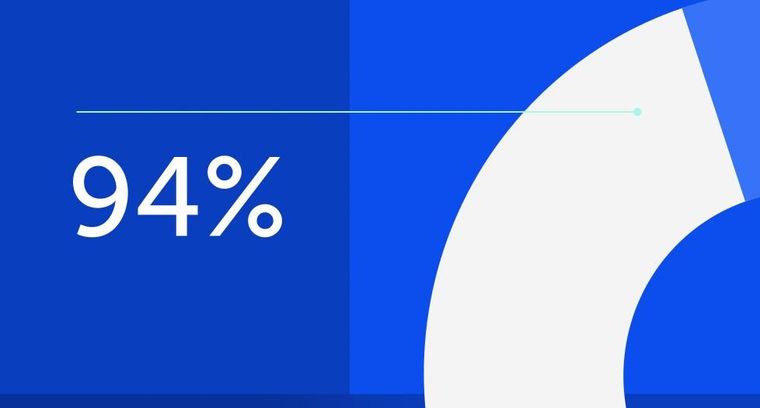
94% of researchers rate our articles as excellent or good
Learn more about the work of our research integrity team to safeguard the quality of each article we publish.
Find out more
REVIEW article
Front. Energy Res., 11 January 2023
Sec. Smart Grids
Volume 10 - 2022 | https://doi.org/10.3389/fenrg.2022.1099890
This article is part of the Research TopicRecent Advances in Renewable Energy Automation and Energy ForecastingView all 14 articles
The rise of electric vehicles (EVs) has a massive impact on the electricity grid due to the electrification of vehicles in the transportation sector. As a result, various techniques are needed to minimize the effects of charging on the grid. One of these techniques is having intelligent coordination between the various components of the EV charging network. This ensures that the network has enough electricity to support the charging needs of the vehicles. This article provides an overview of the many aspects of the EV industry and its charging infrastructure. It also provides a step-by-step approach for implementing the Vehicle to Grid (V2G) deployment, the utilization of recordings from the data by the EV battery through Artificial Intelligence and the cost-benefit analysis from effective utilization of the V2G method. The paper also explores the various aspects of the EV market and the role of aggregators and consumers. Finally, it assesses the possibility of expansion of the EV charging and grid integration system and outlines its challenges and solutions.
Due to the rising concerns about the environment and the scarcity of fossil fuels, the electrification of the transportation sector has attracted wide attention. However, the rapid growth of these vehicles has been hampered by the lack of charging facilities. Various policies and guidelines have been established to encourage the development of EVs on a global scale Nallusamy et al. (2016). By 2030, the world’s population is expected to reach approximately 130 million. Due to the rapid growth of EVs, the existing power grid infrastructure is expected to face significant challenges in handling the penetration of these vehicles’ loads IEA (2022). The grid’s components might break down, and the transformer can become overloaded as a result of the successive increase in its dependence. Although renewable energy sources are considered an ideal solution for addressing the issue of fossil fuel shortages, their intermittent nature could make their operation challenging to manage. Dharavat et al. (2022), PEVs (plug-in electrical vehicles) also have challenges such as restricted range, a shortage of charging stations, less power, high cost (due to expensive batteries), expensive insurance, high maintenance, and pollution (due to battery toxicity) as well as the fact that not all energy is generated from renewable sources. PEVs have difficulties in India owing to poor market penetration, high manufacturing costs owing to the drop in the value of the rupee, an inadequate infrastructure, and the need to import lithium batteries from other nations.
High-capacity energy storage devices are required to support the expansion of the EV network. These systems can be integrated into the grid and provide additional storage capacity. The charging infrastructure connects the transport network and distribution system. It’s crucial to study charging loads and predict their needs while designing electric vehicle charging stations (EVCS). Installing EVCS will raise distribution system demand, which will affect their performance. Integrating local renewable power into the grid may reduce the adverse effects of charging load and reduce greenhouse gas emissions. The increased demand, however, may not be able to be met at certain times of the day due to the stochiastic nature of renewable energy sources like solar, wind, etc. Cleary and Palmer, (2020). The stability of the power grid will be more affected by the intermittent nature of RES and the lack of coordination among EVs. On the other side, the energy storage capacity of EVs may be used to stabilize the power grid, particularly when collaborating with RES to mitigate power transitions, therefore lowering both energy costs and carbon dioxide emissions. Increasing the percentage of RES integrated into the grid is possible in a variety of ways, but the best one, in terms of both cost and complexity, is to store electricity generated by electric vehicles. By mitigating the negative effects of unplanned EV charging on the grid and enhancing the unpredictability of RES, benefits Reddy and Vijayakumar, (2019). To assist distribution operators in their decision-making processes in the event of system violations, it is crucial to study the consequences of quick charging for EVs. The use of charging stations that are integrated into the smart grid can help to minimize air pollution and provide better energy management.
Smart grid operators must determine an ideal power pricing by examining EV charging behaviour with the enormous quantity of electric energy required by EVs. Regular and irregular EV users may be distinguished from one another from the standpoint of charging behaviour Chung et al. (2018). There is some consistency in how common users charge. The quantity of each charge is constant, and it occurs at a specific time each day. Unusual users do not charge in a predictive manner. V2G technology can also help to reduce the impact of additional load demand on the grid. This mode of operation allows the grid to receive and utilize the collected energy from the vehicles. Due to their environmental benefits, energy storage is becoming an integral part of the electric vehicle industry. Its continuous use enables the grid to monitor and control the distributed generation network. Although the grid operation can be beneficial for consumers, there’s a chance that EV batteries could get damaged due to the discharge conditions. To encourage the use of EVs, India is offering subsidies to its buyers. But as the number of EVs on the road increases, so does the need for lithium-ion batteries (LIBs), and therefore measures must be made to guarantee safe battery disposal. This effort contributes to India’s larger goal of making the country’s future pollution-free EVreporter (2022) and the same given in Table 1. Integrating EVs and the grid can be considered an efficient energy management method. Doing so involves establishing a smart contact connection between the grids and the vehicles. This can help in minimizing the effects of varying load conditions and improve the efficiency of the entire system.
TABLE 1. Penetration of four wheeler EVs in 2022 EVreporter, (2022).
