- 1Department of Computer Engineering, Faculty of Computer and Information Sciences, Majmaah University, Al'Majmaah, Saudi Arabia
- 2Department of Electrical Engineering, Faculty of Sciences and Technologies Fez, Sidi Mohamed Ben Abdullah University, Fes, Morocco
- 3High School of Technology Meknes, Moulay Ismail University, Meknes, Egypt
- 4Department of Electrical Power and Machines, Faculty of Engineering, Alexandria University, Alexandria, Egypt
- 5Department of Computer Science, School of Arts and Sciences, University of Central Asia, Bishkek, Kyrgyzstan
- 6Department of Information Systems, College of Computer and Information Sciences, Princess Nourah Bint Abdulrahman University, Riyadh, Saudi Arabia
- 7Faculty of Computers and Information, South Valley University, Qena, Egypt
- 8Department of Informatics, Siberian Federal University, Krasnoyarsk, Russia
Microgrid (MG) technologies offer users attractive characteristics such as enhanced power quality, stability, sustainability, and environmentally friendly energy through a control and Energy Management System (EMS). Microgrids are enabled by integrating such distributed energy sources into the utility grid. The microgrid concept is proposed to create a self-contained system composed of distributed energy resources capable of operating in an isolated mode during grid disruptions. With the Internet of Things (IoT) daily technological advancements and updates, intelligent microgrids, the critical components of the future smart grid, are integrating an increasing number of IoT architectures and technologies for applications aimed at developing, controlling, monitoring, and protecting microgrids. Microgrids are composed of various distributed generators (DG), which may include renewable and non-renewable energy sources. As a result, a proper control strategy and monitoring system must guarantee that MG power is transferred efficiently to sensitive loads and the primary grid. This paper evaluates MG control strategies in detail and classifies them according to their level of protection, energy conversion, integration, benefits, and drawbacks. This paper also shows the role of the IoT and monitoring systems for energy management and data analysis in the microgrid. Additionally, this analysis highlights numerous elements, obstacles, and issues regarding the long-term development of MG control technologies in next-generation intelligent grid applications. This paper can be used as a reference for all new microgrid energy management and monitoring research.
1 Introduction
Real-time power flow management is a contemporary topic in scientific literature. It is gaining prominence to boost the intelligence and adaptability of multi-energy systems, such as smart grids, microgrids, smart homes, and hybrid electric vehicles (George and Ravindran, 2019; George and Ravindran, 2020; George et al., 2021). Microgrid (MG) is a small-scale grid that may unite consumers, conventional power sources, distributed renewable energy sources, and energy storage technologies to form a flexible, self-sufficient, and ecologically beneficial system. A microgrid can operate on AC, DC, or a combination of the two (hybrid mode), and it can be connected to the main grid in parallel or as an isolated power island (George and Ravindran, 2019; George and Ravindran, 2020).
In grid-connected mode, microgrids manage the voltage and frequency of the main power grid. The renewable energy sources are operated in maximum power point mode, supplying the system with the maximum electricity. In islanded mode, the microgrid disconnects from the main grid and relies on distributed energy resources (DERs) to supply load (Sen and Kumar, 2018; Ortiz et al., 2019). In all forms of operation, the balance between power supply and demand is one of the most critical factors governing the microgrid. In grid-connected mode, the main grid is necessary to maintain balance. In island mode, the microgrid must balance the load by increasing its generating capacity or distributing the burden (Kang et al., 2021).
Reduced greenhouse gas emissions, reactive power assistance for enhanced voltage profiles, decentralization of energy supply, heat load integration for cogeneration, auxiliary services, and demand response are among the benefits given by MGs. In addition, it reduces transmission and distribution system interruptions and line losses (Hirsch et al., 2018).
RESs have a high investment cost, whereas MGs have various constraints, including optimal energy consumption, control concerns, a lack of system protection and regulatory standards, and consumer privacy (Azeroual et al., 2020). Due to the increasing deployment of RERs that are intrinsically intermittent and the introduction of probabilistic controlled loads into MGs, research has centered on overcoming its energy management issues. To ensure that a MG operates economically, sustainably, and reliably, its EMS includes both supply- and demand-side control and system limits (Ghiasi, 2019). EMS provides various benefits, from generation dispatch to energy savings, reactive power support to frequency management, reliability to loss cost reduction, energy balancing to lower GHG emissions, and customer engagement to customer privacy (España et al., 2021).
Numerous studies have addressed several MG-related subjects, such as reactive power compensation procedures in MGs, control techniques for enhancing microgrid stability, and MG protection and control schemes. In addition, they studied droop control strategies, voltage, frequency regulation methods, and control strategies for inverter-based MGs (Boujoudar et al., 2020; Vaikund and Srivani, 2021).
They investigated the modeling, design, planning, and designs of hybrid microgrids (Ortiz et al., 2019) and an overview of AC and DC microgrids (Andishgar et al., 2017). In addition, they examined energy management control systems for microgrids (Malik et al., 2017; Tayab et al., 2017). In addition, the literature included evaluations of homeostatic control-based energy-efficient micro generation systems (Amrr et al., 2018), methods for MG uncertainty quantification (Ghiasi et al., 2021a), and a review of network technology-based energy efficiency in buildings and micro generation systems (Fotopoulou et al., 2021). Table 1 contains a compilation of reviews of MG’s energy management.
Unlike other literature studies, this study presents a comprehensive and critical analysis of microgrid energy management systems and control technologies. In addition, the protection and management of Internet of Things-based microgrid monitoring systems are investigated. Several uncertainty quantification approaches are discussed to handle renewable energy resources’ volatile and irregular nature and load demand.
The main goals of this review are to study the development of the MG and EMS as well as to investigate the MG’s components, implementation, classification, objective functions, quality, and protection mechanisms. This study discusses both the present technology and the issues that are addressed with MGs and EMSs. This paper includes an extensive literature review covering the most recent developments in the field of networked MGs. The most important parts of this article are summarized here.
1) A comprehensive discussion on the advantages of MGs as well as their architecture, energy management functions, and topologies in MGs.
2) A comprehensive analysis of the bilateral EMS schemes in MMG, taking into account resilience and transactive operations.
3) Energy management system control techniques used with advantages and drawback of each methods.
4) Microgrid monitoring system using IoT based communication and bulk integration of power sources in MGs. Brief explanations of the most used techniques SACADA system and Thingspeak.
The remainder of the paper will be organized in the following manner: In Section 2, we will discuss the Microgrid control structure and demerits as well as its different functionalities and review the control schemes of the MG and the objectives of an EMS. Section 3 will examine the Microgrid Monitoring systems, which provides an overview of these approaches. Section 4 presents the perspective and discussion. Finally, the Conclusion is documented in Section 5.
2 Microgrid control structure
“A computer system comprising a software platform providing basic support services and a set of applications providing the functionality needed for the effective operation of electrical generation and transmission facilities to assure adequate security of energy supply at minimum cost” (Coelho et al., 2017) is how the International Electrotechnical Commission defines an EMS in the standard IEC 61970 on EMS application program interface in power systems management.
These features are typical of an MG EMS, including decision-making strategy modules. Optimal decisions are transmitted to each generating, storing, and loading unit thanks to DERs/load forecasting, control, supervisory, energy management, and data acquisition modules, among others (Dagar et al., 2021).
MGs can be controlled in ways other parts of the distribution system cannot, making this an important area of study and application. It isn't easy to centrally manage MGs due to their dual functionality (grid-connected and islanded as represented in Figure 1) (Al Alahmadi et al., 2021). Therefore, separate management systems are often developed for each operation mode. Because there are typically multiple DERs and loads connected to a microgrid, coordination challenges arise. When applied consistently, MG control is highly successful (Su and Wang, 2012).
Therefore, a necessary control technique is needed to allow a seamless transition and automation in the event of a sudden disconnection. This is essential for achieving the goal mentioned above regarding power flow between MGs and the power system. In addition, a suitable control strategy is necessary to handle the stochastic generating behavior of DG units in MGs.
The main object of the control strategy is to ensure the microgrid balance during all changes in meteorological conditions and load demands. Stabilizations of voltage and frequency transduce the microgrid balance. Therefore, all proposed techniques in the literatures use frequency or voltage control to ensure the microgrid balance as described.
