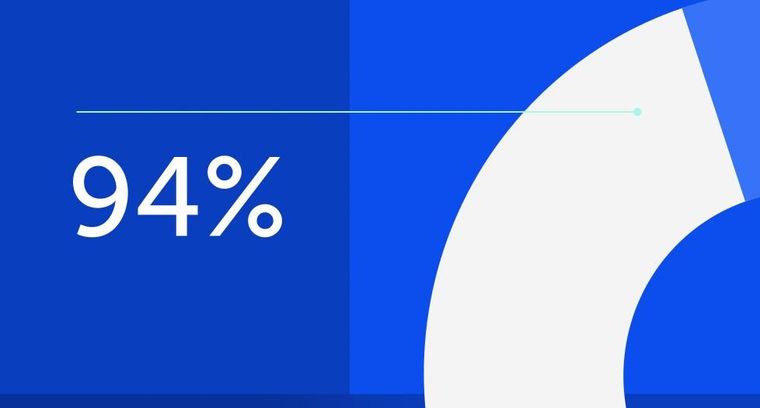
94% of researchers rate our articles as excellent or good
Learn more about the work of our research integrity team to safeguard the quality of each article we publish.
Find out more
REVIEW article
Front. Energy Res., 17 January 2023
Sec. Smart Grids
Volume 10 - 2022 | https://doi.org/10.3389/fenrg.2022.1054597
This article is part of the Research TopicTransactive Energy and Blockchain Approaches to Integrate Distributed Energy ResourcesView all 5 articles
The traditional power generation mix and the geographical distribution of units have faced structural reform with the increasing renewables. The existing scheduling schemes confront the optimization challenges of multi-source collaborative and multi-temporal coordination. This paper reviews the optimization of generation scheduling in power systems with renewables integration in different time scales, which are medium- and long-term, short-term and real-time, respectively. First, the scheduling model and method are summarized. The connections and differences of the multi-source mathematic model with uncertainty, as well as the market mechanism, including thermal power, hydroelectric power, wind power, solar energy, and energy storage, are also indicated. Second, the scheduling algorithm and approach are sorted out from the two dimensions of certainty and uncertainty. The innovation and difference in algorithm between the traditional scheduling and the scheduling problem with renewables are presented. Meanwhile, the interaction and coupling relationship among the different time scales are pointed out in each section. The challenges and shortcomings of current research and references future directions are also provided for dispatchers.
The traditional power system in which thermal units account for the vast majority will face structural reform with the increasing penetration of renewables (Chen et al., 2021a). According to the “Renewable Energy Installed Capacity Statistics 2022” report released by the International Energy Agency, the world in 2021 added almost 257 GW of renewables, increasing the stock of renewable power by 9.1 per cent and contributing to an unprecedented 81 per cent of global power additions. Furthermore, renewables are predicted to reach around 40 per cent in total energy generation across all sectors by 2030. Thus, the original power balance will be changed due to the more complex generation mix and more dispersed geography distribution of power units (Wang et al., 2021). New challenges are proposed to be confronted for the traditional generation scheduling schemes.
However, existing generation scheduling schemes will experience shortfalls with the increasing proportion of renewables in the future. A comprehensive optimal solution under the new power system will be hard to be obtained, resulting in curtailment of wind and solar, frequent congestion of power flow, and even power outages. Two main reasons cause the problem. First, the output characteristics of various power generation entities require scheduling schemes to achieve multi-temporal coordinated optimization. The actual output of hydropower is often determined in medium- and long-term scheduling, while the output of wind power and solar energy can only be accurately considered in short-term and real-time scheduling. Second, higher requirements are put forward for the optimization and coordination of comprehensive energy including thermal power, hydroelectric power, wind power, solar energy, and energy storage (Tiwari et al., 2021). The optimization of generation scheduling is realized efficiently by the complementarity coordination of comprehensive energy.
Several overviews have been conducted on the optimization of generation scheduling (Boqiang and Chuanwen, 2009), (Howlader et al., 2019). On one hand, generation scheduling schemes have been extensively summarized. Reference (Boqiang and Chuanwen, 2009) reviewed the traditional economic dispatch method considering wind power integration. The optimization problem was reviewed based on unit commitment (UC) (Abdi, 2021), and the impact of the renewable energy injection system on UC was also analyzed (Abujarad et al., 2017). However, all the reviews only analyzed the scheduling optimization problem of a single time period in a fragmented manner, and cannot effectively integrate the methods in each part, revealing the correlation among different scheduling schemes. On the other hand, the multi-temporal generation models of power sources were summarized. Reference (Liu et al., 2022) summarized photovoltaic output models and prediction methods at multiple temporal and spatial scales. Wind power generation forecasting methods were reviewed in reference (Jiang et al., 2019). Energy storage applications in various scenarios were also summarized (Ai and Dong, 2015); (Howlader et al., 2019). However, such studies only analyzed the multi-temporal output of a single power source. The applications in scheduling optimization methods were barely summarized. Meanwhile, no existing literature comprehensively integrated all power sources including wind power, photovoltaics, hydropower, thermal power, and energy storage, revealing their optimized coordination methods.
This paper reviews the optimization of multi-temporal generating scheduling in power system with renewables integration. The traditional scheduling optimization scheme and the scheme based on the integrated energy dispatch are sorted out in detail from the perspective of multiple time scales, and the connections and differences between the two schemes are also indicated. In the context of the previous research, our paper provides the following contributions.
1) We summarize the traditional scheduling optimization methods and the methods in the new power system with renewables integration. The mathematic models of multiple power sources, including thermal power, hydroelectric power, wind power, solar energy, and energy storage, are also reviewed, revealing their inherent connections and differences with consideration of uncertainty.
2) We summarize the optimization of scheduling algorithm and approach from the two dimensions of certainty and uncertainty. The innovation and difference of algorithm between the traditional scheduling and the scheduling problem with injection of renewables are indicated.
3) We sort out the similarities and differences of scheduling schemes from the perspective of multiple time scales, which are medium- and long-term, short-term and real-time, respectively, and point out the coordination and relationship in scheduling optimization among three terms.
The structure of the paper is the following: Section 2 reviews the optimization of multi-temporal scheduling method with multiple power sources mathematic models. Section 3 reviews the optimization of multi-temporal scheduling algorithm and approach. The summary of current works and corresponding suggestions for future research are analyzed in Section 4. Conclusions are presented in Section 5.
In this section, common methods and recent developments of scheduling models in medium- and long-term, short-term and real-time scales are concluded in sequence, including mathematical optimization models for each power source as well. Then the interaction and coupling relationship among the different time scales are summarized. What’s more, market mechanism to promote renewables integration is also reviewed. The difference in time dimension such as the scheduling cycle, time resolution between multi-temporal dispatches, as well as the input data and the output results connecting these dispatches are presented in Table 1.
