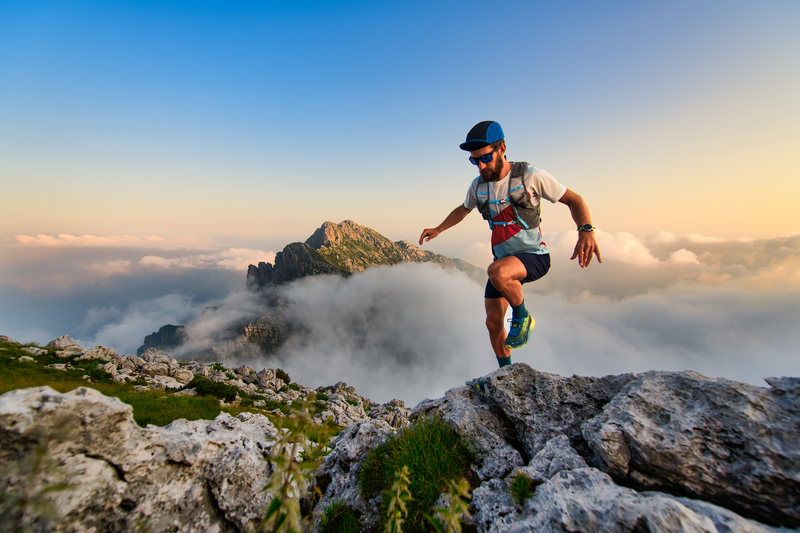
95% of researchers rate our articles as excellent or good
Learn more about the work of our research integrity team to safeguard the quality of each article we publish.
Find out more
ORIGINAL RESEARCH article
Front. Energy Res. , 07 January 2022
Sec. Sustainable Energy Systems
Volume 9 - 2021 | https://doi.org/10.3389/fenrg.2021.821686
This article is part of the Research Topic Progress Towards Achieving Cross-Regional Carbon Mitigation Targets View all 14 articles
Whether the environmental target responsibility system, a typical mandatory environmental regulation, can realize the coordinated development of environmental protection and economic growth has attracted widespread attention. With the difference-in-differences (DID) method, this paper utilizes a policy, “China’s Key Cities for Air Pollution Control to Meet the Standards within the Time Limit (APCMS),” as a quasi-natural experiment to empirically examine the target responsibility system of air pollution control’s effect on both firms’ pollutant emissions and their total factor productivity (TFP). The corresponding mechanisms are also investigated. The results show: 1) The policy not only significantly decreases firms’ pollutant emissions, but also improves their TFP. The results are robust to the exclusion of the impact of other policies in the same period, propensity score matching DID (PSM-DID) test, the adoption of alternative dependent variables, and altering sample interval; 2) The dynamic analysis shows that the policy effect on reducing pollutant emissions has increased over years after a lag of 2 years; 3) The policy reduces pollutant emissions mainly through stimulating the internal innovation rather than end-pipe treatment or production cuts. 4) Capital-intensive and private firms and firms in regions with a high degree of marketization or strong environmental law enforcement are found more responsive to the environmental target responsibility system.
Balancing the relationship between environmental sustainability and continuous economic growth is a challenge for policymakers, and also an important research topic for scholars. As the second-largest economy in the world, China’s remarkable economic growth has been accompanied by serious environmental problems. The report of the 19th National Congress of the Communist Party of China pointed out that “building an ecological civilization is a millennium plan for the sustainable development of the Chinese nation.” In order to effectively deal with environmental pollution and degradation, the environmental target responsibility system, which has been initially established by the “Environmental Protection Law” promulgated in 1989, is an important feature of China’s environmental regulatory policies (Zhang et al., 2021). Different from developed economies, as a transitional economy in China, local governments have tremendous influence and massive resources. However, the incentives of local governments to implement the central government’s environmental policies are seriously inadequate. Therefore, how to effectively encourage local governments has become an important challenge facing China’s environmental governance.
The environmental target responsibility system is an important means of goal-oriented and responsibility-based environmental governance. It has two typical characteristics: one is the top-down environmental goal setting. The central government stipulates major pollutant reduction targets, and then decomposes targets and tasks from top to bottom. The other is the responsibility scheme of environmental assessment. Local government officials assume the main responsibility for environmental protection, and the upper-level government assesses the fulfillment of environmental targets, and the results of the assessment are linked to the rewards, punishments, appointments and promotion of officials. The environmental target responsibility system has incorporated environmental protection goals into the assessment mechanism for local officials, and directly linked the promotion of officials with the implementation of environmental policies (Zhang and Hao, 2020). It has changed the behaviors of local officials from simply focusing on economic development to realizing coordinated development of the economy and ecology. The incentives of local governments for environmental governance have been greatly strengthened (Gao, 2010).
Since the formulation of environmental protection targets is from top to bottom, and the upper-level government assesses and supervises the implementation of environmental goals of local government officials, the environmental target responsibility system is a typical mandatory environmental regulation. Its pollutant emission reduction effect has been demonstrated by many scholars (Calel and Dechezleprêtre, 2016; Wu et al., 2019; Zheng et al., 2021). However, some scholars still question whether the effects of mandatory environmental regulations are sustainable. Becker (2011) and Greenstone (2002) believe that the emission reduction effect faces long-term uncertainty. In addition, there are two completely opposite views on whether the target responsibility system can harm or improve firms’ TFP. Some scholars argue that it will adversely affect the TFP through squeezing resources or delaying investment decisions. In addition, it imposes additional burdens, such as the installation of abatement equipment, which leads firms to shift resources from production and R&D to pollution control; It also increases the uncertainty faced by firms, which may delay the investment decisions, and even affects the R&D of new products and new production processes (Gollop and Roberts, 1983). However, Porter and van der Linde (1995) insist that appropriate environmental regulation can help upgrade firms’ TFP through a variety of channels such as innovation incentive, improvement of resource allocation efficiency, organizational reforms and so on. Whether the target responsibility system can reduce pollutant emissions and improve firms’ TFP at the same time is still not well known empirically despite the controversial qualitative arguments. Moreover, existing research on the similar issue is mainly concentrated in developed countries such as the United States, Germany and Japan (Barbera and McConnell, 1990; Gollop and Roberts, 1983; Viscusi, 1983; Hamamoto, 2006; Testa et al., 2011; Albrizio et al., 2017). There is still a lack of in-depth research in developing countries, particularly in China, which is the world’s largest SO2 emissions and with stricter environmental regulations. This paper is aimed at answering the question, whether the environmental target responsibility system can balance the relationship between environmental protection and economic development.