Below, we will break down the several types of traditional control that can be used to boost MG systems’ effectiveness and dependability to the delight of their clientele.
Various control techniques outlined in Figure 2 (Albarakati et al., 2021) present MG control types typically employed in MG to improve MG’s power and operations. The microgrid control regulates reactive power and MG output voltage.
2.1 Conventional microgrid control techniques
Conventional control methods have been utilized extensively in MGs to maintain voltage and frequency stability and regulation, especially during network failures. MG management is a multi-objective, complicated control system encompassing multiple technical domains, periods, and physical levels. Several areas of interest include load power sharing, voltage/frequency and power quality regulation, market participation, short- and long-term scheduling, etc. (Singh et al., 2020; Singh et al., 2021).
To appropriately handle these challenges, a hierarchical control scheme has been designed and widely regarded as a standard method for efficient MGs management. For example, (Han et al., 2016), describes secondary control for energy management functions and third-level control for multiple MG interactions. Hierarchical control has three levels of control: tertiary, secondary, and primary. Due to the need to maintain real-time frequency and voltage stability in the case of a disruption, primary control levels are considered the most challenging (Zahraoui et al., 2021):
Local electricity, voltage, and current are regulated at the main level. It often executes control operations over interface PCs by adhering to the configuration points specified by upper-level control.
• Secondary level: In the current system, secondary control is positioned above primary control. Along with other things, it handles harmonic compensation, voltage imbalance, and voltage/frequency restoration. Additionally, it is in charge of synchronizing and exchanging electricity with the main grid and other MGs.
• Tertiary management seeks to incorporate intelligence throughout the entire system. Thus, tertiary control will optimize the MG operation in light of competing interests, particularly efficiency and economics. In order to carry out the optimization activities, Information and Communication Technology (ICT) is a significant enabling tool, as it facilitates the acquisition of knowledge from both the MG side and the external grid. Decision-Making (DM) algorithms are used to examine data and decide what steps to take next.
The conventional control approaches in the microgrid are summarized in Table 2.
2.2 Advanced MG control techniques
Improved control performance is a common goal for MG control systems. Hence, sophisticated control techniques are routinely used in these systems. These methods include, for instance, intelligent and adaptive approaches to optimizing the parameters involved in control systems to generate resilient controllers. In the following, we will go over some methods that can be used for MG management. Supervisory controllers in MG energy management can be categorized into three subfields: centralized, decentralized, and distributed. The objective is to improve system performance by adding intelligence while delivering critical functions like power quality monitoring. The relevant group of supervisory controllers is discussed in the next section (Tayab et al., 2017).
2.2.1 Centralized control scheme
The brain of the operation, so to speak, is what we mean when we talk about centralized control schemes. A centralized control management system makes it easy to build and monitor the system in real-time to provide a safe environment. The MG component data and an external grid for the optimization process are necessary for effective operation. We investigated the concept of secondary control for a centralized MG controller in (Jimeno et al., 2011). Hierarchical control, which includes the system’s primary, secondary, and tertiary levels, provides an explanation for the MGs’ centralized control (Atawi and Kassem, 2017).
Centralized microgrid controls are illustrated as a hierarchical network in Figure 3. One Central Processing Unit (CPU) makes all the important decisions in a centralized control setup. It communicates them to the rest of the system’s components based on data and calculations it has received from those components. The MG’s main controller handles every single control and measurement. Local Controllers (LC) obey orders and depend on the MGCC (microgrid central controller) when operating in grid-connected mode but operate autonomously in islanded mode.
2.2.2 Decentralized controls
Decentralized MG administration (Figure 4) has emerged in MG systems, enhancing flexibility and scalability. Due to its plug-and-play functionality, this controller gives great operational flexibility and avoids a single point of failure. Due to decentralization, frequency and voltage regulation, DER coordination, and energy management may all be decentralized (Roslan et al., 2019). In decentralized control, local controllers are primarily responsible for optimizing their production for demand satisfaction and exportation to grids, given current market prices. Multi-Agent Systems (MAS) for decentralized energy management represent an intriguing aspect of supervisory controller research (Sahoo et al., 2021). A MAS is a system composed of several intelligent agents that give local information and interact with one another to pursue various objectives (Khorsandi et al., 2014; Sabri et al., 2019) autonomously. Moreover, a MAS for real-time MG operation is implemented, focusing on generation scheduling and demand-side management, as described in (Al-Saadi et al., 2021). Consequently, there are numerous approaches to the MAS method for the decentralized control of MGs, as explained in detail in (Roslan et al., 2022).
2.2.3 Distributed controls
The distributed control scheme for microgrid management and stability can be described as a method in which local controllers connect with a central controller via communication and networking channels. The central controller is in charge of system monitoring and control, while the local controllers are in order of the other MG components individually (Raya-Armenta et al., 2021).
The following are some of the many advantages of taking this strategy:
• Enhanced sturdiness.
• Improved decision making and system analysis.
• A common control law that can be easily implemented at the central and local levels.
• System monitoring and real-time communication.
According to some studies, the tertiary control level in an MG system may be dispersed. During the deployment of distributed control to compensate for a voltage imbalance in the crucial bus, a current study found power quality disturbances on the generator side and the local bus.
2.3 Microgrid intelligent techniques
A range of intelligent solutions in power system applications can increase control system performance and power source stability. Furthermore, as the number of DG units in MGs increases, so does the complexity of fine-tuning the system’s control settings. Many complex strategies have also been employed to control such parameters in MGs, considering economic load dispatch in MG systems (Azeroual et al., 2022). EMS solutions based on artificial intelligence have been used to increase the efficiency and performance of MG systems, allowing them to fulfill demand while generating the most energy (Chaudhary et al., 2021). Fuzzy logic and neural network techniques consider anticipated values and improve battery lifetime and grid energy trading. These methods are the most commonly employed in microgrid energy management (Pan et al., 2021).
2.3.1 Artificial neural network controller
Data is sent to the central processor via a neural network controller at a delayed rate, much like the human brain. A closed-loop design is used to transfer information to each other while minimizing the error or intended function. The fundamental architecture contains an input/output layer, activation function, weights, and hidden later. Allowing for greater flexibility and a simpler implementation for a variety of operating situations, neural networks are a more intelligent and self-learning controller. MG’s stability is further aided by neural networks’ resilient behavior and swift decision-making capabilities while they are working. In the area of research, Neural Networks (NNs) have gotten a lot of interest. ANNs are among the most successful strategies for optimizing, controlling, and identifying system parameters (Justo et al., 2013).
Furthermore, in MG systems, NNs can tackle difficulties with non-linear data techniques in large-scale systems. Fault tolerance, stability systems, prediction, parametric optimization and identification, self-learning, and load sharing are all examples of NN applications. Furthermore, in grid-connected mode, the main utility grid determines and maintains the nominal voltage and frequency of the entire system. The ANC is used to control the bidirectional DC-DC converter by generating the duty cycle to keep the DC Bus voltage at the reference voltage value in the DC microgrid (Ali and Choi, 2020).
2.3.2 Fuzzy logic controller
The original proposal of the fuzzy theory may be found in (Zia et al., 2018), which was created to provide numerical values for intangible ideas. Fuzzy-logic reasoning is used in the design of a controller in a fuzzy-control system. So-called fuzzy logic aims to create a gray area between zero and one by breaking down logical problems into smaller, more manageable chunks. Figure 5 is a schematic representation of the foundations upon which fuzzy logic control rests. Fuzzification, membership, rule base, fuzzy inference, and defuzzification are all part of the FLC. Distributed control systems have recently incorporated fuzzy logic controllers to choose optimal distributed controller parameters for MG system performance (Rangu et al., 2020). Fuzzy Logic Control (FLC) is an interesting method for MG systems. Many researchers have explored the potential of fuzzy logic methods for resolving the parameters of MG systems. The automatic definition of a fuzzy rule for a fuzzy controller is proposed in (Singh et al., 2022), based on the Tabu search algorithm scheme. A study presented a GA-based fuzzy gain scheduling technique for load frequency regulation in power systems. The GA will construct a fuzzy system without any manual input from the user, saving time and effort that would otherwise be spent on designing and writing fuzzy rules (Hafsi et al., 2022).
