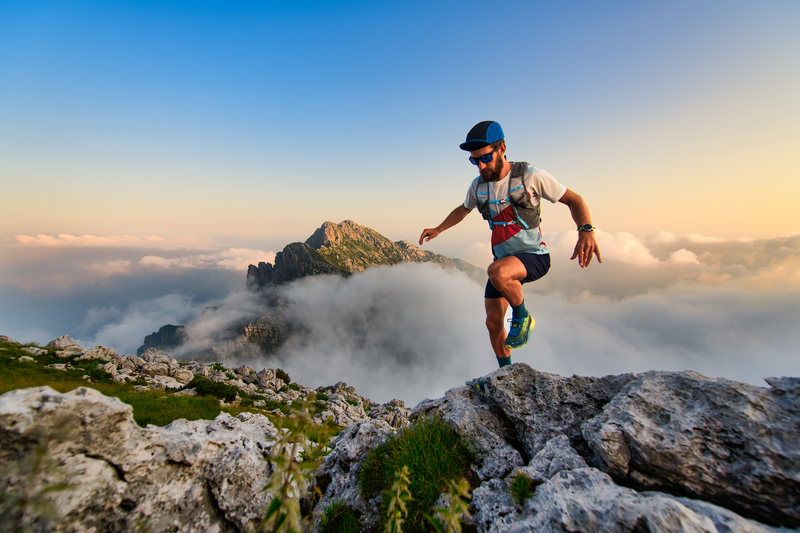
95% of researchers rate our articles as excellent or good
Learn more about the work of our research integrity team to safeguard the quality of each article we publish.
Find out more
ORIGINAL RESEARCH article
Front. Energy Res. , 30 September 2022
Sec. Smart Grids
Volume 10 - 2022 | https://doi.org/10.3389/fenrg.2022.1020607
This article is part of the Research Topic Planning, Operation and Control of Modern Power System with Large-scale Renewable Energy Generations View all 11 articles
The integrated energy system (IES) is recognized as a promising energy utilization approach enabled to both improve the energy efficiency and reduce pollutant emissions. Although the economic-environmental dispatch (EED) problem of the IES has been widely studied, the fact that the IES is operated under off-design conditions, having a significant influence on the efficiency of energy devices, is neglected usually, resulting in the scheduled operations, for the IES could not be accurate enough in many actual situations. This study investigates the EED problem of the IES under off-design conditions. Technically, by integrating an efficiency correction process into the traditional energy hub (EH) model, a dynamic energy hub (DEH) model is first formulated for adapting itself to variable energy conversion efficiencies. Then, a deep neural network (DNN)-based efficiency correction method is proposed to predict and correct the time-varying efficiency of energy devices based on three main off-design conditions including the load rate, air temperature, and pressure. A multi-objective EED model is finally formulated for the IES, with the framework of the DEH model, aiming at establishing a trade-off between operational cost and emitted pollutants. Case studies show that the proposed approach helps in enhancing the accuracy of IES dispatch while having a good performance in both the operational cost and pollutant emission reduction.
With the increasing problem of environmental pollution and energy scarcity, both energy transformation and upgrading have received extensive attention worldwide (Jin et al., 2021). As one of the important energy utilization ways, the integrated energy system (IES) has been regarded as the development direction of the future energy field, which promotes the synergy and complementarity among multi-energy carriers (e.g., electricity, gas, and heating) (Lin et al., 2021), having the advantages of enhancing the energy efficiency and reducing dependence on the fossil fuels. Based on this, aiming to promote effective coordination among multi-energy carriers, the optimal dispatch of the IES has become a prevalent research hotspot in academia and industries (Martínez Ceseña and Mancarella, 2019).
