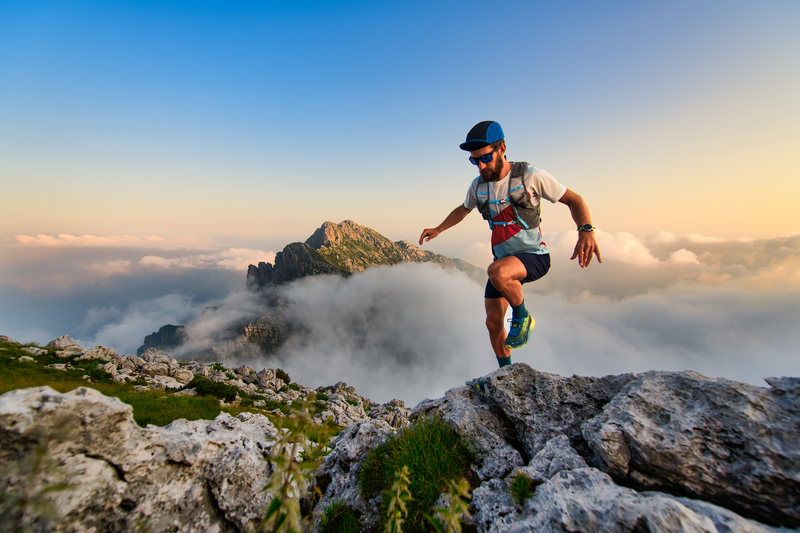
95% of researchers rate our articles as excellent or good
Learn more about the work of our research integrity team to safeguard the quality of each article we publish.
Find out more
ORIGINAL RESEARCH article
Front. Energy Res. , 12 January 2023
Sec. Advanced Clean Fuel Technologies
Volume 10 - 2022 | https://doi.org/10.3389/fenrg.2022.1026860
This article is part of the Research Topic Carbon Emission of Energy Systems View all 5 articles
By virtue of the hedging and price discovery function, carbon futures trading may help carbon market function more effectively. Is it necessary to establish carbon futures trading in China? The authorities have endorsed the idea since 2015, however, the scheme has not yet started; no antecedent pre-assessment quantitative research has been carried out. Therefore this study that attempts to fill this gap in the literature, could be of real significance. Through deriving a potential decarbonization roadmap, this study tries to give some clues pertaining to the converted mitigation strategy imposed by carbon futures trading in China. A model chain has been proposed, which is composed of the Optimal Production Decision-making Model for Producers, Life Cycle Impact Assessment, Monetization, and Genetic Algorithm based optimization, to quantify the environmental benefits (including improvements to human health, ecosystem damage and increased temperature induced GDP losses) of the assumed mitigation trajectories. By setting the maximization of environmental benefits as the objective, the optimal decarbonization roadmap with carbon futures trading is derived. Results show that the optimal emission reductions for power enterprises (covered by the carbon market) for the next 10 years (2021–2030) are around 3.27 billion tonnes CO2e. If 36% of this amount is assigned to previously discussed mitigation trajectories, it is found that 106.98 GW ultra-super critical units, 160.85 GW mono-Si PV facilities and 167.26 GW doubly-fed induction generator wind capacity should be installed. Overall environmental benefits are 4.6 trillion CNY2018, over 5% of China’s 2018 GDP. Results demonstrate the optimal emission reductions and potential decarbonization roadmap for China’s power enterprises (those covered by the carbon market) under the context of carbon futures trading, which can be an important reference for the authorities and therefore encourage the establishment of the scheme.
The emission trading mechanism is considered as one of the most effective tools to tackle global climate change. One of the most valuable experiences from the world’s largest carbon market—the European Union Emissions Trading Scheme (EU ETS)—is that carbon futures could be the dominant trading underliers [actually, back in 2015, the trading volume of carbon futures was nearly 30 times of the carbon spot in EU ETS (Yuan, 2019)]. It is considered that carbon futures trading can provide the potential to hedge carbon price uncertainty and the function of price discovery (Zhang et al, 2020a; Stefan and Wellenreuther, 2020), which are significant for all participants in the carbon market. Some researchers also proposed that carbon futures is necessary as a means of supporting the local carbon market (Kim, 2014; Wang, 2016; Zhou and Li, 2019).
In the context of China, the authorities have been publicly endorsing the establishment of carbon futures trading scheme since 20151 and the ETS-covered power enterprises will be the initial participants. However, it has not yet started due to several unsolved problems. For example, whether the financial institutions can provide professional services for participants; whether there are enough regulation tools to help stabilize the market; and most importantly, what will be its potential influences on China’s ambitious climate change targets? Meanwhile, considering that China’s national carbon market is under construction, new mechanisms and products are expected to be incorporated into it. Consequently, there exists an opportunity for the introduction of carbon futures trading scheme in China. Before that, a full understanding of the significance of carbon futures trading to China is desperately needed.
Plenty of researches on carbon futures trading have been done since the first trading of carbon futures in the EU ETS in 2005. Here, we divided these literatures into three subgroups. The first subgroup mainly focused on the relationship between the carbon futures price, trading volume and volatility. Some analyzed the volume and volatility relationship between two main carbon futures products in the EU ETS (Kim, 2014; Rannou and Barneto, 2016), the basic results showed that there existed the lead-lag relationship between them, but it varied at different frequencies (Zou and Zhang, 2020). Apart from the EU ETS, the volatility dynamics for Carbon Financial Instruments traded in the Chicago Climate Exchange were noticed (Sabbaghi and Sabbaghi, 2011), and thin trading effects was found. The second subgroup was related to the market mechanism of carbon futures trading. Such topics were explored that the memory of futures’ price (Huettner and Sunder, 2012), dependence structure between the price and its pricing fundamentals (Chevallier, 2009; Tan and Wang, 2017), liquidity concerns of the market (Kalaitzoglou and Ibrahim, 2015) even topics like manipulation and fraud matters (Leconte and Pagano, 2010) and scheme transparency (Kirke, 2012). Furthermore, the price discovery function of the ICE (Intercontinental Exchange) and EEX (European Energy Exchange) were also compared (Stefan and Wellenreuther, 2020). It was found that ICE dominated the price discovery and led the price of carbon futures. The final subgroup paid attention to how the carbon futures market interacted with other common commodity futures markets. It provided useful information to better understand the market, and more practically, to design portfolio strategies. Co-movement between carbon assets and energy commodities was analyzed (Ortas and Álvarez, 2016), it was concluded that investors could incorporate carbon futures assets into an energy commodities-oriented portfolio for risk diversification purposes, which was supported by later research (Uddin et al., 2018). Conversely, some pointed out that dynamic diversified portfolios were much preferred for reducing volatility and the downside risks of carbon assets (Wen et al., 2017). To sum up, no antecedent pre-assessment studies on China’s carbon futures trading were carried out. However, the quantitative pre-assessment can uncover China’s carbon reduction potential as well as the environmental benefits, therefore helping policy-makers better understand the mechanism’s potential contribution to the ambitious carbon neutral target by 2060, which is the very point of this study.
In this paper, we try to fill the gap in this research area. This study mainly focuses on deriving the optimal mitigation strategy for the ETS-covered power enterprises in the context of carbon futures trading, the corresponding emission reductions and environmental benefits. In general, our work is based on a model chain which is composed of the Optimal Production Decision-making Model for Producers (OPDP), Life Cycle Impact Assessment (LCIA), monetization of environmental benefits and Genetic Algorithm (GA) based optimal decarbonization roadmap planning.