Medium and long-term power generation scheduling focus on system operation for several weeks, months or a year. Long-term scheduling is generally considered with investment planning and medium-term scheduling gives correction to short-term scheduling instructions based on long-term scheduling results (Flatabø et al., 1998). With the difference in system scale, spatial and temporal resolution, and optimization target, there exist multiple modelling methods with different degrees of accuracy and technological properties.
The model involves various generation sources and numerous operational constraints, which are simulated from months to a year with hourly resolution. These scheduling models are generally designed to be deterministic and linear for the purpose to reduce the computational burden, such as several representative European electricity system models―IDILES (Iterative Optimization of Investment in Large Energy Systems), JMM (Joint Market Model) and ELTRAMOD (Electricity Transshipment Model) (Misconel et al., 2022).
For large-scale systems, the total system costs, including operational costs, investment costs and environmental costs, are commonly used as the optimization targets (Schmid and Knopf, 2015), (Schinko et al., 2019). With high renewable penetration in future power system, how to model wind and solar generation more accurately is important for power balance. To obtain time series of potential wind and solar generation with an hourly resolution, meteorological reanalysis is a popular method in many studies. Liam et al. modelled long-term offshore wind generation by using ERA5 reanalysis, the results indicated that ERA5 (European Centre for Medium-Range Weather Forecasts Reanalysis v5) reanalysis can effectively reduce prediction error of capacity factor compared to MERRA2 (Modern-Era Retrospective Analysis for Research and Applications, version 2) reanalysis (Hayes et al., 2021). Luis et al. generated hourly time series of solar generation by ERA5 reanalysis and validate the result using actual data (Camargo and Schmidt, 2020). Moreover, flexible resources like fast responding thermal units, hydropower and energy storage are critical to reducing system costs with renewable penetration elevated (Yang et al., 2018). For thermal units, the traditional unit commitment model is inapplicable in long-term scheduling problems due to amounts of binary variables. Hence, Han et al. (2019) proposed a fast unit commitment model (FUC) to reconstruct traditional chronological constraints with a linear formulation based on unit grouping techniques, and linear relaxation was considered for the simplification in reference (Palmintier and Webster, 2015). Due to the highly non-convex and nonlinear structure in output characteristics (Taktak and D’Ambrosio, 2017), hydropower generation is generally linearized in these problems (Namely fixed hydraulic head and turbine efficiency) (Liu et al., 2019a). As for storage, charge-discharge characteristics are the main constraint for long-term scheduling which is commonly considered a linear model (Chen et al., 2021a). Besides, Sonja et al. introduced a novel system-states framework to optimize storage operation in medium- and long-term power scheduling (Wogrin et al., 2015) and combined it with Net-constraints in a subsequent study (Tejada-Arango et al., 2017).
The models in the previous section are aimed to minimize the total system costs, however, there exist many studies focusing on other specific optimization strategies, such as renewable curtailment, energy generation and output fluctuation. Scheduling for hybrid power system with reservoirs has gradually become a hot topic. Considering the impact of uncertainty caused by wind and solar power generation, such problems are generally presented as multi-objective and multi-stage optimization models with small-scale systems and daily or monthly resolution (Liu et al., 2019b), (Shen et al., 2020). In some hydro-dominated systems like Norway and Brazil power system, the optimization scheduling of hydropower is a critical component in power dispatch. Linear programming (LP), decomposition techniques and energy equivalent reservoir (ERR) methods have been proposed to model the scheduling of large-scale reservoirs (Brandao, 2010). Stochastic dynamic programming (SDP) and stochastic dual dynamic programming (SDDP) are available for systems with few reservoirs (Zambelli et al., 2006), (Helseth et al., 2017). Meanwhile, a novel econometric model based on water value model has been applied in the deregulation system for reservoir operation (Jahns et al., 2020).
From the aspect of the electricity market, reference (Yao et al., 2020) analyzed renewable energy integration in the Ningxia electricity market while the ancillary service market is yet to be established. The generation contract transfer trading (GCTT) is adopted to ensure the cost recovering of thermal generators. Reference (McPherson and Bryan, 2014) analyzes the new law aiming to promote wind energy by mandating long term power purchase tenders in Panama, as well as the power generation and system marginal cost.
The total optimization horizon for short-term scheduling ranges from 1 day to 1 week with an hourly interval. Unit commitment (UC) and Economic dispatch (ED) models are universally applied in power system to determine on-off states and production plans of units. Classical UC and ED model has been widely discussed in many reviews (Saravanan et al., 2013)- (Bhardwaj et al., 2012), while this paper focuses on the latest development in modelling techniques. Matija et al. compared four clustering methods in Dispa-SET UC model and their results indicated that the differences between the methods and the traditional UC model are acceptable (Pavičević et al., 2019). Luis et al. proposed a frequency-constrained stochastic UC model to efficiently schedule diverse frequency services (Badesa et al., 2019). Brito et al. developed seven piecewise linear generation models for hydro UC problems and compared the corresponding performance in Brazil’s hydro system (Brito et al., 2020). Furthermore, some studies improved traditional ED under the rapid development of distributed energy (Velasquez et al., 2019), (Jian et al., 2020) and combined ED with environmental dispatch (Razeghi et al., 2016).
Uncertainty is becoming an inevitable factor for short-term power scheduling with the increasing share of intermittent resources. As the main source of uncertainty, wind and solar power output should be built more carefully in short-term scheduling, instead of being considered as time series in medium- and long-term models. One of the ways is to approximate power output by probability distribution function which is based on historical data and large amounts of scenarios generated by Monte Carlo simulation (MCS) and other mathematical methods (Zakaria et al., 2020). Another way is to define the uncertainty set by a set of parameters, which includes all possible scenarios (Yi et al., 2018). The two ways mentioned above are universally applied in uncertainty methods such as stochastic optimization and robust optimization. Reference (Kuznia et al., 2013) presents a stochastic mixed integer programming model for a hybrid power system, which consists of thermal units, renewables, storage considering network constraints, for remote areas. Reference (Madaeni and Sioshansi, 2013) combines stochastic optimization and demand response to mitigate the uncertainty of wind power. Li et al. (2020) proposed a stochastic programming for power system with renewables integration. It reduces energy costs with electric heaters and effectively manages renewables with fluctuating output. The two-stage robust optimization solved by the column-and-constraint generation is first proposed in reference (Zeng and Zhao, 2013), which greatly improve the scheduling speed. Based on that, An and Zeng. (2014) further present the expanded robust unit commitment and the risk constrained robust unit commitment model. What’s more, to cope with the wind power penetration, the uncertain sets based on multi-band uncertainty set considering the temporal correlation (MBUSCTC) can be added to the modeling of multi-power units. The method can rigorously and realistically describe the output of wind power sources (Chen et al., 2020). Meanwhile, the outage probability of units and transmission lines can be considered in solving unit commitment problems, which effectively reduce the conservatism of traditional robust contingency constrained unit commitment (Chen et al., 2019).