The optimal dispatch for the multi-energy systems has been studied extensively, and some representative works have also been reported. Bartnik et al. (2022) investigated the economic performances of the combined heating and power (CHP) systems, and an economic-effective dispatch model was developed. A dispatch model was proposed for the IES to reduce energy cost (Yu et al., 2019). On the other hand, the environmental consideration is another important concern of the multi-energy system dispatch. To this, the economic-environmental dispatch (EED) problem of the multi-energy systems has been introduced and is usually formulated as a multi-objective optimization problem to pursue a trade-off between the economic and environmental benefits. For instance, an EED model was proposed by Dougier et al. (2021), Sarfi and Livani (2018), and Nandimandalam et al. (2022) for microgrids including certain energy conversion and storage units, aiming to minimize the operational cost and pollutant emissions. Guo et al. (2022) studied the optimal economic operation problem for the IES, and the environmental consideration was modeled into a constraint. Msigwa et al. (2022) provided an EED framework that functioned as accommodating to the high penetration access of wind power. A multi-objective optimization model was developed by Xu et al. (2018), aiming to obtain the minimum fossil fuels and pollutant emissions of the IES, considering stochastic wind and solar power. Although the EED problem for multi-energy systems has been studied extensively in the aforementioned works, they all ignore the impact of the off-design conditions on the efficiency of energy devices and assume the latter is constant. In fact, the off-design conditions, such as the load rate (Zong et al., 2022), air temperature (Akpan and Fuls, 2019), and pressure (Gong et al., 2021), have a significant impact on the device efficiency and make it variable. As a result, the scheduled operations based on this constant device efficiency are essentially deviated from the true ones, and therefore, the operational results could also be inaccurate (Mu et al., 2022).
In the literature, several methods have been reported to deal with the impact of variable off-design characteristics on the efficiency of energy devices. In general, the existing methods can be classified into the following two streams: 1) the mathematical model-based method and 2) the data-driven-based method. In the first stream, the polynomial fitting method was used by Zheng et al. (2018) to model the energy device efficiency. However, the presented model was a nonlinear programming problem, which was easy to fall into the suboptimal or even infeasible solution. To address this issue, the nonlinear formulation of the device efficiency was piecewise linearized via approximate treatment (Almassalkhi and Towle, 2016). The piecewise linear (PWL) approach was also employed to modify the efficiency of energy devices, and a mixed-integer linear programming model was formulated by Huang et al. (2020). However, following Shao et al. (2020), the mathematical model-based methods are hard in terms of ensuring the accuracy of the calculated solutions and the computational efficiency. To this end, in the second stream, the data-driven-based method is studied further. In particular, the machine learning (ML) method is one of the most widely used methods. Based on the collected historical off-design conditions’ data, many representative ML approaches can be used to predict the variable device efficiencies, such as polynomial regression (Li and Yao, 2021), support vector machines (Liu et al., 2020), and deep neural network (DNN) (Ghimire et al., 2019). The DNN has remarkable performance in both the computational accuracy and speed for nonlinear parameter forecast and has been applied in many machines’ intelligence fields, such as image recognition (Chen S. et al., 2022), parameter forecast (Browell and Fasiolo, 2021), and system control (Li et al., 2021).
Based on the aforementioned discussion, this article focuses on the optimal dispatch of the IES and is intended to study the EED problem for the IES, considering the off-design conditions. Compared to the existing works (Sarfi and Livani, 2018; Xu et al., 2018; Yu et al., 2019; Dougier et al., 2021; Bartnik et al., 2022; Guo et al., 2022; Msigwa et al., 2022; Nandimandalam et al., 2022), the main novelty of this study is to take into account the impact of the off-design conditions on the energy device efficiency. The primary contribution of this article is to formulate an energy hub model, which enabled to operate with the time-varying energy device efficiency, named as the dynamic energy hub (DEH) model. The key idea of such a formulation is to incorporate an efficiency correction process into the traditional energy hub (EH) model. The second contribution of this article is to propose a DNN-based efficiency correction method, in which three main off-design conditions (i.e., load rate, air temperature, and pressure) are considered and taken as the input set of the designed DNN well, and the corrected energy device efficiency is the output. Moreover, to validate the benefits of considering the off-design conditions on the IES’s dispatch, a multi-objective EED model with multi-scale operation considerations including operational economic and environmental impacts is developed for the IES under the framework of the DEH model.