First, after analyzing the essence of carbon futures trading, it is suggested that locking down the executive carbon price2 (ECP) is its greatest significance, therefore locking the carbon price is taken as the proxy for carbon futures trading. Second, the OPDP is employed to derive the optimal mitigation amount for different carbon prices. Basic results reveal that around 81.67 g CO2e should be mitigated for 1 kW·h electricity production, and further, the optimal mitigation amount would decrease as the carbon price goes up. Third, ReCiPe2016 based LCIA analysis is conducted for 1 kW·h electricity production under five technical approaches: subcritical (Sub-C), supercritical (Super-C), ultra-super critical (USC) coal-fired power units, mono-Si PV and doubly-fed induction generator for wind turbines (DFIG). In this step, the endpoint level impact categories of Damage to Human Health (DTH) and Damage to Ecosystem (DTE), as well as the increased temperature induced GDP losses (GDPL) are considered. 5 decarbonization trajectories are then put forward, for example, trajectory 1 (TR 1) is to build Super-C coal-fired units, the corresponding emission reductions and environmental impact differences are calculated based on Sub-C units. Fourth, following the work of Rauner (Rauner et al., 2020), the environmental benefits of different decarbonization trajectories are monetized. Finally, to plan the optimal decarbonization roadmap, GA is utilized with the objective function of maximizing the environmental benefits. Results show that in the next 10 years, the ETS-covered power enterprises should at least newly install 106.98 GW USC units and 160 GW mono-Si PV, 167.26 GW DFIG capacity. The overall environmental benefits account for 4.6 trillion CNY2018, over 5% of China’s 2018 GDP volume.
The rest of the paper can be divided into four parts. Part two presents the methodology, part three is the data and results, a discussion section is demonstrated in part four and the final part presents the conclusion.
A model chain is used to determine the optimal decarbonization pathway for ETS-covered power enterprises under the carbon futures trading context. As shown in Figure 1, it includes a production decision model to determine the optimal mitigation amount, ReCiPe2016 based LCIA analysis to calculate the environmental impacts of different electricity production approaches, a monetization method to transform the environmental impact into capital loss and a Genetic Algorithm based optimal planning tool for deriving the decarbonization roadmap.
The futures trading scheme has existed in the financial industry for a long time. Currently, actively traded products include commodity futures and financial futures. Basically, the futures market is a place for risk transfer, it offers hedging and price discovery function. Specifically, manufacturing enterprises play the role of hedgers, while financial institutions and private investors act as speculators (sometimes are hedgers too), the former is commonly risk-averse, but the latter is risk-tolerant. Thus, the hedger can transfer the price risk to the speculators, as a price they give up the chance to obtain excess income. What’s more, due to the abundant information brought by the participants, the futures prices are very close to the actual value, which helps to realize the price discovery function.
The carbon futures trading scheme is similar to the general financial futures trading scheme. The ETS-covered power enterprises play the role of hedgers, they are extremely risk-averse. Therefore, it is assumed that the main purpose for them to participate in the carbon futures market is to hedge the risk of carbon price volatility, the net effect of hedging is locking in the executive carbon price, though the actual price level varies over time. In short, the determined ECP can be taken as the proxy for carbon futures trading.
The permanent goal for enterprises is to seek for the maximum profits. Here, the OPDP is employed to determine the optimal mitigation amount for the ETS-covered power enterprises in the context of carbon futures trading. Some basic assumptions are adopted in this model, which help the model be more realistic. Firstly, the allowance allocation rule is assumed to be the benchmark method. Currently, free allocation distribution in China’s pilot carbon market is realized through a mixed method of “grandfathering and benchmarking,” however, the latter is expected to be the dominant allocation method in the forthcoming China’s national carbon market (Zhang et al., 2015; Yang et al., 2020). Secondly, the distribution of consumers’ low-carbon awareness is assumed to be the
The general ideas of this model is that power producers will change their production plan according to the market feedbacks, which includes the carbon emission costs, subsidies for greener production and green electricity prices, etc. Through this model, we can derive what should be the most profitable optimal emission reductions in the context of carbon futures trading. The following part will present the model in detail.
Eq. 1 shows the number of free allowances that the ETS-covered power enterprises can obtain under the benchmarking allocation rule. Eq. 2 displays the distribution of consumers’ low-carbon awareness δ, which as stated previously, follow the
As for the number of emission reductions by the enterprises, two ideas are considered in our model: the actual mitigation amount
Finally, we can derive the general profit of the enterprises in Eq. 8. Pertaining to the mitigation cost, following by a literature review (Gao et al., 2004; Zhang et al., 2015; Zhou et al., 2016; Han, 2018) we conclude that it should take a unary quadratic function form, which is shown in Eq. 9. Table 1 sets out the meanings and specific values of parameters in the model.
To derive the optimal mitigation amount, we firstly let the derivative of profit
Life Cycle Assessment (LCA) is widely used to determine the life cycle impact of a product (Di et al., 2007; Gerbinet et al., 2014). Our paper focuses on the life cycle impact assessment of electricity. As we all know, electricity can be produced through many approaches; however, the most relevant approaches in our study are coal-fired power units, PV and wind. Different technical trajectories exist even in the same kind of approach, but for the convenience of analysis, we choose Sub-C, Super-C and USC units for the coal-fired power units; mono-crystal silicon photovoltaic (mono-Si PV) for PV; and DFIGs for wind (see reasons for selection in Section 3.1).
Emission inventory data was collected from existing researches. Literatures quoted have a common feature that the function unit is the production of 1 kW·h electricity. The actual process of different technologies differs. In detail, the LCA for the coal-fired units includes coal-mining, coal transportation and electricity generation process (Liang et al., 2013); the mono-Si PV includes raw material extraction, manufacture of cell and module fabrication, assembling, transportation, application and decommissioning (Celik et al., 2016); DFIG of wind includes raw material supply, manufacturing phase, transportation and assembly process, operation and maintenance phase, dismantling, recycling and waste disposal (Siddiqui and Dincer, 2017).
Regarding the LCIA method, ReCiPe 2016 (Huijbregts et al., 2017) is widely applied (Bergesen et al., 2014; Celik et al., 2016; Huang et al., 2017; Rauner et al., 2020). After comparing ReCiPe2016 and other LCIA methods, it is found that this approach performs better in the context of China for some impact factors are specifically prepared for China; thus, the ReCiPe2016 is selected as the LCIA method in this study. As for the impact categories, we chose Global Warming Potential, Particular Matter, Acidification, Eutrophication and other impact categories from the overall 17 midpoint level impact categories, and the Damage to Human Health and Damage to Ecosystems from the three endpoint level impact categories. The endpoint-level impact categories are used for further calculation, which is to monetize the environmental impacts of 1 kW·h electricity production by different technologies.
The environmental impacts of a product can be understood well through performing LCIA analysis; however, these impact categories are hard to compare since their metrics vary a lot. Monetization is a good pathway to solve this problem, much monetization work has been carried out, from the human health impact (Partridge and Gamkhar, 2012; Gao et al., 2015; Cai et al., 2018; Wang et al., 2020) to ecosystem damage (Rauner et al., 2020).