The foundations of fuzzy logic control are depicted in Figure 5 as a schematic. The FLC includes fuzzification, membership, rule base, fuzzy inference, and defuzzification.
2.4 Microgrid adaptive techniques
In recent years, adaptive control strategies have been a popular research area. These strategies can be used to maintain system stability, robustness convergence, and optimization in MG applications. Furthermore, these strategies are primarily employed in MG applications to solve and cater to uncertainty parameters and disturbance occurrences. The following section examines a few adaptive approaches often used in MG applications to regulate voltage and frequency variations and hence attain optimum power generation values as summarized in Table 3.
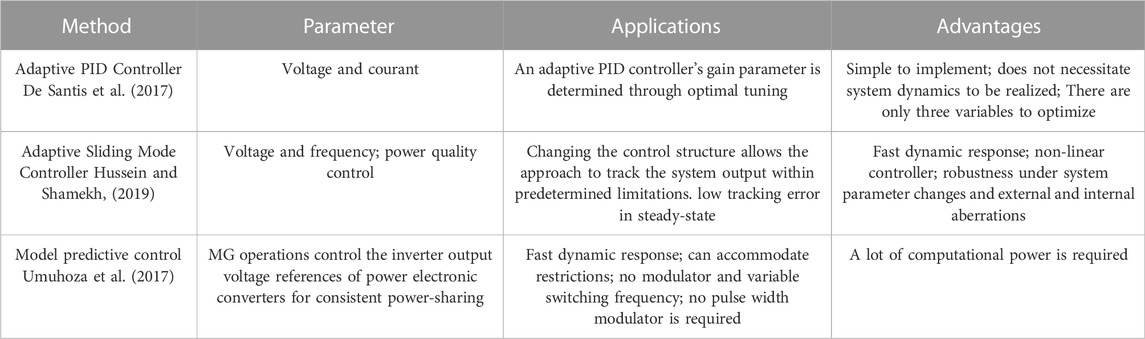
TABLE 3. Comparisons related procedures (De Santis et al., 2017; Albarakati et al., 2021)].
3 Microgrid monitoring system
The monitoring system checks all the equipment’s real-time running status and controls all the equipment to ensure it is safe and stable. Ensuring that the monitor system is always up to date is essential. We ensure that the system is always running in real-time through the software and hardware in this micro-grid.
3.1 IoT
Future network technology, known as the IoT connects information about people, things, and processes to the Internet to create, gather, exchange, and use data. The IoTdid not appear overnight; it has been there for a while. Many other names have referred to it, and as technology develops, so do its technologies and ideas. Machine-to-Machine (M2M) and Radio frequency Identification (RFID) are two examples of good ideas (object intelligence communication). Everything is connected to the Internet through a concept known as the “Internet of Things.” However, why should items be connected to the Internet, not how should they be connected to the Internet? The ultimate objective of the Internet of Objects is to intelligently connect everything in our environment to the Internet, automate it with minimal human involvement, and then deliver knowledge and improved services to people through information fusion across several connections. For assessing and disseminating the resulting information, it is essential to connect human, physical, geographic, and intangible data (Siddique et al., 2019).
The term “Internet of Things” describes a notion that integrates real objects and labor. Put another way, it includes everything we come into contact with daily, such as people, machines, electrical equipment, bicycles, glasses, watches, clothing, cultural artifacts, animals, and plants. A solid connection to the information network, intelligent interfaces, and physical and virtual entities with distinct identifiers and attributes are other components of the IoT (Roy et al., 2020).
IoT devices may interact with and respond to objects, environments, data, and environmental information as active participants in business, news, and social activities. Whether a human is directly involved, an object responds autonomously to a physical event in the real world or runs a process that creates a service or starts a certain activity. The interface of the service type enables Internet-based communication with such smart devices and searches for or exchanges data relevant to the status of the items while taking security and privacy concerns into account (Hu et al., 2021).
Microgrids’ dependability, stability, security, and environmental sustainability are all guaranteed by the IoT. With the help of the Internet of Things, a futuristic network technology, data generated by people, things, processes, and other objects can be produced, gathered, shared, and used. IoT technologies have recently gained popularity due to their development and use in SMG applications. Bidirectional connectivity, self-healing, decentralization, and smart metering are crucial IoT technologies. A flexible and intelligent EMS must monitor and control all SMG variables in real-time (Vermesan et al., 2011).
3.2 Microgrid monitoring system using IoT
MG energy management systems will become substantially more efficient due to collecting and analyzing data from power sources via IoT. Additionally, utilities may perform operational duties such as shortening outage investigation times, optimizing load balancing, optimizing line voltage, finding faults, decreasing service costs, and restoring services more quickly. Additionally, using IoT technology for smart homes, Energy Storage Systems (ESS), Electric Vehicles (EVs), charging stations, and variable loads increases the flexibility and dependability of the SMG (Ahmad et al., 2017). As seen in Figure 6, IoT may improve energy efficiency by managing Demand Response (DR), collecting data, sharing, and trading energy. An optimum DSM system built on the IoT is necessary to address energy conservation and management challenges. This technique should provide a continuous energy supply while preventing power surges in the future (Zamfir et al., 2016).
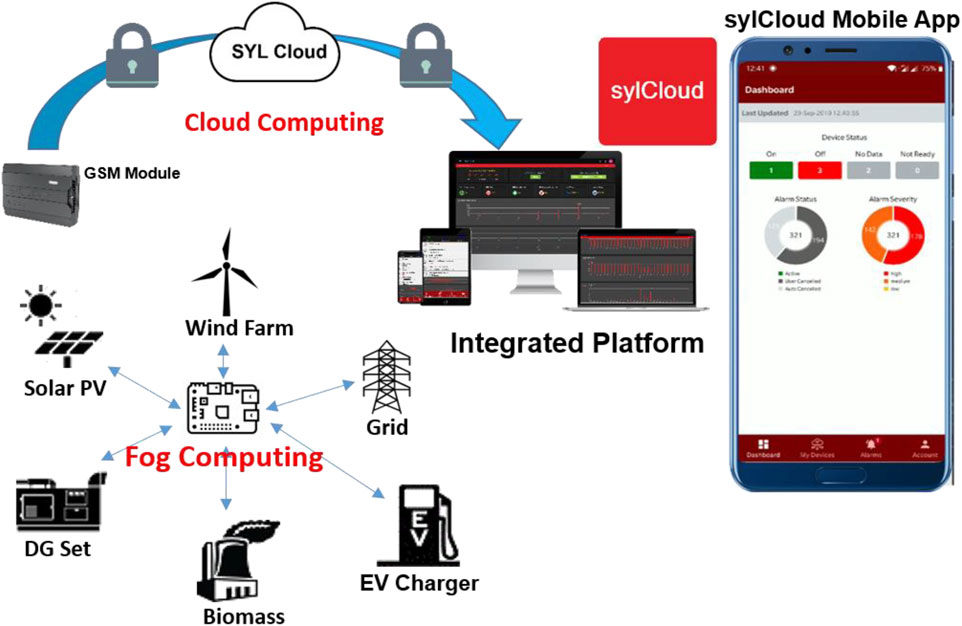
FIGURE 6. Applications of IoT in intelligent power grids (Khatua et al., 2020).
The microgrid is equipped with various sensors for data collection (current, voltage, power, temperature). The data collected from these sensors is analyzed in real-time to determine the optimal control strategy based on current conditions (occupancy, energy consumption-production, and weather conditions data). Additionally, this data must be saved for subsequent study, notably to build predictive control techniques (Elmouatamid et al., 2019). In addition, multiple platforms that incorporate IoT technologies are installed for data collecting, processing, and visualization, and with the upcoming generation of wireless. In the present day, a smart microgrid is all about electronic communication networks, electronic billing systems, and smart meters. The smart microgrid will be equipped with automated distribution and secure DER administration and generation on the road to 5G. Automatic power generation and distribution via real-time load balancing and massively distributed generation services (Sedhom et al., 2021) is the focus of a smart microgrid in the 5G age. The IoT is used in various applications, including smart grid, microgrids, intelligent buildings, and intelligent control devices, to monitor and track essential information about the target environment. Numerous studies have used IoT solutions for energy management and system monitoring in a microgrid (Sylcloud Smart Micro Grid, 2022).