The rest of this article is structured as follows. The overview of EED for the IES considering off-design conditions is described in Section 2. The DEH model is established in Section 3, and the DNN-based device efficiency correction method is developed in Section 4. In Section 5, the multi-objective EED model is introduced. Case studies are applied to verify the proposed method in Section 6, and the conclusion is drawn in Section 7.
Figure 1 illustrates a typical structure of an IES. Various energy devices (e.g., gas boiler (GB), CHP, and battery (BAT)) link the electricity system, gas system, and heating system together in the IES (Jin et al., 2016). Among them, the electrical loads are supplied by the electricity system, CHP, and BAT, and the CHP and GB supply the thermal loads. For the dispatch application, an IES is usually modeled into an energy hub framework based on the physical link topology of the IES.
The ideas of the proposed EED for the IES considering the off-design conditions are depicted in Figure 2. Taking into account the impact of off-design conditions on the efficiency of energy devices, the traditional EH model is remodeled as a DEH model that can operate with the time-varying device efficiency by integrating with an efficiency correction process. In the daily operation of the IES, the energy device efficiency is predicted and corrected in the efficiency correction process, based on the proposed DNN-based efficiency correction method, which is a data-driven method and will be introduced in detail in Section 3. Afterward, a multi-objective EED model is developed based on the obtained DEH model and is solved to realize a trade-off among multi-scale operational considerations over a finite operation horizon, that is, the operational cost and environmental impact reflected by the emitted pollutants.
Based on this typical IES structure shown in Figure 1, the diagram of the traditional EH model can be presented in Figure 3A. With the consideration of variable off-design conditions, the energy device efficiency varies under their operation conditions, such as the load rate and other environmental-related factors (e.g., air temperature and pressure); thus, it is necessary to reformulate the traditional EH model to adapt it to the time-varying energy device efficiency. For this purpose, we plan to incorporate an efficiency correction process into the traditional EH model, as shown in Figure 3B, to develop the DEH model. With the added efficiency correction sector, the energy device efficiency can be adjusted with the variation of the off-design conditions. In this article, the efficiency correction process is functioned by the proposed DNN-based efficiency correction method.
FIGURE 3. Typical topology model: (A) traditional EH model and (B) DEH model with the correction of the energy device efficiency.
The EH model is a linear multiple-input and multiple-output unit, where various energy sources are generated, converted, stored, and consumed, strengthening the integration of multiple energy carriers. The mathematical formulation for the traditional EH model, for a typical structure, as shown in Figure 3A, is presented in model (1).
In model (1), Pe,t and Pg,t denote the input electricity and gas powers, respectively, and Le,t and Lh,t represent the electrical and thermal loads, respectively. ηCHP, ηCHP,h, and ηGB are the electrical efficiency of CHP, thermal efficiency of CHP, and the efficiency of GB, respectively. The dispatch factor is represented by vt∈[0,1], which defines the proportion of gas input to CHP and GB. PBAT,t is the charging/discharging power of BAT at time t.
As the IES is always operated under the off-design conditions, the device efficiency in the EH model is not constant but time-varying, which renders the EH model a multivariable nonlinear system (Chen M. et al., 2022). To address this challenge, the DEH model is modeled in this article, as shown in Figure 3B; the efficiency correction process is embedded in the EH model. ηCHP,t, ηCHP,h,t, and ηGB,t are the electrical efficiency of CHP, thermal efficiency of CHP, and the efficiency of GB at time t, respectively. The coupling matrix of the DEH model is expressed as follows:
The efficiency correction process with execution on the basis of unit time interval dynamically calculates and corrects the energy device efficiency in the EH model based on three time-varying off-designs, including the actual load rate, air temperature, and pressure. Therefore, the formulated DEH model is capable of accurately simulating the operational environment of the IES under the variable off-design conditions.
The off-design conditions have a significant impact on the efficiency of energy devices. Considering three main off-design conditions, namely, the load rate, air temperature, and pressure, the efficiency of energy devices can be modeled as a function of the aforementioned three elements as follows:
where ηi,t denotes the efficiency of the energy device i at time t. Ni,t denotes the load rate of the energy device i at time t. Ti,t and Fi,t denote the air temperature and pressure at time t, respectively.