The conventional power infrastructure cannot provide the rising demand for energy needed to enable industrial innovation and the rise in human living standards due to the ongoing changes in the climate across the world. By integrating smart communication, artificial intelligence, sensor and automated control technologies with electricity infrastructures, the smart grid is developing as the next-generation electrical grid to solve these issues Sun et al. (2020). In contrast to traditional optimization approaches, using Machine Learning to coordinate EVs is more superficial and takes less time and computational resources Shibl et al., (2020). The machine learning algorithms can forecast the amount of electricity EVCS will consume. Machine learning has been used because of its capacity to use previous information to learn and recognize patterns to make future predictions with minimum input from the user. The use of these technologies for improving future forecasts has yet to be realized and shown, despite the fact that the majority of studies and implementations of ML/CI techniques focus on energy use and associated costs, Shibl et al., (2020). There is a need to emphasize and look forward to the future mobility transition while deploying charging infrastructure. They also believe that developing intelligent charging technologies and measures to reduce range anxiety are crucial components of the EV market strategy, as they would promote the widespread use of EVs.
Non-etheless, EV drivers face range anxiety since charging takes longer than filling a non-EV with petrol at a petrol station. This delays the widespread adoption of EVs and directly results from battery chemistry, charger limitations, and power consumption requirements Wu, (2021). As a result, the BSS model of battery exchange has been presented. Battery swapping has been introduced at commercial and private stations by BSS service providers, prompting academics to study the BSS methodology and propose different operating systems and optimization approaches.
There was a period of phenomenal development for the EV industry throughout the last decade. After the previous COP26 summit, India pledged to bring its carbon emissions to zero by 2070. India plans to have a 30% share of the private car industry, a 70% share of the commercial vehicle market, and an 80% share of the two- and three-wheeler market in 2030, originating through sales of EVs. The rising expense of fossil fuels and increased environmental concerns, EVs have attracted the public’s attention. EV owners may swiftly charge their vehicles at home with the aid of charging stations. However, these stations can also overload the grid due to their presence (Bossche, 2010). An intelligent charging system should be implemented to avoid this issue. It should use a strategy that considers the varying factors that affect the charging process and provide a reliable and cost-efficient method of operation.
N. Uddin and Islam, (2019) provide a fuzzy logic-based intelligent power management controller that blends wind, solar, and grid power with backup batteries. To assess the suggested method, the smart energy management system uses optimal fuzzy logic and is thus more economical than other conventional methods.
Zahedmanesh et al., (2019), Proposed a model of VPP, which includes parking spots for EVs, connected to the grid through photovoltaic panels. A CEM-based strategy is explored in order to assign a systematic and cost-effective energy management for the VPP and to control the electric constraints for the power systems. For both energy management and the delivery of auxiliary services, the suggested CEM technique makes use of hierarchies. To meet the needs of the commercial entities in the neighborhood, meet the charging needs of the parking lot, and optimize the VPP controller’s profit, the CEM’s structure employs a daily scheduling strategy. The second-tier aids in satisfying the technical needs of the power system via the VPP’s provision of reactive power compensation (RPC).
Das et al. (2020) explores a multi-objective optimization problem to establish the simultaneous placement and size of DGs and FCSs, with limitations on the number of EVs in each zone and the maximum number of FCSs achievable based on the road and electrical network in the proposed system. In order to reduce the cost of developing FCS, optimise power loss, and enhance the voltage profile of the electrical distribution system, the challenge is framed as a MINLP.
Gampa et al. (2020), For distribution systems, this work proposes a two-stage GOA based Fuzzy multi-objective approach to the size and location of DGs, Shunt Capacitors (SCs), and EVCS. By addressing the voltage and current limits of the distribution system and limiting the actual power losses to a specific value, the fuzzy-based GOA algorithm determines the optimal size and location of EV charging stations.
Zeb et al. (2020) explore the inclusion of all three categories electric vehicle chargers, which are optimized to achieve the best results by Controlling the electric vehicle load efficiently while reducing installation costs, losses, and distribution transformer loading. Probability has a role in the EV load due to the unpredictable nature of vehicle users. The constrained non-linear stochastic issue is solved using PSO. The model is simulated using MATLAB and OpenDSS.
Dogan, (2021), This paper proposes a weighted sum of Evolutionary-based multi-objective optimization technique for substantially decreasing power loss, improving voltage level, and enhancing the DG, EVCS, and ESS integration capacities. Also presented a hSLC-PS optimization technique to improve the optimization performance.
The overall load demand, the generating profiles of solar and wind energy systems’ uncertainties and the DSTATCOM operation capabilities of photovoltaic and wind generating units are taken into account in this research. The potential EV needs are also considered, the time of arrival and departure, the battery’s original and current SoC configurations, the charging methods used, and whether the battery was charged in a regulated or unregulated manner. To handle this complicated planning model, an efficient and accurate bi-level Multiobjective Ant Lion Optimizer (MOALO) solution for the planning model. The MOALO solver has a bi-level structure, with upper-level optimization aimed at maximizing the efficiency of renewable energy sources and lower-level optimization aimed at maximizing the efficiency of personal electric vehicles. The bi-level MOALO solution takes into account sub objectives such as reducing energy losses and maximizing energy from the main grid Ali et al. (2022).
A micro-grid with a fleet of EVs and a confined vehicle-to-grid application is the focus of this research. Chtioui and Boukettaya (2020). The discharging mode is only used when there is a high-demand situation with a long response time. The micro-construction grid’s blocks are described and modelled, as well as a simulation of their operation. The research explores the charging and discharging scenarios and the management strategies employed to govern the power in this simulation.
Javed et al. (2020), present the configuration of V2G and the fundamental concerns related to V2G, which are profoundly analyzed in terms of Battery deterioration, Bi-directional chargers, and charging stations using centralized control and management of the battery system. In addition, the economic cost and income from both the EV owner and the power grid are explored, as well as the problems, benefits, and technologies associated with V2G. The influence of V2G on power systems is investigated in this study using typical test networks.
Based on the value-based pricing strategy, this research proposes a unique methodology of placing DGs and V2G parking lots in the most efficient locations at the same time Mousavi-Khademi et al. (2020). The paper’s essential contribution is the inclusion of pricing the DGs and V2Gs using a value-based approach for the best position, as well as the suggested optimal search algorithm. The network’s technical issues, include improving the voltage profile and lowering the losses, are addressed in this way by identifying and establishing the best capacity of distributed production resources and electric car parking spaces using value-based pricing to attract network investment.
The article’s objective is to reduce power loss in the distribution system when DG is present along with more strategic planning of G2V and V2G operating modes of EVs. Velamuri et al. (2022). To identify the optimal size of the DGs to be placed in the system, the suggested method includes a smart charging mechanism, a voltage stability index, and an EGOA. The electric vehicles are simulated by taking into account the most important aspects, such as the EV SoC, journey circumstances, EV battery capacity, and charging/discharging levels.