China’s Key Cities for Air Pollution Control to Meet the Standards within the Time Limit (APCMS) policy implemented since January 2003 provides a good quasi-experimental environment to study the effect of mandatory environmental regulation in developing countries. It is a typical representative of the environmental target responsibility system. Firstly, it is a large-scale top-down environmental regulation. 113 key cities designated by the central government were required to reach national atmospheric and water environmental quality standards before 2005. Secondly, the fulfillment of this policy is explicitly incorporated into the assessment and promotion of local government officials. It has a strong binding force to motivate local government officials. This paper uses this policy as a quasi-natural experiment, constructs a panel data set from 1998 to 2014, and uses the difference-in-differences (DID) estimator to identify the impact of the environmental target responsibility system on firms’ pollutant emissions and their TFP. This is to answer our research question, whether the mandatory environmental regulation can reduce pollutant emissions at the sacrifice of the economic development (e.g., lower firms’ TFP) or also upgrade the economic growth (i.e., higher firms’ TFP). Our empirical results show that this policy significantly reduces SO2 emissions, smoke and dust and industrial wastewater. Besides, the policy has significantly improved the firms’ TFP. The mechanism analysis results show that the key to realizing a win-win situation between environmental protection and economic growth lies in promoting technological progress, rather than end-pipe treatment or production cuts.
This paper has made contributions to the existing literature in the following three aspects: First, there is still no consensus as to whether the environmental target responsibility system can realize the coordinated development of the economy and ecology (Kostka, 2016; Yuan and Xiang, 2018; Chang et al., 2021; Zheng et al., 2021). Based on the APCMS policy implemented in China, this paper confirms that the policy achieves a win-win situation of pollution reduction and efficiency improvement. It provides further evidence for the debate about whether the mandatory environmental regulation and economic performance are “complementary” or “conflicting” for developing countries. Contrary to previous studies (Greenstone, 2002; Becker, 2011), empirical results demonstrate that pollution reduction effects are sustainable in the long term. Second, this paper analyzes the specific mechanism of the target responsibility system. That is, whether the target responsibility system decreases pollutant emissions and enhances efficiency through technological innovation, end-pipe treatment, or production scale adjustment. This enriches the mechanism research on the analysis of the mandatory environmental regulation. Third, this paper uses the firm-level data available from the China’s Industrial Enterprise database (CIED) and China’s Environmental Statistics database (CESD), while most studies use macro-level data (Jin et al., 2019; Wang et al., 2019; Zhang and Hao, 2020; Jiang et al., 2021). Thus, our estimations could be more accurate and useful to infer on the micro-level impact of the environmental regulation, particularly on each individual affected firm.
The rest of the paper is organized as follows. Section 2 clarifies the theory and hypothesis development. Section 3 summarizes the background of the APCMS policy. Section 4 introduces the research design. Section 5 shows and discusses the results of empirical analysis; Section 6 concludes the paper with some policy recommendations as well.
There has yet been empirical consensus on the relationship between environmental target responsibility system and pollutant emissions. First, disputes exist on whether this mandatory environmental regulation can indeed reduce pollutant emissions. Some scholars believe that environmental target responsibility system can reduce pollutant emissions. The environmental target responsibility system has added environmental criteria to the original performance assessment system, directly linking environmental governance to the promotion of local officials. It has realized the transformation from “soft constraint” to “hard constraint” on environmental protection, effectively restrained the internal impulse of local officials to sacrifice the environment for economic growth, and strengthened the enthusiasm of local governments for environmental protection and governance (Hong et al., 2019; Zhang et al., 2021). China’s mandatory environmental targets have reduced the total amounts of mandatory pollutant emissions (Schreifels et al., 2012; Kostka, 2016). The Blue Sky Defense War has effectively reduced the average concentration of PM2.5 and PM10 (Jiang et al., 2021). The system takes effect by inducing firms’ technological innovation and providing them direct financial incentives to abate emissions and pollutants. Moreover, it promotes the development and adoption of renewable energy, lowing the demand for fossil fuels and the associated carbon dioxide emissions (Zhao et al., 2013; He et al., 2020). However, some argue that the intensity of environmental regulation directly affects whether compliance costs will be considered in firms’ production process. If compliance costs are insufficient, environmental regulation lacks a binding force on behaviors, and the effect of reducing pollutant emissions may not be obvious (Jin et al., 2019). Second, the effect of the environmental target responsibility system faces uncertainty in the long-term and the system may result in undesirable consequences. Becker (2011) finds that the effect of pollutant emission reduction brought by mandatory environmental regulation may be offset by insufficient incentives and constraints. Taking the Clean Air Act in the United States as an example, the bill has been revised several times due to its poor effect (Greenstone, 2002). Kostka (2016) shows that the binding environmental targets have generated numerous problems, such as target rigidity, cyclical behavior, and so on. Liang and Langbein (2015) indicate that performance assessment reduced pollutant emissions only for the most visible air pollutants among the targeted pollutants.
Most literature confirms that the environmental target responsibility system can effectively reduce pollutant emissions. China has a vertical official performance appraisal system. The APCMS policy is a top-down mandatory environmental regulation. The central government assigns administrative environmental goals to local governments at all levels. Controlling pollutant emissions will naturally become performance appraisal indicators of local government officials. Therefore, the local governments must vigorously obey and control pollutant emissions within their jurisdiction. The pollutant emission reduction effect is sustainable. Based on the above analysis, the first hypothesis of this paper is proposed:
Hypothesis 1. The environmental target responsibility system will significantly reduce pollutant emissions.
The impact of the environmental target responsibility system on firms’ TFP is unclear ex-ante. Most studies confirm that this mandatory environmental regulation has a negative impact on firms’ TFP (Gollop and Roberts, 1983; Albrizio et al., 2017). On the one hand, it not only increases the non-productive investment, such as the installation of abatement equipment, which makes the firms’ resources flow into end-pipe treatment; it also increases the production costs of firms, such as the utilization of higher-cost clean energy, and upgrading of production lines, which hinders the increase of TFP. Barbera and McConnell (1990) studied polluting industries in the United States and found that the TFP of regulated firms decreased sharply under environmental regulation. The negative effects of mandatory environmental regulations are divided into direct and indirect effects. The direct effect is that the abatement equipment occupies resources, and the indirect effect refers to increased production costs due to changes in traditional producing techniques. He et al. (2020) found that China’s water quality monitoring system has an adverse effect on the TFP of polluting firms. Polluting firms located upstream of the river are affected by water quality monitoring stations and their TFP drop by 24%. On the other hand, the environmental target responsibility system may increase the uncertainty faced by firms and delay their investment decisions (Viscusi, 1983). And this uncertainty will further affect the R&D of new products and new production processes (Becker, 2011).
However, economists represented by Porter disagree with the above view. They believe that an appropriate environmental regulation may enhance innovation or encourage firms to adopt new organizational modes, improving TFP as a result (Yuan and Xiang, 2018). Hamamoto (2006) studied five manufacturing sectors in Japan and the results demonstrated that the environmental regulation increased firms’ R&D investment, which led to a continuous TFP growth over the past 20 years. The environmental regulation in the EU construction industry also significantly increased the firm’s R&D, thus improving their TFP (Testa et al., 2011; Rubashkina et al., 2015).
