- 1Carbon Solutions LLC, Okemos, MI, United States
- 2Great Plains Institute, Minneapolis, MN, United States
- 3School of Computing, Montana State University, Bozeman, MT, United States
Increasing the capacity of wind power is critical to achieving climate goals, however its continued deployment faces environmental and social siting challenges. For example, the United States government is increasingly emphasizing the importance of a just energy transition by considering the social impacts of energy and environmental justice (EEJ). In this study, we investigate the impact of considering available EEJ metrics and environmental impacts into siting wind power and transmission by applying SimWINDPRO. SimWINDPRO is an infrastructure optimization tool that can site wind energy technologies and transmission by concurrently considering wind resource potential, transmission costs, EEJ, and environmental impacts. We demonstrate the impacts of considering EEJ and environmental factors in the context of Midcontinent Independent System Operator’s (MISO) western region, which includes some of the best wind energy potential in the United States. We show that prioritizing EEJ and environmental considerations in wind deployment can result in exponentially more transmission deployment for the same amount of wind power delivered, and results in selecting different wind farm sites. Our results also show that, depending on how it is considered, it is possible that constraining sites based on EEJ and environmental factors can reduce the available capacity of wind energy enough that energy transition capacity targets cannot be met.
1 Introduction
1.1 Background
The installed capacity of wind energy needs to substantially increase to meet climate goals. The Integrated Assessment Models (IAMs) used by the Intergovernmental Panel on Climate Change (IPCC) rely on renewables, including wind energy, to provide 70–85% of electricity by 2050 to limit global warming to 1.5°C (IPCC, 2018). However, in 2019 only 26.2% of global electricity consumption was provided by wind and solar (IEA et al., 2022). In the United States, the National Renewable Energy Laboratory’s (NREL’s) most recent Standard Scenarios report suggests that meeting the Administration’s 2021 goal of creating a carbon-free electric power system by 2035 may require wind turbine capacities up to ∼400 GW in 2030 and ∼900 GW in 2050 (Cole et al., 2021). For reference, the capacity of wind power in the United States in 2020 was only ∼120 GW (Wiser et al., 2021). In addition to more capacity, the rate of wind turbine installation also needs to increase if the United States will become a net-zero emission economy by 2050. For example, in 2020, 16.8 GW/yr of wind was installed in the United States, which is the highest installation rate the country has ever achieved (Wiser et al., 2021). However, the Princeton Net Zero America Study suggests that the peak wind installation rate will need to increase to between 19 GW/yr and as much as 96 GW/yr to reach a net-zero economy by 2050 (Jenkins et al., 2021; Larson et al., 2021).
Historically, the major constraint for deploying wind energy was cost. As compared to other energy sources, wind turbines were expensive, affordable only in regions with the best wind resources, and then often required government incentives, such as the production tax credit in the United States. However, costs have exponentially declined over the past decade, and the 2021 Lazard Levelized Cost of Energy Analysis listed wind as the least expensive source of electricity on a levelized cost basis, with levelized costs as low as $26/MWh (Lazard, 2021). This cost is expected to continue to decline exponentially until 2050, and every year, the 2050 projected costs have also declined. For example, the projected 2050 cost of wind power in NREL’s Annual Technology Baseline (ATB) report has consistently decreased with updates: it was ∼$30/MWh in the 2015 ATB and ∼$10/MWh in the 2022 ATB (NREL (National Renewable Energy Laboratory), 2022). Given the decline in wind costs, the cost competitiveness of wind energy is arguably no longer the primary constraint limiting deployment. There are now three other factors related to siting that now must be considered at least as heavily, if not more so, than the cost of wind energy technologies when planning how to meet energy transition goals: 1) transmission infrastructure, 2) environmental impacts, and 3) energy and environmental justice (EEJ).
Transmission deployment is critical for wind development and although wind costs have decreased, transmission remains expensive. The best wind sites are often far away from the population centers that need the electricity (Lee et al., 2017), and current transmission networks are insufficient to access these resources (Caspary et al., 2021). For example, the 2021 ATB estimates that a 200 MW wind farm costs 287 million USD (1,436 USD/kW) (NREL (National Renewable Energy Laboratory), 2021) and MISO estimates that a 115 kV power line (capable of ∼300 MW) costs 1.69 million USD/mile (MISO, 2021b).
Environmental conflicts, including land use, make siting wind farms and transmission difficult because infrastructure can have negative impacts on natural resources and ecosystem biodiversity. For example, both NREL and the Nature Conservancy (TNC) have studied the potential of environmental factors and land use to impact siting wind turbines and have found substantial constraints to wind deployment in some locations of the country (Lopez et al., 2021; Harrison-Atlas et al., 2022; The Nature Conservancy, 2022).
The definition of environmental justice we consider in our work is “the distribution of environmental hazards and access to all natural resources; it includes equal protection from burdens, meaningful involvement in decisions, and fair treatment in access to benefits” (Jenkins, 2018). Many concepts in environmental justice are applicable to a wide range of sectors and issues; it can refer to toxic waste (Holifield, 2001), access to national parks (Byrne et al., 2009), and transportation planning (Duthie et al., 2007). While environmental justice refers to understanding the distribution of burdens of decisions and the importance of processes to increase fairness and transparency, it is often too broad to address the issues and trade-offs that are emerging as nations across the world aim to meet climate goals.
Energy justice has recently emerged as a term that extends environmental justice to include activities related to energy systems (Jenkins, 2018). This is in part to reflect the enormous policy, planning, and infrastructure changes that are occurring due to decarbonization of the energy sector. Sovocool and Dworkin characterize energy justice as a conceptual tool that integrates distributive and procedural justice often found in environmental justice work, an analytical tool to explore how values are reflected in the energy system, and as a decision-making tool that aids in energy choice planning (Sovacool and Dworkin, 2015). Aspects of environmental justice and energy justice are both important, and thus we consider them together as energy and environmental justice (EEJ).
The impacts of EEJ must be considered during the energy transition (Jenkins et al., 2018). As countries transition to a net-zero economy, the distributed nature of renewable energy technologies raises considerations of EEJ (Carley and Konisky, 2020) that, while largely absent in historic energy development, now plays an important role in siting energy infrastructure. For example, coal power plants have hazardous emissions such as mercury and lead and, in the United States, they have disproportionately been sited near poor and minority communities (Israel, 2012). Increased attention has come to EEJ, as highlighted by the Administration’s Justice40 initiative that requires 40% of the benefits from federal clean energy investments to flow to disadvantaged communities (Young et al., 2021).
1.2 Literature gap
While these three considerations—transmission infrastructure, environmental impacts, and EEJ—are distinct, they are not necessarily independent from one another. For example, it is possible that the wind resources which are most amenable to development from an environmental/natural resource or EEJ perspective could also be the resources that require the most transmission infrastructure to develop and therefore be too expensive. As a result, it is necessary to consider all three factors simultaneously along with cost when planning wind energy infrastructure. Despite this need, there are no studies we are aware of that robustly investigate how to site wind and transmission infrastructure to meet energy transition goals while considering environmental/natural resource and EEJ factors.
This literature gap exists largely because current tools, as shown in Table 1, were not developed to simultaneously consider all these factors. In contrast, existing energy planning tools are generally focused on optimizing for things other than siting or spatial planning, while the existing wind resource tools that consider spatial constraints are not optimization models and do not offer guidance on which wind resources are most amendable to develop to meet energy transition goals from perspectives beyond the quality of the wind resource (e.g., required transmission, EEJ). Here we briefly review the most related tools in the areas of EEJ, transmission planning, wind planning, and capacity expansion models.