The work presented here is the size and positioning of DGs in the distribution system, with battery storage installed after the DGs to sustain the grid Chellappan et al. (2022). The genetic algorithm is utilized by radial node distribution systems, IEEE-33, IEEE-69, and IEEE-118, to install the battery energy storage system. At the same time, the heuristic technique PSO is employed for the sizing and positioning of DGs.
Ravi and Aziz (2022), provides a summary of the current V2G technology scenario and some potential ancillary services, such as frequency regulation, voltage regulation, peak shaving, load levelling, spinning reserve, congestion mitigation, renewable energy storage, reduction of intermittentness, and curtailment, that could be offered with an infrastructure that supports vehicle grid integration.
The global transportation industry is transitioning from cars powered by traditional fossil fuels to vehicles with zero or ultra-low exhaust emissions. We need a well-developed network of charging stations (CSs), data analytics, intelligent decentralized power generation units, and supportive policy initiatives to facilitate this shift. It is crucial to design and locate a charging station to encourage the widespread usage of electric cars and maximize the benefits of cost-efficient, clean electricity from the grid and renewable energy sources. The transportation industry is undergoing these three transformations in terms of autonomous driving, shared mobility, and electrification Ghosh. (2020). As a result, it is vital to consider the interactions and synergies that may arise between these three impending revolutions while designing the infrastructure for EV charging. A new, considerable electrical demand is being added to the power grid as EV usage rises, forcing infrastructure improvements. Only the distribution grid transfers electrical energy, restricting the amount of energy that may travel via the transmission lines Zhang et al., 2011). The electrical grid must be extensively rebuilt to accommodate the EV’s charging requirements. Figure 1 represents approaches to the conceptualization of the problem regarding the optimal location of EVCS and Figure 2 represents EV Charging Infrastructure.
FIGURE 1. Approaches to the conceptualization of the problem regarding the optimal location of EVCS.
Optimal allocation and size of charging stations is a fundamental planning challenge for the electric vehicle (EV) industry, with considerations including cost, distribution network operational characteristics, and the needs of EV drivers. For EVs to be extensively employed, a well-thought-out charging infrastructure must be in operation. Figure 1 depicts the many steps involved in designing a charging infrastructure. The forecasting of pricing service demand at various times and places throughout the day is to be measured precisely. Its utilization must be known to determine how often a charger has been used and how many charging cycles it has been supported. Scheduling charging entails scheduling various charging operations with the grid’s capacity and the projected charging demand Brenna et al. (2020). Figure 3 represents strategic charging network design: an overview and Figure 4 represents EV charging management structure.
Location and sizing considerations are vital in optimizing charging infrastructure facilities. Large charging stations are able to handle a greater number of EVs since they have the capacity to house more chargers, but at increased electricity consumption and building expense Iqbal et al. (2021). EV battery capacity and power rates determine future charging infrastructure requirements. The profitability and performance of EVCS are directly correlated to the level of planning and management that goes into the operations of the charging stations. The first step in planning an EVCS is to engage in creative thinking and decision-making at different levels. The charging intensity, the expected number of charges, the necessary storage area, the charging infrastructure, and the planned energy storage system are all the factors to be considered. Scheduling charging to support grid functioning, reducing EV charging wait times, and providing a seamless charging experience are all part of a well-managed charging station network. Bhattacharjee et al., (2020). The infrastructure for EVCS, including the distribution system, may be planned, designed, simulated, and optimized using software like MATLAB, HOMER, PVsyst, EVLibSim, etc. Together, EVLib and EVLibSim offer a robust infrastructure for managing EV charging operations at the station level via simulation Rigas et al. (2018).
The pace of EV adoption is crucial to the implementation and profitability of fast and ultra-fast charging stations. Considering that many would-be plug-in electric vehicle (PEV) buyers want public charging times that are on par with traditional refuelling, researchers and policymakers have concentrated on developing rapid charging technologies that can handle larger power loads Sadeghi-Barzani et al. (2014). The public’s adoption of EVs may be significantly aided by the widespread availability of fast and ultra-fast charging stations that reduce the charging time to an acceptable level. However, there will be consequences for grid stability, robustness, and efficiency if these technologies are widely used Amiri et al., (2018). Another novel solution to the issue of EVs taking extended periods to charge is the battery swapping station (BSS), which allows drivers to change their discharged battery for a fully charged one. In Iannuzzi and Franzese (2021), a plan is presented for developing ultra-fast EV charging infrastructure. It has a super-fast charging station for EVs powered by a DC microgrid and uses batteries to store energy. In addition to effectively supporting 800 V DC charging, the system allows rapid EV charging in under 10 min. The developed control system uses load-level managing electricity use in order to ease the load on the AC power grid during high demand. According to the system analysis, the ESS’s charging power surpasses the grid in the case of a high arrival rate, making it hard to lower the system loss rate successfully. For this reason, to increase profit, the ESS configuration must be adjusted according to the charging load. Optimization often entails formalizing the issue in mathematics and then solving it using an appropriate method. The EVFCS-RP problem is an illustration of an evolutionary computation challenge, since it involves maximizing both profitability and the prospect of satisfying EV consumers. In this research, a multiobjective EVFCS-RP mathematical model is developed to facilitate the attainment of a satisfactory solution Shi and Lee (2015).
The widespread adoption of EVs is often viewed as a key component in developing intelligent transportation applications. However, the widespread installation of EVCS presents a number of issues with the electrical grid and other forms of public infrastructure. The straightforward solution of installing additional charging stations to boost overall charging capacity does not work to mitigate the issue of protracted charging times because of the strain that this would place on existing power grids and the constraints imposed by the availability of physical space Shahriar et al. (2020).
With more recent times, there has been a rising demand in using data-driven methods for simulating electric vehicle charging. As a consequence of this, methods are able to recognize patterns in customer charging behavior in the order to gain insights and the potential to do predictive analytics. As a result, academics have emphasized creating modeling and optimization-based intelligent scheduling methods to reduce the need for public charging. Incorporating network for data exchange, an optimization unit to cut down on wait times at charging stations, and a prediction unit to help the optimization unit will make the most informed decisions possible about charging station placement choice feasible, are the three major impediments to creating an efficient charging infrastructure Sheik Mohammed et al. (2022).