(Rauner et al., 2020) gave a good example in the monetization of environmental damage field, we quoted their monetarization factors and made some adjustments to transform the data to make it better fit in the context of China. In detail, the damage to human health is measured by the unit of Disability Adjusted Life Years (DALY), as 1.936 Million CNY2018/DALY; the damage to ecosystem is quantified through species per year loss, the value is set as 1.823 Billion CNY2018/(species·year).
Another important aspect is the cost of rising temperatures, which is primarily caused by Greenhouse Gases (GHGs). First of all, we need to determine the actual temperature rise caused by GHG emissions. Matthews gave a reference that the ambient temperature tends to rise 1.8°C for every 1,000 Gt CO2e emissions (Matthews et al., 2012). Therefore, with the carbon emission inventory and consumed quantity of electricity, the exact temperature rise
According to the final estimates carried out by Nordhaus (2017), a 3°C warming will cause a global income loss of 2.1%, and a 6°C warming will cause a loss of 8.5%. The coefficient is calculated to be
Founded by Holland (1997) in the 1970s, the Genetic Algorithm (GA) has been widely used in the optimization field (Fatemi Aghda and Mirfakhraei, 2020; Yeh et al., 2020). Through imitating the natural selection and biological evolution process, the GA can find the best solution for the optimization problem. The basic idea for GA is, in the biological evolution process, those who survived were best adapted to the changing environment. In its mathematical counterpart, the principle is that only those who can best satisfy the constraints should be selected as the overall optimal solution, while those failed to satisfy the constraints will be weeded out.
In our study, the objective is to find the decarbonization roadmap which has the maximum environmental benefits, which is expressed in Eq. 14. According to the specific condition of our goal, nine constraints are included, which are displayed in Table 2. Furthermore, the number of evolving generations is set to be 3,000.
Objective function: total environmental benefits should be maximized
The ultimate target of carbon futures trading is to vector better emission reduction trajectories. Normally, such trajectories include the optimization of production processes, upgrading production techniques, limiting the operating hours of low-efficiency coal-fired power units or just simply buying allowances to satisfy the emission cap. Chinese coal-fired power plants have gone through the Ultra-low Emission Retrofit Plan since 2015. The latest review reported that over 86% of the coal-fired units now satisfy the emission limits (China Electricity Council, 2020), which indicates that the potential of optimizing the production processes is quite limited. Thus, the choices for ETS-covered power enterprises remain upgrading production techniques, renewable energy investments, carbon allowance trading and adjusting operational strategies (e.g., cutting down the utilization hours of low efficiency thermal units). In this paper, the pathways of production techniques upgrading and renewable energy investment are mainly considered, with the aim of achieving around 36% of the total emission reduction.
Five trajectories are considered when planning the optimal decarbonization roadmap: (TR1) Sub-C replaced by Super-C; (TR2) Super-C replaced by USC; (TR3) Sub-C replaced by USC; (TR4) newly installed mono-Si capacity; (TR5) newly installed DFIG capacity. It is worth noting the basis for this design: the Sub-C coal-fired units generally emit more than Super-C, and much more than USC and renewable energies. Therefore, the Sub-C units should be phased out firstly. As shown in Figure 2B, the newly installed thermal power capacity is mainly composed of the Super-C and USC units. The Super-C units are much more environmentally friendly than Sub-C, but perform worse than USC, further, as commercialized USC units are becoming available according to Figure 2A, in trajectory 2 we consider the possibility of replacing Super-C with USC units.
FIGURE 2. Trends in the installation of coal-fired units. (A) Technological trends since 1950, the data is referenced from (Yu and Guo, 2019). (B) Components of the installed capacity since 1990, data is collected from (Cui et al., 2020).
Emission reductions are difficult to achieve with regard to coal-fired power plants, and consequently power enterprises are becoming more and more interested in renewable energy rather than fossil fuel. As shown in Figure 3, the installed thermal power capacity is decreasing while the PV and Wind are increasing, and the volume of non-thermal power new investment is larger than 70% in recent years (see the spline in Figure 3). In practice, China Huaneng Group, one of the largest power enterprises in China, installed mainly PV and Wind capacity in year 2019 (Juchao Information Network, 2019b), which is echoed by GD Power Development Co., Ltd. (Juchao Information Network, 2019a). PV and Wind seem to be currently the most promising renewable energies4 and some researchers suggested that clean energy investment would help enterprises better adapt to China’s national carbon market (Yu et al., 2020). Consequently, in trajectories 4 and 5, we choose to newly install PV and Wind capacity. However, PV includes three generations of cells which have different environmental impacts, and the Wind industry also includes many types. After investigation, we finally choose mono-Si for PV and DFIG for Wind since their performance and market share are much better or larger [(Celik et al., 2016) and Bloomberg5].
To calculate the total optimal emission reduction for the next 10 years, a reasonable prediction on thermal electricity production and newly installed capacity are necessary, which are influential factors in the OPDP model.
Considering the current world economic environment and its prospects, we project that electricity consumption in China will sustain a 2% average yearly increase for the next 10 years. Now that electricity consumed in 2020 is predicted to be 6362.50 TW·h (China Electricity Council, 2020), we project that electricity consumed in 2021 will be 6916.3 TW·h and 9012.72 TW·h in 2030 as shown in Figure 4. Yu and Guo (2019) demonstrated that the cumulative proportion of Sub-C, Super-C and USC coal-fired units is around 79.4% of total installed thermal power capacity, which is echoed by Figure 2B. Thus, we assume that 79.4% of the projected total thermal electricity volume is produced by the coal-fired units.
FIGURE 4. Projected national electricity consumption, newly installed capacities of thermal power and PV and wind until 2030.
Concerning the assumed annual newly installed capacity, we made several realistic assumptions: 1) if the electricity elasticity6 is 0.8, then the overall growth rate of newly installed capacity can be set at 4% per year; 2) the share of thermal power in the newly installed capacities will decrease substantially in the next 10 years. According to CEC (China Electricity Council, 2020), the non-fossil newly installed capacity in 2020 is projected to be 89 GW or over 75% of total installed capacity. In this paper, it is assumed that in the coming 10 years, the share of newly installed capacity of coal-fired units will decrease from 18% in 2020 to 1.5% in 2030, and the missing market share of thermal power will be gained by PV and Wind. These projections are shown in detail in Figure 4, the national newly installed capacity will increase from 113.57 GW in 2021 to 161.64 GW in 2030; the newly installed coal-fired units will decrease from 18.17 GW to 2.42 GW; PV and Wind are projected to increase from 62.12 GW in 2021 to 154.56 GW in 2030. What should be noted is that, in the Strategy for Energy Production and Consumption Revolution (2016–2030)7, it is required that renewables should constitute the majority of newly installed capacity in 2030. Our projection can satisfy the requirement since newly installed renewables take a share of more than 95.6% in 2030.
Based on the above analysis, we can derive reliable results in the OPDP model. As for the LCIA analysis, emission inventory data under different technical approaches for 1 kW·h electricity production are collected from existing literature (Liang et al., 2013; Celik et al., 2016; Siddiqui and Dincer, 2017), which mainly include the amount of emitted CO2, SO2, NOx, CH4, heavy metals, etc. With the method of ReCiPe 2016, the emission inventory data is calculated as the data of midpoint and endpoint level impact categories. Subsequently, we can derive the human health damage cost, environmental damage cost and economic losses caused by rising temperatures by utilizing the monetization method.