Current EEJ tools and frameworks provide insight but are not well suited to support wind and transmission developers. Swennenhuis et al. used an analytical framework to compare justice indicators for transitioning the steel industry to a lower carbon intensity (Swennenhuis et al., 2022), but neither considers cost nor siting impacts. Heleno et al. present a decision-support framework using optimization that minimizes a population’s energy burden, but is focused on weatherization and energy efficiency (Heleno et al., 2022). Hanssen et al. developed the Consensus-based Siting (ConSite) tool that uses a multi-criteria decision analysis to site wind farms and transmission lines; however, it does not consider cost (Hanssen et al., 2018).
Transmission planning is often performed with specialized tools independent of wind siting. Transmission expansion models have been developed to help with the detailed planning of transmission lines including considerations such as power flow constraints (Hemmati et al., 2013), but they are not designed to assist with deciding between wind development regions.
Existing wind planning tools focus on cost and resource quality, but do not include transmission planning. The Department of Energy’s Energy Zones Mapping Tool enables users to examine geospatial information that could assist with siting, such as suitability for wind turbines, but is difficult to consider more than one layer of information at a time (Shahidehpour and Li, 2014). The State and Local Planning for Energy (SLOPE) tool developed by the National Renewable Energy Laboratory (NREL) provides a visualization platform for users to consider geospatial information such as energy generation potential and historic consumption at the state and county level (NREL (National Renewable Energy Laboratory)). NREL’s Renewable Energy Potential (reV) tool estimates the siting potential of wind farms based on resource potential while considering exclusion zones such as protected areas, but requires a high-performance computer to run (Maclaurin et al., 2019). The reV tool does not help site wind farms, instead it outputs supply curves that are then used in NREL’s capacity expansion model, ReEDS (Maclaurin et al., 2019).
Capacity expansion models help identify regions for wind development but are not well suited to investigate EEJ. Capacity expansion models such as ReEDS (Ho et al., 2021), Temoa (Hunter et al., 2013), OSeMOSYS (Howells et al., 2011), and WIS:dom (Clack et al., 2020) use least cost optimization to project the energy investments required to meet energy transition goals over a time horizon. These models assist with national and regional level planning of wind energy deployment by helping develop target capacities. Some, such as Vibrant Clean Energy’s WIS:dom (Clack et al., 2020), are also able to include regional transmission planning by conducting multiple iterations between regional-scale and national-scale models. However, capacity expansion models are complex and require large volumes of input data, thus they are not well suited for regional scenario analyses of EEJ and wind development that require considering finer spatial resolutions.
1.3 Contributions of this paper
In this study, we introduce a new optimization model called SimWINDPRO, and apply it to study the transmission ramifications of environmental/natural resource and EEJ considerations on siting wind energy to meet energy transition goals. SimWINDPRO builds off the original SimWIND tool (Phillips and Middleton, 2012), which was developed to concurrently consider the wind resource potential and consider factors like land surface cover or within transmission routing, but it was not applied to consider EEJ or energy transition goals. Our study is novel for two reasons. First, the SimWINDPRO tool was specifically developed to investigate how to meet energy transition goals via wind energy and transmission infrastructure deployment while simultaneously considering environmental/natural resource and EEJ impacts. As a result, SimWINDPRO fills the methodological tool gap previously discussed and thus can complement other tools previously developed, such as capacity expansion models. To demonstrate this, we use multiple wind generation capacity targets generated by capacity expansion models in our analysis. Second, to our knowledge, our application of SimWINDPRO is also novel: we are the first study to quantitatively investigate the impact that environmental/natural resource and EEJ siting considerations of wind resources may have on the transmission deployment necessary to meet energy transition goals.
2 Methods
SimWINDPRO is a mixed integer linear program that simultaneously optimizes the placement of wind farms, transmission routing, and electricity delivery. SimWINDPRO is implemented in Java and was solved with the CPLEX solver. Here, we document the optimization program of SimWINDPRO, how parameter values are calculated, and background information on our demonstration case, MISO’s western region.
2.1 SimWINDPRO mixed integer linear program
2.1.1 Sets, parameters, and decision variables
The input variables are provided in Table 2 and the decision variables are provided in Table 3.
2.1.2 Model formulation
The problem is formulated as minimizing the capital cost of new power and transmission infrastructure:
Subject to constraints 2 through 6:
The objective function 1) minimizes the weighted cost of deploying new infrastructure including wind farms, transmission lines, and transmission substations, simultaneously. Constraints 2) ensure that the capacities of the deployed transmission lines are between the maximum and minimum capacity available. Constraints 3) ensure that the power capacity into each transmission capacity node equates to the power capacity out of the node while accounting for power capacity additions, power consumption subtractions, and transmission line losses. Constraints 4) ensure that the deployed power source infrastructure at location i is less than or equal to the greatest capacity available to be deployed at that location. Similarly, constraints 5) ensure that the deployed power consumption infrastructure at location j is less than or equal to the greatest capacity available to be deployed at that location. Finally, constraint 6) ensures the total amount of power capacity deployed meets the target capacity.
The multi-criteria weighting factor, Wi, is used to consider factors such as the capacity factor of each potential source location, or other more qualitative factors such as wildlife habitat areas. All these variables are quantified, normalized, and then multiplied by one another to determine Wi. In this study, we only consider the capacity factor in Wi, which enables the model to prefer potential wind sites with higher capacity factors compared to those with lower capacity factors, all else equal. For example, because capacity factors are between zero and one, including the capacity factor in the denominator will ensure that the model preferentially selects wind sites with higher capacity factors, all else equal.
2.1.3 Sample output
To demonstrate SimWINDPRO, we examine deploying 80 GW of wind power infrastructure in the MISO West region; a detailed description of the case study is below. Figure 1A shows all possible wind resource sites (white dots) which represent the total wind capacity of each county, possible transmission paths (purple lines), and demand centers (black dots). The possible transmission paths are calculated with CostMAP using expert-weighted routing factors such as avoiding stream crossings and preferentially moving along established rights of way (Hoover et al., 2020). This information is fed into the SimWINDPRO model, allowing the model to optimally select the combination of wind resource sites, transmission paths, and demand centers to meet a power target. Figure 1B shows an example of a resulting network of wind farms, transmission, and new demand center substations to achieve 80 GW of power.