Reference (Khan et al., 2018) proposes a communication platform that may operate in both central and dispersed modes in the event of a communication hierarchy failure. This research explores the effect of a hybrid communication system with communication delay on the MMG EMS. In other words, the hierarchical communication system supports the EMS in reducing MMG’s operating expenses.
The authors of (Draft, 2016) demonstrate the importance of the IoTin building and deploying intelligent microgrids, including their benefits, difficulties, and hazards and several processes, techniques, and procedures for controlling and protecting intelligent microgrids.
In (Ali and Choi, 2020), the authors study the real-time features of security mechanisms for IoT connectivity. In addition, the influence of IoT protocols on the real-time needs of smart grid operations are investigated (protection, control, and monitoring).
The authors of (Moghimi et al., 2018) employed an IoT system to monitor company energy use. The monitoring platform incorporated various technologies, including digital instrumentation, communication networks, software, and databases, with the aims of central administration, decentralized control, and remote monitoring.
In (Ghiasi et al., 2022), authors have created a remote energy monitoring system based on the IoT to control, plan, optimize, and conserve energy in smart grids and homes. A system that efficiently collects energy resource information in the house reduces energy wastage and provides information for analyzing energy consumption patterns.
In (Kondoro et al., 2021), the authors propose an EMS based on an advanced IoT system. The framework system was implemented in the building to unify and standardize the things that comprise the built environment. Appropriate guidelines are developed with intelligent energy management and smart Buildings’ available mode of operation.
The authors of (Khoa et al., 2021) present a real-time monitoring system using web server technology for the microgrid. The Arduino embedded system was used as a control core, and an Ethernet network module was used for the data acquisition and the wireless transmission.
In this study, an IoT system was used to measure, track, and record a few power quality (PQ) aspects of a microgrid system (Ku et al., 2017). PQ parameters are measured in the hardware unit by three PZEM-004T modules that have non-invasive Current Transformer (CT) sensors installed. The sensors’ data is gathered simultaneously by an Arduino WeMos D1 R1 ESP8266 microcontroller and transmitted to the server through the Internet.
3.3 Microgrid monitoring system using SCADA
A Supervisory Control and Data Acquisition (SCADA) system is another option for microgrid monitoring and energy management in small and large-scale buildings (Residential, Commercial and Industrial). A SCADA system comprises two components: a hardware system for data collection, communication, control, and operation, and a software system for data storage, elaboration, visualization, optimization, and careful management (Figure 7). The SCADA system, a type of middleware used in intelligent monitoring systems, is discussed in this section. The SCADA system is mostly used to read bundled microgrid data. The SCADA system accesses the microgrid data before saving it in the MySQL database (Marinakis and Doukas, 2018).
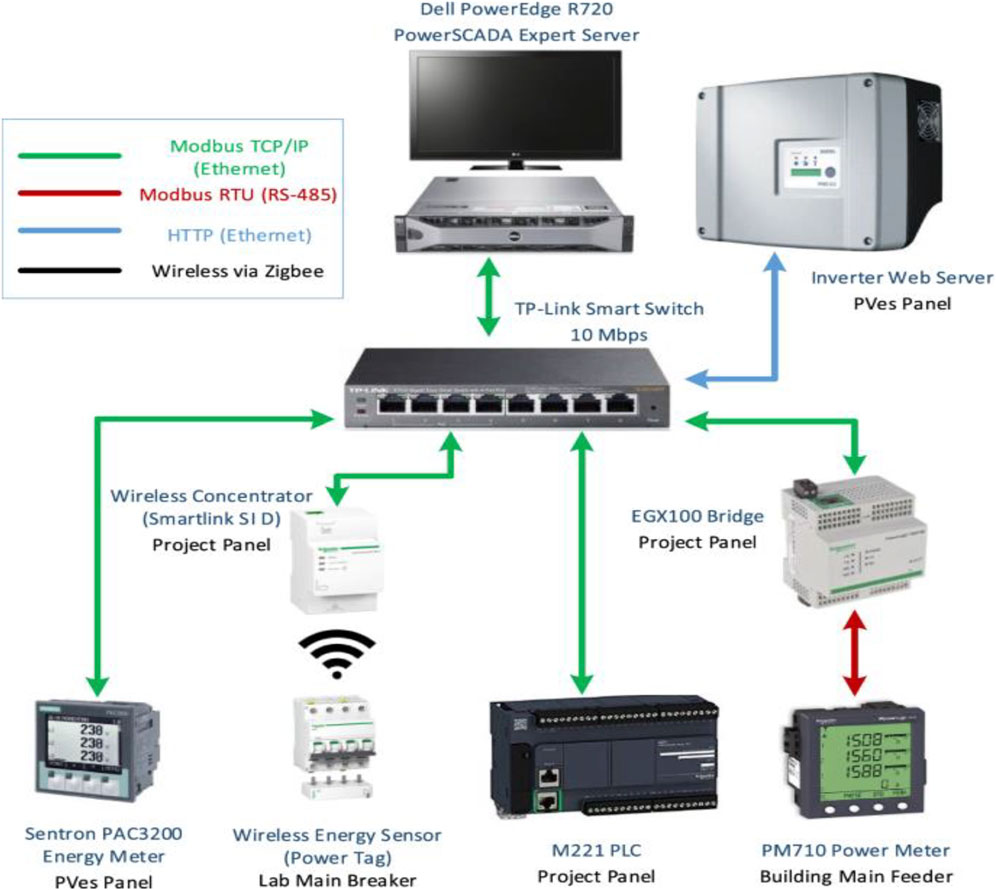
FIGURE 7. SCADA Monitoring system for microgrid (Marinakis and Doukas, 2018).
Four major kinds of SCADA hardware functions exist. The first is the Remote Terminal Unit (RTU), whose primary role is to gather data for the SCADA system. The second role is the communication platform, which facilitates the establishment of data links between devices (Zhuang et al., 2017). The third function is the Programmable Logic Controller (PLC), which is needed to ensure that the MG operates properly in grid-connected and island modes (Khoa et al., 2021).
The Human Machine Interface (HMI), a software component of the SCADA system, is critical for controlling and monitoring. The SCADA component’s conventional structure is server-client, with the primary SCADA application operating on the server and the HMI running on the client. Numerous articles examined various aspects of SCADA use in MGs. By comparison, this work is unusual because it employs all four SCADA elements to accomplish a new aim of intelligent energy management (Ali et al., 2021).
3.4 Microgrid monitoring system using cloud computing
Another approach to microgrid monitoring is based on the communication between powers sources and the monitoring platform using the cloud. The measured data is sent directly to the cloud by measurement unit as shown in Figure 8.
Cloud computing and the IoT have evolved independently thus far. Non-etheless, their integration into microgrids has demonstrated significant reciprocal benefits. The cloud, in particular, can provide an efficient and practical option for administering IoT services and offering some applications to help in data analysis. Many researchers have been used this structure of monitoring due to its benefits in high-quality data transmission (Ghiasi et al., 2021b).
The authors in (Kermani et al., 2020) present a real-time design of efficient monitoring and control of grid power systems using the remote cloud server to improve the universal control and response time, with a new security approach in access to user authentication.
The authors of (Gupta and Rastogi, 2021) provide a cloud computing platform for microgrid power management. The strategy links the system’s current computer and storage capabilities with external computing devices, enhancing data processing and interaction and providing a cost-effective and rapid mechanism for microgrids to meet their computational requirements.
The authors in (Gui and MacGill, 2018) use a low-cost IoT-based innovative communication platform to implement an optimal energy management technique for microgrid systems. The suggested system’s energy monitoring and control architecture is built on a cloud-based Remote Monitoring Unit (RMU) that communicates via a Message Queuing Telemetry Transport (MQTT) server and Thingspeak Middleware.
