpected future issues such as farmers’ discontinuation of SIF or land rented to others. Thus from 50 different solar irrigation sites in three sub-districts, eight farmers were randomly chosen for control and treatment groups. These farmers were interviewed each year between February and April, starting from 2015 till 2021.
The baseline of this study was 2015 and 2016, the treatment period stated in the year 2017, and the end line was 2021. Hence finally, we obtained a panel of (50*8 = 405*7) 2,835 farmers. The Boro season (starting in December and ending in June) was chosen since the maximum rice is produced in this season (BBS, 2020), and irrigation demand is very high. The interview schedule was translated into the local language for implementation. Our interview schedule included farmers’ demographic and socioeconomic characteristics, environmental, agroecology, technology-related knowledge, fee opinion, service quality, and infrastructure-related questions.
This study is based on the random utility theory developed by McFadden in 1974 (McFadden and Zarembka, 1974), which is consistent with Lancaster’s economic theory of value and neoclassical view that hypothesize individuals would choose alternatives that maximize their utility (Lancaster, 1966; Manski, 1977; Hoyos, 2010; Hess et al., 2018). Based on this theory, we would like to see if solar irrigation adoption compared to other irrigation mediums is beneficial or not.
To estimate the factor that influences our study area farmers’ adoption or non-adoption decision of SIF, we consider the following logistic regression model (Neuhaus et al., 1991):
Where
Prior studies on impact assessment suggested that a significant hurdle while conducting related research is constructing appropriate counterfactuals. Because a set of observable and unobservable factors influences the adoption process, failure to do so will cause the corresponding impact estimates to be biased (Mendola, 2007; Wu et al., 2010). Therefore, studies have used various methods to assess the impact of technology adoption that considers selection bias (Mendola, 2007; Becerril and Abdulai, 2010; Wu et al., 2010; Asfaw et al., 2011; Asfaw et al., 2012; Khonje et al., 2015; Alem and Broussard, 2018; Khonje et al., 2018; Nakano et al., 2018; Islam et al., 2019; Manda et al., 2020).
This study, in mitigating the selection and time-invariant source of bias issue and in measuring the adoption impact of SIF on farmers’ irrigation cost and their return on investment (ROI), has adopted difference in difference estimation with propensity score matching (PSM-DID). This approach compares two populace groups (the treated and the non-treated) based on the time sequence of before and after-action states. The effectiveness of the treatment (a course of action) is considered adequate toward the outcome when the intervention group shows off better or worse trends over their controlled counterpart (considering other influencing factors such as ceteris paribus) (Islam et al., 2022b).
The single DID setting proposed by Villa (Villa, 2016) is presented as follows:
Where the baseline period is
If additional covariates are combined with the single DID setting, the model will be:
The DID is a flexible form of causal inference because it can be combined with other procedures, such as kernel propensity score matching (Heckman et al., 1997) and quintile regression (Meyer et al., 1995). Propensity score matching (PSM) helps estimate treatment effects as this method can balance measured covariates across groups (treatment and control) and better estimate the counterfactual for treated individuals (Rosenbaum and Rubin, 1983; Imbens, 2004; Austin, 2011). Kernel propensity-score weights complement the DID treatment effect model. This matching technique (also known as kernel weighting) is beneficial when other matching strategies are not viable for analyzing survey data with sampling weights or continuous or multilevel categorical treatments (Garrido et al., 2014). Villa (Villa, 2016) suggested that, by following Heckman, Ichimura, and Todds’ study (Heckman et al., 1997; Heckman et al., 1998), besides the inclusion of control variables, observed covariates can be used to estimate the propensity score (the likelihood of being treated) and calculate kernel weights. Thus, this alternative approach matches treated and control units based on their propensity score instead accounting for control variables. Each treated unit is matched to the whole sample of control units instead of a limited number of nearest neighbors. To begin, one obtains the propensity score
As explained by Heckman, Ichimura, and Todd, kernel matching is an averaging method that reuses and weights all the comparison group observations in the treatment sample (Heckman et al., 1997). Comparison individuals are weighted by their distance in propensity score from treated individuals within a range, or bandwidth, of the propensity score (Garrido et al., 2014; Villa, 2016). Thus, the kernel weights can be defined,
In Equation 3,
To obtain a kernel propensity-score matching DID treatment effect, the kernel weights (presented in Eq. 3) are then introduced into Equation 1 is presented below:
However, in increasing the internal validity of the DID estimand, it is possible to restrict (Phillips, 2021) to the common support (the overlapping region of the propensity for treated and control groups) of the propensity score for both groups. This sample of
Blundell and Dias have stated that in case of inability to follow treated and control units over the baseline and follow-up phases, the DID treatment effects can be estimated with repeated cross-sections (Blundell and Dias, 2009). This is very common when a treatment has been administered to specific regional or demographic groups over several cross-sections. The kernel propensity score matching with repeated cross-section DID treatment effects thus can be expressed as,
Here,
Besides, the balancing property of the treated and the control can be tested through DID estimates. Given the availability of observable covariates, it can be shown that in the absence of the treatment, the outcome variable is orthogonal to the treatment indicator given the set of covariates. In other words, the balancing property can be tested in the baseline as,
DID estimation also necessitate satisfying the ‘parallel or common trend’ test. Under the trend assumption, in the absence of treatment, the average outcome changes from any pre-treatment period to any post-treatment period for the treated is equal to the equivalent average outcome change for the controls. In pre-treatment trend differentials, it is customary to adjust the econometric specification to try to accommodate for those differences (Mora and Reggio, 2015).
The parallel trends assumption can be tested graphically or by performing a test on the linear-trends model coefficient that captures the differences in the trends between treated and controls. The specification of the linear-trends model test, adapted from the study conducted by Cai (Cai, 2016), is as follows:
Where
Finally, to grasp the impact of solar irrigation on farmers’ irrigation costs, we consider the following econometric expression:
Irrigation cost,
Besides, the econometric illustration of estimating adoption impact on ROI can be expressed as:
In Equations 9, 10, the variable ‘Y′ is the outcome variable for ‘Irrigation cost’ and ‘ROI,’ respectively. ‘Year’ represents time trend. “Treatment” represents treated and control groups. The “Year*Treatment” variable denotes the DID estimand; “Explanatory variables” represent respondents’ socio-economic characteristics, and
Table 1 in below, presents the DID basic variables Year and Treatment (Card and Krueger, 1994; Villa, 2016). The “Year” variable denotes the pre-treatment, treatment start, and follow-up periods. The end line of the research is the year 2021. The “Treatment” variable is the segmentation by the treatment and control groups.
The outcome variables for this study are irrigation cost and ROI. The irrigation cost for solar and electricity-based irrigation system adopters was calculated based on the fee that individual farmers paid per 50 decimals. For diesel irrigation adopters, the cost was calculated based on diesel machine rent that the farmer pays each season and the total diesel cost per 50 decimal. However, for a farmer who owns a diesel machine, the cost was calculated based on the total amount of diesel used per 50 decimal and the repairing cost that an individual farmer paid each season. All cost is measured in Taka (the Bangladeshi currency) and then converted to logarithmic forms to calculate the cost increase or decrease percentage. On the other hand, the ROI is the ratio of total return to the variable costs, calculated based on the study conducted by the Bangladesh Rice Research Institutes (BRRI) agricultural economic division entitled ‘Estimation of costs and return of MV rice cultivation at the farm level’ (BRRI, 2021).