Due to the strong nonlinearity relationship between the energy device efficiency and the off-design conditions (Mu et al., 2022), the mathematical model-based method is difficult to achieve accurate predictions and is prone to overfitting and dimensionality curse. In this section, the DNN method (Ghimire et al., 2019) is employed to forecast the efficiency of energy devices.
A DNN framework is designed first in this article, which is a fully connected neural network with five layers, as presented in Figure 4. The designed DNN framework exhibits the best trade-off between the prediction speed and accuracy when it contains three hidden layers with six, five, and three neurons. The considered three off-design conditions are set as the input data set, and the device efficiency is the forecasted output. More specifically, the DNN model is formulated as follows:
where hl is the output vector of the l-th layer. g( ) is the activation function. Wl and bl-1 denote the connection weight matrixes. Model (4) represents the input sequence of the DNN. Model (5) describes the mathematical principles of forward propagation. Model (6) represents the output sequence of the DNN.
As shown in Figure 4, each layer is interconnected through weights (Wl) and biases (bl). The activation function further introduces nonlinearity into the hidden layers. The sigmoid function is selected as the activation function, given in Eq. 7, which can alleviate the gradient disappearance and improve the convergence rate (Ravnik and Hriberšek, 2019).
After constructing the DNN model, the loss function is designed to guide the training. The mean square error between the obtained efficiency of energy devices and the actual value is defined as the loss function hloss:
The training process is performed by tuning the parameters of the DNN model to minimize the loss of the given training data, as given in model (9). Moreover, we apply the Levenberg–Marquardt algorithm (Long et al., 2020) to solve the problem in model (9), which is effective for the large-scale data set and makes the probability of falling into a local minimum much reduced.
where floss,k is the loss of the lth layer in the training.
Moreover, the efficiency of energy storage devices primarily varies with long-term seasonal factors, and the effects on daily time-scale dispatch are ignorable (Mu et al., 2022). Thus, the variable off-design characteristics of energy storage devices are not taken into account in this article.
In this article, the IES is operated with multi-scale operational considerations, that is, the operational economic and environmental impact, under the off-design conditions. A multi-objective EED model is developed in this section for the IES, with two mutually exclusive objectives, that is, 1) to minimize the IES’s operational costs over a finite dispatching horizon and 2) to minimize the pollutant emissions. The decision-making variables in the proposed multi-objective EED model include purchasing electricity Pe,t, purchasing gas power Pg,t, output power Pi,t of the energy conversion device i, BAT charging power PC,t, and BAT discharging power PD,t.
The first objective is referred to the operational economic consideration and is formulated to minimize the operational cost (fope) composed of the electricity purchase cost (Ce) and gas purchase cost (Cg) as follows:
where T and ∆t are the total dispatching period and unit dispatching period, respectively. ce,t and cg denote the unit prices of purchasing electricity and gas, respectively.
The second objective, that is, the environmental consideration, is to reduce the overall pollutant emissions (femi) caused by electricity usage (Ee) and gas usage (Eg) and is expressed as follows:
where NPG represents the types of pollutant emissions from electricity usage, including CO2, CO, SO2, and NOx. χe,pg represents the emission factor of the pg-type pollutant. χg denotes the emission factor of gas usage. Only CO2 is taken into consideration in the pollutant emissions from gas usage due to the clean nature of gas (Lin et al., 2018).
The proposed multi-objective EED model for the IES is subjected to the following constraints:
The DEH model describes the coupling relationship between input and output power and adjusts the efficiency of energy devices dynamically by the DNN-based efficiency correction method. The energy balance constraint defined in the DEH model should be satisfied, as formulated in Eqs 2, 3.
The energy conversion devices in this article include CHP and GB, and constraints are shown as follows.
where Pin,i,t represents the input power of the energy conversion device i at time t. Pi,cap is the capacity of the energy conversion device i.
where WBAT,t and WBAT,t+1 indicate the energy stored in BAT before and after charging and discharging, respectively. ηC and ηD are the charging and discharging efficiencies of BAT. σ and WBAT,cap are the self-discharge rate and capacity of BAT, respectively. PC,max and PD,max are the maximum charging and discharging power, respectively. Wstart and Wend are the initial and final energy stored in BAT during 1 day (24 h), respectively.