Moreover, the influence of load uncertainty is reckless to ignore in short-term scheduling problems (Dey et al., 2020). Batteries, pumped-hydro and other energy storage techniques are generally used to deal with these uncertainties. Wai et al. analyzed the advantages and disadvantages of storage operation based on daily cycles and weekly cycles respectively, the results showed that storage operated in daily mode is more advisable for renewables intermittency (Ho et al., 2016). Toubeau et al. (2020) presented a two-stage mixed-integer linear programming (MILP) model by data-driven methodology to optimize the day-ahead dispatch of storage systems. In addition, the data-driven robust state estimation (DDSE) method through off-line learning and on-line matching can be used to simplify the calculation of network parameters and effectively improve the scheduling efficiency (Chen et al., 2021b). At the same time, to cope with the uncertainty of renewables, it is possible to further consider the data-driven PF (DDPF) method based on historical/simulated data that includes an offline learning stage and an online computing stage in scheduling optimization (Chen et al., 2021c). Furthermore, multi-type demand response resources are gradually becoming a new direction for tackling uncertainty (Baek and Shin, 2022). For short-term hydro scheduling, MILP is one of the most effective approaches to accurately model the operation of hydropower stations with head-dependent prohibited operation zones (Su et al., 2020), while the uncertainty of reservoir inflow data is commonly handled with the stochastic programming (SP) model (Fleten and Bjørdal, 2020).
From the aspect of the electricity market, in order to cope with the elevated renewable penetrations of wind power, reference (Liu and Xu, 2021) increases corporate profits by analyzing bidding regulations and imbalance pricing mechanism, thereby promoting renewable accommodation. Reference (Zhang et al., 2022a) further using the Stackelberg game model analyze the relationship between renewable energy generation companies (GENCOs) and the day-ahead electricity market. The renewable accommodation in the day-ahead market can be effectively promoted by adjusting the bidding strategy.
Real-time generation scheduling mainly focuses on intraday dispatch problems with 15 min, 5 min or shorter temporal resolution. Accurate prediction information is a critical input for reasonable results in real-time scheduling. Thus, an ultra-short-term forecast is necessary for the violent fluctuation of wind and solar power output. Time series regression models, machine learning techniques, and other approaches such as image-based methods and decomposition methods are practicable to obtain the forecast information of minutes and hours ahead (Tawn and Browell, 2022). Recently, the combination of different methods called hybrid prediction model has been widely used in renewable forecast (Wang et al., 2020). For dynamically updating real-time prediction information, rolling dispatch based on model prediction control (MPC) has been widely used in many studies. Zhang et al. (2021) proposed a multi-objective rolling dispatch model to handle the intermittency of renewable resources in the optimization of active distribution network scheduling. Sheng et al. (2021) developed a mixed-integer second-order cone model with rolling optimization to eliminate the impact of renewable fluctuation on transmission networks. Meanwhile, uncertainty methods such as distributionally robust optimization and stochastic optimization in real-time scheduling has some novel developments. Distributionally robust optimization (DRO) is used to ensure security constrained economic dispatch and assess operating cost expectations affected by renewable energy sources (RES) uncertainty (Lu et al., 2018). Reference (Yang and Wu, 2018) improves the conservativeness in real-time scheduling by the distributionally robust optimization, where the uncertainty of distributed generation output is characterized with the first-order and second-order moments. Overvoltage problems can be eliminated effectively through the combination of ED and corrective control strategies. Based on DRO, reference (Liu et al., 2021) further considers automatic generation control (AGC) in SCED, which reduces the total cost of power generation and frequency regulation. Lin et al. (2020) took a mean-tracking model into stochastic ED to get optimal dispatch solutions with minimal tracking errors. Morteza proposed a two-stage stochastic model to optimize the operation of a hybrid system with the target of maximizing the system owner’s profit (Li et al., 2019).
Real-time scheduling of energy storage is usually determined by markets price and uncertainties. Fang et al. (2018) developed a mean-variance optimization method for the scheduling of storage system, which modelled the uncertainty of day-ahead and real-time price with a Gaussian distribution and aimed at reducing revenue volatility. Gao et al. (2018) introduced a MILP model which considered the impact of battery wear-cost and demand uncertainty based on rolling dispatch. With an intensive coupling relationship between upstream and downstream reservoirs, hydropower system is rarely dispatched at intervals of less than a quarter of an hour. While MILP is the universal approach to model the operation of hydropower system in real-time scheduling (Zhang et al., 2022b).
From the aspect of the electricity market, an integrated dynamic market mechanism (DMM) was proposed combining real-time market and frequency regulation. Renewable generators and flexible consumers can negotiate electricity prices to maximize the profitability of unit output (Shiltz et al., 2015). Reference (Yuan et al., 2021) further proposes a real-time pricing mechanism based on demand-response. The upper and lower models are aimed at maximizing the profit and welfare of suppliers respectively. The scheduling results can effectively reduce the peak-to-valley difference and optimize the output data of each unit.
To sum up, we have sorted out the commonly used methods and recent developments of multi-temporal power generation scheduling models. Power scheduling is generally ordered from longer time scales to shorter time scales. For instance, medium- and long-term model determines the annual scheduling plan for large reservoirs and takes it as the boundary of short-term model, while units on/off states are obtained from the output of short-term model and then directly applied in real-time model. The scheduling models of multiple time scales are closely coupled, where they have commonalities but are different from each other. They are interdependent and complementary to realize the smooth and safe operation of the power system.
Here, we sort out common solution algorithms and approaches for various models at multiple time scales, which are medium- and long-term, short-term and real-time, respectively. Each part the mainstream algorithms based on deterministic schemes in traditional generation scheduling are introduced first. Then the improvement and optimization of the uncertainty algorithm for the renewables integration are presented. The approaches to coordinate with other time scales are introduced at last. We note that optimizers including GUROBI, CPLEX, MINOS, GSOMP and so on are widely used to address the optimization issues. These approaches will not be repeated here.
The power generation scheduling in the medium- and long-term scales mainly focuses on the output schemes of the hydropower system. Meanwhile, relatively less uncertainty will be considered for renewable sources due to the long time scales. Hence, the medium- and long-term scheduling algorithms are mainly deterministic.
Reference (Nolde et al., 2008) proposed a multistage stochastic programming formulation solved via Nested Benders Decomposition. Model predictive control was setup to obtain the solution during the solving program. Horizon length and shape of the event tree were identified to optimize the computational power, and improved the performance and robustness by adjusting parameters.