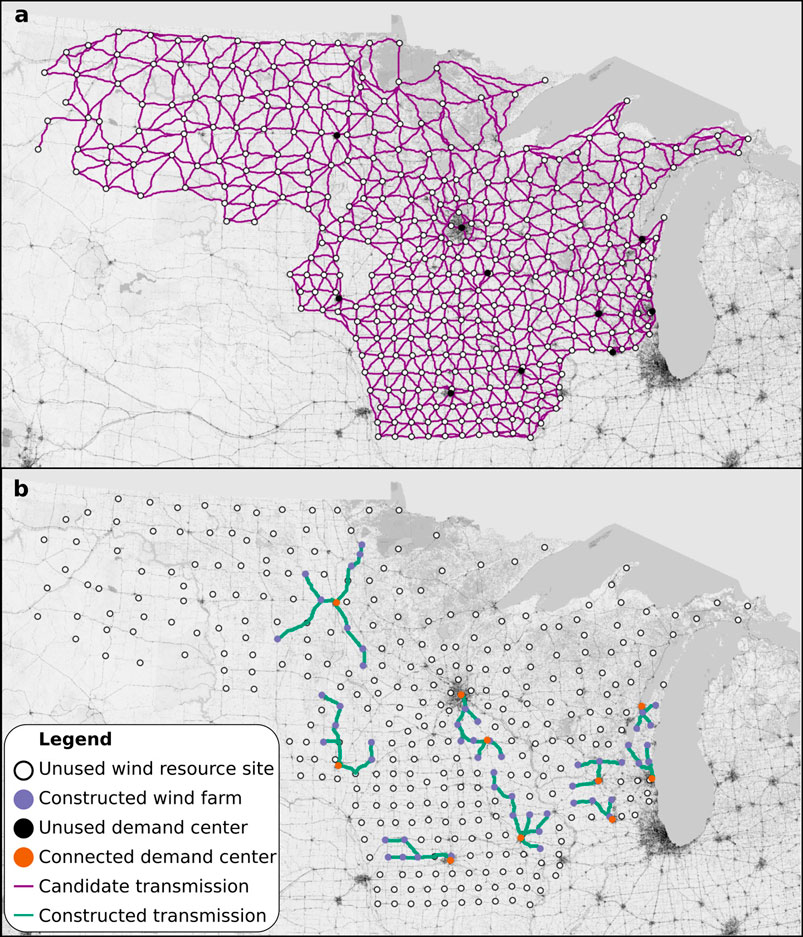
FIGURE 1. (A) Candidate network and resulting (B) deployment of wind farms and transmission centers totaling 80 GW.
2.2 Energy equity index
Social considerations are difficult to quantify, we used a scale from 0 to 11 based on the work of the United States Department of Energy (DOE) 2021 Communities LEAP (Local Energy Action Program) Pilot (Bauer et al., 2021). While no measure is going to perfectly capture the immense nuance of social considerations, the LEAP framework allows the translation of social considerations to a numeric assignment for use in analysis. The quantified scale sums the number of metrics (up to 11) that a census tract meets. Therefore, these metrics are additive, with a larger count corresponding to greater disadvantage or social disparity. LEAP contains the following metrics:
1) Lead paint (% pre-1960 housing),
2) Diesel particulate matter level in the air,
3) Air toxics cancer risk,
4) Air toxics respiratory hazard index,
5) Traffic proximity and volume,
6) Major direct dischargers to water,
7) Proximity to National Priorities List (NPL) sites,
8) Proximity to Risk Management Plan (RMP) facilities,
9) Proximity to Treatment Storage and Disposal Facilities (TSDF),
10) Ozone level in air, and
11) Particulate matter <2.5 in the air.
Our study investigated wind sites at the county level, as opposed to the census tract level provided in LEAP. Therefore, we developed an index that aggregated LEAP data at the county level, that we refer to as the energy equity index (EEI) for this study. A zero EEI value represents no energy equity indicators, and an 11 would be all indicators.
2.3 Case study: MISO West
To demonstrate SimWINDPRO, we explore wind power deployment in the western service area of Midcontinent Independent System Operator, or MISO West (Figure 2). MISO serves 42 million customers (MISO, 2022a) and the western region features some of the best wind energy resources in the United States. As shown in Figure 2C, there are 10 major cities in MISO West, and 172 substations (>=345 kV). MISO is considering massive wind energy deployment, up to 60 GW (MISO Futures Report, 2021). MISO’s transmission network is already significantly over constrained, and in July 2022, MISO announced that it would be investing $10 billion in transmission upgrades (MISO, 2022b).
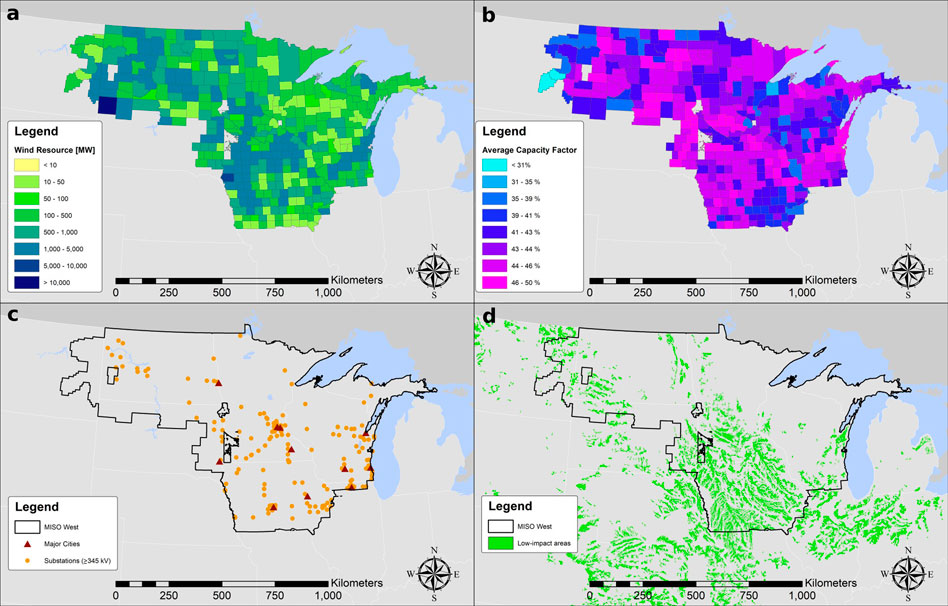
FIGURE 2. MISO West. (A) Wind resource potential tabulated data from NREL WIND Toolkit (Draxl et al., 2015), (B) Wind capacity factor from (Draxl et al., 2015), (C) substations (Homeland Infrastructure Foundation-Level Data (HIFLD, 2021b)), and major cities (Homeland Infrastructure Foundation-Level Data (HIFLD, 2022a)), and (D) low impact areas from The Nature Conservancy’s Site Renewable Right (The Nature Conservancy, 2022).
2.3.1 Wind capacity potential
Wind resource data were taken from NREL’s WIND Toolkit that assume a wind turbine hub height of 100 m (Draxl et al., 2015). For this study, we then aggregated the total capacity and average capacity factor on a county-basis as shown in Figures 2A, B. In MISO West there is a total of 350 GW of wind power potential, with a mean capacity factor of 44% and a mean wind speed of 7.5 m/s.
2.3.2 Wind farm costs
When a new wind farm is constructed, the wind developer must build a transmission spur line from the wind farm to a transmission substation to connect the wind farm to the grid. Modeling these individual wind farm-to-substation transmission lines is too fine a resolution for our regional application of SimWINDPRO. Instead, we assume the population-weighted centroid of each county of our case study has a new substation, to which all wind farm capacity deployed within that county is connected. As a result, the cost of the power source infrastructure in this application of SimWINDPRO (i.e.,
We estimate the cost of the new substations using the Transmission Cost Estimation Guide for the 2021 MISO transmission expansion planning (MISO, 2021b). MISO provides substation costs for varying kV levels, and we use the costs for the 345 kV level: ∼$11,000/MW. As stated in the documentation, this cost estimate includes a 30% contingency and a 7.5% Allowance of Funds Used During Construction (AFUDC). The estimated power capacity is based on the power rating (kV to MVA) that MISO provides in the Transmission Cost Estimation Guide.