The purchasing electrical power should not exceed the maximum tie-line power Pgrid,cap:
The non-dominated sorting genetical algorithm (NSGA-II) (Deb et al., 2002) is utilized in the MATLAB platform to solve the formulated multi-objective EED model in Section 5. The NSGA-II has strong performance in finding the Pareto frontier well of a multi-objective optimization problem and is one of the most recognized multi-objective evolutionary algorithms. With the obtained non-dominated solutions, the fuzzy decision-making criteria (Sakawa and Yano, 1985), with simplicity and capability in accounting for IES operators’ preferences, are then employed to select and output the optimal compromise solution.
The typical IES presented in Figure 1 is considered as an example. The electrical and thermal loads, air temperature, and pressure curves in a typical summer day are depicted in Figures 5, 6. The unit prices of purchasing electricity and gas are shown in Table 1. The emission factors of CO2, CO, SO2, and NOx from electricity usage, the emission factors of CO2 from gas usage, and the maximum tie-line power are shown in Table 2 (Lin et al., 2018). The technical parameters of devices are presented in Table 3 (Mu et al., 2022).
We set two benchmarks to comparatively validate the advantages of the proposed EED method as follows:
Case I. The EED method based on the constant efficiency EH model.
Case 2. The EED method based on the DEH model. In addition, different numbers of training iterations (IT) of DNNs are considered, and accordingly, multiple sub-cases are set. When IT is quite large enough (e.g., 500), the loss function values are approximately zero and DNNs converge completely, as shown in Figure 7. At this point, the dispatch results are considered accurate enough to serve as a reference for comparison with other cases.The optimal compromise results in two cases are presented in Table 4. In case I, the operational cost is 7592.65$ and pollutant emissions are 12.632 t. The relative error reaches 3.726%. This demonstrates that the constant efficiency EH model cannot accurately simulate the off-design operating IES, resulting in a low precision of the dispatch scheme. In case II (IT = 200–500), the relative errors are significantly reduced, which indicates that the accuracy of the model is greatly improved. In addition, the relative errors in case II (IT = 200–500) are reduced as IT increases due to the increasing forecast precision of DNNs, as presented in Figure 7. Although the operating cost and pollutant emissions in case II (IT = 500) are 293.84$ and 0.854 t, respectively, which are higher than those in case I, the dispatch scheme in case II (IT = 500) enjoys the highest precision and is most consistent under the actual off-design operating conditions of the IES.The dispatch results of electrical power in case I and case II (IT = 500) are shown in Figure 8, which have great differences.In case I, since devices are assumed to operate at the rated efficiency, CHP is more economical and is employed as the primary power supply. Electricity is purchased from the grid to satisfy the remainder of electrical loads. BAT is charged during the peak electricity prices and discharged during the low electricity prices.In case II (IT = 500), electrical loads are mostly met by the grid. When the electricity prices are low (e.g., 0–6 h and 23 h), it is more economical to supply electrical loads from the grid. CHP supplies only at the peak electricity prices (10 h and 19–20 h). The charging and discharging states of BAT are mainly affected by electricity prices, which is similar to the state in case I.The dispatch results of thermal power in case I and case II (IT = 500) are quite different, as shown in Figure 9.In case I, although CHP operates at low load levels, it remains as the main heating device, and the variable off-design characteristics are ignored. GB supplies energy only during peak thermal loads to make up for the unmet thermal loads by CHP.In case II (IT = 500), the thermal loads are mainly supplied by GB. To ensure the efficient operation of devices, GB operates alone when thermal loads are below the GB capacity, while most thermal loads are supplied by CHP when the thermal loads exceed the GB capacity (e.g., 4 h, 12 h, and 19–20 h). Moreover, thermal and electrical loads are simultaneously supplied by CHP during the peak electricity prices (10 h) to improve the operating economy of the IES.By comparing the two cases, the conclusions can be drawn that the traditional constant efficiency EH model is difficult to accurately model the IES under off-design conditions, making the dispatch results unreasonable. The impact of the off-design conditions on the energy device’s efficiency is supposed to be taken into consideration in the pursuit of the precise performance of the EED model.