In terms of co-optimization with other time scales, considering the randomness brought by inflow and varying temperatures, risk management and portfolio analysis were studied to face uncertainties in the Norwegian hydro system (Flatabø et al., 1998). Reference (Xinli et al., 2011) proposed a mixed numerical integral algorithm to simulate dynamically in scheduling. Multi-temporal phenomena were also united and coordinated to minimize the cost.
The short-term generation scheduling algorithms further solve the optimization in the hydro-thermal system. Different from medium- and long-term scheduling, the algorithms in short-term scheduling need more randomness and robustness due to the uncertain impacts of wind and solar energy. Hence, the short-term algorithms are innovated to adapt to the uncertainty due to the injection of renewables.
Reference (Ma et al., 2017) proposed a method to enhance the ability to accommodate wind power based on reciprocal peak-regulation trading of inter-regional grids. The uncertainty of wind power in day-ahead scheduling was reduced by the information gap decision algorithm. Reference (Liaquat et al., 2020) took into account the effects of photovoltaic energy in traditional power system. Auto-Regressive Integrated Moving Average (ARIMA) model was applied to solar energy forecasting. Non-linear problems could be addressed effectively by the accelerated particle swarm optimization and the Rey algorithm.
Meanwhile, as the essential hub of multi-temporal scheduling, short-term strategies have more interaction with other time scales. Several researches have been conducted on interaction with medium- and long-term scheduling. Reference (Marwali and Shahidehpour, 2000) proposed a coordination approach based on Monte Carlo simulation. The behavior of power system and potential forced outage scenarios in long-term scheduling were submitted to short-term scheduling, making sure the short-term scheduling and network constraints were satisfied. Three approaches, which are the primal-information approach, the dual-information approach and the marginal resource-valuation functions approach, respectively, were proposed to coordinate the medium-term planning and short-term scheduling. Profitability was increased by the combination of different decision levels (Reneses et al., 2006).
Real-time scheduling algorithms demand higher precision in a shorter scheduling time period. Multiple power sources and inter-regional coordination are important issues that real-time scheduling algorithms need to address. Meanwhile, the greater randomness brought by renewables also puts forward higher requirements on the robustness of the algorithm.
First, deterministic algorithms have been reviewed. Traditionally, real-time scheduling based on security constrained economic dispatch (SCED) was solved by deterministic algorithms. Reference (Kang et al., 2018) proposed deferrable loads control scheduling algorithm, where the power resource utilization was improved. The difference between the total load curve and the supply curve was also reduced. Reference (Huang and Wang, 2007) proposed an approach to optimized real-time generation scheduling combining orthogonal least-squares (OLS) and enhanced particle swarm optimization (EPSO) algorithms. The three-layer network structure has been simplified. The fast response and precise scheduling could be obtained when the inputs of system load with the weight of cost were submitted.
Second, optimization algorithms for the uncertainty have been reviewed. The researches on real-time scheduling algorithms to improve robustness have also been conducted due to the higher uncertainty of real-time renewables. Reference (Patrinos et al., 2011) formulated a real-time optimal generation scheduling problem containing intermittent generation and storage energy system. Then a novel scenario-based stochastic model predictive control (SMPC) algorithm was proposed for the solution of the real-time scheduling problem. Reference (Wei et al., 2015) proposed the concept of real-time dispatch ability (RTDA) of power systems with variable energy resources. A polyhedral representation of RTDA was defined and an efficient Ad-CG algorithm generated its boundaries. Linear characteristics make the method easy to implement. A continuous-time modelling based robust unit commitment was presented to address beyond-the-resolution (BtR) wind power uncertainties (Zhou et al., 2021). The optimization scheduling problem was solved by the column-and-constraint generation algorithm in the function space. Then the results were recovered to algebraic space. Robustness was enhanced at the expense of economic costs. Uncertainties were further reduced by a two-level optimization structure by a policy iteration algorithm based on the Perturbed Markov decision process. The optimal real-time scheduling strategy combined with storage energy was also described (Huo et al., 2015).
In terms of co-optimization with other time scales, coordination strategies between real-time and short-term scales were also applied to improve the robustness of scheduling. Reference (Yu et al., 2018) proposed an improved two-stage robust optimization with recourse method considering the rolling trading of inner-day electricity market based on the analysis of time-varying prediction uncertainty of wind power. Day-ahead scheduling results had been combined with real-time scheduling to both reduce the reserve capacity and the imprecision.
To sum up, the optimization algorithm in medium- and long-term scheduling is mainly deterministic, which solves multi-level planning, and calculates revenue precisely. In short-term scheduling, algorithms with uncertain models are widely used, such as Benders decomposition algorithm, Monte Carlo algorithm and so on. The real-time scheduling algorithms are similar to the short-term ones. However, due to its short scheduling period, iteration algorithms such as SMPC are proposed innovatively. In addition, various integrated optimization solvers are applicable to all time scales.
We have sorted out the typical scheduling methods and their algorithms with consideration of uncertainty in multiple time scales as shown in Table 2. The optimization of main power source models is also listed in detail. The review results show that the existing multi-temporal generating scheduling will confront the challenges under elevated renewable penetrations in the future. A comprehensive optimal solution will be difficult to be obtained, resulting in insufficient accommodation of renewables, frequent congestion of power flow, and even power outages. Two main reasons cause the problem. First, it is hard to achieve a unified scheduling scheme under multiple time scales due to the different physical characteristics of each power source. The actual output of hydropower is often determined in medium- and long-term scheduling, while the output of wind power and solar energy can only be accurately considered in short-term and real-time scheduling. Second, higher requirements are put forward for the optimization and coordination of comprehensive energy under elevated renewable penetrations. In order to ensure the balance of electricity, the comprehensive power abandonment rate of renewables will be very small, where some places require the full accommodation.
Meanwhile, we further found that the existing researches have the following shortcomings through the above review. In terms of scheduling models, no practical modelling method was proposed to ensure computing efficiency for large-scale systems in medium- and long-term scheduling when taking into account the uncertainty of renewable. Meanwhile, the participation of hydroelectric units, especially pumped storage plants, and energy storage were hard to be considered in real-time scheduling. From the perspective of scheduling algorithm and approach, the lack of effective algorithms to efficiently solve the medium- and long-term scheduling problem with consideration of renewables is also worth studying. Furthermore, current studies mainly focus on the coupling relationship between short-term and real-time scheduling models while few studies are concerned about the linkage between medium- and long-term and short-term scheduling. The above problems are demanded to be addressed in future research.
Therefore, future researches can focus on the following directions:
1. The medium- and long-term scheduling optimization problem in large-scale systems considering the hydro-thermal system should be investigated, where the large-scale cascade hydropower can be mainly considered. In particular, the optimization of output curves of various hydropower stations considering constraints such as maintenance plans is worthy of further study.