We estimate the cost of wind farms using the 2021 NREL Annual Technology Baseline (NREL (National Renewable Energy Laboratory), 2021). Specifically, we use the 2022 overnight capital cost estimate for onshore wind capacity in the moderate case scenario and increase this cost by the same financing assumptions from MISO (i.e., 30% contingency and 7.5% AFUDC) for consistency: ∼$1.7M/MW.
2.3.3 Transmission costs
Transmission costs are based on exploratory cost estimates from the MISO Transmission Expansion Plan (MTEP) (MISO, 2021b). To maintain computational efficiency, SimWINDPRO uses a trend approach as shown in Figure 3 which enables the model to select continuous transmission line capacities. Costs are included in the model on a power basis by assuming a 95% power factor (Zhu et al., 2019).
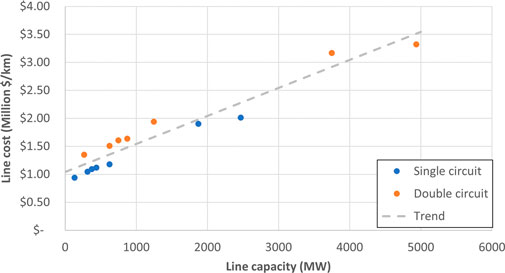
FIGURE 3. Transmission cost trend based on (MISO, 2021b).
2.3.4 Grid connection cost
We estimate the cost of grid connection infrastructure, where electricity is delivered and consumed (i.e.,
2.3.5 EEJ and environmental factors
We apply EEJ in our study by developing scenarios around examples of EEJ considerations. Specifically, we consider historic fossil fuel communities as classified by the Department of Energy’s Communities LEAP Pilot (Bauer et al., 2021). For communities previously dependent on fossil fuel mining or production for their economic success, a transition away from fossil fuel can negatively impact their local economies. Understanding how siting renewables in these communities, as a potential source of local jobs, and outside of these communities, to reduce impacts on communities who have already experienced environmental consequences of previous energy decisions, can be important for understanding regional planning.
To examine the impact of siting when considering environmental impacts, we apply The Nature Conservancy’s Site Renewables Right (The Nature Conservancy, 2022). Site Renewables Right (Figure 2D) indicates which regions are low impact for renewable energy deployment. As the US rapidly expands wind energy infrastructure, the footprint of this technology can alter wildlife habitat and protected land health, which is often co-located in areas likely to be impacted by climate change (The Nature Conservancy, 2022). By considering Site Renewables Right, we are able to integrate areas supportive of wind energy, which also incorporates protecting sensitive land areas. We applied Site Renewables Right to NREL’s Wind Toolkit and removed any sites that were not low development.
2.4 Scenarios
To investigate the impact of EEJ and environmental considerations on wind farm siting we explored a variety of scenarios, summarized in Table 4. We looked at four scenarios to examine EEJ and environmental considerations. The first was a baseline, where no consideration was given to EEJ. For the second, we considered fossil fuel communities by targeting them for development and third, avoiding developing in these regions. Finally, we applied Site Renewables Right to include environmental concerns.
Next, we use four scenarios of different wind power target capacities: 11 GW, 25 GW, 60 GW, and 80 GW. There are three potential “futures” provided in the 2021 MISO Futures Report (MISO Futures Report, 2021), each with progressively higher CO2 reductions. Our first three scenarios are the approximate wind capacities deployed by 2040 across the states in MISO West in that report, which are a result of capacity expansion modeling. The final 80 GW capacity scenario is informed by the NREL 2021a Standard Scenario Report: across a wide range of technology cost assumptions, the NREL 2021b Standard Scenario Report suggests that reducing the nation’s power sector CO2 emissions to 95% below 2005 levels by 2035 and completely eliminating them by 2050 will require deploying approximately 80 GW of wind capacity across the states that comprise MISO West (Cole et al., 2021). This scenario is also a result of capacity expansion modeling. In addition to the four main capacity scenarios, we ran additional capacity target scenarios to add resolution to our results when necessary.
Finally, scenarios are run under two different assumptions about grid connection points. First, we assumed that new transmission would be necessary to connect to major cities because the existing transmission capacity in MISO West is over constrained. However, transmission planners, may want to instead focus on increasing the capacity of existing transmission lines and substations. Therefore, we also consider the possibility of connecting wind sites to existing major substations (>=345 kV) in MISO West. We assume that existing substation locations could be upgraded to handle a 345 kV double circuit, which is capable of approximately 4 GW.
3 Results
The results of our SimWINDPRO scenario analysis are shown and discussed in Section 3.2. As stated in the introduction, given the decline in wind costs, the cost competitiveness of wind energy is no longer the primary constraint limiting deployment. Now, transmission infrastructure, environmental impacts, and EEJ must be considered at least as heavily, if not more so, than cost. Despite this, there are no studies we are aware of that investigate how to site wind and transmission infrastructure to meet energy transition goals while including environmental and/or EEJ considerations. As such, the final result we quantify in section 3.2 is the length of transmission, in kilometers, that SimWINDPRO estimates is needed in different environmental and EEJ scenarios. Before presenting these results, however, we first present the impacts of including environmental and EEJ considerations on the spatial distribution of wind energy (Section 3.1) because it gives context to the ramifications on transmission.
3.1 Impacts of environmental and EEJ considerations on the spatial distribution and capacity of wind energy
Figure 4 shows the energy equity index (EEI) by county for a) all of MISO West (Figures 4A), b) counties labeled as low-impact by Site Renewables Right (Figures 4B), c) counties labeled as fossil fuel communities by the Department of Energy’s Communities LEAP Pilot (Bauer et al., 2021) and d) counties that are not historic fossil fuel communities. Collectively, these maps suggest that as more considerations are included during wind farm siting, the number of counties available for wind development decreases. For example, applying Site Renewables Right (Figure 4B) eliminated 148 counties (41% of the counties) and targeting fossil fuel communities (Figure 4C) eliminated 284 counties (77% of the counties). Conversely, avoiding fossil fuel communities (Figure 4D) would eliminate 86 counties (24%).
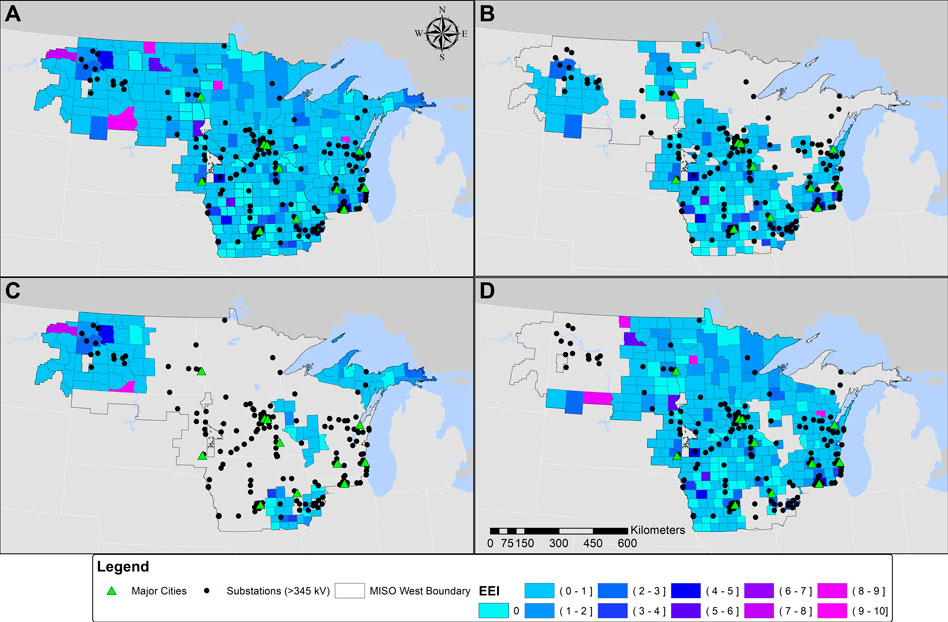
FIGURE 4. Energy equity index, EEI, by county for (A) all counties, (B) counties with low-impact wind per Site Renewables Right, (C) counties that are historic fossil fuel communities, and (D) counties that are not historic fossil fuel communities. For EEI ranges, parenthesis indicate exclusive, and brackets indicate inclusive.