It is of the utmost importance to manage and effectively plan to charge the electric car at the nearest available charging station to prevent situations in which there is a high demand for charging at one charging station, while there is less demand at other neighbouring stations. This will assist in the strategic management of the queues of vehicles at EVCS. The delays at the charging stations might be more efficiently managed and monitored with an efficient communication network. A negotiating strategy based on agents was developed to schedule charging at an available charging station and allocate EVs to those stations. This system might be used to manage the wait time at the charging station. To facilitate energy trading between individuals and the supply of ancillary services to the grid, Seitaridis et al. (2020) presented an algorithm for bidirectional smart charging of EVs linked to the grid using bidirectional converters. A combination of soft restrictions and optimization variables allows the EV user’s preferences to be accounted for in the scheduling model. Mathematical analyses show that taking into account user preferences improved the overall income earned by the EV scheduling scheme. In addition, the established user-centric model increased the number of peer-to-peer energy transactions between the EVs by nearly 90% and the number of ancillary services provided to the grid by about 11% Seitaridis et al. (2020).
An optimal EV charging communication protocol is required to provide the following functions when technological innovations like “smart grid” and “V2G” are introduced Dhianeshwar et al., (2017).
• Identifying the vehicle and simplifying the procedure of paying the customer.
• Cost-effective optimization of the charging process is achieved by determining the optimal charging slot and settling on the most economical charging rates.
• Management of charger power rating to grid demand, resulting from loading optimization.
• Vehicle-to-grid (V2G) technology assists the grid during peak demand.
• The ability to charge users and compensate them for their time and energy spent using V2G services.
Several parties, including utilities and vehicle manufacturers, are involved in developing this communication protocol. A joint working group made up of representatives from IEC TC69, ISO TC22 SC3, and TC22 SC21 is addressing the standardization of a communication protocol.
The operational conditions and equipment capabilities of distribution networks are established based on the predicted loads of EV charging. Therefore, it is crucial to strengthen the distribution system’s consistence and efficiency by improving the accuracy of load forecasts. Traditional, non-artificial intelligence approaches, machine learning methods, and artificial intelligence methods are the broad categories that may categorize the many available techniques for load forecasting. The temporal features of the load needs are often the foundation for non-AI approaches. After then, long-term load demand forecasting is usually achieved via statistical approaches Yang (2015). Also, the non-linear properties, fluctuating power demands and their time-dependent, unpredictable nature are becoming increasingly apparent as EVs and renewable power production equipment are integrated on a massive scale on the load side. Due to the lack of consistency and precision in conventional forecasting approaches, it is challenging to develop an appropriate mathematical model that can represent the correlations between predicting outcomes and influencing factors.
Artificial intelligence algorithms that are data-driven rather than model-based have shown promising growth in electricity forecasting in recent years. A wide variety of approaches have been used by researchers in power forecasting, including random forests Huang et al., (2016); Lahouar and Ben Hadj Slama (2015), BP neural networks, support vector machines, extended short-term memory networks and convolutional neural networks Choi et al., (2020). FTS and CNN are combined to provide a short-term load forecasting approach. On the other hand, conventional CNN requires a lot of time to train and fails to adequately capture the temporal information included in the time series. An approach to load forecasting using a genetic algorithm and an extended short-term memory network is presented in Santra et al. (2019). The load curve is the final product after carefully considering the input data as load-demanding elements.
In contrast, the LSTM network augments each hidden-layer neural unit with sophisticated gate components. Therefore, LSTM training efficiency may be poor. The SVM technique suggested in Barman and Nalin Behari, (2020) for load demand forecasting uses grey wolf optimization. SVM approach, on the other hand, when the amount of training data is too large, its classification performance may suffer from a lack of precision due to the continuous nature of the data samples.
To prevent abrupt spikes in peak load demand, it is essential that charging activity at charging stations be managed. V2G technology and scheduled EVCS were the primary emphasis of Dang et al., (2019), which also used reinforcement learning to assess the operational benefit of EVs, also models the scheduling of EV charging and discharging as a constrained Markov Decision Process (CMDP). The constraint is to reduce the cost of charging the EV while still ensuring it can be completely charged, hence a limited charging/discharging scheduling technique is being sought. Model-free safe deep reinforcement learning (SDRL) is offered as a means to resolve the CMDP. With the suggested method, familiarity with randomization is not necessary. It uses a DNN for the constrained optimum charging and discharging schedules directly. The authors of Li et al., (2020) present a method based on reinforcement learning to schedule constrained EV charging times. The authors of Cong Zhang et al. (2021) posed the issue of charging schedule creation as an NP-hard one and then used reinforcement learning to solve it. It was recommended that an intelligent pricing strategy be used at charging stations, and an ANN was presented as a remedy for the issue with charging patterns. Using the Q-learning technique, the authors of Dang et al., (2020) determined that charging electric vehicles at a fast-charging station connected to an intelligent grid was optimum. The attractive feature is the intelligent charging scheduling system, which considers in the state of charge, the distance travelled, the proximity to charging stations, the number of scheduled events, and the average speed. The scenario simulator that creates the labelled datasets needed to train the Machine Learning/Reinforcement Learning algorithms is a unique part of the proposed approach as well, given the scarcity of such datasets Viziteu et al. (2022).
The fields of machine learning and artificial intelligence include the subfield of supervised learning, commonly known as supervised machine learning. It is characterised by training algorithms that properly categorise data or predict outcomes using labelled datasets. Cross-validation is the iterative process of adjusting a model’s weights when new data is added until the model is well suited to the data. Predictions based on labelled data may be made more accurately and save time with the help of supervised learning models.
Unsupervised learning makes use of data that has not been labelled. Patterns useful for handling cluster or association difficulties are uncovered within the information. This is especially helpful when domain experts lack knowledge of the similarities present in a dataset. In popular use, methods like the hierarchical, k-means, and Gaussian mixture models cluster data. Machine learning includes the discipline of reinforcement learning. It involves appropriately increasing rewards in a certain circumstance. To determine the optimal course of action to pursue in each circumstance, it is used by different software and robots. The process of reinforcement learning relies heavily on sequential decision-making. To put it another way, the state of one input determines the value of the next input, and the value of the next input determines the value of the preceding output. Figure 5 represents the types of ML Rajbanshi Sabita, (2021).
FIGURE 5. Types of machine learning Rajbanshi Sabita (2021).
The exponential growth of EV production on the road has shifted the focus of urban planners, utilities, and business owners towards the BSS and BCS initiation. Models of BCS and BSS are included in cutting-edge research on EV charging to provide additional energy sources for EVs. During the BCS mode, EV drivers connect their vehicles to the charging connector and let the batteries recharge over a long time. While in BSS mode, electric vehicle drivers will discharge the spent, then swap it out with one that has been fully charged, which will take less time Wu, (2022). Figure 6 represents battery swaping system.