2. The optimization of scheduling strategies from medium- and long-term to short-term is worth studying. The coordinated operation of hydro-thermal and renewables can effectively improve the accommodation. Meanwhile, the connection of dispatch results including constraints and generator output between medium- and long-term dispatching and short-term dispatch is the focus of research.
3. The multi-source optimization model and cooperating strategy in real-time scheduling demand further improvement. The participation of pumped hydro and energy storage is an essential issue to be addressed. In addition, researchers can conduct the research on electricity market mechanism to address the problem of uncertainty under elevated renewables penetrations considering network constraints.
4. The trading mechanism of the electricity market can be further improved. The mechanism design of agency power purchase in the medium- and long-term scale, the renewables bidding strategy and the clearing model in the spot market are all worth studying.
The original power generation mix and the geographical distribution of units have changed with the increasing transition of power generation structure from fossil energy to renewable energy. Therefore, traditional optimization of scheduling methods based on the deterministic model has experienced shortfalls, while coordinated operation of hybrid system considering various uncertainties has gradually become concerned. This paper first introduces generation scheduling models and the latest improvement of mathematical modelling methods systematically, including wind power, photovoltaic, hydropower, thermal units and energy storage, from the perspective of multiple time scales. The coupling relationship and coordinated operation mechanism in different scheduling time scales are also indicated. The scheduling algorithms and approaches to problems at different time scales are reviewed then. The deterministic algorithms and the improved optimization algorithms for the uncertainty brought by renewables are introduced in detail. The medium- and long-term to short-term, short-term to real-time scheduling coupling algorithms and approaches are summarized as well. Furthermore, this review also points out the shortcomings of current research on large-scale systems in medium and long-term scheduling, as well as the lack of a strategic mechanism for the coordination between medium and long-term and short-term scheduling. Three references are presented for future research directions.
This review summarizes detailed multi-temporal generation scheduling schemes and corresponding algorithms with the purpose of providing assistance for dispatchers. The basis can be referenced for corresponding scheduling to face the increasing proportion of renewables injection.
KC, QS, BZ, QY, HP, and TJ: Data analyze, writing–original draft preparation SL: Supervision and editing.
The Reserve Talents Program for Middle-aged and Young Leaders of Disciplines in Science and Technology of Yunnan Province, China (Grant No. 202105AC160014). This work is supported by the Science and Technology Program of Yunnan Power Grid Co., Ltd. (Grant No.YNKJXM20210100).
KC, QS, BZ, and SL were empolyed by the System Operation Department, Yunnan Power Grid Co., Ltd.
The remaining authors declare that the research was conducted in the absence of any commercial or financial relationships that could be construed as a potential conflict of interest.
The authors declare that this study received funding from the Science and Technology Program of Yunnan Power Grid Co., Ltd. The funder had the following involvement in the study: data analyze, writing-original draft preparation, supervision and editing.
All claims expressed in this article are solely those of the authors and do not necessarily represent those of their affiliated organizations, or those of the publisher, the editors and the reviewers. Any product that may be evaluated in this article, or claim that may be made by its manufacturer, is not guaranteed or endorsed by the publisher.
Abdi, H. (2021). Profit-based unit commitment problem: A review of models, methods, challenges, and future directions. Renew. Sustain. Energy Rev. 138, 110504. doi:10.1016/j.rser.2020.110504
Abujarad, S. Y., Mustafa, M. W., and Jamian, J. J. (2017). Recent approaches of unit commitment in the presence of intermittent renewable energy resources: A review. Renew. Sustain. Energy Rev. 70, 215–223. doi:10.1016/j.rser.2016.11.246
Ai, X., and Dong, C. F. (2015). Review on the application of energy storage technology in power system with renewable energy sources. Mod. Electr. power 32 (5), 1–9.
An, Y., and Zeng, B. (2014). Exploring the modeling capacity of two-stage robust optimization: Variants of robust unit commitment model. IEEE Trans. Power Syst. 301, 109–122. doi:10.1109/tpwrs.2014.2320880
Badesa, L., Teng, F., and Strbac, G. (2019). Simultaneous scheduling of multiple frequency services in stochastic unit commitment. IEEE Trans. Power Syst. 34 (5), 3858–3868. doi:10.1109/tpwrs.2019.2905037
Baek, M. K., and Shin, B. D. (2022). Hybrid operation strategy for demand response resources and energy storage system. J. Electr. Eng. Technol. 17 (1), 25–37. doi:10.1007/s42835-021-00848-9
Bhardwaj, A., Kamboj, V. K., Shukla, V. K., Singh, B., and Khurana, P. (2012). “Unit commitment in electrical power system-a literature review,” in 2012 IEEE international power engineering and optimization conference Melaka (Malaysia: IEEE), 275–280.
Boqiang, R., and Chuanwen, J. (2009). A review on the economic dispatch and risk management considering wind power in the power market. Renew. Sustain. Energy Rev. 13 (8), 2169–2174. doi:10.1016/j.rser.2009.01.013
Brandao, J. L. B. (2010). Performance of the equivalent reservoir modelling technique for multi-reservoir hydropower systems. Water Resour. manage. 24 (12), 3101–3114. doi:10.1007/s11269-010-9597-9
Brito, B. H., Finardi, E. C., and Takigawa, F. Y. K. (2020). Mixed-integer nonseparable piecewise linear models for the hydropower production function in the unit commitment problem. Electr. Power Syst. Res. 182, 106234. doi:10.1016/j.epsr.2020.106234
Camargo, L. R., and Schmidt, J. (2020). Simulation of multi-annual time series of solar photovoltaic power: Is the ERA5-land reanalysis the next big step? Sustain. Energy Technol. Assessments 42, 100829. doi:10.1016/j.seta.2020.100829
Chen, X., Liu, Y., Wang, Q., Lv, J., Wen, J., Chen, X., et al. (2021). Pathway toward carbon-neutral electrical systems in China by mid-century with negative CO2 abatement costs informed by high-resolution modeling. Joule 5 (10), 2715–2741. doi:10.1016/j.joule.2021.10.006
Chen, X., Lv, J., McElroy, M. B., Han, X., Nielsen, C. P., and Wen, J. (2018). Power system capacity expansion under higher penetration of renewables considering flexibility constraints and low carbon policies. IEEE Trans. Power Syst. 33 (6), 6240–6253. doi:10.1109/tpwrs.2018.2827003
Chen, Y., Chen, H., Jiao, Y., Ma, J., and Lin, Y. (2021). Data-driven robust state estimation through off-line learning and on-line matching. J. Mod. Power Syst. Clean Energy 94, 897–909. doi:10.35833/mpce.2020.000835
Chen, Y., Wu, C., and Qi, J. (2021). Data-driven power flow method based on exact linear regression equations. J. Mod. Power Syst. Clean Energy 3, 800–804. doi:10.35833/mpce.2020.000738
Chen, Y., Zhang, Z., Chen, H., and Zheng, H. (2020). Robust UC model based on multi-band uncertainty set considering the temporal correlation of wind/load prediction errors. IET Gener. Transm. &. Distrib. 142, 180–190. doi:10.1049/iet-gtd.2019.1439
Chen, Y., Zhang, Z., Liu, Z., Zhang, P., Ding, Q., Liu, X., et al. (2019). Robust N–k CCUC model considering the fault outage probability of units and transmission lines. IET Gener. Transm. &. Distrib. 1317, 3782–3791. doi:10.1049/iet-gtd.2019.0780
Dey, B., Bhattacharyya, B., Raj, S., and Babu, R. (2020). Economic emission dispatch on unit commitment-based microgrid system considering wind and load uncertainty using hybrid MGWOSCACSA. J. Electr. Syst. Inf. Technol. 7 (1), 15–26. doi:10.1186/s43067-020-00023-6
Fang, X., Hodge, B. M., Bai, L., Cui, H., and Li, F. (2018). Mean-variance optimization-based energy storage scheduling considering day-ahead and real-time LMP uncertainties. IEEE Trans. Power Syst. 33 (6), 7292–7295. doi:10.1109/tpwrs.2018.2852951
Flatabø, N., Haugstad, A., Mo, B., and Fosso, O. B. (1998). “Short-term and medium-term generation scheduling in the Norwegian hydro system under a competitive power market structure,” in EPSOM’98 (International Conference on Electrical Power System Operation and Management) (Switzerland.