The explanatory variables chosen for this study were based on the existing literature on technology adoption (Albrecht and Ladewig, 1985; Caswell et al., 2001; Pandey and Mishra, 2004; Simtowe and Zeller, 2006; Tiwari et al., 2008; Deressa et al., 2011; Idrisa et al., 2012; Genius et al., 2013; Reza and Hossain, 2013; Challa and Tilahun, 2014; Mottaleb et al., 2016; Chuchird et al., 2017; Ntshangase et al., 2018; Sunny et al., 2018; Zeng et al., 2018; Sarker et al., 2021; Sunny et al., 2022a; Sunny et al., 2022b; Sunny et al., 2022c), and their description are given in Table 1.
The Chi-square and F-test were performed to check if any significant difference exists between the treatment and control groups. Panel logit regression using the “xtlogit” command was performed to determine the influential factors of adoption. In order to satisfy the pre-requisite of DID estimation parallel trend test was conducted. This test asserts that the group participating in the program would have experienced a similar change in the outcome variable between the pre-program and the post-program periods as those not participating. If this assumption holds and we can credibly rule out any other over-time changes that may confound the treatment, then the estimators are highly reliable ((Lechner, 2011). We used the ‘diff’ command to estimate PSM-DID (Villa, 2016). We chose kernel matching with the Epanechnikov kernel function and used the bandwidth .03 and .06 (DiNardo and Tobais, 2001; Caliendo and Kopeinig, 2008; Islam et al., 2019). The bootstrapped application was applied with 1,000 repetitions of resampling (Wooldridge, 2012). Besides checking overlap and common support, we also conducted balancing tests on the differences in means after matching. For the robustness check, the ‘xtdidregress’ command was used to estimate the time and panel fixed effect DID and ‘drdid’ for estimating doubly robust DID (StataCorp, 2021; Sant’Anna and Zhao, 2020). All the analysis was performed through software for statistics and data science (STATA) version 17.0. Finally, these analysis results have been presented using frequency tables and cross-tabulations.
Prior studies have suggested that the technology adoption among smallholder farmers is generally influenced by their socio-economic, environmental, and institutional profiles (Albrecht and Ladewig, 1985; Feder et al., 1985; Alauddin and Tisdell, 1988). Descriptive statistics of respondents’ important socio-economic characteristics were analyzed to understand the factors affecting adoption decisions. Among the total respondents, 51.4% belong to the treatment group. The χ2 and F-test result in Table 2 below indicates significant differences between treatment and control groups based on irrigation cost, ROI, educational background, land ownership, farmlands typology, farming experience, knowledge level, fee opinion, soil water retention condition, irrigation machinery ownership, close acquaintances’ adoption, environmental awareness, and secondary income status.
The mean irrigation cost of the control group is higher than the treatment group, but their ROI is lower than the treatment group. Among the total respondents, 47.98% of the treatment group respondents’ age is higher than 30 years, and the treatment group respondents’ literacy rate is nearly 2.7% higher than the control group. 79.75% of farmers cultivate on mid-low land, while 5.43% do not possess land ownership rights. The average farming experience and the farm size for control group farmers is 7.34% higher and .87% larger than the treatment group. Among 42.89% of households with more than four members, 22.50% belong to the treatment and the rest, 20.39%, belong to the control group. Even though 40.04% of treatment and 35.41% of control group farmers hold proper knowledge of SIF technology, 56.65% of total respondents, including 61.49% control and 52.06% treatment respondents, did not know SIFs adoption aids the environment. Regarding fee opinion, 22.47% of control and 30.51% of treatment group farmers, compared to the rest (47.02%), think the acquisition cost is not high. 45.68% of our respondent farmers have also reported owning other irrigation machinery. Among 1,059 respondents whose close acquaintances have adopted SIF holds, 13.44% belong to the control and 23.92% to the treatment group. In addition, 85.61% of farmers’ seasonal off-farm income is more than 25,000 Taka.
The factors influencing farm households’ adoption of solar irrigation facilities were analyzed through panel data logit models, and the results are presented below (Table 3). The marginal effects were estimated, as the coefficient result does not express the probability or magnitude. The calculated Variance Inflation Factor (VIF) ranged from 1.06 to 1.84—well below the conventional threshold of 10, suggesting no issue of multicollinearity (Maddala, 1983).
The estimated marginal effect for the age variable indicates that the receptiveness toward solar irrigation technology increase by .27% if the farmers’ age is below 30 years. Similar findings from prior work (Sunny et al., 2018; Sunny et al., 2022a) suggested that younger farmers’ more vehement nature invigorates them in trying newer innovations. In contrast, higher experience farmers’ cautiousness in technology choices is more highly associated with their knowledge of the technology and the expected return against investment aspects.
Land typology results demonstrate that farmers cultivating in mid-high land are 19.8% more likely to adopt SIF than low-midland cultivators. A prior study states that at higher relative landscape positions, water tends to drain more quickly (Krupnik et al., 2017), and Boro rice cultivation requires an adequate and timely water supply (Sunny et al., 2022c). Therefore, farmers cultivating in mid-high land are more likely to adopt SIF.
The negative marginal effect value signified that the adoption chance of SIF decreases to .15% when a household size is more than four people. Similar findings suggested that the consumption need of a larger household tends to compete with the investment of new technology adoption (Sunny et al., 2022a).
As expected, the marginal effect value suggests that farmers possessing proper SIF knowledge have a .9% higher probability of adopting the technology. Our result matches with prior study findings that suggested knowledge about a specific technology helps farmers to develop insights into the consequences of each option and can counterbalance the negative effect of a lack of years of formal education in the overall decision to adopt a technology (Sunny et al., 2018).
The marginal effects result of the ‘Fee opinion’ predictor indicates that farmers who urge for more reduced service fees are .07% more likely to adopt SIF. Our descriptive statistics also revealed that approximately 54% of control farmers believed that solar irrigation service fees were excessive. Therefore, the relevant authorities must take appropriate measures regarding acquisition fees so that the scheme can attract more farmers and operational organizations and farmers’ possibility of achieving higher economic returns does not diminish.
The negative and significant ‘Soil fertility’ predictor indicates that a farmer with the greater belief that their farmland soil is fertile is .06% less likely to adopt SIF. This result is coherent with findings stating that soil fertility perceptions for Bangladeshi farmers are not fundamentally based on scientific classifications of soil composition (e.g., soil nutrient composition) but on perceived yield (Sunny et al., 2022b).