Figure 4 also shows that in addition to reducing the number of counties available for development, EEJ and environmental scenarios can create situations in which there is little to no wind potential co-located with grid connection points (cities and substations). For example, when no EEJ siting considerations are included (Figure 4A), each substation or major city in MISO West is located in a county with wind development potential. However, when Site Renewables Right is considered (Figure 4B), there are 18 substations located in counties without wind development potential. Similarly, when fossil fuel communities are targeted for wind development exclusively (Figure 4C), there are no major cities and only 22 substations located in counties with potential for wind development.
Including EEJ and environmental considerations reduces wind development potential which, in turn, can increase the distance between wind potential and grid connection points because EEJ and environmental factors are not evenly distributed in MISO West. Further, as shown in Figure 2, the potential wind capacity is also not evenly distributed across MISO West. Figure 5 shows MISO West wind capacity supply curves and can be used to investigate the impact that considering these EEJ and environmental factors may have on the wind capacity available to be deployed.
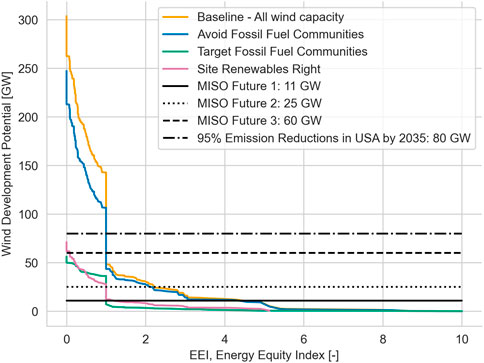
FIGURE 5. Comparison of wind power development supply curves as a function of the energy equity index (EEI) against target capacities from the 2021 MISO Futures Report (MISO Futures Report, 2021) and the NREL 2022 Standard Scenario Report (Cole et al., 2021).
Figure 5 shows the cumulative development potential of wind energy as a function of the EEI using all the wind capacity data (orange) and also using just the portions of capacity that are available for development according to each of the scenarios: avoiding fossil fuel communities (blue), targeting fossil fuel communities (green), and Site Renewables Right (purple). The results show that approximately 30 GW of wind capacity in MISO West is in counties that have been labeled with at least two energy equity indicators and this capacity decreases to approximately 10 GW when Site Renewables Right is also considered.
Figure 5 shows that constraining the potential for wind development by considering the EEI substantially decreases the potential of wind development in MISO West. For example, when applying Site Renewables Right, there is less than 11 GW of wind available in locations with at least an EEI of 5. Considering EEI in this way, at least for the MISO West region considered, reduces the capacity substantially because the EEI is not evenly spread out and there are few counties with an EEI greater than one. For example, as shown in Figure 4, when no other EEJ factors are considered, there are 59 counties with an EEI of zero, 237 counties with an EEI greater than zero and less than one, and 69 counties with an EEI above one.
Figure 5 also compares the available wind development potential against the three target capacities of wind from the MISO futures report (11 GW, 25 GW and 60 GW) as well as the 80 GW target that corresponds to the 95% decarbonized by 2035 NREL scenario. By comparing these capacity goals to the supply curves, Figure 5 suggests that it is not possible to achieve the 80 GW target while avoiding developing wind in the areas that the Site Renewables Right tool suggests should be avoided. It also shows that the 60 GW target cannot be achieved if wind development is only allowed to occur in fossil fuel communities. Further, even without including Site Renewables Right, there is insufficient wind capacity reaching the MISO futures capacity targets if this development must occur in locations with an EEI greater than 1. Overall, the results in Figure 5 suggest that, depending on how they are considered, applying EEJ considerations to the development of wind capacity in MISO West may be at odds with deploying sufficient capacity to meet regional (e.g., MISO Futures capacities) or national (e.g., NREL capacity) climate goals.
3.2 Impacts of including environmental and EEJ considerations in wind planning on new transmission infrastructure
In Figures 6A–D, we compare the network build-out for each EEJ and environmental scenario when 50 GW of wind capacity are deployed. We chose this wind capacity because it was the maximum wind deployment that was possible across all EEJ and environmental scenarios (Figure 5). At this target deployment, there is a substantial difference in transmission networks between the baseline scenario, targeting fossil fuel communities scenario, and site renewables right scenario because of the geospatial differences between where the grid connection points are located and where the wind capacity is located (Figures 2, 4). Further, there is no visually discernable difference in the transmission network build-outs between the baseline and avoiding fossil fuel communities scenario, because comparatively less wind deployment potential is eliminated from the total capacity (Figure 5) and the remaining capacity that is available to be deployed is generally more co-located in the counties with grid connection points (Figure 4).
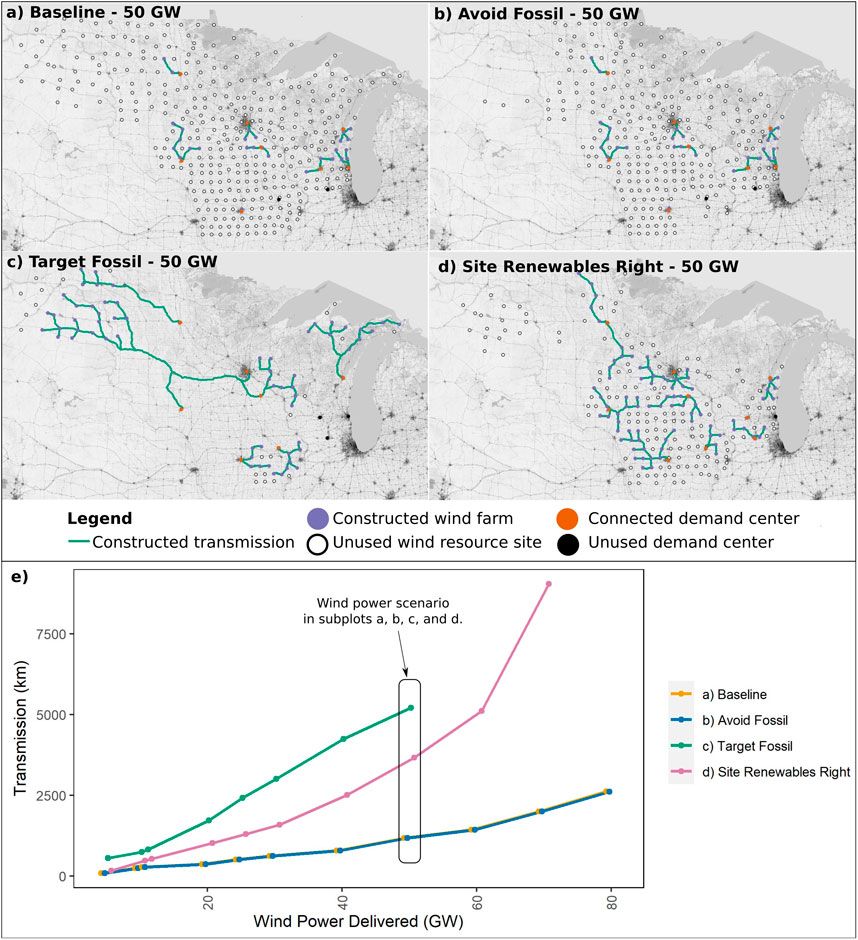
FIGURE 6. Comparison of networks at 50 GW power delivered for (A) baseline, (B) avoiding fossil fuel communities, (C) targeting fossil fuel communities, and (D) Site Renewables Right; against the (E) transmission build-out for each scenario.