Based on the above analysis, we believe that the environmental target responsibility system may increase the burdens of firms in the short term, but in the long term, it enhances the incentives of innovation, which offsets the compliance costs of environmental regulation, and thus improves firms’ TFP. Therefore, the second hypothesis is proposed as follows:
Hypothesis 2. The environmental target responsibility system will significantly increase the TFP of firms.
Based on existing literature, there exist three possible impact mechanisms of the environmental target responsibility system on pollutant reduction and TFP: adjustments of production scale, end-pipe treatment, or technological innovation. Firstly, mandatory environmental regulations increase the production costs of enterprises, reduce their production efficiency and operating profits, certain firms have no choice but to close some high-polluting production lines, adjust production scales and even exit the heavily-polluting industries. For example, Greenstone (2002) demonstrated that Clean Air Act in the United States had significantly reduced the production and operation scales of firms. Liu et al. (2017) also found that stricter wastewater discharge standards reduced the labor demand and production scales of enterprises. Secondly, the installation of abatement equipment is widely used by many firms to reduce pollutant emissions (Becker, 2011; Li et al., 2020). Thirdly, the “Porter Hypothesis” insists that appropriate environmental regulation will stimulate enterprises to carry out technological innovation and produce innovation compensation effect. Clarke et al. (1994) argue that whether the environmental target responsibility system can realize pollutant emission reduction and the improvement of the TFP lies in whether firms can incorporate compliance costs into the strategic decisions and focus on innovation and product upgrading. If firms only focus on passive end-pipe treatment or production scale adjustment, the effect of reducing pollutant emissions may be significant in the short term, but it may have a negative impact on their TFP. If it enhances innovation incentives and firms focus on internal innovation to improve efficiency, the coordinated development of ecology and economy can be achieved at the same time.
However, there are two opposite views on whether the mandatory environmental regulations enhance technological innovation. Some scholars insist that the environmental target responsibility system hinders firms’ innovation. Innovation activities rely heavily on large capital investments and have a high risk of failure (Long et al., 2017; Luo et al., 2020). Under the pressure of mandatory environmental regulation, firms purchase abatement equipment and even take measures such as production cuts and shutdown, which squeezes necessary funds to finance R&D and innovation activities. Thus, it adversely impacts firms’ TFP (Albrizio et al., 2017; Petroni et al., 2019). On the contrary, others support that the environmental target responsibility system enhances innovation. It is believed that appropriate environmental regulation enables firms to allocate resources efficiently and actively engage in innovation activities, which may weaken or offset the impact of the compliance costs (Poter and van der Linde, 1995; Wang et al., 2021; Ouyang and You, 2021). By exerting strong external pressure, it can overcome firms’ inertia, enhance the enthusiasm of innovation, and form a good complementary relationship with the internal governance mechanism (Ambec and Barla, 2002).
Based on the above literature review, we believe that the environmental target responsibility system can improve firm’s TFP by stimulating their innovations. Borghesi et al. (2015) insist that due to the high risks of failure of innovation activities, the willingness of innovation depends largely on firms’ incentives. The environmental target responsibility system may promote innovation through the following two paths: firstly, it provides firms with more market information for technology upgrading (Goulder and Parry, 2008), which reduces the uncertainty of innovation. Secondly, it imposes high compliance costs on firms and enhances their willingness and motivation to innovate. Therefore, this paper puts forward the third hypothesis:
Hypothesis 3. The key reason for the effect of reducing pollutant emissions and improving TFP of the environmental target responsibility system is that it induces firm innovation.
In order to grapple with the problem of air pollution, the central government of China has implemented a series of top-down environmental regulations. The earliest one is the “Two Control Areas” policy. A lot of research has evaluated the effect of this policy (Tanaka, 2015; Cai et al., 2016). The APCMS policy discussed in this paper is also a top-down mandatory environmental regulation based on the target responsibility system (Liu et al., 2021). As early as 1998, the State Environmental Protection Administration promulgated a specific work plan, that is, the National Work Plan for Meeting the Discharge Standards of Industrial Pollution Sources and Environmental Function Zones in Key Environmental Protection Cities in 2000 (referred to as the “Two Compliance Policy”), which designated 47 cities as the first batch of key cities for air pollution control. These cities include Municipalities directly under the Central Government, provincial capital cities, coastal open cities and so on. They were required to reach urban atmospheric and water environmental quality standards before December 31, 2000. The ranking and implementation of the first batch of key cities were announced in the Statistical Bulletin of Environmental Conditions and released to the public.
In order to ensure that the air quality of key cities meets the target requirements within time limit, the State Environmental Protection Administration issued the Designation Plan of Key Cities for Air Pollution Control at the end of 2002. Through the analysis of comprehensive economic conditions and pollution status of each city, and the commitment of certain provincial governments to meeting atmospheric standards by 2005, the document designated other 66 cities as the second batch of key cities for air pollution control. The air quality of the 113 key cities mentioned above must meet the national environmental atmospheric quality standards by 2005. The distribution of key cities is shown in Figure 1.
Since January 2003, the State Environmental Protection Administration promulgated the APCMS policy. It is a typical mandatory environmental regulation based on a target responsibility system. Firstly, above 113 key cities were required by the central government to reach urban atmospheric and water environmental quality standards before 2005. Secondly, the State Environmental Protection Administration would strictly supervise and regularly announce the air quality of each key city. Performance of environmental protection targets were incorporated into the assessment and promotion of local officials. For key cities that failed to meet the standards before 2005, new projects that caused air pollution would be strictly restricted. The specific contents of the relevant documents are shown in Table 1.
This paper uses 66 key cities designated in the second batch of air pollution control to investigate the impact of the environmental target responsibility system on pollutant emissions and TFP of firms. On the one hand, this design is limited by the availability of data. The China’s Industrial Enterprise database (CIED) and China’s Environmental Statistics database (CESD) used in this paper have been counted since 1998. On the other hand, the first batch of designated cities are Municipalities directly under the Central Government, provincial capital cities, coastal open cities, key tourist cities, and special economic zone cities, which are among the richest areas in China. Their economic and social conditions are quite different from that of other cities. It is difficult to find similar control groups in the samples not affected by the policy.
In order to test the impact of the environmental target responsibility system on firm’s pollutant emissions, this paper constructs a DID model as follows (Long et al., 2018; Zhang et al., 2018; Wu et al., 2019; Chen et al., 2021):
In order to test whether the environmental target responsibility system can promote the efficiency of firms, we construct the following model:
The China’s Environmental Statistics database (CESD) provides data on firms’ pollutant emissions that account for 85% of China’s total emissions. The indicators include industrial output, SO2 emissions, smoke and dust, industrial wastewater and so on, as well as end-pipe treatment equipment. These data are reported by firms, collected and monitored by environmental protection departments to ensure data quality. They are considered to be the most comprehensive and reliable environmental micro-economic data in China (Zhang et al., 2018).