The marginal effect of “secondary income” indicates that the likelihood of adoption is .67% higher for farmers with higher secondary income than their counterparts. This result confirms earlier studies’ findings that higher off-farm income influences new technology adoption (Rahman et al., 2021; Sunny et al., 2022b)). However, farmers having no cash constraints during the cropping season have .37% less probability of being SIF adopters. This finding is meaningful because loan availability does not indicate that the farmers have utilized that money for irrigation purposes and not to avail other essential inputs (i.e., fertilizer, pesticide, and herbicides) (Rizwan et al., 2019; Ouattara et al., 2020).
Finally, the marginal effect indicates that farmers knowing that SIF acceptance will aid in carbon footprint reduction are .12% more likely to adopt SIF. This result matches previous research outcomes suggesting that environmental knowledge positively impacts environmental attitudes and environmental attitudes influence behavioral intentions towards the environment. Thus, behavioral intentions toward the environment positively affect pro-environmental behavior (Liu et al., 2020).
Before finalizing, we tested the appropriateness of the models. Hence, we first checked the parallel trend assumption through the graphical representation. The observed means and the linear-trends model over the pretreatment periods indicate that the trends are parallel (Figures 1A,B). Besides, the insignificant F value for the ROI (.73) and Irrigation cost (.32) in Table 4 also suggested the appropriateness of employing the difference-in-differences method. Besides, within the PSM-DID framework, we check the matching quality based on the common support. The common support is the overlap interval of the propensity scores for the treated and control groups. The findings revealed a significant overlap in the propensity scores of treatment and control group respondents, suggesting better matching quality condition is met showed in Figure 2; Figure 3 (before and after matching). The balancing test was also performed to compare the balance of the pre-existing variables between the treatment and the control groups after matching. The test result indicated that the mean bias reduces from 12.7 to 4.2 after matching, which indicates that the propensity score matching method reduces the differences between treatment and control groups and eliminates the biases (Table 5; Table 6).
Tables 7 below represent the PSM-DID estimates for the impact of SIF adoption on ROI and irrigation cost. The findings show that ROI increased by 20%–30% and irrigation costs reduced by 21%–30% for treatment group farmers (adopters) compared to the control group. The findings match studies documenting solar irrigation adoption benefits in water-stressed areas (Hossain and Karim, 2020; Sunny et al., 2022a).
The positive impact of adoption has significant contributions to the energy sector. The recent energy crisis is not unexpected when considering global geopolitical matters. About 320,000 pumps are run by electricity to irrigate crops on a total of 54.48 lakh hectares in the dry season, which consumes approximately 2000 MW of electricity (Kanojia, 2019). Due to Bangladesh’s energy crisis, the government has decided not to sanction new electricity connections for irrigation. A recent cost comparison study shows that with falling prices, solar irrigation systems have become competitive with grid electricity, while with increasing diesel prices, diesel-based irrigation is getting more expensive (Haque, 2022). Hence, Bangladesh must strongly take initiatives to keep the agricultural sector free from the negative impact of global diesel and other fossil fuel prices’ oscillation and availability issues. The country uses between 15% and 20% of the grid electricity for irrigation purposes. Hence, installing enough solar-based irrigation systems to offset this loss and utilize this energy in other sectors seems more logical.
Studies in India revealed that solar irrigation system adoption not only satisfies farmers’ water requirement for irrigation but also provides an incentive to economies for their energy and contributes to the energy sector by supplying unused energy to the grid (Patil, 2017). Another study revealed that the total power needed for irrigation in southern Europe is 16 GW; substituting this with solar power could offset over 16 million tons of CO2 yearly (Gillman, 2017). Likewise, adopting a solar irrigation system in Spain has increased yield by 35% and reduced energy consumption by 478 MW h annually, delivering 52 TEUR/year financial savings (Danfoss, 2020). Therefore, scale-up SIF adoption can contribute significantly to enabling a sustainable supply of food, energy, and water, particularly in water-stressed areas.
We conducted several robustness checks to confirm our main results using fixed effect DID and doubly robust DID estimation methods presented in Table 8.
Even though Table 7 result in the above slightly differs from Table 8 results in terms of the magnitude of the ATT, the results are similar in terms of ATT’s sign and effect. Both tables’ results suggested that SIF significantly increases ROI and reduces irrigation costs, confirming that the PSM-DID estimates are robust.
Table 9 below shows that before 2018 none of the farmers complained about service quality. However, 7.3% of adopters in 2018, 11.7% in 2019, 10.7% in 2020, and 20% in 2021 stated dissatisfaction with the site operators’ behavior and performance. These farmers reported that many site operators practice partiality by providing water to their close acquaintances first, and sometimes they do not care about farmers’ priority.
Likewise, around 4.9% of farmers in 2018, 7.8% in 2019, 13.7% in 2020, and 15.1% in 2021 were unhappy with the solar irrigation systems’ performance as the system fails to supply adequate water in the cloudy period and to mitigate the issue, diesel pumps require reinstating. Similarly, 2.9% in 2020% and 4.9% in 2021 expressed disappointment with the service providers’ indifferent attitude toward valuing farmers’ views delaying support issues.
Since farmers were not satisfied with site operators, it would be worth knowing what their counterparts think. Among 30 site operators, 63% stated that not allowing adopted farmers to pay less is the main reason for their dissatisfaction. Further, 23.33% expressed that it becomes difficult to satisfy everyone when water requirements are high in the dry season. The rest, 13.33%, indicated that delay in repairing work due to a lack of skilled workforce is associated with dissatisfaction.
While discussing the challenges, five site operators reported that from 2020 they have been encountering steeling issues with cables and solar panels. They reasoned that the diffusion of solar irrigation facilities hampers diesel and electric pump owners’ businesses, making them unhappy. Apart from highlighting the need to deal with theft, these findings also urge initiatives for solar technicians’ skill development training in remote areas.
This study examines the impact of solar irrigation facilities adoption on rural household welfare indicators (i.e., irrigation cost and ROI), using panel studies data on 2,835 households from 2015 to 2021. The results of the ATT estimates exhibited a positive impact of SIF adoption on irrigation cost and ROI.
This study’s finding has practical policy implications. Firstly, the beneficial effect of SIF adoption highlighted the need for the government, investors, and shareholders greater focus on designing more appropriate schemes through experimentation and multiple iterations. However, to do so, ministries and agencies responsible for reforming and implementing customs duties, tariffs, and tax incentives need to reassess the market condition and find a solution to minimize the bureaucratic complexity for technology producers and distributors. It should be cognizant that the benefactors’ loan repayment and the sustainability of the operating company depend on generating satisfactory revenue, which is only possible through appropriate site selection. Therefore, before finalizing the site, the responsible organizations should extensively study farmers’ seasonal crop-choosing patterns, future underground pipeline expansion plans, soil slope, potential customers’ attitudes regarding acquisition cost and perceptions towards SIF, and the market price of water-intensive crops. Because shifting the solar site from one place to another would not be cost-effective once the installation is done. Anecdotal evidence from service providers and site operators suggested that our study area farmers’ crop cultivation patterns depend on earlier years’ crop market prices. Likewise, private actors and public agencies need more information and tools to access water resource availability and soil water retention condition to enable more effective and sustainable solar irrigation investment planning. National implementing and regulatory agencies require more robust monitoring capacities. At the same time, the education sector needs to contribute to solar development efforts through training programs and capacity building to expand solar energy and solar irrigation. It is also essential to understand that the schemes to scale up of adoption process must be appealing enough to create strong demand from farmers.