This article has proposed a multi-objective EED method for the IES, considering the off-design conditions. Technically, the traditional EH model is remodeled into the DEH model enabled to operate with the time-varying energy device efficiency, by integrating with an efficiency correction process. Here, this efficiency correction is functioned by the proposed DNN-based efficiency correction strategy, which is a data-driven approach and has good performance in terms of nonlinear data processing. A multi-objective EED model of the IES is proposed to evaluate the benefits of considering the off-design conditions on the IES dispatch. Case studies are conducted, and several key observations are obtained from the numerical simulation: 1) the load rate of devices exerts a significant impact on the device efficiency, while the device efficiency in turn affects its load rate; 2) compared to the traditional EH model, the proposed DEH model is more reasonable and appropriate for following the actual operational environment of the IES; thus, more accurate operation schedules can be obtained; and 3) the proposed IES multi-objective EED scheme based on the DEH model can balance the IES’s multi-scale operational considerations well after taking into account the off-design conditions.
Currently, the authors are studying the real-time operation of the IES with the consideration of the off-design conditions, in which a rolling horizon strategy is utilized for coping with the realization of variable off-design conditions.
The original contributions presented in the study are included in the article; further inquiries can be directed to the corresponding author.
YX and YD conceptualized the study, performed the methodology, and validated the results. YX and YD prepared the original draft. GX, WJ, and ZJ reviewed and edited the draft. WJ and ZJ supervised the study.
This study was funded by the Science and Technology Project of State Grid Corporation (No. 5700-202229196A-1-1-ZN).
The authors declare that the research was conducted in the absence of any commercial or financial relationships that could be construed as a potential conflict of interest.
All claims expressed in this article are solely those of the authors and do not necessarily represent those of their affiliated organizations, or those of the publisher, the editors, and the reviewers. Any product that may be evaluated in this article, or claim that may be made by its manufacturer, is not guaranteed or endorsed by the publisher.
Akpan, P. U., and Fuls, W. F. (2019). Application and limits of a constant effectiveness model for predicting the pressure of steam condensers at off-design loads and cooling fluid temperatures. Appl. Therm. Eng. 158, 113779. doi:10.1016/j.applthermaleng.2019.113779
Almassalkhi, M. R., and Towle, A. (2016). Power systems computation conference. PSCC, 1–7. doi:10.1109/PSCC.2016.7540981enabling city-scale multi-energy optimal dispatch with energy hubs
Bartnik, R., Buryn, Z., Hnydiuk-Stefan, A., and Kowalczyk, T. (2022). Thermodynamic and economic comparative analyses of a hierarchic gas-gas combined heat and power (CHP) plant coupled with a compressor heat pump. Energy 244, 123116. doi:10.1016/j.apenergy.2016.07.080
Browell, J., and Fasiolo, M. (2021). Probabilistic forecasting of regional net-load with conditional extremes and gridded NWP. IEEE Trans. Smart Grid 12 (6), 5011–5019. doi:10.1109/TSG.2021.3107159
Chen, M., Zhou, D., Tayyebi, A., Prieto-Araujo, E., Dörfler, F., and Blaabjerg, F. (2022b). Generalized multivariable grid-forming control design for power converters. IEEE Trans. Smart Grid 13 (4), 2873–2885. doi:10.1109/TSG.2022.3161608
Chen, S., Chen, K., Zhu, X., Jin, X., and Du, Z. (2022a). Deep learning-based image recognition method for on-demand defrosting control to save energy in commercial energy systems. Appl. Energy 324, 119702. doi:10.1016/j.apenergy.2022.119702