In order to comprehensively investigate the impact of the environmental target responsibility system on pollutant emission reduction and TFP, this paper merges the China’s Environmental Statistics Database (1998–2014) and the China’s Industrial Enterprise database (1998–2014). 44.4% of the samples are successfully matched, with a total of 734,290 samples. The reasons for the mismatch of the remaining samples are as follows: First, the two databases have different coverage. CIED only includes industrial firms, while CESD covers a wider range and also includes non-industrial firms that emit pollutants, such as hospitals; Second, CIED only contains industrial firms whose main business income exceeds 5 million yuan (10 million yuan after 2011), while CESD contains some high-polluting firms whose main business income is less than 5 million yuan (10 million yuan after 2011) (Liu et al., 2021). In addition, in order to verify the mechanisms of the environmental target responsibility system, this paper also uses the China’s Patent Database (CPD) from 1998 to 2014.
Firm’s Pollutant emissions. Considering the availability of data and requirements of this policy, this paper selects SO2 emissions, smoke and dust and industrial wastewater as explanatory variables and examines the comprehensive effect of the environmental regulation on pollutant emissions.
Firm’s TFP. The commonly used methods of measuring the TFP include OLS and fixed effect regression, OP method, LP method and ACF method proposed by Ackerberg et al. (2015). It is generally believed that OLS and fixed effect regression will produce large deviations in calculating the TFP. Therefore, this paper uses the ACF method to calculate TFP, and uses the OP method and LP method for robustness test (Olley and Pakes, 1996; Levinsohn and Petrin, 2003). These methods can solve the endogenous problem. This paper uses them to fit the production function, and the residual error obtained is TFP. For the fitting process, we follow the practice of Brandt et al. (2017), and the variables used in the function include the industrial added value, capital stock, investment, intermediate inputs, the number of employees and so on. All data for calculating TFP come from China’s Industrial Enterprise Database from 1998 to 2014.
Since 2007, the database lacked key variables for calculating the TFP such as industrial value-added, depreciation, intermediate input, wages, and so on. In order to calculate the TFP from 2008 to 2014, the missing variables need to be estimated. Following the practice of Yu et al. (2018), the depreciation rates after 2007 equal the depreciation rate of 2007, which equals the ratio of depreciation amount to fixed assets in 2007. For firms without depreciation in 2007, take the average depreciation rate of each industry by using a four-digit code. Since there is no industrial added value, the income approach is used to calculate the value. The specific formula is: industrial added value = accrued wages + value-added tax + income tax + business tax + total profit + depreciation amount of the current year (Zhu and Chen, 2020). For missing values of intermediate input, this paper assumes that intermediate input = total output value + value-added tax-added value. Considering there is a lack of wage data in certain years, the sum of the main business cost and administration expenses are used as the conversion basis for labor costs. Take the wages of 2009 for example, wages of 2009 = wages of 2008 × the growth rate of the conversion basis for labor costs. Due to the serious lack of enterprise code in 2010, it is difficult to guarantee the data quality, and many key variables are missing in 2010 and cannot be estimated and supplemented, so the TFP of enterprises in 2010 is not calculated. Samples of 2010 are not included in the model of TFP.
In theory, the production technology adopted by each firm is different. If a unified production function is used to calculate the firm’s TFP, it is at variance with reality. Therefore, we assume that the firm’s production mode in the same industry is relatively similar, and then estimate the capital and labor elasticity coefficient by industry based on the two-digit industry classification, so as to calculate the firm’s TFP. In addition, since the index in the CIED is the book value, it is necessary to convert it into real value. Specifically, the calculation of TFP also requires an output deflation index and input deflation index. The output deflation index comes from the chain price index of total output by industry in the “China Urban (Town) Life and Prices Yearbook.” According to the method of Brandt et al. (2012), the input deflation index is calculated using the input-output (IO) table.
In order to reduce the endogenous problem caused by possible omitted variables, this paper includes as many as control variables, including the firm’s size (total assets), total liabilities, capital intensity, the nature of property rights, and whether it is an exporting firm. Capital intensity is the ratio of the total fixed assets to the number of employees. This paper takes the natural logarithm of size, total liabilities and capital intensity. Finally, to reduce the influence of outliers, we shrink the tail of continuous variables. The descriptive statistics of variables are shown in Table 2.
Table 3 reports the regression results of the impact of the APCMS policy on firm’s pollutant emissions. The dependent variables in Table 3 are SO2 emissions, smoke and dust emissions, and industrial wastewater emissions in order. Column (1) controls firm fixed effects, year fixed effects, the intersection term of industry fixed effects and year fixed effects, and the intersection term of province fixed effects and year fixed effects. The result shows that the coefficient of the policy variable is significantly negative, indicating that the environmental target responsibility system has significantly reduced the firm’s SO2 emissions. Control variables are added to the column (2), the coefficient of the policy variable is still negative. It also confirms the emission reduction effect of the environmental target responsibility system. Columns (3) to (6) examine the impact of the policy on the emissions of smoke and dust and industrial wastewater. The coefficients of the policy variable are all significantly negative, indicating that the policy has also decreased the firm’s smoke and dust emissions and industrial wastewater discharge. Therefore, the environmental target responsibility system has effectively reduced pollutant emissions, and Hypothesis 1 has been verified.
Table 4 demonstrates the estimation results of the effect of the environmental target responsibility system on firms’ TFP. The result of column (1) shows that the coefficient of this policy is 0.0669, which is significantly at 1% level, indicating that the environmental target responsibility system has increased the firm’s TFP. Column (2) adds the intersection of industry and year fixed effects and the intersection of province and year fixed effects, and the result is basically unchanged. Column (3) adds control variables, and the result is consistent with columns (1) and (2). Therefore, the environmental target responsibility system has increased the firm’s TFP, thus confirming our Hypothesis 2.
The validity of the DID model lays on the fundamental common trend or parallel trend assumption. That is, the experimental and control group should exhibit the same trend of outcomes in the counterfactual case where the experiment does not occur. Thus, we need to verify an assumption to guarantee the validity of the above DID estimation results. In addition, since the policy may take some time to be effective, which is the lagging policy effect. We thus examine the possible dynamic effects of the environmental regulation on firms’ pollutant emissions and TFP in this subsection. Specifically, we follow the event study approach for empirical testing (Greenstone and Hanna, 2014) and build the following models:
We take 1998 as the base year,
Figure 2 plots the estimated results of
Besides, the pollution reduction effect of the environmental target responsibility system has a certain degree of sustainability. The reasons for the increasing impact of the policy may come from the following two aspects: First, industrial firms continue to invest in clean production-related innovation under the external pressure of compliance costs, so as to reduce pollutant emissions; Second, new environmental regulations have exerted additional impact on firms. In 2007, the State Council promulgated the “Eleventh Five-Year Plan for National Environmental Protection,” which designated 113 cities as key environmental protection cities. Among the 66 key cities for air pollution control discussed in this paper, 59 cities were designated as key environmental protection cities except for Weinan, Yuxi, Zigong, Deyang, Nanchong, Zhenjiang, and Sanmenxia. Therefore, these key cities are simultaneously affected by two policies after 2007. There exists a positive synergy between the two policies, which makes pollution reduction effects gradually increase over time. The parallel trend assumption is also verified for smoke and dust emissions and industrial wastewater discharge. The pollution reduction effect of the environmental target responsibility system becomes larger over time. Due to space limitations, the results are not included in the main text, and interested readers can ask the author for it.