Compared to the BCS model, the only direction in which the duration of battery charge is transitioning from the EV to the BSS. In other circumstances, even after switching the batteries, the BSS must spend a significant amount of time for recharging them. The BSS operator may decide on the most effective battery and EV charging schedule based on the battery condition and swapping/charging demand, that supports the station to increase operational profit and reduce power expenses. As a result, the BSS’s Quality of Service and service capacity may be enhanced. An ideal charging schedule can also assist the BSS with gratifying more EV swapping and charging demands Wang and Pedram (2019). The following are some of the reasons why charging management is so important while operating in BSS mode:
• If an ideal charging schedule is achieved, then modifying the charging rate at the recharging centre may be used to control the BSS’s service availability, which corresponds to the batch of completely recharged batteries.
• The BSS operator is responsible for managing the charging process while considering many factors, such as the needs of EV drivers to swap out their batteries, the limits of the power grid, and the cost to operate (which includes the cost to buy batteries and electricity).
• As the lithium-ion battery’s charging power exhibits non-linear features, determining the precise time required to charge one is challenging entirely. To simulate the constant current constant-voltage features Wu et al., (2020), several researchers have developed non-linear mathematical models that can approximate the quantity of power used throughout each period and the time necessary to charge various chargers fully.
EV distribution, road transportation, traffic patterns, and business and residential zones should all be considered when examining the need for charging and switching utilities from an urban planning standpoint Pardo-Bosch et al. (2021). Then, by providing charging facilities, a new charging station may be built, or an existing one can be improved. Additionally, new BSSs might be designed in a given area to serve commercial EVs, with significant energy demands and the need for quick charging. The charging and switching stations function as high-energy units that impact voltage stability from the standpoint of the power system. Therefore, the power grid’s limitations should be considered when choosing BSS or BCS centres. In addition, the BSS/BCS imports supply of electricity from the mains, utilizes it to charge EVs and batteries and then starts selling it to drivers for a profit. As a result, the station manager and the power grid may agree on an electrical strategy. With the aid of V2G and B2G technologies, the BCS/BSS may potentially be able to sell power to the grid., which allow them to benefit from price fluctuations and aid the system in maintaining voltage stability. Providing EV owners with access to battery switching and charging stations is an opportunity for BSS/BCS providers to generate revenue. The operators’ primary objective during the planning phase is to choose an area with high swapping/charging demand to optimize their operational profit. On the contrary, intelligent optimization decision models may be used to reduce the cost of their operations, which include things like the BSS’s initial stock of batteries, charging infrastructure, and station development. Increasing assessing demand and reducing planning expenses is preferable to optimize the station’s operational profit.
If an EV driver wants to switch, the control centre needs to know how far away they are from the BSS and how long it will take them to get there. Due to traffic and travel patterns, it is important to include the unpredictability of appointment information as input to the decision-making model Bonsall, (2004). As a result of needing to travel to their destination BSSs without running out of power, EVs’ remaining SoCs are associated with the distance to those BSSs. Because of this, as a constraint in the dispatching and routing problem, the remaining SoC in the EV and the distance to each BSS must be taken into account. In contrast to the single BSS mode, the multiple BSS mode requires the control centre to improve the quality of the choice by synchronizing the needs of EV drivers with the battery status of the dispersed BSSs. To validate their choice, EV drivers will get a response from the control centre. They could negotiate new arrival times or switch to a different BSS if they decided not to accept the assignment. On the BSS side, the control centre should check each BSS’s power reserves and make charging adjustments in real time as necessary. Coordination amongst decision makers is, thus, essential in an intelligent BSS system for achieving the best possible outcome.
Decentralized decision-making models that support swapping and charging orders might be used to describe the combined BSS and BCS modes. Due to the integrated nature of these models, they can perform both of these functions. An EV operator may choose battery exchange or recharge when making a service request Sun et al., (2018). Two independent decision models have been established to manage to swap and charge requests from the viewpoint of the control centre. Based on the volatile demands placed on the BSS and BCS, these models determine whether to accept or not to provide access for the request.
A centralised decision model might be used to describe the combined BSS and BCS station., in contrast to the decentralized approach, where the BSS and BCS are complimentary while taking into account the order needs from EV drivers and the state of each station. This centralized approach has two operational techniques.
• The control centre makes the assignment without charging or swapping the desired signal from an EV driver. The control centre thus mainly concentrates on the changing battery count at the BSS and BCS, allowing for optimal decision-making given the system’s overall state of health.
• If the BSS is overloaded (because of a lack of completely charged batteries), some batteries might be brought to the BSS after being recharged in EVs at the BCS. Once the parking duration of the EVs at the BCS and the switching load at the BSS are known, the interaction may be maximized.
The BSS and BCS models are combined, and the benefits of doing so are addressed. First, the control centre handles the switching and charging requests simultaneously, improving the level of service for EV users. Secondly, by allocating the swapping and charging operations, the choice is optimized, and the overall optimization outcome may be reached. Third, depending on demand and station state, In both the BSS and BCS, the control schedule for the recharging process has been optimised. Fourth, the control centre may ask EV drivers to designate their preferred method, such as swap or charge, since it is believed that they would use a system of distributed decision-making for handling incoming requests Cui et al., (2022). Finally, by synchronising the BCS with the BSS infrastructure, the service capacity may be increased, resulting in enhanced QoS on a Global Scale in BSS and BCS modes due to the adoption of centralized methods.
The research can be further progressed on the following cases.
• A comparison in the effectiveness of planning issues for charging infrastructure using machine learning approaches, heuristics, and metaheuristics techniques.
• The implementation of various pricing levels for energy based on the intermittent EV load demand, where the price of electricity in EVCS varies and will be calculated based on the overall power use in the region as well as the demand on the power system.
• It is possible to look at leveraging automation and IoT architecture to operate EVCS. As a consequence, EV charging and discharging may be automated based on energy use.
• For EVs to be more sustainable, recycling rates must increase so that valuable metals used in battery production may be reused. The energy density and charging velocity of solid-state batteries are enhanced by the use of ceramic or other solid electrolytes.
This article provides a comprehensive review of studies examining the use of machine learning in various contexts and forecasting of EV charging behaviour. Standard ML algorithms for predicting EV charging behaviour were defined, including supervised and unsupervised methods. Using EV and load volatility, a comparison of different EV modes of operation is accomplished. One might deduce that better EV and DG scheduling contributes to enhanced DS performance. It is also clear that if EV owners can plan their vehicles according to the system consumption pattern, they may generate income using V2G mode.