Secondly, respondents’ concern regarding SIF performance indicates solar panels’ efficiency issues. Even though the project report states that these’ panels’ estimated shelf life is 10 years, the farmers are experiencing considerable efficiency decreases in the first 5 years of use. Thus, it seems that there is a need to extensively investigate, develop, and improve the technologies involved while emphasizing the technology’s quality and after-sales service support. Likewise, substituting polycrystalline solar panels with copper bismuth oxide absorber-based thin-film solar cells or mono-crystalline panels will avoid reinstating diesel irrigation systems on peak time and can enhance the SIFs efficiency. These adjustments, nevertheless, need extra funding. Therefore, authorities should consider raising the tenure and grace period from 10 years to at least 20 years and facilitating lower interest rates than the banks offer for general projects.
Thirdly, SIF adoption, apart from contributing to farmers’ wellbeing, can play a vital role in resolving future energy crises if the government speeds up the grid-tied Solar System expansion process. Because due to coal and furnace oil supply-chain disruptions, the future electricity production cost is anticipated to rise compared to the present.
Besides, initiatives introducing insurance schemes or safety nets to hedge against potential theft or production risk are expected to boost farmers’ and investors’ confidence and downside risk. In addition, focusing on region-specific installation of the small, medium, and high-capacity SIFs and strict prohibition of mixed types installation in the same region to avoid internal conflicts between services providers should include in policy priority.
Our findings also pointed to the significance of creative management strategies emphasizing field demonstration programs and campaigns to raise environmental consciousness and benefit recipients rather than just adoption. To better understand farmers’ risk management practices, we also call for more research on how people of different ages perceive SIF and their knowledge of environmental severity.
Finally, implementing farm or community-level evidence-based best practices on solar irrigation solutions while considering the watershed scales and founded on principles of natural resource sustainability and equity will advance us towards achieving a sustainable food production sector.
The original contributions presented in the study are included in the article/Supplementary Material, further inquiries can be directed to the corresponding author.
FS and MI planned, designed, analyzed and interpreted the data. FS, TK, and JL wrote the first draft. JL, MR, and HZ critically reviewed the manuscript that went through multiple revisions by FS, TK, MI, and MR. All authors read and approved the final manuscript.
The authors declare that the research was conducted in the absence of any commercial or financial relationships that could be construed as a potential conflict of interest.
All claims expressed in this article are solely those of the authors and do not necessarily represent those of their affiliated organizations, or those of the publisher, the editors and the reviewers. Any product that may be evaluated in this article, or claim that may be made by its manufacturer, is not guaranteed or endorsed by the publisher.
The Supplementary Material for this article can be found online at: https://www.frontiersin.org/articles/10.3389/fenrg.2022.1101404/full#supplementary-material
ADB (2018). 4 million to spur off-grid solar driven pumping for irrigation in Bangladesh. Philippines: Asian Development Bank-ADB. [Internet].
Afrin, R., Hossain, F., and Mamun, S. A. (2019). Analysis of drought in the northern region of Bangladesh using standardized precipitation index (SPI). Environ. Sci. Nat. Resour. 11 (1 and 2), 199–216. doi:10.3329/jesnr.v11i1-2.43387
Agrawal, S., and Jain, A. (2018). Sustainable deployment of solar irrigation pumps: Key determinants and strategies. WIREs Energy Env. 8 (2), e325. doi:10.1002/wene.325
Alam, Md.J., Mahmud, A. A., Islam, Md.A., Hossain, Md.F., Ali, Md.A., Dessoky, E. S., et al. (2021). Crop diversification in rice—based cropping systems improves the system productivity, profitability and sustainability. Sustainability 13 (11), 6288. doi:10.3390/su13116288
Alaofè, H., Burney, J., Naylor, R., and Taren, D. (2016). Solar-powered drip irrigation impacts on crops production diversity and dietary diversity in northern Benin. Food Nutr. Bull. 37 (2), 164–175. doi:10.1177/0379572116639710
Alauddin, M., and Tisdell, C. (1988). Dynamics of adoption and diffusion of HYV technology: New evidence of inter-farm differences in Bangladesh. New South Wales: University of Newcastle, Department of Economics.
Albrecht, D. E., and Ladewig, H. (1985). Adoption of irrigation technology: The effects of personal, structural, and environmental variables. J. Rural. Soc. Sci. 3 (1), 26–41.
Alem, Y., and Broussard, N. H. (2018). The impact of safety nets on technology adoption: A difference-in-differences analysis. Agric. Econ. 49 (1), 13–24. doi:10.1111/agec.12392
Ali, B. (2018). Comparative assessment of the feasibility for solar irrigation pumps in Sudan. Renew. Sustain Energy Rev. 81 (1), 413–420. doi:10.1016/j.rser.2017.08.008
Asfaw, S., Shiferaw, B., Simtowe, F., and Haile, M. (2011). Agricultural technology adoption, seed access constraints and commercialization in Ethiopia. J. Dev. Agric. Econ. 3 (9), 436–477.
Asfaw, S., Shiferaw, B., Simtowe, F., and Lipper, L. (2012). Impact of modern agricultural technologies on smallholder welfare: Evidence from Tanzania and Ethiopia. Food Policy 37 (3), 283–295. doi:10.1016/j.foodpol.2012.02.013
Austin, P. C. (2011). An introduction to propensity score methods for reducing the effects of confounding in observational studies. Multivar. Behav. Res. 46 (3), 399–424. doi:10.1080/00273171.2011.568786
Barreto, H. J., and Bell, M. A. (1994). Assessing risk associated with N fertilizer recommendations in the absence of soil tests. Fertil. Res. 40 (3), 175–183. doi:10.1007/bf00750463
BBS (2020). Estimate of major crops. [Internet]. Available from: http://www.bbs.gov.bd/site/page/453af260-6aea-4331-b4a5-7b66fe63ba61/Agriculture. [cited 2021 Aug 12].
BBS, WFP (2020). Poverty maps of Bangladesh 2016. Dhaka, Bangladesh: Bangladesh Bureau of Statistics (BBS) and World Food Program-WFP. [Internet].
Becerril, J., and Abdulai, A. (2010). The impact of improved maize varieties on poverty in Mexico: A propensity score-matching approach. World Dev. 38 (7), 1024–1035. doi:10.1016/j.worlddev.2009.11.017
BGEF (2016). Solar irrigation pump [internet]. Available from: https://www.greenenergybd.com/sip.php. [cited 2018 Jun 1].