Figure 3 plots the estimated results of
Since the beginning of this century, China has implemented a number of environmental regulations. The representative ones are the “Two Control Areas” policy and “Regulation on the Collection and Use of Pollutant Discharge Fees” of 2003 (referred to as the “Pollutant Discharge Fees” policy). The implementation of multiple policies at the same period may lead to the inclusion of the influence of other policies in the estimated results of the above model.
In order to exclude the influence of other policies on our policy effect estimations, more control variables of these other policies have also been added for robustness check. The “Two Control Areas” policy is represented by the intersection of two dummy variables of grouping dummy variable and year dummy variable (Fujii et al., 2013; Tanaka, 2015). If the firms are located in the city specified by the “Two Control Areas” policy, the grouping dummy variable is assigned a value of 1 and 0 otherwise. The year dummy variable is set to 1 in 2000 and afterward.
According to the “Pollutant Discharge Fees” policy, the management of pollutant discharge fees had become more stringent after 2003, and the charging fees for unit pollutants had been greatly increased, which constituted a relatively good “quasi-natural experiment.” In the Economic Census of 2004, CIED contained statistics on the pollution discharge fees in the management expenses item, which laid an important basis for dividing the experimental group and the control group. We assign a value of 1 to the firms that paid the pollution fees as the experimental group, and 0 to those that did not pay as the control group. The year dummy variable is set 1 in 2003 and afterwards. Similarly, the “Pollutant Discharge Fees” policy is represented by the intersection of grouping dummy variable and year dummy variable.
Table 5 reports the results after accounting for the possible effects of the “Pollutant Discharge Fees” policy and “Two Control Areas” policy. The results of columns (1) to (3) show that the APCMS policy still has a significant impact on reducing the three types of pollutants. “Two Control Areas” policy has significantly reduced SO2 emissions and smoke and dust emissions but has no effect on wastewater discharges. However, the “Pollutant Discharge Fees” policy has reduced wastewater discharges but has no effect on air pollutants. Column (4) estimates the impact on firm’s TFP after controlling for these two other policies. The result shows that the coefficient of the policy variable is significantly positive, indicating the results are robust. Besides, the other two policies also exert a positive impact on the TFP of firms.
It is possible that the selection of pilot cities by the central government is not random under the APCMS policy. Then, the pilot cities (i.e., the experimental group) can have very heterogeneous characteristics compared to the non-pilot cities (i.e., the control group). Then, the DID estimation could be subject to the “uncommon support bias” due to such selection bias caused by the non-random policy assignments. In order to overcome such possible selectivity bias, we adopt the propensity score matching (PSM) to select the most resembling control group cities for the DID estimation. This is basically the widely adopted PSM-DID for robustness testing (Dehejia and Wahba, 2002; Greenstone, 2004). PSM method has been widely used in the field of policy analysis. For example, PSM was used to study the impact of the United States Clean Air Act on SO2 emissions and analyze the impact of environmental regulation on employment in the power industry (Ferris et al., 2014).
The selection of key cities in the APCMS policy is likely to be based on some economic conditions and their pollutant emissions. Therefore, this paper selects the pollutant emissions and economic conditions of key cities as covariants for propensity score matching. Samples are matched using a one-to-one nearest neighbor matching method. 60 cities are successfully matched for our PSM-DID.
Table 6 reports the results of the PSM-DID model. The results show that the environmental target responsibility system has reduced the pollutant emissions of firms and improved their TFP significantly, which is basically consistent with the results in Table 3.
This paper divides the firm’s pollutant emissions by the total output as the dependent variables, SO2 emission intensity for example (Petroni et al., 2019). In addition, the measurement of the TFP is replaced with the LP and OP methods. As the Industrial Enterprise Database of 2007 did not provide relevant basic data for the OP method, and it could not be estimated by other indicators, the sample interval of TFP measured by OP method is 1998–2007. Table 7 reports the results of using alternative dependent variables. The results of columns (1) and (2) show that the policy has significantly reduced the intensity of SO2 emissions. The results of columns (3) and (6) demonstrate that the environmental target responsibility system has improved the TFP of firms. It is consistent with the results in Tables 3, 4, which confirms the robustness and reliability of the above conclusions of this paper.
This paper estimates the TFP of industrial enterprises from 1998 to 2014. However, the Industrial Enterprise Database after 2007 did not provide basic data required for measuring TFP, such as industrial added value and intermediate investment. For those missing data, we estimate according to the accounting standards and related literature, which may lead to the deviation between the estimated index and the actual value. In addition, some scholars argue that the data quality after 2007 is relatively poor. Therefore, in order to mitigate the impact of TFP measurement on the results, this section deletes the samples after 2007 to test the robustness of the results. Columns 1–4 in Table 8 are the regression results of the APCMS policy on SO2 emissions, smoke and dust emissions, wastewater discharges and TFP, respectively. The regression results are basically consistent with those in Tables 3, 4. The corresponding parallel trend test is shown in Figures 4, 5, verifying the parallel trend assumption. Altering the sample interval does not affect the conclusions of this paper.
This paper has confirmed that the environmental target responsibility system can achieve a win-win situation of environmental protection and economic efficiency, but is there any heterogeneity in the effect of this policy? In order to answer this question, this paper conducts a heterogeneity analysis.
The impact of the environmental target responsibility system on different factor-intensive firms may be totally different. Table 9 shows the results of group-level regression based on factor intensity. Panel A is the estimation of SO2 emissions; Panel B is the estimation of the TFP; Columns (1) and (2) are samples of capital-intensive firms and labor-intensive firms respectively.
Based on empirical results in Table 9, we find that the policy has reduced pollutant emissions of capital-intensive firms, while also increasing their TFP. The environmental target responsibility system has no significant impact on labor-intensive firms. The possible reasons are: Firstly, compared with labor-intensive firms, capital-intensive firms are more dependent on innovation. In addition, the profit margins of capital-intensive firms are generally higher than that of labor-intensive firms, so they have more funds for innovative activities. Therefore, the environmental target responsibility system has a greater impact on capital-intensive firms. The heterogeneity regression results of smoke and dust emissions and industrial wastewater emissions are not reported in the text due to space limitations.
The impact of the environmental target responsibility system on state-owned and private firms may be quite different. Panel A in Table 10 is the result of the impact on SO2 emissions; Panel B is the result of the impact on TFP; Columns (1) and (2) report results of state-owned firms without and with control variables respectively. Results indicate that the environmental target responsibility system has no significant impact on state-owned firms. Columns (3) and (4) report the results of private firms without and with control variables. Results show that for private firms, the environmental target responsibility system not only reduces their pollutant emissions, but also increases the overall TFP. Private firms are more sensitive to the environmental target responsibility system and more likely to realize the coordinated development of environmental protection and economic development.