The article also summarizes various EVs, storage facilities, charging EVs using DGs integrated with EVCS, and a variety of other socio-technical difficulties related to EVs. The adoption rate, as well as the current situation of EVs all around the world, have been emphasized. The increased weight of EVs owing to the inclusion of battery storage mitigates the benefits of EVs’ reduced particulate matter production, but only to a limited extent. Electric cars have fundamental problems, such as the absence of high-performance rapid charging infrastructure and the inability to compete in terms of mileage with conventional fuel-based vehicles due to inefficiencies in energy storage and battery management. Government policies that provide attractive incentives and advantages are crucial to boost EV adoption. Willingness to spend and socioeconomic status are two factors influencing consumers’ decisions to purchase EVs. The production of EV sales should focus on the variety and design of a model that will appeal to a wide range of buyers. Monadi et al. (2022).
Conceptualization, NG, ND, SS, SV and MVVPK; Methodology, NG, ND, SS, SV and MVVPK; Validation, NG, ND, SS, SV and MVVPK; Formal analysis, NG, ND, SS, SV and MVVPK; Investigation, NG, ND, SS, and SV; Resources, HK and KA; Data curation, NG, ND, SS, SV and MVVPK; Writing—original draft preparation, NG, ND, SS; Writing—review and editing, NG, ND, SS; Visualization, NG, ND, SS, SV, MVVPK, HK, and KA; Supervision, SS, and SV; Project administration, SS, MVVPK, HK and KA; Funding acquisition, HK and KA. All authors have read and agreed to the published version of the manuscript.
The authors declare that the research was conducted in the absence of any commercial or financial relationships that could be construed as a potential conflict of interest.
All claims expressed in this article are solely those of the authors and do not necessarily represent those of their affiliated organizations, or those of the publisher, the editors and the reviewers. Any product that may be evaluated in this article, or claim that may be made by its manufacturer, is not guaranteed or endorsed by the publisher.
ANN, Artificial neural network; B2G, Battery to Grid; BCS, Battery Charging Station; BSS, Battery Swapping Station; CEM, Consecutive Energy Management; CI, Computational Intelligence; CNN, Convolutional Neural Networks; DGs, Distributed Generators; EGOA, Extended Grasshopper Optimization Algorithm; ESS, Energy Storage System; EV, Electric Vehicle; EVCS, Electric Vehicle Charging Station; FTS, Fuzzy Time Series; G2V, Grid to Vehicle; GOA, Grasshopper Optimization Algorithm; HSLC-PS, Hybrid Soccer League Competition–Pattern Search; LSTM, Long short-term memory; MINLP, Mixed Integer Non-Linear Problem; ML, Machine Learning; PSO, Particle Swarm Optimization; QoS, Quality of Service; SVM, Support Vector Machine; V2G, Vehicle to Grid; VPP, Virtual Power Plant.
Ali, A., Mahmoud, K., and Lehtonen, M. (2022). Optimal planning of inverter-based renewable energy sources towards autonomous microgrids accommodating electric vehicle charging stations. IET Generation, Transm. Distribution 16 (2), 219–232. doi:10.1049/gtd2.12268
Amiri, S., Jadid, S., and Saboori, H. (2018). Multi-objective optimum charging management of electric vehicles through battery swapping stations. Energy 165, 549–562. doi:10.1016/j.energy.2018.09.167
Barman, M., and Nalin Behari, D. V. (2020). A similarity based hybrid GWO-SVM method of power system load forecasting for regional special event days in anomalous load situations in Assam, India. Sustain. Cities Soc. 61, 102311. doi:10.1016/j.scs.2020.102311
Bhattacharjee, A., Mohanty, R. K., and Ghosh, A. (2020). Design of an optimized thermal management system for Li-ion batteries under different discharging conditions. Energies 13 (21), 5695. doi:10.3390/en13215695
Bonsall, P. (2004). “Traveller behavior: Decision-making in an unpredictable world,” in Intelligent transportation systems (Oxfordshire UK: Taylor & Francis Group), 8, 45–60.1
Bossche, P. (2010). Electric vehicle charging infrastructure. Electr. Hybrid Veh. Power Sources, Models, Sustain. Infrastructure Mark., 517–543. doi:10.1016/B978-0-444-53565-8.00020-8
Brenna, M., Foiadelli, F., Leone, C., and Longo, M. (2020). Electric vehicles charging technology review and optimal size estimation. J. Electr. Eng. Technol. 15 (6), 2539–2552. doi:10.1007/s42835-020-00547-x
Chellappan, K., Krishnan, N., Sharma, G., and Senjyu, T. (2022). Real power loss minimization considering multiple DGs and battery in distribution system. Electr. Power Components Syst. 49 (6-7), 563–572. doi:10.1080/15325008.2021.2011488
Choi, E., Cho, S., and Kim, K. (2020). Power demand forecasting using long short-term memory (LSTM) deep-learning model for monitoring energy sustainability. Sustain. Switz. 12 (3), 1109. doi:10.3390/su12031109
Chtioui, H., and Boukettaya, G. (2020). “Vehicle-to-Grid management strategy for smart grid power regulation,” in Proceedings of the 6th IEEE International Energy Conference, Chengdu, China, November 2020, 988–993. doi:10.1109/ENERGYCon48941.2020.92365303
Chung, Y., Khaki, B., Chu, C., and Gadh, R. (2018). “Electric vehicle user behavior prediction using hybrid kernel density estimator,” in Proceedings of the 2018 International Conference on Probabilistic Methods Applied to Power Systems, Boise, ID, USA, July 2018. doi:10.1109/PMAPS.2018.8440360
Cleary, K., and Palmer, K. (2020). Renewables 101: Integrating renewable energy resources into the grid. https://www.rff.org/publications/explainers/renewables-101-integrating-renewables/.
Cui, D., Wang, Z., Liu, P., Wang, S., Dorrell, D. G., Li, X., et al. (2022). Operation optimization approaches of electric vehicle battery swapping and charging station: A literature review. Energy 263, 126095. doi:10.1016/j.energy.2022.126095
Dang, Q., Wu, D., and Benoit, B. (2019). “A Q-learning based charging scheduling scheme for electric vehicles,” in Proceedings of the 2019 IEEE Transportation Electrification Conference and Expo, Seogwipo-si,South Korea, May 2019. doi:10.1109/ITEC.2019.8790603
Dang, Q., Wu, D., and Boulet, B. (2020). “EV charging management with ANN-based electricity price forecasting,” in Proceedings of the 2020 IEEE Transportation Electrification Conference & Expo (ITEC), Chicago, IL, USA, June 2020 (IEEE), 626–630.