Biswas, H., and Hossain, F. (2013). Solar pump: A possible solution of irrigation and electric power crisis of Bangladesh. Int. J. Comput. Appl. 62 (16), 1–5. doi:10.5120/10161-4780
Blundell, R., and Dias, M. C. (2009). Alternative approaches to evaluation in empirical microeconomics. J. Hum. Resour. 44 (3), 565–640. doi:10.1353/jhr.2009.0009
BRRI (2021). Annual report of Bangladesh rice research Institute 2019-2020. Dhaka, Bangladesh: Bangladesh Rice Research Institute-BRRI. [Internet].
Cai, J. (2016). The impact of insurance provision on household production and financial decisions. Am. Econ. J. Econ. Policy 8 (2), 44–88. doi:10.1257/pol.20130371
Caliendo, M., and Kopeinig, S. (2008). Some practical guidance for the implementation of propensity score maching. J. Econ. Surv. 22 (1), 31–72. doi:10.1111/j.1467-6419.2007.00527.x
Campana, P. E., Leduc, S., Kim, M., Olsson, A., Zhang, J., Liu, J., et al. (2017). Suitable and optimal locations for implementing photovoltaic water pumping systems for grassland irrigation in China. Appl. Energy 185 (2), 1879–1889. doi:10.1016/j.apenergy.2016.01.004
Card, D., and Krueger, A. B. (1994). Minimum wages and employment: A case study of the fast-food industry in New Jersey and Pennsylvania. Am. Econ. Rev. 84 (4), 772–793.
Caswell, M., Fuglie, K., Ingram, C., Jans, S., and Kascak, C. (2001). Adoption of Agricultural production practices: Lessons learned from the US. Washington DC: US Department of Agriculture, Resource Economics Division, Economic Research Service.
Challa, M., and Tilahun, U. (2014). Determinants and impacts of modern agricultural technology adoption in west wollega: The case of gulliso district. J. Biol. Agric. Healthc. 4 (20), 63–77.
Chowdhury, B., Ula, S., and Stokes, K. (1993). Photovoltaic-powered water pumping - design, and implementation: Case studies in Wyoming. IEEE Trans. Energy Convers. 8 (4), 646–652. doi:10.1109/60.260976
Chuchird, R., Sasaki, N., and Abe, I. (2017). Influencing factors of the adoption of agricultural irrigation technologies and the economic returns: A case study in chaiyaphum province, Thailand. Sustainability 9 (9), 1524. doi:10.3390/su9091524
Coady, D. P. (1995). An empirical analysis of fertilizer use in Pakistan. Economica 62 (246), 213–234. doi:10.2307/2554904
Danfoss (2020). Solar irrigation pump lowers emissions and saves energy [Internet]. Available from: https://www.danfoss.com/en/service-and-support/case-stories/dds/solar-irrigation-pump-lowers-emissions-and-saves-energy/. [cited 2022 Oct 15].
Deressa, T. T., Hassan, R. M., and Ringler, C. (2011). Perception of and adaptation to climate change by farmers in the Nile basin of Ethiopia. J. Agric. Sci. 149 (1), 23–31. doi:10.1017/s0021859610000687
DiNardo, J., and Tobais, J. L. (2001). Nonparametric density and regression estimation. J. Econ. Perspect. 15 (4), 11–28. doi:10.1257/jep.15.4.11
Doby, L. (2018). Spreading solar irrigation in Bangladesh. Available from: https://borgenproject.org/spreading-solar-irrigation-in-bangladesh/. [cited 2018 Dec 30].
Dong, W., Zhang, X., Wang, H., Dai, X., Sun, X., Qiu, W., et al. (2012). Effect of different fertilizer application on the soil fertility of paddy soils in red soil region of southern China. Plos One 7 (9), e44504. doi:10.1371/journal.pone.0044504
Duflo, E., Kremer, M., and Robinson, J. (2011). Nudging farmers to use fertilizer: Theory and experimental evidence from Kenya. Am. Econ. Rev. 101 (6), 2350–2390. doi:10.1257/aer.101.6.2350
Encyclopedia (2018). Wikipedia, Dinajpur district, Bangladesh. Bangladesh: Wikipedia, the free encyclopedia.
Energypedia (2020). Powering agriculture: Irrigation [internet] energypedia. Available from: https://energypedia.info/wiki/Powering_Agriculture:_Irrigation. [cited 2021 May 10].
Ershadullah, Md (2021). Solar irrigation pumps: Transforming to smart irrigation and improving agriculture in Bangladesh. Available from: https://smartwatermagazine.com/blogs/md-ershadullah/solar-irrigation-pumps-transforming-smart-irrigation-and-improving-agriculture. [cited 2021 Dec 10].
Fanus, A., Aregay, F., and Minjuan, Z. (2012). Impact of irrigation on fertilizer use decision of farmers in China: A case study in weihe river basin. J. Sustain Dev. 5 (4), 74–82. doi:10.5539/jsd.v5n4p74
FAO (2019). Prospects for solar-powered irrigation systems in developing countries. Rome, Italy: Food and Agriculture Organization of the United Nations. [Internet].
FAO (1989). The state of food and agriculture. World and regional reviews. Sustainable development and natural resource management. Rome, Italy: FAO. [Internet].
Feder, G., Just, E. R., and Zilberman, D. (1985). Adoption of agricultural innovations in developing countries: A survey. Econ. Dev. Cult. Change 33 (2), 255–298. doi:10.1086/451461
Foster, T., Adhikari, R., Urfels, A., Adhikari, S., and Krupnik, T. J. (2019). Costs of diesel pump irrigation systems in the Eastern Indo-Gangetic Plains: What options exist for efficiency gains? Washington, D.C: International Food Policy Research Institute IFPRI. [Internet].
García, A. M., Gallagher, J., McNabola, A., Poyato, E. C., Barrios, P. M., and Díaz, J. A. R. (2019). Comparing the environmental and economic impacts of on- or off-grid solar photovoltaics with traditional energy sources for rural irrigation systems. Renew. Energy 140, 895–904. doi:10.1016/j.renene.2019.03.122
Garrido, M. M., Kelley, A. S., Paris, J., Roza, K., Meier, D. E., Morrison, R. S., et al. (2014). Methods for constructing and assessing propensity scores. Health Serv. Res. 49 (5), 1701–1720. doi:10.1111/1475-6773.12182
Genius, M., Koundouri, P., Nauges, C., and Tzouvelekas, V. (2013). Information transmission in irrigation technology adoption and diffusion: Social learning, extension services, and spatial effects. Amer J. Agr. Econ. 96 (1), 328–344. doi:10.1093/ajae/aat054
Guno, C. S., and Agaton, C. B. (2022). Socio-economic and environmental analyses of solar irrigation systems for sustainable agricultural production. Sustainability 14 (11), 6834. doi:10.3390/su14116834
Haque, A. (2022). Solar irrigation systems are gaining popularity, but challenges remain. Bus. Stand., 21.
Hasnat, Md.A., Hasan, M. N., and Hoque, N. (2014) A brief study of the prospect of hybrid solar irrigation system in Bangladesh. Khulna, Bangladesh: Khulna University of Engineering and Technology-KUET.