Thingspeak is a cloud-based IoT analytics platform that lets users visualize and analyze real-time data streams. It can do online data stream analysis and processing and immediately visualize data presented by system gateways. Thingspeak is frequently used in IoT systems that require analytics for prototyping and proof of concept (Khatua et al., 2020). Thingspeak is an IoT platform that stores data delivered by apps or devices via channels. You have numerous options for writing data to your Thingspeak platform: HTTP requests through the REST API, MQTT. Thingspeak is frequently used in IoT systems that require analytics for prototyping and proof of concept (Figure 9).
Due to the development of the connection between Matlab and Thingspeak by adding the IoT Matlab toolbox. Thingspeak is the most used platform during the simulation of the power system at Matlab/Simulink. The quality of communication and microgrid monitoring depends on the sensors used for measurement (Bera et al., 2014).
To make an intelligent distribution system functional, many networks and devices must come together to create a dependable system. The IoT and smart meters are the significant components that will transform the traditional link between consumer and operator into an innovative interdependent system capable of quicker and more reliable communication (Karthick et al., 2021). In recent years, disc-type meters have been phased out in favor of electronic integrated circuit embedded meters, which distribution utility companies effectively employ to provide consumers with authentic and electronic invoicing (Chen et al., 2019). The requirement for enhanced flexible billing and management of billing information in the case of two-way power flow necessitates intelligent meter technology. In commercial and industrial settings, smart meter technology gives customers daily market values for their energy use (Mehrizi-Sani and Iravani, 2010). Historically, Automated Meter Reading (AMR) technology collected data on customers’ and utilities’ energy use, resulting in a one-way flow of energy and communication. The AMR, a recent advancement in Advanced Metering Infrastructure (AMI), enables bidirectional communication and power flow between the meter and the central control system (Mohanty et al., 2016).
A smart grid is a novel approach to transmission and distribution, and intelligent meters play a critical role in connecting with consumers and collecting data as an essential element of the smart grid. The smart meter comprises three major components: network management, advanced metering element, and data management unit (Vuddanti and Salkuti, 2021). The smart meter has a memory chip that enables users to monitor their energy consumption using a software interface, allowing it to communicate in two directions (Zheng et al., 2013). The smart meter regulates the functioning of the distribution system’s switches and recloses, ensuring an efficient and reliable delivery system. Due to the smart meter’s two-way communication capability and energy interface, it is possible to regulate distribution infrastructure by sending directives to the control center, a process known as distribution automation at the load end (Guo et al., 2015). The benefit of the smart meter is that it enables central control to intervene quickly when tampering occurs, based on the accessible rapid report supplied by the smart meter as part of the data collection process (Barai et al., 2015). This contributes to reducing power theft while also enhancing the security of the power system. The availability of daily billing information to consumers enables them to regulate their loads and save money using the smart meter (Avancini et al., 2021).
4 Perspective and discussion
This work presents an extensive literature analysis of the issues of stability, control, and power management of AC, DC, and hybrid AC/DC microgrids. According to the research, AC and DC microgrids are both very common. Still, hybrid AC/DC microgrids are gaining popularity due to their lower conversion losses, greater reliability, and increased efficiency. The microgrids use a hierarchical control architecture that features main, secondary, and tertiary controllers in the chain of command. The primary control, often known as the LC, is built into the DER to guarantee dependable operation by preserving stable voltage and frequency levels and regulating power. Secondary control regulates microgrid communication protocols to improve power quality by reducing steady-state voltage and frequency mistakes created by primary control. The main control caused these faults. The tertiary management manages the power flow between the main grid and the microgrid to ensure that the system operates cost-effectively. Nevertheless, possibilities to develop improved planning, operation, and control strategies. It is possible to do extensive studies in these areas to improve microgrids’ development, operation, and control.
• The microgrids require accurate system modeling for efficient planning and operation. Considering the stochastic character of the integrated DGs in microgrids, a new or enhanced model can be designed to reduce planning and operation expenses.
• It is possible to redesign or develop several advanced control approaches, such as adaptive control, model predictive control, robust control, and optimum control, to ensure that microgrids have efficient DC and AC buses voltage management and concurrent power sharing.
• Integrating renewable sources in microgrids lowers the overall inertia of DC microgrids, decreasing the microgrids’ voltage control performance. Even though certain control strategies have been established to deal with such a scenario, more work should be undertaken in this regard because the DC microgrid is becoming a system based entirely on renewable energy.
• In the future, MGs may use various energy sources, such as large-scale decentralized resources, to be flexible and fit their needs. The way energy is made and moved could change because of energy storage systems.
• Future MGs could improve at finding faults and fixing themselves, which would cut down on recovery time, get more loads back online, and find gaps between research and implementation.
• The IoT makes it easier for real-time platforms to form and connect
• decentralized and transactional energy markets in a very important way. From their previous research, the authors also know that two-way energy exchanges between customers and producers will likely be the most difficult in the future. However, new technologies are likely to solve this problem.
• Deep learning, like ANN, could be used in MGs instead of classical and mathematical methods to achieve dynamic changes in energy flow, lower GHG emissions, and better protection for MGs. Blockchains and smart contracts should be used in MGs to ensure that energy transactions and DER operations are safe (Zahraoui et al., 2021).
5 Conclusion
This article examines recent research on the various energy management techniques proposed for microgrids, including classical, heuristic, and intelligent algorithms. Additionally, this article discusses the design of microgrids, their many classifications, the components of a microgrid, the communication technologies utilized, and the auxiliary services necessary in a microgrid. It addresses significant energy management applications, data management, and control structure. Additionally, this article provides a brief examination of monitoring methods used for the data analysis of the microgrid. The EMS is vital to the MG’s proper operation. An overview of MG control and various energy management schemes has been studied to optimize and stabilize the MG’s functioning while optimizing the use of renewable energy resources. The developed hardware and software for the computer control and monitoring system for electricity generation allows the process to provide continuous user access to sensor data regardless of the state of the external power supply, to unify the approach to data collection via the use of an intermediate data aggregation node, and to provide convenient access to data processing via a diverse set of client interfaces. The impacts of the integration of electric vehicles on microgrid stability and balance and microgrid energy management during cybersecurity attacks represent the topics of our future works.
Data availability statement
The original contributions presented in the study are included in the article/supplementary material, further inquiries can be directed to the corresponding author.
Author contributions
All authors listed have made a substantial, direct, and intellectual contribution to the work and approved it for publication.
Conflict of interest
The authors declare that the research was conducted in the absence of any commercial or financial relationships that could be construed as a potential conflict of interest.
Publisher’s note
All claims expressed in this article are solely those of the authors and do not necessarily represent those of their affiliated organizations, or those of the publisher, the editors and the reviewers. Any product that may be evaluated in this article, or claim that may be made by its manufacturer, is not guaranteed or endorsed by the publisher.