Pertaining to the optimal decarbonization path planning, the initial derived results will be expressed in the form of electricity production volume for different trajectories. Then, through the respective utilization hours, we can convert the electricity into capacity. Actually, the annual utilization hours (AUH) of different electricity production techniques vary a lot. According to our investigation, the national overall AUH increased from 3,790 h in 2017 to 3,820 h in 2019. If we separate the overall AUH into thermal power AUH, PV AUH and Wind AUH, we can find that the thermal power AUH increase from 4,219 to 4,307 h, PV from 1,205 to 1,291 h and 1949 h–2083 h for Wind.
A unique phenomenon of “PV and Wind abandon” has appeared in northwest China over the past few years, which is disturbing to the power sector. However, with the ultra-high voltaic transmission project coming into production and the construction of flexible peak shaving ability, such abandoning will ease (Si et al., 2019). Thus, we made some forecasts on the AUH in the next 10 years: 4,200 h for general coal-fired power units, 1,200 h for PV and 2,000 h for Wind.
Firstly, the optimal mitigation amount is calculated. We take the scope of ECP from 20 CNY2018 to 300 CNY2018, which is relatively close to the carbon price scope in the pilot carbon markets of China. The optimal mitigation volume under different ECP is shown in Figure 5.
FIGURE 5. Optimal mitigation volume
The general trend is that the optimal mitigation volume will decrease with the increasing ECP, which indicates that the power enterprises are sensitive to the carbon price and they tend to mitigate less GHGs once the carbon price increases. One possible explanation for this phenomenon is that, if carbon prices are higher than marginal mitigation cost, profits of ETS-covered power enterprises will be affected, which impels enterprises to react. Actually, (Kang et al., 2019) concluded that controlling the carbon price is beneficial for the encouragement of enterprises’ emission reductions.
Generally, the ECP will suffer potential increases in the coming years, for tightening quotas turn the carbon allowance into a deflationary asset. Therefore, in this paper, we assume that the ECP will increase by 10 CNY2018 per year based the level of 50 CNY2018 in 2020, so that in 2030, the ECP will be 150 CNY2018. The actual emission reductions for the next 10 years is derived on this basis, and combined with the projected thermal electricity production and newly installed capacity, final emission reductions for ETS-covered enterprises is estimated to be 3.27 billion tonnes CO2e, and 1.18 billion tonnes CO2e to be achieved by the selected five trajectories.
As shown in Figure 6, the global warming potential (GWP) takes the largest share for all five technique trajectories, the percentage of Sub-C units is 98.91%, 98.67% for Super-C units, and 98.66% for USC units, mono-Si even reaches 99.60%, DFIG is much lower, the corresponding value being 93.62%. Then, if we make a comparison on the endpoint level impact categories, we can find that the human health impact value takes a share of more than 98%, but it seems that the mono-Si is relatively human health friendly, with the lowest share of 98.32%, while the remaining four techniques have the percentage over 99%. Abbreviations for the terms are shown in Table 3.
FIGURE 6. LCIA results for different technical trajectories when producing 1 kW·h electricity. (A) Results for midpoint level impact categories. (B) Results for endpoint impact categories.
As shown in Figure 7, when producing 1 kW·h electricity, Sub-C units have the largest environmental cost, either DTE, DTH or GDPL. Specifically, the damage to human health is 1.66 CNY2018, the damage to ecosystems is around 1.70 CNY2018, and the GDP loss induced by rising temperatures is nearly 1.34E-03 CNY2018. In comparison, the cost of mono-Si and DFIG are much less, corresponding costs for mono-Si are 3.62E-03CNY2018, 5.82E-02CNY2018, 3.18E-05CNY2018, and 2.10E-01CNY2018, 1.18E-02CNY2018, 1.01E-05CNY2018 for DFIGURE Based on these, environmental benefits of the five mitigation trajectories are calculated and the results are as shown in Figure 8. In Figure 8, it is obvious that Track 4 has the largest environmental benefits: 1.66 CNY2018 for DTH, 1.64 CNY2018 for DTE and 1.31E-03 CNY2018 for GDPL, however, the emission reduction of Track four is not the largest, which is 0.820 kg CO2e compared to 0.825 kg CO2e for DFIG.
FIGURE 7. Monetization of different technical trajectories when producing 1 kW·h electricity. *Note that the actual value for GDPL is 1.0E-03 times of the data shown in this figure. Units for the three parameters are CNY2018.
FIGURE 8. Environmental benefits when producing 1 kW·h electricity for different tracks. *Note that, the actual value for
When planning the decarbonization roadmap, the objective is to maximize the environmental benefits. Nine constraints are included. As has been stated in the previous section, we utilize GA to solve the optimization problem. The final results are displayed in Figure 9.
The basic conclusion are: significant attention should be given on mono-Si and DFIG since their contributions to the overall emission reductions are the largest. Compared with TR 2, TR 3 should be given the priority. As shown in Figure 9, the optimal electricity production volume for the next 10 years are: 0 TW·h for TR 1, 179.70 TW·h for TR 2, 269.60 TW·h for TR 3, 675.58 TW·h for TR 4 and 702.50 TW·h for TR 5. Then, we transfer the electricity value into capacity volume, based on the assumed AUH for different technical approaches.
As a result, during the period of 2021–2030, the optimal newly installed capacity for mono-Si PV should be around 160.85 GW in total, DFIG is 167.26 GW; as for the former three trajectories, TR 1 contributes 0, TR 2 accounts for 42.79 GW, and this figure will be 64.19 GW for TR 3. Finally, under this decarbonization roadmap, the environmental benefits account to nearly 4.6 trillion CNY2018, which equals to over 5% of China’s 2018 GDP.
Compared with existing literatures, our results are reasonable and realistic. Namely, the mitigation amount in the next 10 years is estimated to be 3.27 billion tonnes CO2e, compared with the 3.00 billion tonnes CO2e derived by (Zhao et al., 2017). Concerning to the decarbonization pathways, former researches came up with a mitigation roadmap for Yunnan province by the year 2030 (Zhang et al, 2020b), featuring an increasing share of clean energy (e.g., hydropower, wind, even high efficiency coal-fired power units) in the power sector. Our results echo this proposal.
The results are subject to various uncertainties embodied in steps of the modeling process, for example, LCIA analysis, monetary valuation, etc. In this paper, we take three main aspects into consideration when performing the uncertainty analysis: (UA 1) LCIA analysis; (UA 2) monetarization factors; (UA 3) percentage change of the thermal power in total newly installed capacity from 2020 to 2030.
The LCIA can potentially induce a few uncertainties to the overall results. Since the c) emission reduction datasets are under constant review and validation, uncertainty in this area mainly lies in the selection of system boundary and the performance of LCIA. In this paper, considering that the system boundary for different trajectories are all from cradle-to-gate, the LCIA therefore contributes more to the uncertainty. Thus, the uncertainty range can be set based on the two endpoint level impact categories. Actually, we set the baseline results as the 50th, and select the 10th, 25th, 75th, 90th as the uncertainty scope.