Heckman, J. J., Ichimura, H., Smith, J., and Todd, P. (1998). Characterizing selection bias using experimental data. Econometrica 66 (5), 1017–1098. doi:10.2307/2999630
Heckman, J. J., Ichimura, H., and Todd, P. E. (1997). Matching as an econometric evaluation estimator: Evidence from evaluating a job training programme. Rev. Econ. Stud. 64 (4), 605–654. doi:10.2307/2971733
Hess, S., Daly, A., and Batley, R. (2018). Revisiting consistency with random utility maximisation: Theory and implications for practical work. Theory Decis. 84, 181–204. doi:10.1007/s11238-017-9651-7
Hoque, N., Roy, A., Beg, M. R. A., and Das, B. K. (2016). Techno-economic evaluation of solar irrigation plants installed in Bangladesh. Int. J. Renew. Energy Dev. 5 (1), 73–78. doi:10.14710/ijred.5.1.73-78
Hossain, M. A., Hassan, M. S., Mottaleb, M. A., and Hossain, M. (2015). Feasibility of solar pump for sustainable irrigation in Bangladesh. Int. J. Energy Environ. Eng. 6, 147–155. doi:10.1007/s40095-015-0162-4
Hossain, Md.I., Bari, Md.N., and Miah, Md.S. U. (2021). Opportunities and challenges for implementing managed aquifer recharge models in drought-prone Barind tract, Bangladesh. Appl. Water Sci. 11 (12), 181. doi:10.1007/s13201-021-01530-1
Hossain, M., and Karim, A. (2020). Does renewable energy increase farmers’ well-being? Evidence from solar irrigation interventions in Bangladesh. Tokyo: Asian Development Bank Institute-ADBI. [Internet].
Hoyos, D. (2010). The state of the art of environmental valuation with discrete choice experiments. Ecol. Econ. 69, 1595–1603. doi:10.1016/j.ecolecon.2010.04.011
Idrisa, Y. L., Ogunbameru, B. O., and Madukwe, M. C.Department of Agricultural Extension, and University of Nigeria (2012). Logit and Tobit analyses of the determinants of likelihood of adoption and extent of adoption of improved soybean seed in Borno State, Nigeria. Greener J. Agric. Sci. 2 (2), 037–045. doi:10.15580/gjas.2013.3.1231
Imbens, G. W. (2004). Nonparametric estimation of average treatment effects under exo-geneity: A review. Rev. Econ. Stat. 86 (1), 4–29. doi:10.1162/003465304323023651
IRENA (2016). Solar pumping for irrigation: Improving livelihoods and sustainability. Abu Dhabi: The International Renewable Energy Agency. [Internet].
Islam, M. A., Rahman, M. C., Sarker, M. A., and Siddique, M. A. B. (2019). Assessing impact of BRRI released modern rice varieties adoption on farmers’ welfare in Bangladesh: Application of panel treatment effect model. Bangladesh Rice J. 23 (1), 1–11. doi:10.3329/brj.v23i1.46076
Islam, Md.T., and Hossain, Md.E. (2022). Economic feasibility of solar irrigation pumps: A study of northern Bangladesh. Int. J. Renew. Energy Dev. 11 (1), 1–13. doi:10.14710/ijred.2022.38469
Islam, M. S., Samreth, S., Islam, A. H. M. S., and Sato, M. (2022). Climate change, climatic extremes, and households’ food consumption in Bangladesh: A longitudinal data analysis. Environ. Chall. 7, 100495. doi:10.1016/j.envc.2022.100495
Islam, S. M. S., Islam, K. M. A., and Mullick, M. R. A. (2022). Drought hot spot analysis using local indicators of spatial autocorrelation: An experience from Bangladesh. Environ. Chall. 6, 100410. doi:10.1016/j.envc.2021.100410
Khonje, M. G., Manda, J., Mkandawire, P., Tufa, A. H., and Alene, A. D. (2018). Adoption and welfare impacts of multiple agricultural technologies: Evidence from eastern Zambia. Agric. Econ. 49 (5), 599–609. doi:10.1111/agec.12445
Khonje, M., Manda, J., Alene, A. D., and Kassie, M. (2015). Analysis of adoption and impacts of improved maize varieties in eastern Zambia. World Dev. 66, 695–706. doi:10.1016/j.worlddev.2014.09.008
Kleemann, L., Abdulai, A., and Buss, M. (2014). Certification and access to export markets: Adoption and return on investment of organic-certified pineapple farming in Ghana. World Dev. 64, 79–92. doi:10.1016/j.worlddev.2014.05.005
Krejcie, R. V., and Morgan, D. W. (1970). Determining sample size for research activities. Educ. Psychol. Meas. 38, 607–610. doi:10.1177/001316447003000308
Krupnik, T. J., Schulthess, U., Ahmed, Z. U., and McDonald, A. J. (2017). Sustainable crop intensification through surface water irrigation in Bangladesh? A geospatial assessment of landscape-scale production potential. Land Use Policy 60, 206–222. doi:10.1016/j.landusepol.2016.10.001
Kumar, V., Hundal, B. S., and Kaur, K. (2019). Factors affecting consumer buying behaviour of solar water pumping system. Smart Sustain Built Environ. 8 (4), 351–364. doi:10.1108/sasbe-10-2018-0052
Kumar, V., Syan, A. S., Kaur, A., and Hundal, B. S. (2020). Determinants of farmers’ decision to adopt solar powered pumps. Int. J. Energy Sect. Manag. 14 (4), 707–727. doi:10.1108/ijesm-04-2019-0022
Lancaster, K. (1966). A new approach to consumer theory. J. Polit. Econ. 74 (2), 132–157. doi:10.1086/259131
Lechner, M. (2011). The estimation of causal effects by difference-in-difference MethodsEstimation of spatial panels. Found. Trends® Econom. 4 (3), 165–224. doi:10.1561/0800000014
Liu, P., Teng, M., and Han, C. (2020). How does environmental knowledge translate into pro-environmental behaviors?: The mediating role of environmental attitudes and behavioral intentions. Sci. Total Environ. 728 (2), 138126. doi:10.1016/j.scitotenv.2020.138126
Maddala, G. S. (1983). Limited-dependent and qualitative variables in econometrics. New York: Cambridge University Press.
Manda, J., Khonje, M. G., Alene, A. D., Tufa, A. H., Abdoulaye, T., Mutenje, M., et al. (2020). Does cooperative membership increase and accelerate agricultural technology adoption? Empirical evidence from Zambia. Technol. Forecast Soc. Change 158, 120160. doi:10.1016/j.techfore.2020.120160
Manski, C. F. (1977). The structure of random utility models. Theory Decis. 8 (1), 229–254. doi:10.1007/bf00133443
Martey, E., Wiredu, A. N., Etwire, P. M., Fosu, M., Buah, S. S. J., Bidzakin, J., et al. (2013). Fertilizer adoption and use intensity among smallholder farmers in northern Ghana: A case study of the agra soil health project. Sustain Agric. Res. 3 (1), 24–36. doi:10.5539/sar.v3n1p24
McFadden, D. (1974). “Chapter Four: Conditional logit analysis of qualitative choice behavior,” in Frontiers in econometrics. Editor P. Zarembka (New York: Academic Press).