References
Ahmad, F., Alam, M. S., and Asaad, M. (2017). Developments in xEVs charging infrastructure and energy management system for smart microgrids including xEVs. Sustain. cities Soc. 35, 552–564. doi:10.1016/j.scs.2017.09.008
Ahmethodzic, L., and Music, M. (2021). Comprehensive review of trends in microgrid control. Renew. Energy Focus 38, 84–96. doi:10.1016/j.ref.2021.07.003
Al Alahmadi, A. A., Belkhier, Y., Ullah, N., Abeida, H., Soliman, M. S., Khraisat, Y. S. H., et al. (2021). Hybrid wind/PV/battery energy management-based intelligent non-integer control for smart DC-microgrid of smart University. IEEE Access 9, 98948–98961. doi:10.1109/access.2021.3095973
Al-Saadi, M., Al-Greer, M., and Short, M. (2021). Strategies for controlling microgrid networks with energy storage systems: A review. Energies 14 (21), 7234. doi:10.3390/en14217234
Albarakati, A. J., Boujoudar, Y., Azeroual, M., Jabeur, R., Aljarbouh, A., El Moussaoui, H., et al. (2021). Real-time energy management for DC microgrids using artificial intelligence. Energies 14 (17), 5307. doi:10.3390/en14175307
Ali, M. A., Barakat, M. M., Abokhalaf, M. M., Fadel, Y. H., Kandil, M., Rasmy, M. W., et al. (2021). Micro-grid monitoring and supervision: Web-based SCADA approach. J. Electr. Eng. Technol. 16 (5), 2313–2331. doi:10.1007/s42835-021-00762-0
Ali, S. S., and Choi, B. J. (2020). State-of-the-art artificial intelligence techniques for distributed smart grids: A review. Electronics 9 (6), 1030. doi:10.3390/electronics9061030
Amrr, S. M., Alam, M. S., Asghar, M. J., and Ahmad, F. (2018). Low cost residential microgrid system based home to grid (H2G) back up power management. Sustain. Cities Soc. 36, 204–214. doi:10.1016/j.scs.2017.10.016
Andishgar, M. H., Gholipour, E., and Hooshmand, R. A. (2017). An overview of control approaches of inverter-based microgrids in islanding mode of operation. Renew. Sustain. Energy Rev. 80, 1043–1060. doi:10.1016/j.rser.2017.05.267
Arsad, A. Z., Hannan, M. A., Lipu, M. H., Rahman, S. A., Ker, P. J., Mansor, M., et al. (2022). Rule-based fuzzy controller for solid-state transfer switch towards fast sensitive loads transfer. IEEE Trans. Industry Appl. 58, 1888–1898. doi:10.1109/tia.2022.3146100
Atawi, I. E., and Kassem, A. M. (2017). Optimal control based on maximum power point tracking (MPPT) of an autonomous hybrid photovoltaic/storage system in microgrid applications. Energies 10 (5), 643. doi:10.3390/en10050643
Avancini, D. B., Rodrigues, J. J., Rabêlo, R. A., Das, A. K., Kozlov, S., and Solic, P. (2021). A new IoT-based smart energy meter for smart grids. Int. J. Energy Res. 45 (1), 189–202. doi:10.1002/er.5177
Azeroual, M., Boujoudar, Y., Iysaouy, L. E., Aljarbouh, A., Fayaz, M., Qureshi, M. S., and Markhi, H. E. (2022). Energy management and control system for microgrid based wind-PV-battery using multiagent systems. Wind Eng. 46, 0309524X221075583. doi:10.1177/0309524X221075583
Azeroual, M., Lamhamdi, T., El Moussaoui, H., and El Markhi, H. (2020). Intelligent EMS of a smart microgrid using multiagent systems. Archives Electr. Eng., 23–38.
Barai, G. R., Krishnan, S., and Venkatesh, B. (20152015). IEEE electrical power and energy conference (EPEC). IEEE, 138–145.Smart metering and functionalities of smart meters in smart grid-a review
Bera, S., Misra, S., and Rodrigues, J. J. (2014). Cloud computing applications for smart grid: A survey. IEEE Trans. Parallel Distributed Syst. 26 (5), 1477–1494. doi:10.1109/tpds.2014.2321378
Boujoudar, Y., Azeroual, M., El Moussaoui, H., and Lamhamdi, T. (2020). Intelligent controller based energy management for stand-alone power system using artificial neural network. Int. Trans. Electr. Energy Syst. 30 (11), e12579. doi:10.1002/2050-7038.12579
Chaudhary, G., Lamb, J. J., Burheim, O. S., and Austbø, B. (2021). Review of energy storage and energy management system control strategies in microgrids. Energies 14 (16), 4929. doi:10.3390/en14164929
Chen, Y. Y., Lin, Y. H., Kung, C. C., Chung, M. H., and Yen, I. H. (2019). Design and implementation of cloud analytics-assisted smart power meters considering advanced artificial intelligence as edge analytics in demand-side management for smart homes. Sensors 19 (9), 2047. doi:10.3390/s19092047
Coelho, V. N., Cohen, M. W., Coelho, I. M., Liu, N., and Guimarães, F. G. (2017). Multiagent systems applied for energy systems integration: State-of-the-art applications and trends in microgrids. Appl. energy 187, 820–832. doi:10.1016/j.apenergy.2016.10.056
Dagar, A., Gupta, P., and Niranjan, V. (2021). Microgrid protection: A comprehensive review. Renew. Sustain. Energy Rev. 149, 111401. doi:10.1016/j.rser.2021.111401
De Santis, E., Rizzi, A., and Sadeghian, A. (2017). Hierarchical genetic optimization of a fuzzy logic system for energy flows management in microgrids. Appl. Soft Comput. 60, 135–149. doi:10.1016/j.asoc.2017.05.059
Dileep, G. (2020). A survey on smart grid technologies and applications. Renew. energy 146, 2589–2625. doi:10.1016/j.renene.2019.08.092
Elmouatamid, A., Ouladsine, R., Bakhouya, M., El Kamoun, N., Zine-Dine, K., and Khaidar, M. (2019). “A model predictive control approach for energy management in micro-grid systems,” in 2019 international conference on smart energy systems and technologies (SEST) (IEEE), 1–6.
España, N., Barco-Jiménez, J., Pantoja, A., and Quijano, N. (2021). Distributed population dynamics for active and reactive power dispatch in islanded microgrids. Int. J. Electr. Power & Energy Syst. 125, 106407. doi:10.1016/j.ijepes.2020.106407
Fotopoulou, M., Rakopoulos, D., Trigkas, D., Stergiopoulos, F., Blanas, O., and Voutetakis, S. (2021). State of the art of low and medium voltage direct current (Dc) microgrids. Energies 14 (18), 5595. doi:10.3390/en14185595
George, A., and Ravindran, A. (2019). “Distributed middleware for edge vision systems,” in 2019 IEEE 16th international conference on smart cities: Improving quality of life using ICT & IoT and AI (HONET-ICT) (IEEE), 193–194.
George, A., Ravindran, A., Mendieta, M., and Tabkhi, H. (2021). Mez: An adaptive messaging system for latency-sensitive multi-camera machine vision at the iot edge. IEEE Access 9, 21457–21473. doi:10.1109/access.2021.3055775
George, A., and Ravindran, A. (2020). “Scalable approximate computing techniques for latency and bandwidth constrained IoT edge,” in International summit smart city 360° (Cham: Springer), 274–292.
Ghiasi, M., Dehghani, M., Niknam, T., Kavousi-Fard, A., Siano, P., and Alhelou, H. H. (2021). Cyber-attack detection and cyber-security enhancement in smart DC-microgrid based on blockchain technology and Hilbert Huang transform. Ieee Access 9, 29429–29440. doi:10.1109/access.2021.3059042
Ghiasi, M. (2019). Detailed study, multi-objective optimization, and design of an AC-DC smart microgrid with hybrid renewable energy resources. Energy 169, 496–507. doi:10.1016/j.energy.2018.12.083
Ghiasi, M., Niknam, T., Dehghani, M., Siano, P., Haes Alhelou, H., and Al-Hinai, A. (2021). Optimal multi-operation energy management in smart microgrids in the presence of ress based on multi-objective improved de algorithm: Cost-emission based optimization. Appl. Sci. 11 (8), 3661. doi:10.3390/app11083661
Ghiasi, M., Wang, Z., Mehrandezh, M., Jalilian, S., and Ghadimi, N. (2022). Evolution of smart grids towards the Internet of energy: Concept and essential components for deep decarbonisation. IET Smart Grid. doi:10.1049/stg2.12095
Ghosal, A., and Conti, M. (2019). Key management systems for smart grid advanced metering infrastructure: A survey. IEEE Commun. Surv. Tutorials 21 (3), 2831–2848. doi:10.1109/comst.2019.2907650
Gui, E. M., and MacGill, I. (2018). Typology of future clean energy communities: An exploratory structure, opportunities, and challenges. Energy Res. Soc. Sci. 35, 94–107. doi:10.1016/j.erss.2017.10.019
Guo, Y., Tan, C. W., Hu, S., and Weaver, W. W. (2015). “Modeling distributed denial of service attack in advanced metering infrastructure,” in 2015 IEEE power & energy society innovative smart grid technologies conference (ISGT) (IEEE), 1–5.