Figure 6E compares the amount of transmission constructed for each EEJ and environmental scenario across every wind deployment capacity scenario. The relative change in transmission required as compared to the baseline scenario is also tabulated in Table 5. Figure 6E and Table 5 suggest that the scenarios that result in less total wind deployment potential (Figure 5) require more transmission infrastructure for the same amount of wind deployed. For example, compared to any other scenario investigated, the targeting fossil fuel communities scenario reduced the total amount of wind capacity the most, and requires the most transmission. Targeting fossil fuel communities requires between 190% and 517% additional transmission over the baseline scenario. Similarly, the Site Renewables Right scenario was the second most restrictive scenario for wind development and thus generally resulted in the second least amount of wind capacity potential and requires more transmission than the baseline scenario or avoiding fossil fuel communities scenario. Site Renewables Right required between 89% and 352% additional transmission as compared to the baseline. For the same reasons, there is a negligible difference between the transmission networks of the baseline scenario and avoiding fossil fuel communities scenario.
While it is expected that more transmission will be required when more wind siting considerations are included, Figure 6E also shows this trend can be non-linear. In other words, our results suggest that a ramification of considering EEJ in wind energy siting could be that exponentially more transmission is required to connect wind energy sites to the electricity grid. Further, our results also suggest these general trends hold true when using existing substation locations as the grid connection points instead of major cities (see Figure A1).
4 Discussion
SimWINDPRO is designed to complement existing tools that are already used to study and support the energy transition: 1) modern siting tools that identify regions where wind power could be developed, 2) capacity expansion models that calculate the amount of wind capacity needed to meet energy and climate goals, and 3) power flow models that determine the infrastructure necessary to transport electricity. To illustrate this complementarity, and the potential for SimWINDPRO to enhance the current process of identifying wind sites and transmission corridors, we used wind deployment targets from capacity expansion models in this study. SimWINDPRO is unique from existing tools, and thus its results cannot be directly compared to existing tools.
Our major finding from this study is that incorporating potential social and environmental impacts into wind siting will likely require additional, and possibly exponentially more, investment into transmission infrastructure. The ramification of this finding is far reaching because transmission is already widely recognized as a “bottleneck” for deploying the renewable energy capacities needed to meet energy transition goals. That said, the results of our study are dependent on our inputs and assumptions. Therefore, we have elaborated on several assumptions and resulting caveats:
1) EEJ factors—EEJ has been identified as a critical consideration for the development of infrastructure, however, the definition of EEJ is rapidly evolving. Our study presented an EEI metric based on LEAP, but we recognize that there are many other important EEJ factors that could and should be considered. It is also possible to apply LEAP indices differently than we did in this study. Further research is required to identify which EEJ considerations should be prioritized for wind development, and how those considerations may vary across communities.
2) Site Renewables Right—The Nature Conservancy developed Site Renewables Right to identify regions that are expected to be low impact for developing renewable energy, not to remove areas completely from consideration. Areas not identified as low impact, were not intended to show that development is not possible, but rather identify the area as having a higher environmental risk. There is the possibility that with appropriate mitigation, the regions with higher environmental risks could still be developed. Our study applied Site Renewables Right as a worst-case scenario around potential environmental harm, where only low impact sites could be developed. Future studies would benefit from including mitigation approaches and costs.
3) Environmental factors—Our study limited the consideration of environmental impacts to Site Renewables Right. We recognize that there are additional factors to consider. Also, the scope of Site Renewables Right is limited to the United States Midwest, so studies focusing on other regions will need to identify an alternative approach for considering environmental factors.
4) Location- Our results are specific to our case study of MISO West. Thus, it is possible that studies of other locations may have substantially different results, for example, if limiting areas for deployment based on EEJ or environmental factors did not eliminate substantial portions of the potential wind energy.
We hope that this study can serve as a template for 1) communities as they grapple with deciding how to site energy infrastructure to meet energy transition goals, and 2) infrastructure planners as they refine their siting approaches to consider communities and the environment.
5 Conclusion
In this study we introduce an approach for incorporating energy and environmental justice (EEJ) into wind and transmission siting while considering cost and resource potential and we show that wind siting is substantially impacted when considering these factors. Our study focused on the western region of MISO as an example. The results we present of applying SimWINDPRO are not meant to speak for the preferences of any community, instead we are examining the impact on planning under different scenarios and aiming to provide a tool that helps facilitate an equitable siting process. In the future, communities could apply SimWINDPRO to investigate siting options for their locality. Here, we broadly interpret our results to support future wind energy planning studies. We conclude that:
1) In MISO West, applying EEJ and environmental considerations in wind energy planning can reduce the number of wind sites available and can lead to situations where the remaining sites do not have the development potential capacity to meet regional planning targets determined with capacity expansion models (Figure 5).
2. In MISO West, including EEJ and environmental considerations in wind energy planning can lead to scenarios where grid connection points are no longer in the counties where wind development potential is available (Figure 4).
3) In MISO West, considering EEJ planning scenarios that decrease the available wind development potential will require developing more transmission to meet a given wind energy deployment target (Figure 6).
4) In MISO West, the increase in transmission required when considering EEJ can be non-linear.
5) In MISO West, we found that considering EEJ and environmental impacts can result in selecting different wind farm sites.
Data availability statement
The original contributions presented in the study are included in the article/Supplementary Material, further inquiries can be directed to the corresponding author.
Author contributions
JB: Conceptualization, Methodology, Software, Formal analysis, Writing—Original Draft, Visualization. JO-H: Conceptualization, Methodology, Writing—Original Draft. EM: Conceptualization, Formal Analysis. JE: Formal analysis. MP: Writing—Review and Editing. BR: Conceptualization, Writing—Review and Editing. SY: Conceptualization, Methodology, Software, Writing—Review and Editing, RM: Conceptualization, Methodology, Software, Project administration, Writing—Review and Editing.
Funding
This material is based upon work supported by the U.S. Department of Energy, Office of Science, Small Business Innovations Research program under Award DE-SC0021888.
Conflict of interest
JB, JO-H, EM, JE, and RM were employed by the Company Carbon Solutions LLC.
The remaining authors declare that the research was conducted in the absence of any commercial or financial relationships that could be construed as a potential conflict of interest.
Publisher’s note
All claims expressed in this article are solely those of the authors and do not necessarily represent those of their affiliated organizations, or those of the publisher, the editors and the reviewers. Any product that may be evaluated in this article, or claim that may be made by its manufacturer, is not guaranteed or endorsed by the publisher.