Fleten, K. K., and Bjørdal, M. F. (2020). The value of considering uncertainty in short-term hydropower scheduling–combining machine learning and stochastic programming (Master's thesis, NTNU).
Gao, H. C., Choi, J. H., Yun, S. Y., Lee, H. J., and Ahn, S. J. (2018). Optimal scheduling and real-time control schemes of battery energy storage system for microgrids considering contract demand and forecast uncertainty. Energies 11 (6), 1371. doi:10.3390/en11061371
Han, X., Chen, X., McElroy, M. B., Liao, S., Nielsen, C. P., and Wen, J. (2019). Modeling formulation and validation for accelerated simulation and flexibility assessment on large scale power systems under higher renewable penetrations. Appl. energy 237, 145–154. doi:10.1016/j.apenergy.2018.12.047
Hayes, L., Stocks, M., and Blakers, A. (2021). Accurate long-term power generation model for offshore wind farms in Europe using ERA5 reanalysis. Energy 229, 120603. doi:10.1016/j.energy.2021.120603
Helseth, A., Fodstad, M., Askeland, M., Mo, B., Nilsen, O. B., Pérez-Díaz, J. I., et al. (2017). Assessing hydropower operational profitability considering energy and reserve markets. IET Renew. power Gener. 11 (13), 1640–1647. doi:10.1049/iet-rpg.2017.0407
Ho, W. S., Macchietto, S., Lim, J. S., Hashim, H., Muis, Z. A., and Liu, W. H. (2016). Optimal scheduling of energy storage for renewable energy distributed energy generation system. Renew. Sustain. Energy Rev. 58, 1100–1107. doi:10.1016/j.rser.2015.12.097
Howlader, H. O. R., Adewuyi, O. B., Hong, Y. Y., Mandal, P., Mohamed Hemeida, A., and Senjyu, T. (2019). Energy Storage System Analysis Review for Optimal Unit Commitment. Energies (Basel). 13 (1), 158. doi:10.3390/en13010158
Huang, C. M., and Wang, F. L. (2007). An RBF network with OLS and EPSO algorithms for real-time power dispatch. IEEE Trans. Power Syst. 22 (1), 96–104. doi:10.1109/tpwrs.2006.889133
Huo, Y., Jiang, P., Zhu, Y., Feng, S., and Wu, X. (2015). Optimal real-time scheduling of wind integrated power system presented with storage and wind forecast uncertainties. Energies 8 (2), 1080–1100. doi:10.3390/en8021080
Jahns, C., Podewski, C., and Weber, C. (2020). Supply curves for hydro reservoirs–Estimation and usage in large-scale electricity market models. Energy Econ. 87, 104696. doi:10.1016/j.eneco.2020.104696
Jian, L., Qian, Z., Liangang, Z., and Mengkai, Y. (2020). Distributed economic dispatch method for power system based on consensus. IET Renew. Power Gener. 14 (9), 1424–1432. doi:10.1049/iet-rpg.2019.1085
Jiang, Z. Y., Jia, Q. S., and Guan, X. H. (2019). A review of multi-temporal-and-spatial-scale wind power forecasting method. Acta Autom. Sin. 45 (1), 51–71. doi:10.16383/j.aas.c180389
Kang, M., Wen, C., and Wu, C. (2018). A model predictive scheduling algorithm in real-time control systems. IEEE/CAA J. Autom. Sin. 5 (2), 471–478. doi:10.1109/jas.2017.7510826
Kuznia, L., Zeng, B., Centeno, G., and Miao, Z. (2013). Stochastic optimization for power system configuration with renewable energy in remote areas. Ann. Oper. Res. 2101, 411–432. doi:10.1007/s10479-012-1110-9
Li, R., Guo, S., Yang, Y., and Liu, D. (2020). Optimal sizing of wind/concentrated solar plant/electric heater hybrid renewable energy system based on two-stage stochastic programming. Energy 209, 118472. doi:10.1016/j.energy.2020.118472
Li, Y., Miao, S., Yin, B., Yang, W., Zhang, S., Luo, X., et al. (2019). A real-time dispatch model of CAES with considering the part-load characteristics and the power regulation uncertainty. Int. J. Electr. Power & Energy Syst. 105, 179–190. doi:10.1016/j.ijepes.2018.08.024
Liaquat, S., Fakhar, M. S., Kashif, S. A. R., Rasool, A., Saleem, O., and Padmanaban, S. (2020). Performance analysis of APSO and firefly algorithm for short term optimal scheduling of multi-generation hybrid energy system. IEEE Access 8, 177549–177569. doi:10.1109/access.2020.3027436
Lin, Z., Chen, H., Wu, Q., Li, W., Li, M., and Ji, T. (2020). Mean-tracking model based stochastic economic dispatch for power systems with high penetration of wind power. Energy 193, 116826. doi:10.1016/j.energy.2019.116826
Liu, C., Li, M., Yu, Y., Wu, Z., Gong, H., and Cheng, F. (2022). A review of multi-temporal and multi-spatial scales photovoltaic forecasting methods. IEEE Access.