The possible reason for the above heterogeneous effect is that the environmental target responsibility system promotes firms to reduce pollutant emissions and improve the TFP under the pressure of compliance costs. State-owned firms are under the control of the central government or local governments, which have great advantages in resource allocation, especially in financial support. They have soft budget constraints and may not be sensitive to the compliance cost pressure brought by environmental regulation. In contrast, private firms are responsible for their own profits and losses, and they hope to make up for the economic losses caused by compliance pressure by improving production efficiency.
The effect of the policy may be impacted by environmental law enforcement. If local officials relax environmental regulation and ignore the illegal discharge and leakage of polluting enterprises in order to protect local interests, it may result in the failure to achieve the goal of reducing pollutant emissions within a time limit. Generally speaking, the greater the intensity of environmental law enforcement, the higher the cost of violations faced by the company and the stronger the effect of the policy. In order to test the heterogeneity of the effects of the policy under different environmental law enforcement, this paper uses the number of environmental administrative punishments cases in each province to measure the intensity of environmental law enforcement, and divides the samples into two groups according to the median. The results of columns (1) and (2) in Table 11 show that in regions with high environmental law enforcement, the APCMS policy significantly reduces SO2 emissions and improves the TFP of enterprises. The results of columns (3) and (4) indicate that in regions with low environmental law enforcement, the policy has no significant impact on pollutant emissions and TFP. The results demonstrate that the effective implementation of environmental regulation relies on environmental law enforcement of local governments.
Enterprises in regions with a high degree of marketization often have better legal mechanisms and more transparent enterprise information. In order to explore whether the degree of marketization level will impact the implementation effect of mandatory environmental regulations, this study uses the marketization index to measure the degree of marketization (Wang et al., 2019). The samples are divided according to the median of the marketization index. The results of grouping regressions are shown in Table 12. The results of columns (1) and (2) illustrate that in regions with a high degree of marketization, the policy significantly reduces SO2 emissions and also enhances the TFP of enterprises. The results of columns (3) and (4) indicate that in regions with a low degree of marketization, the policy has no significant impact. This result shows that enterprises in regions with a high degree of marketization are more sensitive to the environmental target responsibility system.
The above empirical results show that the environmental target responsibility system has achieved the win-win between ecology and economy. There are three possible paths for the environmental target responsibility system to reduce pollutant emissions: Firstly, firms install emission reduction facilities, which effectively reduces pollutant emissions; secondly, firms close some high-polluting production lines or adjust production scales; thirdly, environmental compliance pressure forces firm to carry out technological innovations, which has a profound effect on pollutant emission reduction. These three paths have completely different effects on the TFP of firms. If firms have only relied on end-pipe treatment or production cuts, it will be difficult to increase the TFP. At the same time, the effect of pollutant emission reduction may not be sustainable in the long term. However intrinsic technological innovation not only helps firms increase the TFP, but also consolidates the achievements of emission reduction.
This paper uses the wastewater treatment facilities, desulfurization facilities and waste gas treatment facilities in the CESD as proxy variables for end-pipe treatment and verifies whether the policy reduces pollutant emissions through end-pipe governance. The results in Table 13 show that the environmental target responsibility system has no significant impact on the end-pipe treatment facilities.
The APCMS policy sets strict limits on the emissions of pollutants. Under the pressure of environmental regulation, companies may shut down certain high-pollution production lines or cut down production. In order to test the mechanism of production scale adjustment, this paper selects the industrial output value and industrial sales value as proxy variables for production scale.
Table 14 demonstrates the estimation results of the effect of the mandatory environmental regulation on firm’s production scale. The explained variables are industrial output value and industrial sales value respectively. The results of columns (1) to (4) indicate that the coefficients of the policy are not significantly different from 0. The environmental target responsibility system will not significantly reduce the production scale of enterprises.
In order to test the mechanism of technological innovation, this paper merges CIED and CPD, and obtains a panel data set from 1998 to 2014, with a total of 734,290 samples. The total number of patent applications is selected as a proxy variable for technological innovation. In order to test whether the environmental target responsibility system exerts heterogeneous effects on different types of patents, this paper divides patents into invention patents, utility model patents and design patents. Among them, invention patents have the highest degree of innovation. The results in Table 15 show that the environmental target responsibility system has not only significantly increased the total number of patent applications, but also increased different types of patents. It indicates that the APCMS policy not only increases the number of innovations, but also improved the quality of innovation.So we can conclude that the policy reduces pollutant emissions and improves the TFP by inducing technological innovation rather than passive end-pipe treatment or production cuts, which verifies Hypothesis 3.
Achieving the coordinated development of both environmental protection and economic efficiency is not only important for high-quality economic development, but also vital for the sustainable environmental protection for developing countries. Our empirical results based on the environmental target responsibility system, namely the APCMS policy, have shown that, the target responsibility system significantly reduces pollutant emission reduction. This finding is consistent with many previous studies (Zhao et al., 2013; Hong et al., 2019; He et al., 2020; Jiang et al., 2021). Although Becker (2011) argued that the pollution reduction effect of the mandatory environmental regulation faces uncertainty in the long-term, our empirical results confirm that the impact of the policy on reducing pollutant emissions is sustainable. And more importantly, results of dynamic effects show that the impact has increased year by year. The main reason for it is that the system enhances the enthusiasm of enterprises for technological innovation, which consolidates the effect of the environmental regulation. Besides, it can also be conducive to firm’s efficiency measured by TFP. Results are essential and inspiring for developing countries. It indicates that it is feasible to achieve a win-win outcome to improve the economy and ecology at the same time, through the mandatory environmental regulations. The environmental target responsibility system serves as a good instrument for encouraging local governments for environmental governance. This paper proposes and explores three possible mechanisms of mandatory environmental regulations (Greenstone, 2002; Albrizio et al., 2017; Liu et al., 2017; Petroni et al., 2019). The results of the mechanism analysis show that the inherent technological innovation rather than passive end-pipe treatment or production cuts is the key path for firms to achieve pollutant emission reduction and TFP improvement at the same time, verifying the “Porter Hypothesis”. Heterogeneity analysis shows that private firms and capital-intensive firms are more sensitive to the environmental target responsibility system. Only for enterprises in regions with a high degree of marketization or strong environmental law enforcement, the policy can play a significant positive role, indicating whether the effect of the environmental target responsibility can give full play depends heavily on the improvement of the external institutional environment.
Based on the above conclusions, this paper puts forward the following policy suggestions:
First, deepen the reform of the environmental target responsibility system, and strengthen the environmental protection incentives of local governments. The results of this paper illustrate that the environmental target responsibility system has effectively reduced enterprise pollutant emissions. Therefore, it is necessary to increase the proportion of environmental performance in the performance appraisal and audit on the departure of local officials. In addition, in order to improve the scientificity of environmental performance assessment, big data technology should be fully used in environmental monitoring, implementation, etc., to achieve real-time monitoring and upload of environmental data, and to ensure the accuracy of environmental monitoring data.