Mendola, M. (2007). Agricultural technology adoption and poverty reduction: A propensity-score matching analysis for rural Bangladesh. Food Policy 32 (3), 372–393. doi:10.1016/j.foodpol.2006.07.003
Meyer, B. D., Viscusi, W. K., and Durbin, D. L. (1995). Workers’ compensation and injury duration: Evidence from a natural experiment. Am. Econ. Rev. 85 (3), 322–340.
Mirta, A., Alam, M. F., and Yashodha, Y. (2021). Solar irrigation in Bangladesh A situation analysis report. Colombo, Sri Lanka: International Water Management Institute IWMI. [Internet].
Mora, R., and Reggio, I. (2015). Didq: A command for treatment-effect estimation under alternative assumptions. Stata J. 15 (3), 796–808. doi:10.1177/1536867x1501500312
Mottaleb, K. A., Krupnik, T. J., and Erenstein, O. (2016). Factors associated with small-scale agricultural machinery adoption in Bangladesh: Census findings. J. Rural. Stud. 46, 155–168. doi:10.1016/j.jrurstud.2016.06.012
Nakano, Y., Tsusaka, T. W., Aida, T., and Pede, V. O. (2018). Is farmer-to-farmer extension effective? The impact of training on technology adoption and rice farming productivity in Tanzania. World Dev. 105, 336–351. doi:10.1016/j.worlddev.2017.12.013
Neuhaus, J. M., Kalbfleisch, J. D., and Hauck, W. W. (1991). A comparison of cluster-specific and population-averaged approaches for analyzing correlated binary data. Int. Stat. Rev. 59 (1), 25–35. doi:10.2307/1403572
Ntshangase, N. L., Muroyiwa, B., and Sibanda, M. (2018). Farmers’ perceptions and factors influencing the adoption of No-till conservation agriculture by small-scale farmers in zashuke, KwaZulu-natal province. Sustainability 10 (2), 555. doi:10.3390/su10020555
Odarno, L. (2017). 1.2 billion people lack electricity. Washington, D.C., United States: World Resources Institute. Increasing Supply Alone Won’t Fix the Problem [Internet].
Ouattara, N., Xiong, X., Traore, L., Turvey, C. G., Sun, R., Ali, A., et al. (2020). Does credit influence fertilizer intensification in rice farming? Empirical evidence from côte D’ivoire. Agronomy 10 (8), 1063. doi:10.3390/agronomy10081063
Pandey, V. L., and Mishra, V. (2004). Adoption of zero tillage farming: Evidences from Haryana and Bihar. SSRN Electron J. 2004, 17. doi:10.2139/ssrn.529222
Patil, M. (2017). Solar irrigation: India’s farmers can sell electricity and save groundwater. Bus. Stand., 5.
Phillips, A. K. (2021). “Unleashing a solar irrigation pump revolution for smallholder farmers in Myanmar,” (Baecelona, Spain: Universitat Politècnica de Catalunya BarcelonaTech-UPC). [Internet] [Master thesis].
Pretty, J. (2008). Agricultural sustainability: Concepts, principles and evidence. Philos. Trans. R. Soc. Lond B Biol. Sci. 363 (1491), 447–465. doi:10.1098/rstb.2007.2163
Qureshi, A. S. (2014). Reducing carbon emissions through improved irrigation management: A case study from Pakistan†. Irrig. Drain. 63, 132–138. doi:10.1002/ird.1795
Rahman, M. S., Kazal, M. M. H., Rayhan, S. J., and Manjira, S. (2021). Adoption determinants of improved management practices and productivity in pond polyculture of carp in Bangladesh. Aquac. Fish. 8 (1), 96–101. doi:10.1016/j.aaf.2021.08.009
Rahman, S. M., Faruk, Md.O., Rahman, Md.H., and Rahman, S. M. (2022). Drought index for the region experiencing low seasonal rainfall: An application to northwestern Bangladesh. Arab. J. Geosci. 15 (3), 277. doi:10.1007/s12517-022-09524-2
Rana, J., Kamruzzaman, M., Oliver, M. H., and Akhi, K. (2021). Financial and factors demand analysis of solar powered irrigation system in Boro rice production: A case study in meherpur district of Bangladesh. Renew. Energy 167, 433–439. doi:10.1016/j.renene.2020.11.100
Rana, M. J., Kamruzzaman, M., Oliver, Md.M. H., and Akhi, K. (2021). Influencing factors of adopting solar irrigation technology and its impact on farmers’ livelihood. A case study in Bangladesh. Future Food J. Food Agric. Soc. 9 (5), 14.
Raza, F., Tamoor, M., Miran, S., Arif, W., Kiren, T., Amjad, W., et al. (2022). The socio-economic impact of using photovoltaic (PV) energy for high-efficiency irrigation systems: A case study. Energies 15 (3), 1198. doi:10.3390/en15031198
Rentschler, J., and Bazilian, M. (2016). Reforming fossil fuel subsidies: Drivers, barriers and the state of progress. Clim. Policy 17 (7), 891–914. doi:10.1080/14693062.2016.1169393
Reza, Md.S., and Hossain, Md.E. (2013). Factors affecting farmers’ decisions on fertilizer use: A case study of rajshahi district in Bangladesh. Bangladesh J. Polit. Econ. 29 (1), 211–221.
Rizwan, M., Ping, Q., Iram, S., Nazir, A., and Wang, Q. (2019). Why and for what? An evidence of agriculture credit demand among rice farmers in Pakistan [internet]. Tokyo: Asian Development Bank Institute-ADBI.
Rosenbaum, P. R., and Rubin, D. B. (1983). The central role of the propensity score in observational studies for causal effects. Biometrika 70 (1), 41–55. doi:10.1093/biomet/70.1.41
Sanap, S., Bagal, S., and Pawar, D. (2020). Factors affecting farmer’s decision of adoption of solar powered pumps. Eur. J. Mol. Clin. Med. 7 (10), 3762–3773.
Sant’Anna, P. H. C., and Zhao, J. (2020). Doubly robust difference-in-differences estimators. J. Econom. 219 (1), 101–122. doi:10.1016/j.jeconom.2020.06.003
Sarker, M. N. I., and Ghosh, H. R. (2017). Techno-economic analysis and challenges of solar powered pumps dissemination in Bangladesh. Sustain Energy Technol. Assess. 20, 33–46. doi:10.1016/j.seta.2017.02.013
Sarker, M. R., Galdos, M. V., Challinor, A. J., and Hossain, A. (2021). A farming system typology for the adoption of new technology in Bangladesh. Food Energy Secur 10 (3), e287. doi:10.1002/fes3.287
Schwanitz, V. J., Piontek, F., Bertram, C., and Luderer, G. (2014). Long-term climate policy implications of phasing out fossil fuel subsidies. Energy Policy 67, 882–894. doi:10.1016/j.enpol.2013.12.015
Shew, A. M., Morat, A. D., Putman, B., Nally, L. L., and Ghosh, A. (2019). Rice intensification in Bangladesh improves economic and environmental welfare. Environ. Sci. Policy 95, 46–57. doi:10.1016/j.envsci.2019.02.004
Simtowe, F., and Zeller, M. (2006). The impact of access to credit on the adoption of hybrid maize in Malawi: An empirical test of an agricultural household model under credit market failure. Available from: https://mpra.ub.uni-muenchen.de/45/. [cited 2018 Apr 29].