Das, H. S., Rahman, M. M., Li, S., and Tan, C. W. (2020). Electric vehicles standards, charging infrastructure, and impact on grid integration: A technological review. Renew. Sustain. Energy Rev. 120, 109618. doi:10.1016/j.rser.2019.109618
Dharavat, N., Kumar Sudabattula, S., and Suresh, V. (2022). “Review on the integration of distributed generations (solar, wind) and electric vehicles connected to the distribution system to minimize power loss and voltage profile enhancement,” in Proceedings of the Innovations and Research in Marine Electrical and Electronics Engineering: Icirmeee, Chennai, India, October 2022, 020001. doi:10.1063/5.01009572455
Dhianeshwar, A., Kaur, P., and Nagarajan, S. (2017). “EV: Communication infrastructure management system,” in Proceedings of the 2017 1st International Conference on Sustainable Green Buildings and Communities, SGBC, Chennai, India, December 2017. doi:10.1109/SGBC.2016.7936090
Dogan, A. (2021). Optimum sitting and sizing of WTs, PVs, ESSs and EVCSs using hybrid soccer League competition-pattern search algorithm. Eng. Sci. Technol. Int. J. 24 (3), 795–805. doi:10.1016/j.jestch.2020.12.007
Evreporter, (2022). EVreporter magazine. Bengaluru, India: EVreporter.Com. https://evreporter.com/wp-content/uploads/2022/11/EVreporter-NOV-2022-magazine.pdf.
Gampa, S. R., Jasthi, K., Goli, P., Das, D., and Bansal, R. C. (2020). Grasshopper optimization algorithm based two stage fuzzy multiobjective approach for optimum sizing and placement of distributed generations, Shunt Capacitors and electric vehicle charging stations. J. Energy Storage 27, 101117. doi:10.1016/j.est.2019.101117
Ghosh, A. (2020). Possibilities and challenges for the inclusion of the electric vehicle (EV) to reduce the carbon footprint in the transport sector: A review. Energies 13 (10), 2602. doi:10.3390/en13102602
Huang, N., Lu, G., and Xu, D. (2016). A permutation importance-based feature selection method for short-term electricity load forecasting using random forest. Energies 9 (10), 767. doi:10.3390/en9100767
Iannuzzi, D., and Franzese, P. (2021). Ultrafast charging station for electrical vehicles: Dynamic modelling, design and control strategy. Math. Comput. Simul. 184, 225–243. doi:10.1016/j.matcom.2020.04.022
IEA (2022). Global EV outlook 2022 - securing supplies for an electric future. Glob. EV Outlook 2022, 221. doi:10.1787/c83f815c-en
Iqbal, M., Kütt, L., Lehtonen, M., Millar, R., Püvi, V., Rassõlkin, A., et al. (2021). Travel activity based stochastic modelling of load and charging state of electric vehicles. Sustain. Switz. 13 (3), 1550–1614. doi:10.3390/su13031550
Javed, M., Deb, S., Alam, M., Rafat, Y., and Hameed, S. (2020). “Impact of vehicle to grid on power system,” in Proceedings of the 2020 5th IEEE International Conference on Recent Advances and Innovations in Engineering, ICRAIE, Jaipur, India, December 2020. doi:10.1109/ICRAIE51050.2020.9358388
Lahouar, A., and Ben Hadj Slama, J. (2015). Day-ahead load forecast using random forest and expert input selection. Energy Convers. Manag. 103, 1040–1051. doi:10.1016/j.enconman.2015.07.041
Li, H., Wan, Z., and He, H. (2020). Constrained EV charging scheduling based on safe deep reinforcement learning. IEEE Trans. Smart Grid 11 (3), 2427–2439. doi:10.1109/TSG.2019.2955437
Monadi, M., Farzin, H., Reza Salehizadeh, M., and Rouzbehi, K. (2022). Integrated control and monitoring of a smart charging station with a proposed data exchange protocol. IET Renew. Power Gener. 16 (3), 532–546. doi:10.1049/rpg2.12358
Mousavi-Khademi, K., Reza, M., Chamorro, H. R., Vijay, K., Guerrero, J. M., et al. (2020). “Optimal value-based prices placement of der and V2G using planet search algorithm,” in Proceedings of the 2020 IEEE Electric Power and Energy Conference, EPEC, Edmonton, Canada, October 2020, 1–6. doi:10.1109/EPEC48502.2020.93200443
Nallusamy, N., Sakthivel, P., Chausalkar, A., and Arumugam, S. (2016). Electric vehicles. Altern. Fuels Transp., 295–320. doi:10.1201/b16260-10
Pardo-Bosch, F., Pujadas, P., Morton, C., and Cervera, C. (2021). Sustainable deployment of an electric vehicle public charging infrastructure network from a city business model perspective. Sustain. Cities Soc. 71, 102957. doi:10.1016/j.scs.2021.102957
Ravi, S., and Aziz, M. (2022). Utilization of electric vehicles for vehicle-to-grid services: Progress and perspectives. Energies 15 (2), 589. doi:10.3390/en15020589
Reddy, K. R., Meikandasivam, S., and Vijayakumar, D. (2019). A novel strategy for maximization of plug-In electric vehicle’s storage utilization for grid support with consideration of customer flexibility. Electr. Power Syst. Res. 170, 158–175. doi:10.1016/j.epsr.2018.12.031
Rigas, E. S., Karapostolakis, S., Bassiliades, N., and Ramchurn, S. D. (2018). EVLibSim: A tool for the simulation of electric vehicles’ charging stations using the EVLib library. Simul. Model. Pract. Theory 87, 99–119. doi:10.1016/j.simpat.2018.06.007
Sabita, R. (2021). Everything you need to know about machine learning. https://www.analyticsvidhya.com/blog/2021/03/everything-you-need-to-know-about-machine-learning/#h2_1.