Monetary factors can affect the decarbonization roadmap optimization since they are important parts of our objective function. The human health valuation factor is based on the willingness of humans to pay for eliminating a unit of DALY; obviously, this factor can be affected by the current and future state of personal finances. Furthermore, as shown in (Rauner et al., 2020), since the factor is obtained by meta-analysis of foreign cases, it is potentially inaccurate when applied to China. As for the monetization value of ecosystem damage, it is measured by the cost of restoring diminished habitat for a species, but the cost varies for the heterogeneity of species, which directly lead to the uncertainty. The uncertainty ranges of both factors are also set from 10th to 90th.
The change of thermal power share in newly installed capacity may impact the constraints of decarbonization roadmap optimization. The prospect of thermal power is quite fuzzy, support or opposition for further installation can be easily heard. We are of the view that further installation will slow down but would not stop. The uncertainty range is set from 10th to 90th, which means the share of thermal power in 2030 varies from 0.30% to 2.7%.
It is found that the decarbonization roadmap under the three uncertainties remains the same, which means the decarbonization roadmap is quite robust. However, due to the changing parameters of the objective function, corresponding environmental benefits varies.
As shown in Figure 10. 1) Focusing on UA 1, we find that the LCIA results seems to have a minor impact on the overall results: In the 10th case, the environmental benefit is around 0.92 trillion CNY2018, which is 79.97% lower than the baseline study (50th), the 25th case suffers a 49.98% decrease, the 75th case increases by 50% and the 90th case increases by 79.97%. These data are within the expected range of variation, we can then conclude that the final result is insensitive to the LCIA. 2) As for UA 2, or the monetary factors, it seems that it has larger impact on the overall results. The 10th, 25th and 75th cases perform much the same with reference to UA 1; however, the 90th case outperforms, which increases by 169.92% over the baseline study. It can be concluded that the employed monetary factors have larger impacts on the final result. 3) With regard to UA 3, it seems that the assumed share of thermal power capacity is completely insensitive to the final result.
FIGURE 10. Uncertainty analysis of environmental benefits. *Note that, 50th refers to the baseline study.
In short, among all the three factors, monetary factors have the most significant impact on the final result, while, the LCIA and the share of thermal power capacity are almost insensitive to the final result.
In a well-known report about the basis for futures and options market in 1985, four questions were raised to test whether there is a concrete basis for the derivative market: 1) the economic function, 2) co-movement with the spot market, 3) regulation tools’ abundance and 4) protection on investors’ rights. Here, we try to briefly analyze the basis for carbon futures trading in China based on this framework.
Generally, the economic functions of futures market mainly include hedging and price discovery (Daskalakis, 2018). According to the analysis shown in Section 2, we hold the view that as long as enough speculators participate in this market, the depth and liquidity of the futures market can be significantly improved, and either market efficiency or the pricing efficiency can be elevated. Given that the trading cost in the futures market is much lower than the spot market, hedgers (power producers in this study) can transfer the risk to speculators, then the economic functions can be realized. Some scholars put forward the idea that futures trading will not play a significant economic function in mature financial market (Xiao, 2020), for all available information is already incorporated into the spot price. Concerning the carbon futures market, it is relatively immature, so carbon futures trading is expected to play an important role in the realization of the economic function, which have been verified in the EU ETS (Rittler, 2012)).
Considering that the carbon futures product is much like financial futures, experience in China’s traditional derivative market can give a hint when researching the co-movement between carbon spot and carbon futures (Su and Xie, 2017). Generally, it is considered that China’s financial futures market will enlarge the volatility of spot market in the short term, but financial derivatives can help to stabilize the spot market and therefore contribute to risk prevention in the long run (Agriculture, 1985). Thus, we can infer that, once the carbon futures market has been established, the market will potentially suffer temporary volatility, but it will ultimately benefit the carbon spot market, which is desired by the regulators.
Though a very limited variety of derivatives are traded in China’s financial derivative market, after several years’ operation, a set of regulation tools had been developed. For instance, there are limitations on positions and a special report scheme for influential investors, which mean that a relatively complete regulation framework has been created in China. Therefore, it can be expected that abundant regulation tools will be available for the carbon futures market.
To some extent, protection of investors’ rights is an obvious flaw in China’s financial market. Since the hierarchy of laws for China’s futures market is relatively low, investors’ rights cannot be protected well (Zhou and Li, 2019; Xiao, 2020). However, since the “Law for futures” is under enactment, protection of investor’s right will be significantly improved in the future.
According to the above analysis, we generally hold the opinion that in the course of time, the economic function, regulation of the market and protection of investors’ rights will significantly improve. As a result, introducing carbon futures trading should be a promising financial innovation in China.
Before discussing concerns about specific futures contracts, we should note that most positions held by the participants will be closed out prior to maturity, thus delivery is unusual (John, 2013), which means the actual influence of specific contracts is quite limited. Nonetheless, some brief discussions related to the contract concerns are made in this paper out of academic rigor.
Generally, the exact nature of the futures agreement will be specified in the contracts, for example, the underlier, contract size, delivery arrangements, etc. (Schwager and Etzkorn, 2016) listed 12 representative trading details for the specifications of futures products. Contract size varies for different futures, too big or too small is inappropriate for participants: investors who wish to hedge small positions will be unable to do so if the contract size is too small, and the cost of trading will be too large. The correct size can be ascertained by analyzing the likely users. Delivery places and delivery month will be determined in the delivery arrangements, occasionally, there will be alternatives for the delivery locations, which are determined by the Exchange. Futures contracts are for delivery in a specific month, take the carbon futures traded in European Climate Exchange as example, the contracts are listed on a quarterly expiry cycle (March, June, etc.) (Daskalakis et al., 2011). Price limits and positions limits exist in many futures contracts. The former is designed to constrain the over-movement induced by speculation, the latter is to prevent market manipulation (John, 2013).
The realization of expected hedging and the price discovery function is partly dependent on the specification of futures contracts. Specifically, realization of hedging is related to the contract size. The market should enable investors to hedge their positions, no matter how large or small the hedging positions are. Thus, with appropriate set of the contract sizes, the hedging function can be better realized. On the other hand, the expiry date will affect the evolution of futures prices, which has been proved by (Daskalakis, 2018), whose basic conclusion was that the longer the expiry date, the closer the futures price more likely to follow the spot price. Since the carbon futures have been traded in EU ETS for 15 years, reasonable delivery arrangements of China’s carbon futures contracts can be attained through following foreign experiences.
In general, the contract specifications have limited influences on the realization of carbon futures’ economic function. Furthermore, the above analysis also gives support to the previous conclusion that the essence of carbon futures trading can be viewed as locking in the executive carbon price, which is the basis for this study.
In this paper, we present the optimal decarbonization roadmap for the ETS-covered power enterprises under the context of carbon futures trading, and corresponding environmental benefits are also displayed. Since the carbon futures trading scheme has not yet been established in China, few papers related to China’s carbon futures trading can be found, not to mention the pre-assessment of this scheme. Thus, this study is quite innovative in the field.
After analyzing the essence of carbon futures trading and its potential influences on power enterprises, we calibrate the effect of locking the executive carbon price. That is, the actual trading price for participants in the carbon futures market is pre-locked, though the exact price level varies on a yearly basis. Then, a model chain is put forward to calculate the optimal decarbonization roadmap and corresponding environmental benefits for the next 10 years.