Gupta, B. K., and Rastogi, V. (2021). Integration of technology to access the manufacturing plant via remote access system-A part of Industry 4.0. Mater. Today Proc. 56, 3497–3505. doi:10.1016/j.matpr.2021.11.135
Hafsi, O., Abdelkhalek, O., Mekhilef, S., Soumeur, M. A., Hartani, M. A., and Chakar, A. (2022). Integration of hydrogen technology and energy management comparison for DC-Microgrid including renewable energies and energy storage system. Sustain. Energy Technol. Assessments 52, 102121. doi:10.1016/j.seta.2022.102121
Han, Y., Li, H., Shen, P., Coelho, E. A. A., and Guerrero, J. M. (2016). Review of active and reactive power sharing strategies in hierarchical controlled microgrids. IEEE Trans. Power Electron. 32 (3), 2427–2451. doi:10.1109/tpel.2016.2569597
Hirsch, A., Parag, Y., and Guerrero, J. (2018). Microgrids: A review of technologies, key drivers, and outstanding issues. Renew. Sustain. Energy Rev. 90, 402–411. doi:10.1016/j.rser.2018.03.040
Hu, J., Shan, Y., Guerrero, J. M., Ioinovici, A., Chan, K. W., and Rodriguez, J. (2021). Model predictive control of microgrids–An overview. Renew. Sustain. Energy Rev. 136, 110422. doi:10.1016/j.rser.2020.110422
Hussein, T., and Shamekh, A. (2019). Design of PI fuzzy logic gain scheduling load frequency control in two-area power systems. Designs 3 (2), 26. doi:10.3390/designs3020026
Jimeno, J., Anduaga, J., Oyarzabal, J., and de Muro, A. G. (2011). Architecture of a microgrid energy management system. Eur. Trans. Electr. Power 21 (2), 1142–1158. doi:10.1002/etep.443
Justo, J. J., Mwasilu, F., Lee, J., and Jung, J. W. (2013). AC-microgrids versus DC-microgrids with distributed energy resources: A review. Renew. Sustain. energy Rev. 24, 387–405. doi:10.1016/j.rser.2013.03.067
Kang, K. M., Choi, B. Y., Lee, H., An, C. G., Kim, T. G., Lee, Y. S., et al. (2021). Energy management method of hybrid AC/DC microgrid using artificial neural network. Electronics 10 (16), 1939. doi:10.3390/electronics10161939
Karthick, T., Chandrasekaran, K., and Jeslin., D. N. J. (2021). Design of IoT based smart compact energy meter for monitoring and controlling the usage of energy and power quality issues with demand-side management for a commercial building. Sustain. Energy, Grids Netw. 26, 100454. doi:10.1016/j.segan.2021.100454
Kaur, A., Kaushal, J., and Basak, P. (2016). A review on microgrid central controller. Renew. Sustain. Energy Rev. 55, 338–345. doi:10.1016/j.rser.2015.10.141
Kermani, M., Carnì, D. L., Rotondo, S., Paolillo, A., Manzo, F., and Martirano, L. (2020). A nearly zero-energy microgrid testbed laboratory: Centralized control strategy based on SCADA system. Energies 13 (8), 2106. doi:10.3390/en13082106
Khan, K. R., Rahman, A., Nadeem, A., Siddiqui, M. S., and Khan, R. A. (2018). “Remote monitoring and control of microgrid using smart sensor network and Internet of thing,” in 2018 1st international conference on computer applications & information security (ICCAIS) (IEEE), 1–4.
Khatua, P. K., Ramachandaramurthy, V. K., Kasinathan, P., Yong, J. Y., Pasupuleti, J., and Rajagopalan, A. (2020). Application and assessment of internet of things toward the sustainability of energy systems: Challenges and issues. Sustain. Cities Soc. 53, 101957. doi:10.1016/j.scs.2019.101957
Khoa, N. M., Dai, L. V., Tung, D. D., and Toan, N. A. (2021). An advanced IoT system for monitoring and analysing chosen power quality parameters in micro-grid solution. Archives Electr. Eng. 70 (1).
Khorsandi, A., Ashourloo, M., and Mokhtari, H. (2014). A decentralized control method for a low-voltage DC microgrid. IEEE Trans. Energy Convers. 29 (4), 793–801. doi:10.1109/tec.2014.2329236
Kondoro, A., Dhaou, I. B., Tenhunen, H., and Mvungi, N. (2021). Real time performance analysis of secure IoT protocols for microgrid communication. Future Gener. Comput. Syst. 116, 1–12. doi:10.1016/j.future.2020.09.031
Ku, T. Y., Park, W. K., and Choi, H. (2017). “IoT energy management platform for microgrid,” in 2017 IEEE 7th international conference on power and energy systems (ICPES) (IEEE), 106–110.
Kumar, K. P., and Saravanan, B. (2017). Recent techniques to model uncertainties in power generation from renewable energy sources and loads in microgrids–a review. Renew. Sustain. Energy Rev. 71, 348–358. doi:10.1016/j.rser.2016.12.063
Mahmoud, M. S., Alyazidi, N. M., and Abouheaf, M. I. (2017). Adaptive intelligent techniques for microgrid control systems: A survey. Int. J. Electr. Power & Energy Syst. 90, 292–305. doi:10.1016/j.ijepes.2017.02.008
Malik, S. M., Ai, X., Sun, Y., Zhengqi, C., and Shupeng, Z. (2017). Voltage and frequency control strategies of hybrid AC/DC microgrid: A review. IET Generation, Transm. Distribution 11 (2), 303–313. doi:10.1049/iet-gtd.2016.0791
Marinakis, V., and Doukas, H. (2018). An advanced IoT-based system for intelligent energy management in buildings. Sensors 18 (2), 610. doi:10.3390/s18020610
Mehrizi-Sani, A., and Iravani, R. (2010). Potential-function based control of a microgrid in islanded and grid-connected modes. IEEE Trans. Power Syst. 25 (4), 1883–1891. doi:10.1109/tpwrs.2010.2045773
Moghimi, M., Jamborsalamati, P., Hossain, J., Stegen, S., and Lu, J. (2018). “A hybrid communication platform for multi-microgridEMSoptimization,” in 2018 IEEE 27th international symposium on industrial electronics (ISIE) (IEEE), 1215–1220.
Mohanty, S. P., Choppali, U., and Kougianos, E. (2016). Everything you wanted to know about smart cities: The Internet of things is the backbone. IEEE Consum. Electron. Mag. 5 (3), 60–70. doi:10.1109/mce.2016.2556879
Ortiz, L., Orizondo, R., Águila, A., González, J. W., López, G. J., and Isaac, I. (2019). Hybrid AC/DC microgrid test system simulation: Grid-connected mode. Heliyon 5 (12), e02862. doi:10.1016/j.heliyon.2019.e02862
Pan, Y., Sangwongwanich, A., Yang, Y., and Blaabjerg, F. (2021). Distributed control of islanded series PV-battery-hybrid systems with low communication burden. IEEE Trans. Power Electron. 36 (9), 10199–10213. doi:10.1109/tpel.2021.3063111
Rangu, S. K., Lolla, P. R., Dhenuvakonda, K. R., and Singh, A. R. (2020). Recent trends in power management strategies for optimal operation of distributed energy resources in microgrids: A comprehensive review. Int. J. Energy Res. 44 (13), 9889–9911. doi:10.1002/er.5649
Raya-Armenta, J. M., Bazmohammadi, N., Avina-Cervantes, J. G., Saez, D., Vasquez, J. C., and Guerrero, J. M. (2021). Energy management system optimization in islanded microgrids: An overview and future trends. Renew. Sustain. Energy Rev. 149, 111327. doi:10.1016/j.rser.2021.111327
Roslan, M. F., Hannan, M. A., Ker, P. J., Mannan, M., Muttaqi, K. M., and Mahlia, T. I. (2022). Microgrid control methods toward achieving sustainable energy management: A bibliometric analysis for future directions. J. Clean. Prod. 348, 131340. doi:10.1016/j.jclepro.2022.131340
Roslan, M. F., Hannan, M. A., Ker, P. J., and Uddin, M. N. (2019). Microgrid control methods toward achieving sustainable energy management. Appl. Energy 240, 583–607. doi:10.1016/j.apenergy.2019.02.070
Roy, S., Baldi, S., and Fridman, L. M. (2020). On adaptive sliding mode control without a priori bounded uncertainty. Automatica 111, 108650. doi:10.1016/j.automatica.2019.108650
Sabri, Y., El Kamoun, N., and Lakrami, F. (2019). “A survey: Centralized, decentralized, and distributed control scheme in smart grid systems,” in 2019 7th mediterranean congress of telecommunications (CMT) (IEEE), 1–11.