Sadeghi-Barzani, B., Payam, A., and Kazemi-Karegar, H. (2014). Optimal fast charging station placing and sizing. Appl. Energy 125, 289–299. doi:10.1016/j.apenergy.2014.03.077
Santra, A., Samanta, A., and Lin, J. (2019). Integrating long short-term memory and genetic algorithm for short-term load forecasting. Energies 12 (11), 2040–2111. doi:10.3390/en12112040
Scott, C., Ahsan, M., and Albarbar, A. (2021). Machine learning based vehicle to grid strategy for improving the energy performance of public buildings. Sustain. Switz. 13 (7), 4003. doi:10.3390/su13074003
Seitaridis, A., Rigas, E. S., Bassiliades, N., and Ramchurn, S. D. (2020). An agent-based negotiation scheme for the distribution of electric vehicles across a set of charging stations. Simul. Model. Pract. Theory 100, 102040. doi:10.1016/j.simpat.2019.102040
Shahriar, S., Al-Ali, A. R., Osman, A. H., Dhou, S., and Nijim, M. (2020). Machine learning approaches for EV charging behavior: A review. IEEE Access 8, 168980–168993. doi:10.1109/ACCESS.2020.3023388
Sheik Mohammed, S., Femin, T., and Sudhakar Babu, T., Sulaiman, S. M., Deb, S., and Kumar, N., (2022). Charge scheduling optimization of plug-in electric vehicle in a PV powered grid-connected charging station based on day-ahead solar energy forecasting in Australia. Sustain. Switz. 14 (6), 3498. doi:10.3390/su14063498
Shi, R., and Lee, K. Y. (2015). Multi-objective optimization of electric vehicle fast charging stations with SPEA-II. IFAC-PapersOnLine 48 (30), 535–540. doi:10.1016/j.ifacol.2015.12.435
Shibl, M., Ismail, L., and Ahmed, M. (2020). Machine learning-based management of electric vehicles charging: Towards highly-dispersed fast chargers. Energies 13 (20), 5429–5524. doi:10.3390/en13205429
Sun, B., Tan, X., Danny, H., and Tsang, K. (2018). Optimal charging operation of battery swapping and charging stations with QoS guarantee. IEEE Trans. Smart Grid 9 (5), 4689–4701. doi:10.1109/TSG.2017.2666815
Sun, D., Ou, Q., Yao, X., Gao, S., Wang, Z., Ma, W., et al. (2020). Integrated human-machine intelligence for EV charging prediction in 5G smart grid. Eurasip J. Wirel. Commun. Netw. 2020 (1), 139. doi:10.1186/s13638-020-01752-y
Uddin, N., and Islam, M. (2019). “Optimal fuzzy logic based smart energy management system for real time application integrating RES, grid and battery,” in Proceedings of the 4th International Conference on Electrical Engineering and Information and Communication Technology, ICEEiCT 2019, Dhaka, Bangladesh, May 2019, 296–301. doi:10.1109/CEEICT.2018.8628057
Velamuri, S., Hari Charan Cherukuri, S., Kumar Sudabattula, S., Natarajan, P., and Hossain, E. (2022). Combined approach for power loss minimization in distribution networks in the presence of gridable electric vehicles and dispersed generation. IEEE Syst. J. 16 (2), 3284–3295. doi:10.1109/JSYST.2021.3123436
Viziteu, A., Furtună, D., Robu, A., Senocico, S., Cioată, P., Marian, R., et al. (2022). Smart scheduling of electric vehicles based on reinforcement learning. Sensors 22 (10), 3718–3814. doi:10.3390/s22103718
Wang, L., and Pedram, M. (2019). QoS guaranteed online management of battery swapping station under dynamic energy pricing. IET Cyber-Physical Syst. Theory Appl. 4 (3), 259–264. doi:10.1049/iet-cps.2018.5041
Wu, H. (2022). A survey of battery swapping stations for electric vehicles: Operation modes and decision scenarios. IEEE Trans. Intelligent Transp. Syst. 23 (8), 10163–10185. doi:10.1109/TITS.2021.3125861
Wu, H., Grantham, K., and Xia, L. (2020). “A realistic and non-linear charging process model for parking lot’s decision on electric vehicles recharging schedule,” in Proceedings of the 2020 IEEE Transportation Electrification Conference and Expo, ITEC, Chicago, IL, USA, June 2020, 2–7. doi:10.1109/ITEC48692.2020.9161535
Wu, H. (2021). A survey of battery swapping stations for electric vehicles: Operation modes and decision scenarios. IEEE Trans. Intelligent Transp. Syst. 23, 10163–10185. doi:10.1109/tits.2021.3125861
Yang, Z. (2015). Electric load movement evaluation and forecasting based on the fourier-series model extend in the least-squares sense. J. Control, Automation Electr. Syst. 26 (4), 430–440. doi:10.1007/s40313-015-0186-2
Zahedmanesh, A., Muttaqi, K. M., and Sutanto, D. (2019). “A consecutive energy management approach for a VPP comprising commercial loads and electric vehicle parking lots integrated with solar PV units and energy storage systems,” in Proceedings of the 2019 IEEE 1st Global Power, Energy and Communication Conference, GPECOM, Nevsehir, Turkey, June 2019. doi:10.1109/GPECOM.2019.8778609
Zeb, M., Zulqarnain, M., Imran, K., Khattak, A., Janjua, A., Pal, A., et al. (2020). Optimal placement of electric vehicle charging stations in the active distribution network. IEEE Access 8, 68124–68134. doi:10.1109/ACCESS.2020.2984127
Zhang, C., Huang, Q., Tian, J., Chen, L., Cao, Y., and Zhang, R. (2011). Smart grid facing the new challenge: The management of electric vehicle charging loads. Energy Procedia 12, 98–103. doi:10.1016/j.egypro.2011.10.014
Keywords: electrification, charging infrastructure, V2G, artificial intelligence, cost analysis
Citation: Dharavat N, Golla NK, Sudabattula SK, Velamuri S, Kantipudi MVVP, Kotb H and AboRas KM (2023) Impact of plug-in electric vehicles on grid integration with distributed energy resources: A review. Front. Energy Res. 10:1099890. doi: 10.3389/fenrg.2022.1099890
Received: 16 November 2022; Accepted: 08 December 2022;
Published: 11 January 2023.
Edited by:
Vikram Kulkarni, SVKM’s NMIMS University, IndiaReviewed by:
Gaddam Sridhar, Jawaharlal Nehru Technological University, Hyderabad, IndiaCopyright © 2023 Dharavat, Golla, Sudabattula, Velamuri, Kantipudi, Kotb and AboRas. This is an open-access article distributed under the terms of the Creative Commons Attribution License (CC BY). The use, distribution or reproduction in other forums is permitted, provided the original author(s) and the copyright owner(s) are credited and that the original publication in this journal is cited, in accordance with accepted academic practice. No use, distribution or reproduction is permitted which does not comply with these terms.
*Correspondence: Hossam Kotb, SG9zc2FtLmtvdGJAYWxleHUuZWR1LmVn
Disclaimer: All claims expressed in this article are solely those of the authors and do not necessarily represent those of their affiliated organizations, or those of the publisher, the editors and the reviewers. Any product that may be evaluated in this article or claim that may be made by its manufacturer is not guaranteed or endorsed by the publisher.
Research integrity at Frontiers
Learn more about the work of our research integrity team to safeguard the quality of each article we publish.