Specifically, the OPDP model helps to derive the optimal emission reductions, with the basis of maximizing enterprises’ profits. According to our estimates, ETS-covered power enterprises should mitigate around 81.67 g CO2e when producing 1 kW·h electricity (around 15% of current emission level). To achieve this mitigation target, we assume that the upgrading of power production techniques and renewable energy investments, can contribute to nearly 36% of total emission reduction, and this paper takes five technical approaches (Sub-C, Super-C, USC, mono-Si PV and wind turbine DFIG) into consideration as the basis of mitigation pathways. 5 decarbonization trajectories are designed based on the environmental damage potential and industry practices. Specific LCIA analysis is conducted on the 5 decarbonization trajectories to derive the actual environmental benefits. Since the environmental impacts have different metrics, we transfer the environmental benefits into market value through monetization. Based on the work above, we finally plan the optimal decarbonization roadmap through the Genetic Algorithm.
Results show that in the next 10 years (2021–2030), 106.98 GW USC coal-fired units, 160.85 GW mono-Si and 167.26 GW DFIG wind capacity should be installed. Furthermore, the environmental benefits account to nearly 4.6 trillion CNY2018, which equals to over 5% of China’s 2018 GDP. The derived overall emission reduction is 3.27 billion tonnes CO2e, which is comparable with 3.00 billion tonnes CO2e estimated by previous studies. As a result, the final outcome is considered realistic.
Considering that no previous work in the field of pre-assessment on Chinese carbon futures trading has been carried out, the unique contribution of this study is filling this gap by deriving a potential decarbonization roadmap under the context of carbon futures trading for ETS-covered power enterprises in the next 10 years. However, there still exists some space for improvements, the most prominent, being a more accurate estimation on valuation factors, which is proved to be influential in the uncertainty analysis. Furthermore, some assumptions are relatively tight, like the low-carbon awareness of consumers, which assumes that consumers are willing to pay extra 0.014 CNY2018 for 1 kW·h green electricity; however, with the raising low-carbon awareness of Chinese people, greater paying-willingness is possible. Future studies should take these factors into account (cninfo, 2020a; International Renewable Energy Agency, 2019; Juchao Information Network, 2019a; cninfo, 2020b; Juchao Information Network, 2019b; Tong et al., 2017).
The original contributions presented in the study are included in the article/supplementary material, further inquiries can be directed to the corresponding authors.
JL: Conceptualization, funding acquisition, data curation, writing-original draft, writing-review and editing. XL: Data curation, funding acquisition. CW: Funding acquisition, investigation. JD: Software, writing-review and editing. SC: Data curation. YuC: Conceptualization, writing-review and editing. YiC: Conceptualization, data curation, supervision, writing-original draft, writing-review and editing.
This work is supported by the Enterprise Management Consulting Services Project in 2022 for State Grid Jiuquan Power Supply Company (No. SGGSJQ00FCWT2200097). And the State Grid Corporation Central China Division 2022 Management Consulting Project, Project No. SGTYHT/21-WT-225.
Authors JL, XL, and CW were employed by the company State Grid Jiuquan Power Supply Company and author JD was employed by the company ZTE Corporation.
The remaining authors declare that the research was conducted in the absence of any commercial or financial relationships that could be construed as a potential conflict of interest.
The authors declare that this study received funding from State Grid Jiuquan Power Supply Company and CentralChina Branch of State Grid Corporation of China. The funders had the following involvement in the study: investigation, data collection, manuscript writing and reviewing and decision to publish.
All claims expressed in this article are solely those of the authors and do not necessarily represent those of their affiliated organizations, or those of the publisher, the editors and the reviewers. Any product that may be evaluated in this article, or claim that may be made by its manufacturer, is not guaranteed or endorsed by the publisher.
1Back to 2015, the State Council of China already proposed to research on the establishment of an innovative futures exchange with carbon emissions as the first trading product. In 2018, China Securities Regulatory Commission put forward the idea of researching on the establishment of carbon emissions futures trading after an important meeting. Recently, in February 2019 and May 2020, similar ideas were also mentioned in the supporting policies to the Guangdong-Hongkong-Macao Greater Bay Area.
2Executive carbon price (ECP) means the carbon price on which the allowances trading happens.
3See: http://www.mee.gov.cn/gkml/hbb/bwj/201512/t20151215_319170.htm, Chinese version.
4As shown in Figure 3, more and more renewable energy capacities, in which PV and Wind take most of the share, are being installed in China. Actually, in the context of limiting coal utilization, more and more power and electricity enterprises are considering using PV and Wind to replace conventional coal-fired power units in future newly installed capacities. Further, the cost of PV and Wind has nearly decreased to the level of coal (IRENA, 2019).
5See: https://mp.weixin.qq.com/s/-PLujVDIMLNSINEJMGhaDg (Chinese Version).
6Equals to the “growth rate of electricity/growth rate of GDP.”
7National Development and Reform Commission, National Energy Administration, 2017. See: http://www.gov.cn/xinwen/2017-04/25/content_5230568.htm (Chinese Version).
Agriculture, C. O. (1985). A study of the effects on the economy of trading in futures and options. Available at: https://books.google.com.hk/books?id=CH_pAAAAMAAJ&printsec=frontcover&hl=zh-CN#v=onepage&q&f=false (Accessed July 13, 2020).
Bergesen, J. D., Heath, G. A., Gibon, T., and Suh, S. (2014). Thin-film photovoltaic power generation offers decreasing greenhouse gas emissions and increasing environmental co-benefits in the long term. Environ. Sci. Technol. 48, 9834–9843. doi:10.1021/es405539z
Cai, W., Hui, J., Wang, C., Zheng, Y., Zhang, X., Zhang, Q., et al. (2018). The lancet countdown on PM 2.5 pollution-related health impacts of China’s projected carbon dioxide mitigation in the electric power generation sector under the paris agreement: A modelling study. Lancet Planet. Health 2, e151–e161. doi:10.1016/s2542-5196(18)30050-0
Celik, I., Song, Z., Cimaroli, A. J., Yan, Y., Heben, M. J., and Apul, D. (2016). Life Cycle Assessment (LCA) of perovskite PV cells projected from lab to fab. Sol. Energy Mat. Sol. Cells 156, 157–169. doi:10.1016/j.solmat.2016.04.037
Chevallier, J. (2009). Carbon futures and macroeconomic risk factors: A view from the EU ETS. Energy Econ. 31 (4), 614–625. doi:10.1016/j.eneco.2009.02.008
cninfo (2020a). Available at: http://www.cninfo.com.cn/new/disclosure/detail?plate=sse&orgId=gssh0600011&stockCode=600011&announcementId=1207441578&announcementTime=2020-04-01 (Accessed July 16, 2020).
cninfo (2020b). Available at: http://www.cninfo.com.cn/new/disclosure/detail?plate=sse&orgId=gssh0600795&stockCode=600795&announcementId=1207491643&announcementTime=2020-04-14 (Accessed July 16, 2020).
Cui, R., Hultman, N., Jiang, K., McJeon, H., Yu, S., Cui, D., et al. (2020). A hight ambition coal phaseout in China: Feasible strategies through a comprehensive plant-by-plant assessment. College Park, Maryland: Center for Global Sustainability, 37.
Daskalakis, G., Ibikunle, G., and Diaz-Rainey, I. (2011). “The CO2 trading market in europe: A financial perspective,” in Financial aspects in energy, 51–67.