Deb, K., Pratap, A., Agarwal, S., and Meyarivan, T. (2002). A fast and elitist multiobjective genetic algorithm: NSGA-II. IEEE Trans. Evol. Comput. 6 (2), 182–197. doi:10.1109/4235.996017
Dougier, N., Garambois, P., Gomand, J., and Roucoules, L. (2021). Multi-objective non-weighted optimization to explore new efficient design of electrical microgrids. Appl. Energy 304, 117758. doi:10.1016/j.apenergy.2021.117758
Ghimire, S., Deo, R. C., Raj, N., and Mi, J. (2019). Deep solar radiation forecasting with convolutional neural network and long short-term memory network algorithms. Appl. Energy 253, 113541. doi:10.1016/j.apenergy.2019.113541
Gong, L., Zhang, Y., and Bai, Z. (2021). Geothermal-solar hybrid power with the double-pressure evaporation arrangement and the system off-design evaluation. Energy Convers. Manag. 244, 114501. doi:10.1016/j.enconman.2021.114501
Guo, S., Song, G., Li, M., Zhao, X., He, Y., Kurban, A., et al. (2022). Multi-objective Bi-level quantity regulation scheduling method for electric-thermal integrated energy system considering thermal and hydraulic transient characteristics. Energy Convers. Manag. 253, 115147. doi:10.1016/j.enconman.2021.115147
Huang, W., Zhang, N., Wang, Y., Capuder, T., Kuzle, I., and Kang, C. (2020). Matrix modeling of energy hub with variable energy efficiencies. Int. J. Electr. Power Energy Syst. 119, 105876. doi:10.1016/j.ijepes.2020.105876
Jin, X., Kgs, L., Wu, Q., Jia, H., and Hatziargyriou, N. (2021). Optimal integration of building heating loads in integrated heating/electricity community energy systems: A Bi-level mpc approach. IEEE Trans. Sustain. Energy 12 (3), 1741–1754. doi:10.1109/TSTE.2021.3064325
Jin, X., Mu, Y., Jia, H., Wu, J., Xu, X., and Yu, X. (2016). Optimal day-ahead scheduling of integrated urban energy systems. Appl. Energy 180, 1–13. doi:10.1016/j.apenergy.2016.07.071
Li, C., Zhang, Z., Li, J., Ma, Y., and Zou, J. (2021). Design of control strategy and effect evaluation for primary frequency regulation of wind storage system. Front. Energy Res. 9. doi:10.3389/fenrg.2021.739439
Li, X., and Yao, R. (2021). Modelling heating and cooling energy demand for building stock using a hybrid approach. Energy Build. 235, 110740. doi:10.1016/j.enbuild.2021.110740
Lin, W., Jin, X., Mu, Y., Jia, H., Xu, X., Yu, X., et al. (2018). A two-stage multi-objective scheduling method for integrated community energy system. Appl. Energy 216, 428–441. doi:10.1016/j.apenergy.2018.01.007
Lin, W., Jin, X., Jia, H., Mu, Y., Xu, T., Xu, X., et al. (2021). Decentralized optimal scheduling for integrated community energy system via consensus-based alternating direction method of multipliers. Appl. Energy 302, 117448. doi:10.1016/j.apenergy.2021.117448
Liu, X., Chen, X., Li, J., Zhou, X., and Chen, Y. (2020). Facies identification based on multikernel relevance vector machin. IEEE Trans. Geosci. Remote Sens. 58 (10), 7269–7282. doi:10.1109/TGRS.2020.2981687
Long, Q., Yu, H., Xie, F., Lu, N., and Lubkeman, D. (2020). Diesel generator model parameterization for microgrid simulation using hybrid box-constrained levenberg-marquardt algorithm. IEEE Trans. Smart Grid 12 (2), 943–952. doi:10.1109/TSG.2020.3026617
Martínez Ceseña, E. A., and Mancarella, P. (2019). Energy systems integration in Smart districts: Robust optimisation of multi-energy flows in integrated electricity, heat and gas networks. IEEE Trans. Smart Grid 10 (1), 1122–1131. doi:10.1109/TSG.2018.2828146
Msigwa, G., Ighalo, J. O., and Yap, P. (2022). Considerations on environmental, economic, and energy impacts of wind energy generation: Projections towards sustainability initiatives. Sci. Total Environ. 157755. doi:10.1016/j.scitotenv.2022.157755