References
Bauer, J., Rose, K., Romeo, L., Justman, D., Popovich, N., Shah, M., et al. (2021). Datasets for DOE 2021 communities LEAP Pilot. doi:10.18141/1820030
Byrne, J., Wolch, J., and Zhang, J. (2009). Planning for environmental justice in an urban national park. J. Environ. Plan. Manag. 52, 365–392. doi:10.1080/09640560802703256
Carley, S., and Konisky, D. M. (2020). The justice and equity implications of the clean energy transition. Nat. Energy 5, 569–577. doi:10.1038/s41560-020-0641-6
Caspary, J., Gardiner, D., Gramlich, R., and Schneider, J. (2021). Disconnected: The need for a new generator interconnection policy. Am. a Clean. Energy Grid. Available at: https://cleanenergygrid.org/wp-content/uploads/2021/01/Disconnected-The-Need-for-a-New-Generator-Interconnection-Policy-1.pdf.
Clack, C. T. M., Choukulkar, A., Coté, B., and McKee, S. A. (2020). Weather-informed energy systems : For design , operations and markets (planning version). Available at: https://vibrantcleanenergy.com/wp-content/uploads/2020/08/WISdomP-Model_Description(August2020).pdf.
Cole, W., Carag, V. J., Brown, M., Brown, P., Cohen, S., Eurek, K., et al. (2021). 2021 standard scenarios report: A U.S. Electricity sector outlook. Golden, CO. Available at: www.nrel.gov/publications.
Draxl, C., Clifton, A., Hodge, B. M., and McCaa, J. (2015). The wind integration national dataset (WIND) Toolkit. Appl. Energy 151, 355–366. doi:10.1016/j.apenergy.2015.03.121
Duthie, J., Cervenka, K., and Waller, S. T. (2007). Environmental justice analysis: Challenges for metropolitan transportation planning. Transp. Res. Rec. 8, 8–12. doi:10.3141/2013-02
Hanssen, F., May, R., Van Dijk, J., and Rød, J. K. (2018). Spatial multi-criteria decision analysis tool suite for consensus-based siting of renewable energy structures. J. Environ. Assess. Policy Manag. 20, 1840003–1840028. doi:10.1142/S1464333218400033
Harrison-Atlas, D., Lopez, A., and Lantz, E. (2022). Dynamic land use implications of rapidly expanding and evolving wind power deployment. Environ. Res. Lett. 17, 044064. doi:10.1088/1748-9326/ac5f2c
Heleno, M., Sigrin, B., Popovich, N., Heeter, J., Jain Figueroa, A., Reiner, M., et al. (2022). Optimizing equity in energy policy interventions: A quantitative decision-support framework for energy justice. Appl. Energy 325, 119771. doi:10.1016/j.apenergy.2022.119771
Hemmati, R., Hooshmand, R. A., and Khodabakhshian, A. (2013). State-of-the-art of transmission expansion planning: Comprehensive review. Renew. Sustain. Energy Rev. 23, 312–319. doi:10.1016/j.rser.2013.03.015
HIFLD (2022a). Homeland infrastructure foundation-level data (HIFLD) cities and towns NTAD. Available at: https://hifld-geoplatform.opendata.arcgis.com/datasets/geoplatform::cities-and-towns-ntad (Accessed February 8, 2022).
HIFLD (2021b). Homeland infrastructure foundation-level data (HIFLD) electric substations. Available at: https://hifld-geoplatform.opendata.arcgis.com/datasets/geoplatform::electric-substations/about (Accessed December 29, 2021).
Ho, J., Becker, J., Brown, M., Brown, P., Chernyakhovskiy, I., Cohen, S., et al. (2021). Regional energy deployment system (ReEDS) model documentation: Version 2020. Natl. Renew. Energy Lab. Available at: https://www.nrel.gov/docs/fy21osti/78195.pdf.
Holifield, R. (2001). Defining environmental justice and environmental racism. Urban Geogr. 22, 78–90. doi:10.2747/0272-3638.22.1.78
Hoover, B., Yaw, S., and Middleton, R. (2020). CostMAP: An open-source software package for developing cost surfaces using a multi-scale search kernel. Int. J. Geogr. Inf. Sci. 34, 520–538. doi:10.1080/13658816.2019.1675885
Howells, M., Rogner, H., Strachan, N., Heaps, C., Huntington, H., Kypreos, S., et al. (2011). OSeMOSYS: The open source energy modeling system. Energy Policy 39, 5850–5870. doi:10.1016/j.enpol.2011.06.033
Hunter, K., Sreepathi, S., and DeCarolis, J. F. (2013). Modeling for insight using tools for energy model optimization and analysis (Temoa). Energy Econ. 40, 339–349. doi:10.1016/j.eneco.2013.07.014
IEAIRENAUNSDWorld BankWHO (2022). Tracking SDG7: The energy progress report. Available at: https://trackingsdg7.esmap.org/data/files/download-documents/2021_tracking_sdg7_chapter_6_outlook_for_sdg7.pdf.
Israel, B. (2012). Coal plants smother communities of color. Sci. Am. Available at: https://www.scientificamerican.com/article/coal-plants-smother-communities-of-color/.
Jenkins, J. D., Mayfield, E. N., Larson, E. D., Pacala, S. W., and Greig, C. (2021). Mission net-zero America: The nation-building path to a prosperous, net-zero emissions economy. Joule 5, 2755–2761. doi:10.1016/j.joule.2021.10.016
Jenkins, K. (2018). Setting energy justice apart from the crowd: Lessons from environmental and climate justice. Energy Res. Soc. Sci. 39, 117–121. doi:10.1016/j.erss.2017.11.015
Jenkins, K., Sovacool, B. K., and McCauley, D. (2018). Humanizing sociotechnical transitions through energy justice: An ethical framework for global transformative change. Energy Policy 117, 66–74. doi:10.1016/j.enpol.2018.02.036
Larson, E., Greig, C., Jenkins, J., Mayfield, E., Pascale, A., Zhang, C., et al. (2021). Mission net-zero America: The nation-building path to a prosperous, net-zero emissions economy. Princet. Univ. 1–345, 2755–2761.
Lazard (2021). Lazard’s levelized cost of energy analysis — version 15.0. Available at: https://www.lazard.com/media/451905/lazards-levelized-cost-of-energy-version-150-vf.pdf.
Lee, N., Flores-espino, F., and Hurlbut, D. (2017). Renewable energy zone (rez) transmission planning process: A guidebook for practitioners. Golden, CO.
Lopez, A., Mai, T., Lantz, E., Harrison-Atlas, D., Williams, T., and Maclaurin, G. (2021). Land use and turbine technology influences on wind potential in the United States. Energy 223, 120044. doi:10.1016/j.energy.2021.120044
Maclaurin, G., Grue, N., Lopez, A., and Heimiller, D. (2019). The renewable energy potential (reV) model: A geospatial platform for technical potential and supply curve modeling. Available at: https://www.nrel.gov/docs/fy19osti/73067.pdf.
IPCC (2018). “Summary for policymakers,” in Global Warming of 1.5°C. An IPCC Special Report on the impacts of global warming of 1.5°C above pre-industrial levels and related global greenhouse gas emission pathways, in the context of strengthening the global response to the threat of climate change. Editors V. Masson-Delmotte, P. Zhai, H.-O. Pörtner, D. Roberts, J. Skea, and P. R. Shukla. doi:10.1016/j.oneear.2019.10.025
MISO Futures Report (2021). MISO futures report. Available at: https://cdn.misoenergy.org/MISO Futures Report538224.pdf.