Liu, H., Andresen, G. B., Brown, T., and Greiner, M. (2019). A high-resolution hydro power time-series model for energy systems analysis: Validated with Chinese hydro reservoirs. MethodsX 6, 1370–1378. doi:10.1016/j.mex.2019.05.024
Liu, L., Hu, Z., Duan, X., and Pathak, N. (2021). Data-driven distributionally robust optimization for real-time economic dispatch considering secondary frequency regulation cost. IEEE Trans. Power Syst. 365, 4172–4184. doi:10.1109/tpwrs.2021.3056390
Liu, T., and Xu, J. (2021). Equilibrium strategy based policy shifts towards the integration of wind power in spot electricity markets: A perspective from China. Energy Policy 157 (2021), 112482. doi:10.1016/j.enpol.2021.112482
Liu, Z., Zhang, Z., Zhuo, R., and Wang, X. (2019). Optimal operation of independent regional power grid with multiple wind-solar-hydro-battery power. Appl. energy 235, 1541–1550. doi:10.1016/j.apenergy.2018.11.072
Lu, Xi, Chan, K. W., Xia, S., Zhou, B., and Luo, X. (2018). Security-constrained multiperiod economic dispatch with renewable energy utilizing distributionally robust optimization. IEEE Trans. Sustain. Energy 102, 768–779. doi:10.1109/tste.2018.2847419
Ma, H., Han, X., Yan, Z., and Song, Y. (2017). Robustness Driving Reciprocal Peak regulation Trading Method of Inter-regional Grids Containing Wind Power Uncertainty. Automation Electr. Power Syst. 41 (07), 28–36.
Madaeni, S. H., and Sioshansi, R. (2013). The impacts of stochastic programming and demand response on wind integration. Energy Syst. 42, 109–124. doi:10.1007/s12667-012-0068-7
Marwali, M. K. C., and Shahidehpour, S. M. (2000). Coordination between long-term and short-term generation scheduling with network constraints. IEEE Trans. Power Syst. 15 (3), 1161–1167. doi:10.1109/59.871749
McPherson, M., and Bryan, K. (2014). Long-term scenario alternatives and their implications: LEAP model application of Panama׳ s electricity sector. Energy Policy 68, 146–157. doi:10.1016/j.enpol.2014.01.028
Misconel, S., Leisen, R., Mikurda, J., Zimmermann, F., Fraunholz, C., Fichtner, W., et al. (2022). Systematic comparison of high-resolution electricity system modeling approaches focusing on investment, dispatch and generation adequacy. Renew. Sustain. Energy Rev. 153, 111785. doi:10.1016/j.rser.2021.111785
Nolde, K., Uhr, M., and Morari, M. (2008). Medium term scheduling of a hydro-thermal system using stochastic model predictive control. Automatica 44 (6), 1585–1594. doi:10.1016/j.automatica.2008.03.002
Palmintier, B. S., and Webster, M. D. (2015). Impact of operational flexibility on electricity generation planning with renewable and carbon targets. IEEE Trans. Sustain. Energy 7 (2), 672–684. doi:10.1109/tste.2015.2498640
Patrinos, P., Trimboli, S., and Bemporad, A. (2011). “Stochastic MPC for real-time market-based optimal power dispatch,” in 2011 50th IEEE Conference on Decision and Control and European Control Conference (IEEE), 7111–7116.
Pavičević, M., Kavvadias, K., Pukšec, T., and Quoilin, S. (2019). Comparison of different model formulations for modelling future power systems with high shares of renewables–The Dispa-SET Balkans model. Appl. Energy 252, 113425. doi:10.1016/j.apenergy.2019.113425
Razeghi, G., Brouwer, J., and Samuelsen, S. (2016). A spatially and temporally resolved model of the electricity grid–Economic vs environmental dispatch. Appl. Energy 178, 540–556. doi:10.1016/j.apenergy.2016.06.066
Reneses, J., Centeno, E., and Barquin, J. (2006). Coordination between medium-term generation planning and short-term operation in electricity markets. IEEE Trans. Power Syst. 21 (1), 43–52. doi:10.1109/tpwrs.2005.857851
Saravanan, B., Das, S., Sikri, S., and Kothari, D. P. (2013). A solution to the unit commitment problem—a review. Front. Energy 7 (2), 223–236. doi:10.1007/s11708-013-0240-3
Schinko, T., Bohm, S., Komendantova, N., Jamea, E. M., and Blohm, M. (2019). Morocco’s sustainable energy transition and the role of financing costs: a participatory electricity system modeling approach. Energy sustain. Soc. 9 (1), 1–17. doi:10.1186/s13705-018-0186-8
Schmid, E., and Knopf, B. (2015). Quantifying the long-term economic benefits of European electricity system integration. Energy Policy 87, 260–269. doi:10.1016/j.enpol.2015.09.026
Shen, J., Cheng, C., Wang, S., Yuan, X., Sun, L., and Zhang, J. (2020). Multiobjective optimal operations for an interprovincial hydropower system considering peak-shaving demands. Renew. Sustain. Energy Rev. 120, 109617. doi:10.1016/j.rser.2019.109617
Sheng, H., Wang, C., and Liang, J. (2021). Multi-timescale active distribution network optimal scheduling considering temporal-spatial reserve coordination. Int. J. Electr. Power & Energy Syst. 125, 106526. doi:10.1016/j.ijepes.2020.106526
Shiltz, D. J., Cvetković, M., and Annaswamy, A. M. (2015). An integrated dynamic market mechanism for real-time markets and frequency regulation. IEEE Trans. Sustain. Energy 7 (2), 875–885. doi:10.1109/tste.2015.2498545
Su, C., Yuan, W., Cheng, C., Wang, P., Sun, L., and Zhang, T. (2020). Short-term generation scheduling of cascade hydropower plants with strong hydraulic coupling and head-dependent prohibited operating zones. J. Hydrology 591, 125556. doi:10.1016/j.jhydrol.2020.125556
Taktak, R., and D’Ambrosio, C. (2017). An overview on mathematical programming approaches for the deterministic unit commitment problem in hydro valleys. Energy Syst. 8 (1), 57–79. doi:10.1007/s12667-015-0189-x
Tawn, R., and Browell, J. (2022). A review of very short-term wind and solar power forecasting. Renew. Sustain. Energy Rev. 153, 111758. doi:10.1016/j.rser.2021.111758
Tejada-Arango, D. A., Wogrin, S., and Centeno, E. (2017). Representation of storage operations in network-constrained optimization models for medium-and long-term operation. IEEE Trans. Power Syst. 33 (1), 386–396. doi:10.1109/tpwrs.2017.2691359
Tiwari, S., Dwivedi, B., Dave, M. P., Shrivastava, A., Agrawal, A., and Bhadoria, V. S. (2021). Unit commitment problem in renewable integrated environment with storage: A review. Int. Trans. Electr. Energ. Syst. 31 (10), e12775. doi:10.1002/2050-7038.12775
Toubeau, J. F., Bottieau, J., De Grève, Z., Vallee, F., and Bruninx, K. (2020). Data-driven scheduling of energy storage in day-ahead energy and reserve markets with probabilistic guarantees on real-time delivery. IEEE Trans. Power Syst. 36 (4), 2815–2828. doi:10.1109/tpwrs.2020.3046710
Velasquez, M. A., Barreiro-Gomez, J., Quijano, N., Cadena, A. I., and Shahidehpour, M. (2019). Distributed model predictive control for economic dispatch of power systems with high penetration of renewable energy resources. Int. J. Electr. Power & Energy Syst. 113, 607–617. doi:10.1016/j.ijepes.2019.05.044
Wang, Y., Wang, D., and Tang, Y. (2020). Clustered hybrid wind power prediction model based on ARMA, PSO-SVM, and clustering methods. IEEE Access 8, 17071–17079. doi:10.1109/access.2020.2968390
Wang, Y., Zhu, T., Zhang, Y., and Chen, X. (2021). Preliminary Study on Capacity Compensation Mechanism Adapted to Development of Electricity Spot Market in China. Automation Electr. Power Syst. 45 (06), 52–61.