Second, it is essential to pay attention to the synergy between different environmental regulations. The results of dynamic effects suggest that the pollutant emission reduction effects of environmental regulation have gradually increased since 2007, indicating that the “Eleventh Five-Year Plan for National Environmental Protection” promulgated in 2007 also exerted a positive effect. The objectives and policy tools of this policy are similar to that of APCMS policy concerned in this paper. Besides, there is little difference in the coverage of cities, which has played a good synergistic effect. Therefore, the proper utilization of similar types of policies should be advocated.
Third, it is necessary to improve the pertinence and applicability of the environmental target responsibility system and related environmental regulatory policies. Our heterogeneity analysis demonstrates that the private firms are more sensitive to environmental regulation, while the impact on state-owned firms is particularly weak. Thus, it is essential to optimize the performance appraisal system of state-owned firms, incorporating relevant indicators of ecological environment protection, and strengthen the incentives and restraints of state-owned firms.
Fourth, it is vital to be vigilant about the adverse impact of technological progress incurred by the environmental target responsibility system on employment and other social issues. Our empirical results confirm that the environmental target responsibility system can achieve the coordinated development of ecology and economy through internal technological innovations of firms. However, the technological progress brought about by the mandatory environmental regulation may lead to the substitution of capital for labor, which in turn leads to the unemployment of low-skilled workers. The government needs to provide subsidies re-employment training to the unemployed, so as to reduce the possible negative effects of the environmental target responsibility system on social issues.
This study explores the effect of the environmental target responsibility system on pollutant emissions and TFP. However, due to data limitations, there are still areas that need to be improved and expanded. First, due to the availability of data, this article selects non-listed industrial enterprises as samples. However, there are still a large number of listed companies in China. For listed enterprises, follow-up research needs to be carried out through the manual collection of data. Second, the environmental target responsibility system is in the process of advancing and expanding. With the increase of data disclosure, more dimensions of research can be carried out, such as environmental investment, environmental information disclosure of enterprises and so on.
The raw data supporting the conclusions of this article will be made available by the authors, without undue reservation.
Conceptualization: JX, JW, and HX. Methodology: JX, JW, and HX. Software: HX. Data curation: HX. Writing—original draft preparation: HX, JW, and JX. Writing—review and editing: JW, JX, and HX. All authors have read and agreed to the published version of the manuscript.
This research was funded by the National Natural Science Foundation of China (Grant number 71903008) and the Research Plan of Beijing Municipal Education Commission (Grant number SM202010011012).
The authors declare that the research was conducted in the absence of any commercial or financial relationships that could be construed as a potential conflict of interest.
All claims expressed in this article are solely those of the authors and do not necessarily represent those of their affiliated organizations, or those of the publisher, the editors and the reviewers. Any product that may be evaluated in this article, or claim that may be made by its manufacturer, is not guaranteed or endorsed by the publisher.
Ackerberg, D. A., Caves, K., and Frazer, G. (2015). Identification Properties of Recent Production Function Estimators. Econometrica 83, 2411–2451. doi:10.3982/ECTA13408
Albrizio, S., Kozluk, T., and Zipperer, V. (2017). Environmental Policies and Productivity Growth: Evidence across Industries and Firms. J. Environ. Econ. Manage. 81, 209–226. doi:10.1016/j.jeem.2016.06.002
Ambec, S., and Barla, P. (2002). A Theoretical Foundation of the Porter Hypothesis. Econ. Lett. 75, 355–360. doi:10.1016/S0165-1765(02)00005-8
Barbera, A. J., and McConnell, V. D. (1990). The Impact of Environmental Regulations on Industry Productivity: Direct and Indirect Effects. J. Environ. Econ. Manage. 18, 50–65. doi:10.1016/0095-0696(90)90051-Y
Becker, R. A. (2011). Local Environmental Regulation and Plant-Level Productivity. Ecol. Econ. 70, 2516–2522. doi:10.1016/j.ecolecon.2011.08.019
Borghesi, S., Cainelli, G., and Mazzanti, M. (2015). Linking Emission Trading to Environmental Innovation: Evidence from the Italian Manufacturing Industry. Res. Pol. 44, 669–683. doi:10.1016/j.respol.2014.10.014
Brandt, L., Van Biesebroeck, J., Wang, L., and Zhang, Y. (2017). WTO Accession and Performance of Chinese Manufacturing Firms. Am. Econ. Rev. 107, 2784–2820. doi:10.1257/aer.20121266
Brandt, L., Van Biesebroeck, J., and Zhang, Y. (2012). Creative Accounting or Creative Destruction? Firm-Level Productivity Growth in Chinese Manufacturing. J. Dev. Econ. 97, 339–351. doi:10.1016/j.jdeveco.2011.02.002
Cai, X., Lu, Y., Wu, M., and Yu, L. (2016). Does Environmental Regulation Drive Away Inbound Foreign Direct Investment? Evidence from a Quasi-Natural Experiment in China. J. Dev. Econ. 123, 73–85. doi:10.1016/j.jdeveco.2016.08.003
Calel, R., and Dechezleprêtre, A. (2016). Environmental Policy and Directed Technological Change: Evidence from the European Carbon Market. Rev. Econ. Stat. 98, 173–191. doi:10.1162/REST_a_00470
Chang, L., Wang, J., Xiang, Z., and Liu, H. (2021). Impact of Green Financing on Carbon Drifts to Mitigate Climate Change: Mediating Role of Energy Efficiency. Front. Energ. Res. 9, 785588. doi:10.3389/fenrg.2021.785588
Chen, Y., Long, X., and Salman, M. (2021). Did the 2014 Nanjing Youth Olympic Games Enhance Environmental Efficiency? New Evidence from a Quasi-Natural experiment. Energy Policy 159, 112581. doi:10.1016/j.enpol.2021.112581
Clarke, R. A., Stavins, R. N., Ladd Greeno, J., Bavaria, J. L., Cairncross, D., and Etsy, D. C. (1994). The Challenge of Going Green. Harv. Bus. Rev. 72, 37–48.