Mu, Y., Xu, Y., Cao, Y., Chen, W., Jia, H., Yu, X., et al. (2022). A two-stage scheduling method for integrated community energy system based on A hybrid mechanism and data-driven model. Appl. Energy 323, 119683. doi:10.1016/j.apenergy.2022.119683
Nandimandalam, H., Aghalari, A., Gude, V. G., and Marufuzzaman, M. (2022). Multi-objective optimization model for regional renewable biomass supported electricity generation in rural regions. Energy Convers. Manag. 266, 115833. doi:10.1016/j.enconman.2022.115833
Ravnik, J., and Hriberšek, M. (2019). A method for natural gas forecasting and preliminary allocation based on unique standard natural gas consumption profiles. Energy 180, 149–162. doi:10.1016/j.energy.2019.05.084
Sakawa, M., and Yano, H. (1985). Interactive fuzzy decision-making for multiobjective nonlinear programming using reference membership intervals. Int. J. Man-Mach. Study 23 (4), 407–421. doi:10.1016/S0020-7373(85)80043-2
Sarfi, V., and Livani, H. (2018). An economic-reliability security-constrained optimal dispatch for microgrids. IEEE Trans. Power Syst. 33 (6), 6777–6786. doi:10.1109/TPWRS.2018.2835421
Shao, S., Zhang, H., You, S., Wang, Y., and Li, Y. (2020). Prediction of thermal potentials of multi-panel refrigerant heated radiator based on a mathematical model considering refrigerant flow distribution. Energy Build. 228, 110446. doi:10.1016/j.enbuild.2020.110446
Xu, J., Wang, F., Lv, C., Huang, Q., and Xie, H. (2018). Economic-environmental equilibrium based optimal scheduling strategy towards wind-solar-thermal power generation system under limited resources. Appl. Energy 231, 355–371. doi:10.1016/j.apenergy.2018.09.113
Yu, J., Li, Z., Guo, Y., and Sun, H. (2019). Decentralized chance-constrained economic dispatch for integrated transmission-district energy systems. EEE Trans. Smart Grid 10 (6), 6724–6734. doi:10.1109/TSG.2019.2910757
Zheng, J. H., Huang, J., Deng, S., Wu, Q. H., Li, M., Zhou, X., et al. (2018). Comparison and error analysis of off-design and design models of energy hubs. CSEE J. Power Energy Syst. 5 (4), 454–465. doi:10.17775/CSEEJPES.2018.00630
Keywords: integrated energy system (IES), energy hub (EH), off-design conditions, economic-environmental dispatch (EED), multi-objective optimization, deep neural network (DNN)
Citation: Xu Y, Deng Y, Guo X, Wang J and Zhang J (2022) Economic-environmental dispatch for the integrated energy system considering off-design conditions. Front. Energy Res. 10:1020607. doi: 10.3389/fenrg.2022.1020607
Received: 16 August 2022; Accepted: 02 September 2022;
Published: 30 September 2022.
Edited by:
Youbo Liu, Sichuan University, ChinaReviewed by:
Yuehui Ji, Tianjin University of Technology, ChinaCopyright © 2022 Xu, Deng, Guo, Wang and Zhang. This is an open-access article distributed under the terms of the Creative Commons Attribution License (CC BY). The use, distribution or reproduction in other forums is permitted, provided the original author(s) and the copyright owner(s) are credited and that the original publication in this journal is cited, in accordance with accepted academic practice. No use, distribution or reproduction is permitted which does not comply with these terms.
*Correspondence: Youjun Deng, ZGVuZ195b3VqdW5AaG90bWFpbC5jb20=
Disclaimer: All claims expressed in this article are solely those of the authors and do not necessarily represent those of their affiliated organizations, or those of the publisher, the editors and the reviewers. Any product that may be evaluated in this article or claim that may be made by its manufacturer is not guaranteed or endorsed by the publisher.
Research integrity at Frontiers
Learn more about the work of our research integrity team to safeguard the quality of each article we publish.