Wei, W., Liu, F., and Mei, S. (2015). Real-time dispatchability of bulk power systems with volatile renewable generations. IEEE Trans. Sustain. Energy 6 (3), 738–747. doi:10.1109/tste.2015.2413903
Wogrin, S., Galbally, D., and Reneses, J. (2015). Optimizing storage operations in medium-and long-term power system models. IEEE Trans. Power Syst. 31 (4), 3129–3138. doi:10.1109/tpwrs.2015.2471099
Xinli, S., Yong, T., Wuzhi, Z., Wenzhuo, L., Guoyang, W., and Tao, L. (2011). IEEE, 1–7.New mixed integral algorithm for unified dynamic power system simulations of transient, medium-term and long-term stabilities2011 IEEE/PES Power Systems Conference and Exposition
Yang, Y., and Wu, W. (2018). A distributionally robust optimization model for real-time power dispatch in distribution networks. IEEE Trans. Smart Grid 104, 3743–3752. doi:10.1109/tsg.2018.2834564
Yang, Y., Zhang, H., Xiong, W., Zhang, D., and Zhang, X. (2018). Regional power system modeling for evaluating renewable energy development and CO2 emissions reduction in China. Environ. Impact Assess. Rev. 73, 142–151. doi:10.1016/j.eiar.2018.08.006
Yao, Y., Gao, C., Tian, H., and Zhang, H. (2020). Review of mid-to long-term trading mechanism for renewable electricity consumption in Ningxia, China. Renew. Sustain. Energy Rev. 134, 110325. doi:10.1016/j.rser.2020.110325
Yi, W., Zhang, Y., Zhao, Z., and Huang, Y. (2018). Multiobjective robust scheduling for smart distribution grids: Considering renewable energy and demand response uncertainty. IEEE Access 6, 45715–45724. doi:10.1109/access.2018.2865598
Yu, J., Xingying, C., Kun, Y., Yingchen, L., and Jun, X. (2018). Robust optimization method for day-ahead generation scheduling considering prediction uncertainty of wind power. Automation Electr. Power Syst. 42 (19), 57–63.
Yuan, G., Gao, Y., and Ye, B. (2021). Optimal dispatching strategy and real-time pricing for multi-regional integrated energy systems based on demand response. Renew. Energy 179, 1424–1446. doi:10.1016/j.renene.2021.07.036
Zakaria, A., Ismail, F. B., Lipu, M. H., and Hannan, M. A. (2020). Uncertainty models for stochastic optimization in renewable energy applications. Renew. Energy 145, 1543–1571. doi:10.1016/j.renene.2019.07.081
Zambelli, M., Siqueira, T. G., Cicogna, M., and Soares, S. (2006). “Deterministic versus stochastic models for long term hydrothermal scheduling,” in 2006 IEEE Power Engineering Society General Meeting (IEEE), 7.
Zeng, B., and Zhao, L. (2013). Solving two-stage robust optimization problems using a column-and-constraint generation method. Operations Res. Lett. 415, 457–461. doi:10.1016/j.orl.2013.05.003
Zhang, J., Li, Z., and Wang, B. (2021). Within-day rolling optimal scheduling problem for active distribution networks by multi-objective evolutionary algorithm based on decomposition integrating with thought of simulated annealing. Energy 223, 120027. doi:10.1016/j.energy.2021.120027
Zhang, X., Guo, X., and Zhang, X. (2022). Bidding modes for renewable energy considering electricity-carbon integrated market mechanism based on multi-agent hybrid game. Energy 263, 125616. doi:10.1016/j.energy.2022.125616
Zhang, Z., Wu, X., Liao, S., and Cheng, C. (2022). An ultra-short-term scheduling model for cascade hydropower regulated by multilevel dispatch centers suppressing wind power volatility. Int. J. Electr. Power & Energy Syst. 134, 107467. doi:10.1016/j.ijepes.2021.107467
Keywords: optimization, generation scheduling, multi-temporal, renewables integration, power system
Citation: Chen K, Shao Q, Zhou B, Yang Q, Pan H, Jin T and Liu S (2023) Optimization of multi-temporal generation scheduling in power system under elevated renewable penetrations: A review. Front. Energy Res. 10:1054597. doi: 10.3389/fenrg.2022.1054597
Received: 27 September 2022; Accepted: 04 November 2022;
Published: 17 January 2023.
Edited by:
Yue Zhou, Cardiff University, United KingdomReviewed by:
Kaiping Qu, China University of Mining and Technology, ChinaCopyright © 2023 Chen, Shao, Zhou, Yang, Pan, Jin and Liu. This is an open-access article distributed under the terms of the Creative Commons Attribution License (CC BY). The use, distribution or reproduction in other forums is permitted, provided the original author(s) and the copyright owner(s) are credited and that the original publication in this journal is cited, in accordance with accepted academic practice. No use, distribution or reproduction is permitted which does not comply with these terms.
*Correspondence: Qiyu Yang, eWFuZ19xeTIwMjBAMTYzLmNvbQ==
Disclaimer: All claims expressed in this article are solely those of the authors and do not necessarily represent those of their affiliated organizations, or those of the publisher, the editors and the reviewers. Any product that may be evaluated in this article or claim that may be made by its manufacturer is not guaranteed or endorsed by the publisher.
Research integrity at Frontiers
Learn more about the work of our research integrity team to safeguard the quality of each article we publish.