Daskalakis, G. (2018). Temporal restrictions on emissions trading and the implications for the carbon futures market: Lessons from the EU emissions trading scheme. Energy Policy 115, 88–91. doi:10.1016/j.enpol.2018.01.008
Di, X., Nie, Z., Yuan, B., and Zuo, T. (2007). Life cycle inventory for electricity generation in China. Int. J. Life Cycle Assess. 12 (4), 217–224. doi:10.1065/lca2007.05.331
Fatemi Aghda, S. A., and Mirfakhraei, M. (2020). Improved routing in dynamic environments with moving obstacles using a hybrid Fuzzy-Genetic algorithm. Future Gener. Comput. Syst. 112, 250–257. doi:10.1016/j.future.2020.05.024
Gao, J. G. (2006). Product quality competition and enterprisers' decision under multidimensional model. Shanxi, China: Journal of Shanxi Finance Economics University.
Gao, P., Chen, W., and He, J. (2004). Marginal carbon abatement cost in China. Qinghua Daxue Xuebao/Journal Tsinghua Univ. 44 (9), 1192–1195. doi:10.16511/j.cnki.qhdxxb.2004.09.011
Gao, T., Li, G. X., Xu, M. M., Wang, X. Y., Liang, F. C., Zeng, Q., et al. (2015). Health economic loss evaluation of ambient PM2.5 pollution based on willingness to pay. J. Environ. Health 32, 697–700. [in Chinese]. doi:10.16241/j.cnki.1001-5914.2015.08.011
Gerbinet, S., Belboom, S., and Léonard, A. (2014). Life cycle analysis (LCA) of photovoltaic panels: A review. Renew. Sustain. Energy Rev. 38, 747–753. doi:10.1016/j.rser.2014.07.043
Han, C., Wang, S., Li, Z., Chen, C., Hou, J., Xu, D., et al. (2018). Bioactivity-guided cut countercurrent chromatography for isolation of lysine-specific demethylase 1 inhibitors from Scutellaria baicalensis Georgi. Anal. Chim. Acta 38, 59–68. doi:10.1016/j.aca.2018.01.014
Holland, J. H. (1997). Hidden order: How adaptation builds complexity. Bull. Sci. Technol. Soc. 17, 205. [Chinese Version].
Huang, B., Zhao, J., Chai, J., Xue, B., Zhao, F., and Wang, X. (2017). Environmental influence assessment of China’s multi-crystalline silicon (multi-Si) photovoltaic modules considering recycling process. Sol. Energy 143, 132–141. doi:10.1016/j.solener.2016.12.038
Huettner, F., and Sunder, M. (2012). Axiomatic arguments for decomposing goodness of fit according to Shapley and Owen values. Electron. J. Stat. 6, 1239–1250. doi:10.1214/12-ejs710
Huijbregts, M. A., Steinmann, Z. J., Elshout, P. M., Stam, G., Verones, F., Vieira, M., et al. (2017). ReCiPe2016: A harmonised life cycle impact assessment method at midpoint and endpoint level. Int. J. Life Cycle Assess. 22 (2), 138–147. doi:10.1007/s11367-016-1246-y
Juchao Information Network (2019a). GD power development Co., Ltd. Available at: http://www.cninfo.com.cn/new/disclosure/detail?plate=sse&orgId=gssh0600795&stockCode=600795&announcementId=1207491643&announcementTime=2020-04-14 (Accessed July 16, 2020).
Kalaitzoglou, I. A., and Ibrahim, B. M. (2015). Liquidity and resolution of uncertainty in the European carbon futures market. Int. Rev. Financial Analysis 37, 89–102. doi:10.1016/j.irfa.2014.11.006
Kang, K., Zhao, Y., Zhang, J., and Qiang, C. (2019). Evolutionary game theoretic analysis on low-carbon strategy for supply chain enterprises. J. Clean. Prod. 230, 981–994. doi:10.1016/j.jclepro.2019.05.118
Kim, E.-S. (2014). Imagining future Korean carbon markets: Coproduction of carbon markets, product markets, and the Government. J. Environment. Pol. Plan. 16, 459–477.
Kirke, L. (2012). Carbon derivatives and their application within an Australian context. SSRN J. doi:10.2139/ssrn.1942711
Lamperti, F., Bosetti, V., Roventini, A., and Tavoni, M. (2019). The public costs of climate-induced financial instability. Nat. Clim. Chang. 9 (11), 829–833. doi:10.1038/s41558-019-0607-5
Leconte, A., and Pagano, T. (2010) Carbon derivatives: A destabilizing or a virtuous mechanism to build a carbon-free economy? The example of the European CO2 trading scheme. The example of the European CO2 trading scheme. USAEE-IAEE Working Paper (10-058).
Liang, X., Wang, Z., Zhou, Z., Huang, Z., Zhou, J., and Cen, K. (2013). Up-to-date life cycle assessment and comparison study of clean coal power generation technologies in China. J. Clean. Prod. 39, 24–31. doi:10.1016/j.jclepro.2012.08.003
Matthews, H. D., Solomon, S., and Pierrehumbert, R. (2012). Cumulative carbon as a policy framework for achieving climate stabilization. Phil. Trans. R. Soc. A 370, 4365–4379. doi:10.1098/rsta.2012.0064
Nordhaus, W. D. (2017). Revisiting the social cost of carbon. Proc. Natl. Acad. Sci. U. S. A. 114, 1518–1523. doi:10.1073/pnas.1609244114
Ortas, E., and Álvarez, I. (2016). The efficacy of the European Union Emissions Trading Scheme: Depicting the co-movement of carbon assets and energy commodities through wavelet decomposition. J. Clean. Prod. 116, 40–49. doi:10.1016/j.jclepro.2015.12.112
Partridge, I., and Gamkhar, S. (2012). A methodology for estimating health benefits of electricity generation using renewable technologies. Environ. Int. 39, 103–110. doi:10.1016/j.envint.2011.10.003
Rannou, Y., and Barneto, P. (2016). Futures trading with information asymmetry and OTC predominance: Another look at the volume/volatility relations in the European carbon markets. Ener. Econ. 53, 159–174.
Rauner, S., Bauer, N., Dirnaichner, A., Dingenen, R. V., Mutel, C., and Luderer, G. (2020). Coal-exit health and environmental damage reductions outweigh economic impacts. Nat. Clim. Chang. 10 (4), 308–312. doi:10.1038/s41558-020-0728-x
Rittler, D. (2012). Price discovery and volatility spillovers in the European union emissions trading scheme: A high-frequency analysis. J. Bank. Finance 36, 774–785. doi:10.1016/j.jbankfin.2011.09.009
Sabbaghi, O., and Sabbaghi, N. (2011). Carbon financial Instruments, thin trading, and volatility: Evidence from the Chicago climate exchange. Q. Rev. Econ. Finance 51 (4), 399–407. doi:10.1016/j.qref.2011.07.004
Schwager, J. D., and Etzkorn, M. (2016). A complete guide to the futures market. doi:10.1002/9781119209713
Si, R. G., Jia, Y. G., Cao, D., and Liu, H. Y. (2019). Analysis on key factors of new energy consumption and study on solutions. Energy Conservation Environ. Prot. 10, 86–87. [Chinese Version].