MISO (2022a). Operating the power grid, managing the energy markets, planning the future grid. Available at: https://www.misoenergy.org/about/ (Accessed March 10, 2022).
MISO (2021a). Points of interconnection. Available at: https://giqueue.misoenergy.org/PoiAnalysis/index.html (Accessed November 18, 2021).
MISO (2022b). Reliability imperative: Long range transmission planning. Available at: https://cdn.misoenergy.org/20220725 Board of Directors Item 02a Reliability Imperative LRTP625714.pdf.
MISO (2021b). Transmission cost estimation Guide for MTEP21. Available at: https://cdn.misoenergy.org/20210209 PSC Item 06a Transmission Cost Estimation Guide for MTEP21519525.pdf.
NREL (National Renewable Energy Laboratory) (2021b). 2021 annual technology baseline. Available at: https://atb.nrel.gov/ (Accessed March 10, 2022).
NREL (National Renewable Energy Laboratory) (2022). 2022 annual technology baseline. Available at: https://atb.nrel.gov/electricity/2022/data.
NREL (National Renewable Energy Laboratory) (2021a). Slope: State and local planning for energy. Available at: https://maps.nrel.gov/slope (Accessed March 10, 2022).
Phillips, B. R., and Middleton, R. S. (2012). SimWIND: A geospatial infrastructure model for optimizing wind power generation and transmission. Energy Policy 43, 291–302. doi:10.1016/j.enpol.2012.01.006
Sovacool, B. K., and Dworkin, M. H. (2015). Energy justice: Conceptual insights and practical applications. Appl. Energy 142, 435–444. doi:10.1016/j.apenergy.2015.01.002
Swennenhuis, F., Gooyert, V. D., and Coninck, H. D. (2022). Energy Research & Social Science towards a CO 2 -neutral steel industry : Justice aspects of CO 2 capture and storage , biomass- and green hydrogen-based emission reductions. Energy Res. Soc. Sci. 88, 102598. doi:10.1016/j.erss.2022.102598
The Nature Conservancy (2022). Site renewables right : Accelerating a clean and green renewable energy buildout in the Central United States. The Nature Conservancy's Great Plains Renewable Energy Initiative. Available at: https://www.nature.org/en-us/what-we-do/our-priorities/tackle-climate-change/climate-change-stories/site-wind-right/
Wiser, R., Bolinger, M., Hoen, B., Millstein, D., Rand, J., Barbose, G., et al. (2021). Land-based wind market report: 2021 edition. Available at: http://www.osti.gov.
Young, S., Mallory, B., and McCarthy, G. (2021). The path to achieving Justice40. Washington, DC: White House Brief. Room. Available at: https://www.whitehouse.gov/omb/briefing-room/2021/07/20/the-path-to-achieving-justice40/.
Zhu, Y., Dinakar, A., Wang, W., and Brown, D. (2019). MISO DPP 2017 february west area phase 3 study. Minnetonka, MN. Available at: https://cdn.misoenergy.org/GI-DPP-2017-FEB-West-Phase3_System_Impact_Report_PUBLIC391580.pdf.
Appendix A
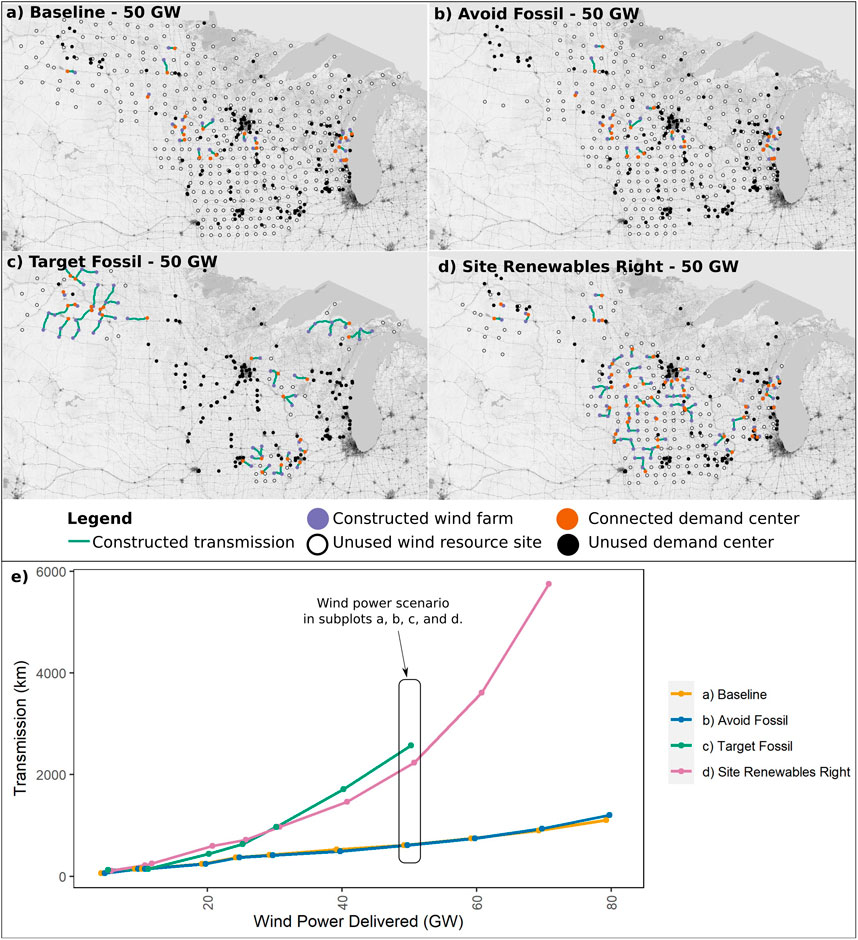
FIGURE A1. Comparison of networks using substations as grid connection points at 50 GW power delivered for (A) baseline, (B) avoiding fossil fuel communities, (C) targeting fossil fuel communities, (D) Site Renewables Right against the (E) transmission build-out for each scenario.
Keywords: wind power, energy and environmental justice, optimization, GIS, energy transition
Citation: Bennett JA, Ogland-Hand JD, Middleton EJ, Eidbo JB, Prorok M, Ross B, Yaw S and Middleton RS (2023) The transmission ramifications of social and environmental siting considerations on wind energy deployment. Front. Energy Res. 10:1040957. doi: 10.3389/fenrg.2022.1040957
Received: 09 September 2022; Accepted: 12 December 2022;
Published: 06 January 2023.
Edited by:
Shiwei Yu, China University of Geosciences Wuhan, ChinaCopyright © 2023 Bennett, Ogland-Hand, Middleton, Eidbo, Prorok, Ross, Yaw and Middleton. This is an open-access article distributed under the terms of the Creative Commons Attribution License (CC BY). The use, distribution or reproduction in other forums is permitted, provided the original author(s) and the copyright owner(s) are credited and that the original publication in this journal is cited, in accordance with accepted academic practice. No use, distribution or reproduction is permitted which does not comply with these terms.
*Correspondence: Jeffrey A. Bennett, amVmZnJleS5iZW5uZXR0QGNhcmJvbnNvbHV0aW9uc2xsYy5jb20=