Sahoo, B., Routray, S. K., and Rout, P. K. (2021). AC, DC, and hybrid control strategies for smart microgrid application: A review. Int. Trans. Electr. Energy Syst. 31 (1), e12683. doi:10.1002/2050-7038.12683
Sahri, Y., Belkhier, Y., Tamalouzt, S., Ullah, N., Shaw, R. N., Chowdhury, M. S., et al. (2021). Energy management system for hybrid PV/wind/battery/fuel cell in microgrid-based hydrogen and economical hybrid battery/super capacitor energy storage. Energies 14 (18), 5722. doi:10.3390/en14185722
Sedhom, B. E., El-Saadawi, M. M., El Moursi, M. S., Hassan, M. A., and Eladl, A. A. (2021). IoT-based optimal demand-side management and control scheme for smart microgrid. Int. J. Electr. Power & Energy Syst. 127, 106674. doi:10.1016/j.ijepes.2020.106674
Sen, S., and Kumar, V. (2018). Microgrid control: A comprehensive survey. Annu. Rev. control 45, 118–151. doi:10.1016/j.arcontrol.2018.04.012
Siddique, A. B., Munsi, M. S., Sarker, S. K., Das, S. K., and Islam, M. R. (2019). Voltage and current control augmentation of islanded microgrid using multifunction model reference modified adaptive PID controller. Int. J. Electr. Power & Energy Syst. 113, 492–501. doi:10.1016/j.ijepes.2019.05.065
Singh, K., Amir, M., Ahmad, F., and Khan, M. A. (2020). An integral tilt derivative control strategy for frequency control in multimicrogrid system. IEEE Syst. J. 15 (1), 1477–1488. doi:10.1109/jsyst.2020.2991634
Singh, K., Amir, M., Ahmad, F., and Refaat, S. S. (2021). Enhancement of frequency control for stand-alone multi-microgrids. IEEE Access 9, 79128–79142. doi:10.1109/access.2021.3083960
Singh, S. K., Matwankar, C. S., Jee, M., and Alam, A. (2022). MRAS-based current estimator for DC-DC converters considering time-variant load impedance. J. Power Electron. 22 (2), 210–221. doi:10.1007/s43236-021-00353-2
Soliman, M. S., Belkhier, Y., Ullah, N., Achour, A., Alharbi, Y. M., Al Alahmadi, A. A., et al. (2021). Supervisory energy management of a hybrid battery/PV/tidal/wind sources integrated in DC-microgrid energy storage system. Energy Rep. 7, 7728–7740. doi:10.1016/j.egyr.2021.11.056
Su, W., and Wang, J. (2012). Energy management systems in microgrid operations. Electr. J. 25 (8), 45–60. doi:10.1016/j.tej.2012.09.010
Sylcloud Smart Micro Grid Sylcloud smart micro grid. Available at: https://sylcloud.com/smartmicrogrid (Accessed March 26 2022).
Tayab, U. B., Roslan, M. A. B., Hwai, L. J., and Kashif, M. (2017). A review of droop control techniques for microgrid. Renew. Sustain. Energy Rev. 76, 717–727. doi:10.1016/j.rser.2017.03.028
Umuhoza, J., Zhang, Y., Zhao, S., and Mantooth, H. A. (2017). “An adaptive control strategy for power balance and the intermittency mitigation in battery-PV energy system at residential DC microgrid level,” in 2017 IEEE applied power electronics conference and exposition (APEC) (IEEE), 1341–1345.
Vaikund, H., and Srivani, S. G. (2021). Trends inEMSfor smart microgrid—An overview. Adv. Signal Data Process., 15–28.
Vermesan, O., Friess, P., Guillemin, P., Gusmeroli, S., Sundmaeker, H., Bassi, A., and Doody, P. (2011). IoTstrategic research roadmap. Internet things-global Technol. Soc. trends 1, 9–52.
Völker, B., Reinhardt, A., Faustine, A., and Pereira, L. (2021). Watt’s up at home? Smart meter data analytics from a consumer-centric perspective. Energies 14 (3), 719. doi:10.3390/en14030719
Vuddanti, S., and Salkuti, S. R. (2021). Review of energy management system approaches in microgrids. Energies 14 (17), 5459. doi:10.3390/en14175459
Zahraoui, Y., Alhamrouni, I., Mekhilef, S., Basir Khan, M. R., Seyedmahmoudian, M., Stojcevski, A., et al. (2021). Energy management system in microgrids: A comprehensive review. Sustainability 13 (19), 10492. doi:10.3390/su131910492
Zamfir, M., Florian, V., Stanciu, A., Neagu, G., Preda, Ş., and Militaru, G. (2016). “Towards a platform for prototyping IoT health monitoring services,” in International conference on exploring services science (Cham: Springer), 522–533.
Zheng, J., Gao, D. W., and Lin, L. (2013). “Smart meters in smart grid: An overview,” in 2013 IEEE green technologies conference (GreenTech) (IEEE), 57–64.
Zhuang, J., Shen, G., Yu, J., Xiang, T., and Wang, X. (2017). The design and implementation of intelligent microgrid monitoring system based on WEB. Procedia Comput. Sci. 107, 4–8. doi:10.1016/j.procs.2017.03.047
Zia, M. F., Elbouchikhi, E., and Benbouzid, M. (2018). Microgrids energy management systems: A critical review on methods, solutions, and prospects. Appl. energy 222, 1033–1055. doi:10.1016/j.apenergy.2018.04.103
Nomenclature
AMI Advanced metering infrastructure
ANN Artificial neural network
AMR Automated Meter Reading
CANADA Supervisory control and data acquisition
CT Current transformer
DER Distributed energy resources
DG Distributed generators
DR Demand response
EMS Energy management system
ESS Energy storage system
ESS Energy Storage Systems
FL Fuzzy logic
GA Genetic algorithm
GHG Greenhouse gas
HMI Human machine interface
IoT Internet of things
LC Local controller
M2M Machine to machine
MAS Multiagent system
MG Microgrid
MGCC Microgrid central controller
MMG Multi-microGrid
MQTT Message queuing telemetry transport
PID Proportional–integral–derivative
PLC Programmable logic controller
PQ Power quality
PSO Particle swarm optimization
RER Renewable energy resource
RMU Remote monitoring unit
RTU Remote terminal unit
SMG Smart grid
Keywords: microgrid, energy management system, control techniques, monitoring system, IoT
Citation: Albarakati AJ, Boujoudar Y, Azeroual M, Eliysaouy L, Kotb H, Aljarbouh A, Khalid Alkahtani H, Mostafa SM, Tassaddiq A and Pupkov A (2022) Microgrid energy management and monitoring systems: A comprehensive review. Front. Energy Res. 10:1097858. doi: 10.3389/fenrg.2022.1097858
Received: 14 November 2022; Accepted: 12 December 2022;
Published: 28 December 2022.
Edited by:
Salah Kamel, Aswan University, EgyptReviewed by:
Youcef Belkhier, Maynooth University, IrelandMohammad Ghiasi, University of Regina, Canada
Copyright © 2022 Albarakati, Boujoudar, Azeroual, Eliysaouy, Kotb, Aljarbouh, Khalid Alkahtani, Mostafa, Tassaddiq and Pupkov. This is an open-access article distributed under the terms of the Creative Commons Attribution License (CC BY). The use, distribution or reproduction in other forums is permitted, provided the original author(s) and the copyright owner(s) are credited and that the original publication in this journal is cited, in accordance with accepted academic practice. No use, distribution or reproduction is permitted which does not comply with these terms.
*Correspondence: Younes Boujoudar, eW91bmVzLmJvdWpvdWRhckB1c21iYS5hYy5tYQ==; Hossam Kotb, aG9zc2FtLmtvdGJAYWxleHUuZWR1LmVn