SREDA (2022). National database of renewable energy [internet]. Available from: http://www.renewableenergy.gov.bd/index.php?id=01&i=4&s=&ag=&di=&ps=1&sg=&fs=&ob=1&submit=Search. [cited 2022 Feb 4].
SREDA (2015). Scaling up renewable energy in low income countries (SREP), investment plan for Bangladesh. Bangladesh: Sustainable and Renewable Development Authority-SREDA. [Internet].
StataCorp, L. L. C. (2021). STATA Treatment-Effects reference manual: Potential outcome/counterfactual outcomes. Texus: Stata Press. Version17 [Internet].
Sunny, F. A., Fu, L., Rahman, M. S., and Huang, Z. (2022). Determinants and impact of solar irrigation facility (SIF) adoption: A case study in northern Bangladesh. Energies 15 (7), 2460. doi:10.3390/en15072460
Sunny, F. A., Fu, L., Rahman, M. S., Karimanzira, T. T. P., and Zuhui, H. (2022). What influences Bangladeshi Boro rice farmers’ adoption decisions of recommended fertilizer doses: A case study on Dinajpur district. Plos One 17 (6), e0269611. doi:10.1371/journal.pone.0269611
Sunny, F. A., Huang, Z., and Karimanzira, T. T. P. (2018). Investigating key factors influencing farming decisions based on soil testing and fertilizer recommendation facilities (STFRF)—a case study on rural Bangladesh. Sustainability 10 (11), 4331. doi:10.3390/su10114331
Sunny, F. A., Karimanzira, T. T. P., Peng, W., Rahman, M. S., and Zuhui, H. (2022). Understanding the determinants and impact of the adoption of technologies for sustainable farming systems in water-scarce areas of Bangladesh. Front. Sustain Food Syst. 6, 961034. doi:10.3389/fsufs.2022.961034
The Business Standard (2022). Electricity demand may reach 15, 500MW in irrigation season. Bus. Stand., 10.
The World Bank (2018). Access to energy is at the heart of development [internet]. Washington, D.C: The World Bank-IBRD-IDA.
The World Bank (2022). Agriculture, forestry, and fishing, value added (% of GDP). Available from: https://data.worldbank.org/indicator/NV.AGR.TOTL.ZS. [cited 2022 Jan 23].
The World Bank (2021). Employment in agriculture (% of total employment) (modeled ILO estimate). Available from: https://data.worldbank.org/indicator/SL.AGR.EMPL.ZS?locations=BD. [cited 2021 Sep 11].
Tiwari, K. R., Sitaula, B. K., Nyborg, I. L. P., and Paudel, G. S. (2008). Determinants of farmers’ adoption of improved soil conservation technology in a middle mountain watershed of Central Nepal. Environ. Manage 42, 210–222. doi:10.1007/s00267-008-9137-z
United Nations (2015). Transforming our world: The 2030 agenda for sustainable development A/RES/70/1. [Internet]. United Nations. Available from: https://www.unfpa.org/sites/default/files/resource-pdf/Resolution_A_RES_70_1_EN.pdf. [cited 2019 Mar 24].
UNSDG (2022). Global impact of war in Ukraine: Energy crisis - BRIEF NO.3. New York, United States: UN Sustainable Development Group-UNSDG. [Internet].
Villa, J. M. Diff (2016). Diff: Simplifying the estimation of difference-in-differences treatment effects. Stata J. 16 (1), 52–71. doi:10.1177/1536867x1601600108
WFP (2022). Understanding the energy crisis and its impact on food security. Rome, Italy: World Food Programme-WFP. [Internet].
Wooldridge, J. M. (2012). “Appendix 6A. A brief introduction to bootstrapping,” in Introductory econometrics: A modern approach [internet]. Fifth Edition (USA: South-Western).
Wu, H., Ding, S., Pandey, S., and Tao, D. (2010). Assessing the impact of agricultural technology adoption on farmers’ well-being using propensity-score matching analysis in rural China. Asian Econ. J. 24 (2), 141–160. doi:10.1111/j.1467-8381.2010.02033.x
Zeng, D., Alwang, J., Norton, G., Jaleta, M., Shiferaw, B., and Yirga, C. (2018). Land ownership and technology adoption revisited: Improved maize varieties in Ethiopia. Land Use Policy 72, 270–279. doi:10.1016/j.landusepol.2017.12.047
Zheng, H., and Ma, W. (2021). Smartphone-based information acquisition and wheat farm performance: Insights from a doubly robust IPWRA estimator. Electron Commer. Res., 1–26. doi:10.1007/s10660-021-09481-0
Zhou, D., and Abdullah, (2017). The acceptance of solar water pump technology among rural farmers of northern Pakistan: A structural equation model. Cogent Food Agric. 3 (1), 1280882. doi:10.1080/23311932.2017.1280882
Keywords: Energy, Solar Irrigation, Adoption Impact, Panel Data, Difference-in-Difference method, PSM-DID, Fixed effect DID, Doubly robust DID
Citation: Sunny FA, Islam MA, Karimanzira TTP, Lan J, Rahman MS and Zuhui H (2023) Adoption impact of solar based irrigation facility by water-scarce northwestern areas farmers in Bangladesh: Evidence from panel data analysis. Front. Energy Res. 10:1101404. doi: 10.3389/fenrg.2022.1101404
Received: 17 November 2022; Accepted: 30 December 2022;
Published: 12 January 2023.
Edited by:
Alessandro Burgio, Independent researcher, Rende, ItalyReviewed by:
Edward Martey, CSIR-Savanna Agricultural Research Institute, GhanaCopyright © 2023 Sunny, Islam, Karimanzira, Lan, Rahman and Zuhui. This is an open-access article distributed under the terms of the Creative Commons Attribution License (CC BY). The use, distribution or reproduction in other forums is permitted, provided the original author(s) and the copyright owner(s) are credited and that the original publication in this journal is cited, in accordance with accepted academic practice. No use, distribution or reproduction is permitted which does not comply with these terms.
*Correspondence: Juping Lan, bGpwMjg3M0AxNjMuY29t
†These authors have contributed equally to this work
Disclaimer: All claims expressed in this article are solely those of the authors and do not necessarily represent those of their affiliated organizations, or those of the publisher, the editors and the reviewers. Any product that may be evaluated in this article or claim that may be made by its manufacturer is not guaranteed or endorsed by the publisher.
Research integrity at Frontiers
Learn more about the work of our research integrity team to safeguard the quality of each article we publish.