Siddiqui, O., and Dincer, I. (2017). Comparative assessment of the environmental impacts of nuclear, wind and hydro-electric power plants in ontario: A life cycle assessment. J. Clean. Prod. 164, 848–860. doi:10.1016/j.jclepro.2017.06.237
Stefan, M., and Wellenreuther, C. (2020). London vs. Leipzig: Price discovery of carbon futures during Phase III of the ETS. Econ. Lett. 188, 108990. doi:10.1016/j.econlet.2020.108990
Su, L. Y., and Xie, X. W. (2017). The development path of carbon market and the realization of function: An analysis based on the particularity of carbon emission right. J. Guangdong Univ. Finance Econ. 150, 24–31. [Chinese Version].
Tan, X.-P., and Wang, X.-Y. (2017). Dependence changes between the carbon price and its fundamentals: A quantile regression approach. Appl. Energy 190, 306–325. doi:10.1016/j.apenergy.2016.12.116
Tol, R. S. J. (2010). The economic impact of climate change. Perspekt. Der Wirtsch. 1, 13–37. doi:10.1111/j.1468-2516.2010.00326.x
Tong, Y. E., Guan, Z. M., Tao, J., and You, Q. U. (2017). Dynamic optimization and coordination about joint emission reduction in a supply chain considering consumer preference to low carbon and reference low-carbon level effect. Chin. J. Manag. Sci. 25, 52–61. doi:10.16381/j.cnki.issn1003-207x.2017.10.006
Uddin, G. S., Hernandez, J. A., Shahzad, S. J. H., and Hedström, A. (2018). Multivariate dependence and spillover effects across energy commodities and diversification potentials of carbon assets. Energy Econ. 71, 35–46. doi:10.1016/j.eneco.2018.01.035
Wang, W. Q. (2016). Research on the development of Chinese carbon futures. Nanjing Forestry University. Master dissertation. [Chinese Version].
Wang, T., Jiang, Z., Zhao, B., Gu, Y., Liou, K. N., Kalandiyur, N., et al. (2020). Health co-benefits of achieving sustainable net-zero greenhouse gas emissions in California. Nat. Sustain. 3, 597–605. doi:10.1038/s41893-020-0520-y
Wen, X., Bouri, E., and Roubaud, D. (2017). Can energy commodity futures add to the value of carbon assets? Econ. Model. 62, 194–206. doi:10.1016/j.econmod.2016.12.022
Xiao, G. (2020). Transformation of China's capital market. Beijing, China: China CITIC Press. [Chinese Version].
Yang, K., and Liu, X. Y. (2016). Supply chain decision-making of carbon emission based on consumer low-carbon preferences and internal financing. Syst. Eng. 34 (11), 87–97.
Yang, W., Pan, Y., Ma, J., Yang, T., and Ke, X. (2020). Effects of allowance allocation rules on green technology investment and product pricing under the cap-and-trade mechanism. Energy Policy 139, 111333. doi:10.1016/j.enpol.2020.111333
Ye, T., Guan, Z. M., Tao, J., and Qu, Y. (2017). Dynamic optimization and coordination about joint emission reduction in a supply chain considering consumer preference to low carbon and reference low-carbon level effect. Chinese J. Manage. Sci. 25, 52–61.
Yeh, W. C., He, M. F., Huang, C. L., Tan, S. Y., Zhang, X., Huang, Y., et al. (2020). New genetic algorithm for economic dispatch of stand-alone three-modular microgrid in DongAo Island. Appl. Energy 263, 114508. doi:10.1016/j.apenergy.2020.114508
Yu, G., and Guo, Y. (2019). Development status and prospects of clean and efficient coal-fired power technology in China. E3S Web Conf. 118, 02059. doi:10.1051/e3sconf/201911802059
Yu, X., Wu, Z., Wang, Q., Sang, X., and Zhou, D. (2020). Exploring the investment strategy of power enterprises under the nationwide carbon emissions trading mechanism: A scenario-based system dynamics approach. Energy Policy 40, 111409. doi:10.1016/j.enpol.2020.111409
Yuan, D. (2019). Research on the development of EU carbon financial market and its experience for reference to China. Beijing, China: Master CASS Graduate School.
Zhang, Y.-J., Wang, A.-D., and Tan, W. (2015). The impact of China's carbon allowance allocation rules on the product prices and emission reduction behaviors of ETS-covered enterprises. Energy Policy 86, 176–185. doi:10.1016/j.enpol.2015.07.004
Zhang, X., Chen, Y., Jiang, P., Liu, L., Xu, X., and Xu, Y. (2020a). Sectoral peak CO2 emission measurements and a long-term alternative CO2 mitigation roadmap: A case study of yunnan, China. J. Clean. Prod. 247, 119171. doi:10.1016/j.jclepro.2019.119171
Zhang, Y. J., Wang, A. D., and Tan, W. (2020b). The impact of China's carbon allowance allocation rules on the product prices and emission reduction behaviors of ETS-covered enterprises. Energy Policy 86, 176–185. doi:10.1016/j.enpol.2015.07.004
Zhao, R., Min, N., Geng, Y., and He, Y. (2017). Allocation of carbon emissions among industries/sectors: An emissions intensity reduction constrained approach. J. Clean. Prod. 142, 3083–3094. doi:10.1016/j.jclepro.2016.10.159
Zhou, Y., Bao, M., Chen, X., and Xu, X. (2016). Co-op advertising and emission reduction cost sharing contracts and coordination in low-carbon supply chain based on fairness concerns. J. Clean. Prod. 133, 402–413. doi:10.1016/j.jclepro.2016.05.097
Zhou, K., and Li, Y. W. (2019). Carbon finance and carbon market in China: Progress and challenges. J. Clean. Prod. 214, 536–549.
Keywords: carbon futures trading, life cycle impact assessment, genetic algorithm, decarbonization roadmap, carbon mitigation
Citation: Li J, Li X, Wei C, Deng J, Chen S, Cao Y and Chen Y (2023) Decarbonization roadmap for China’s energy sector in the context of carbon futures trading: An energy-environment-economy assessment perspective. Front. Energy Res. 10:1026860. doi: 10.3389/fenrg.2022.1026860
Received: 24 August 2022; Accepted: 31 October 2022;
Published: 12 January 2023.
Edited by:
Pietro Bartocci, Spanish National Research Council (CSIC), SpainReviewed by:
Huamei Duan, Chongqing University, ChinaCopyright © 2023 Li, Li, Wei, Deng, Chen, Cao and Chen. This is an open-access article distributed under the terms of the Creative Commons Attribution License (CC BY). The use, distribution or reproduction in other forums is permitted, provided the original author(s) and the copyright owner(s) are credited and that the original publication in this journal is cited, in accordance with accepted academic practice. No use, distribution or reproduction is permitted which does not comply with these terms.
*Correspondence: Yuwei Cao, eXdjYW9AaHVzdC5lZHUuY24=; Yingqi Chen, MTc4NjI5Nzg4NzJAMTYzLmNvbQ==
Disclaimer: All claims expressed in this article are solely those of the authors and do not necessarily represent those of their affiliated organizations, or those of the publisher, the editors and the reviewers. Any product that may be evaluated in this article or claim that may be made by its manufacturer is not guaranteed or endorsed by the publisher.
Research integrity at Frontiers
Learn more about the work of our research integrity team to safeguard the quality of each article we publish.