Dehejia, R. H., and Wahba, S. (2002). Propensity Score-Matching Methods for Nonexperimental Causal Studies. Rev. Econ. Stat. 84, 151–161. doi:10.1162/003465302317331982
Ferris, A. E., Shadbegian, R. J., and Wolverton, A. (2014). The Effect of Environmental Regulation on Power Sector Employment: Phase I of the Title IV SO2 Trading Program. J. Assoc. Environ. Resource Economists 1, 521–553. doi:10.1086/679301
Fujii, H., Managi, S., and Kaneko, S. (2013). Decomposition Analysis of Air Pollution Abatement in China: Empirical Study for Ten Industrial Sectors from 1998 to 2009. J. Clean. Prod. 59, 22–31. doi:10.1016/j.jclepro.2013.06.059
Gao, J. (2010). Hitting the Target but Missing the Point: The Rise of Non-Mission-Based Targets in Performance Measurement of Chinese Local Governments. Adm. Soc. 42, 56S–76S. doi:10.1177/0095399710361854
Gollop, F. M., and Roberts, M. J. (1983). Environmental Regulations and Productivity Growth: The Case of Fossil-Fueled Electric Power Generation. J. Polit. Economy 91, 654–674. doi:10.1086/261170
Goulder, L. H., and Parry, I. W. H. (2008). Instrument Choice in Environmental Policy. Rev. Environ. Econ. Pol. 2, 152–174. doi:10.1093/reep/ren005
Greenstone, M. (2004). Did the Clean Air Act Cause the Remarkable Decline in Sulfur Dioxide Concentrations? J. Environ. Econ. Manage. 47, 585–611. doi:10.1016/j.jeem.2003.12.001
Greenstone, M., and Hanna, R. (2014). Environmental Regulations, Air and Water Pollution, and Infant Mortality in India. Am. Econ. Rev. 104, 3038–3072. doi:10.1257/aer.104.10.3038
Greenstone, M. (2002). The Impacts of Environmental Regulations on Industrial Activity: Evidence from the 1970 and 1977 Clean Air Act Amendments and the Census of Manufactures. J. Polit. Economy 110, 1175–1219. doi:10.1086/342808
Hamamoto, M. (2006). Environmental Regulation and the Productivity of Japanese Manufacturing Industries. Resource Energ. Econ. 28, 299–312. doi:10.1016/j.reseneeco.2005.11.001
He, G., Wang, S., and Zhang, B. (2020). Watering Down Environmental Regulation in China. Q. J. Econ. 135, 2135–2185. doi:10.1093/qje/qjaa024
Hong, T., Yu, N., and Mao, Z. (2019). Does Environment Centralization Prevent Local Governments from Racing to the Bottom? --Evidence from China. J. Clean. Prod. 231, 649–659. doi:10.1016/j.jclepro.2019.05.181
Jiang, X., Li, G., and Fu, W. (2021). Government Environmental Governance, Structural Adjustment and Air Quality: A Quasi-Natural Experiment Based on the Three-Year Action Plan to Win the Blue Sky Defense War. J. Environ. Manage. 277, 111470. doi:10.1016/j.jenvman.2020.111470
Jin, W., Zhang, H.-q., Liu, S.-s., and Zhang, H.-b. (2019). Technological Innovation, Environmental Regulation, and Green Total Factor Efficiency of Industrial Water Resources. J. Clean. Prod. 211, 61–69. doi:10.1016/j.jclepro.2018.11.172
Kostka, G. (2016). Command without Control: The Case of China's Environmental Target System. Regul. Governance 10, 58–74. doi:10.1111/rego.12082
Levinsohn, J., and Petrin, A. (2003). Estimating Production Functions Using Inputs to Control for Unobservables. Rev. Econ. Stud. 70, 317–341. doi:10.1111/1467-937X.00246
Li, W., Sun, H., Du, Y., Li, Z., and Taghizadeh-Hesary, F. (2020). Environmental Regulation for Transfer of Pollution-Intensive Industries: Evidence from Chinese Provinces. Front. Energ. Res. 8, 335. doi:10.3389/fenrg.2020.604005
Liang, J., and Langbein, L. (2015). Performance Management, High-Powered Incentives, and Environmental Policies in China. Int. Public Manage. J. 18, 346–385. doi:10.1080/10967494.2015.1043167
Liu, M., Shadbegian, R., and Zhang, B. (2017). Does Environmental Regulation Affect Labor Demand in China? Evidence from the Textile Printing and Dyeing Industry. J. Environ. Econ. Manage. 86, 277–294. doi:10.1016/j.jeem.2017.05.008
Liu, M., Tan, R., and Zhang, B. (2021). The Costs of “Blue Sky”: Environmental Regulation, Technology Upgrading, and Labor Demand in China. J. Dev. Econ. 150, 102610. doi:10.1016/j.jdeveco.2020.102610
Long, X., Chen, B., and Park, B. (2018). Effect of 2008's Beijing Olympic Games on Environmental Efficiency of 268 China's Cities. J. Clean. Prod. 172, 1423–1432. doi:10.1016/j.jclepro.2017.10.209
Long, X., Chen, Y., Du, J., Oh, K., and Han, I. (2017). Environmental Innovation and its Impact on Economic and Environmental Performance: Evidence from Korean-Owned Firms in China. Energy Policy 107, 131–137. doi:10.1016/j.enpol.2017.04.044
Luo, Y., Lu, Z., and Long, X. (2020). Heterogeneous Effects of Endogenous and Foreign Innovation on CO2 Emissions Stochastic Convergence across China. Energ. Econ. 91, 104893. doi:10.1016/j.eneco.2020.104893
Olley, G. S., and Pakes, A. (1996). The Dynamics of Productivity in the Telecommunications Equipment Industry. Econometrica 64, 1263–1297. doi:10.2307/2171831
Ouyang, L., and You, D. (2021). The Green Innovation Effect on Heavy-Polluting Enterprises under the Environmental Protection Law. Front. Energ. Res. 9, 681334. doi:10.3389/fenrg.2021.681334
Petroni, G., Bigliardi, B., and Galati, F. (2019). Rethinking the Porter Hypothesis: The Underappreciated Importance of Value Appropriation and Pollution Intensity. Rev. Pol. Res. 36, e0001–140. doi:10.1111/ropr.12317
Porter, M. E., and Linde, C. V. D. (1995). Toward a New Conception of the Environment-Competitiveness Relationship. J. Econ. Perspect. 9, 97–118. doi:10.1257/jep.9.4.97
Rubashkina, Y., Galeotti, M., and Verdolini, E. (2015). Environmental Regulation and Competitiveness: Empirical Evidence on the Porter Hypothesis from European Manufacturing Sectors. Energy Policy 83, 288–300. doi:10.1016/j.enpol.2015.02.014
Schreifels, J. J., Fu, Y., and Wilson, E. J. (2012). Sulfur Dioxide Control in China: Policy Evolution during the 10th and 11th Five-Year Plans and Lessons for the Future. Energy Policy 48, 779–789. doi:10.1016/j.enpol.2012.06.015
Tanaka, S. (2015). Environmental Regulations on Air Pollution in China and Their Impact on Infant Mortality. J. Health Econ. 42, 90–103. doi:10.1016/j.jhealeco.2015.02.004
Testa, F., Iraldo, F., and Frey, M. (2011). The Effect of Environmental Regulation on Firms' Competitive Performance: The Case of the Building & Construction Sector in Some EU Regions. J. Environ. Manage. 92, 2136–2144. doi:10.1016/j.jenvman.2011.03.039
Viscusi, W. K. (1983). Frameworks for Analyzing the Effects of Risk and Environmental Regulation on Productivity. Am. Econ. Rev. 73, 793–801.
Wang, P., Dong, C., Chen, N., Qi, M., Yang, S., Nnenna, A. B., et al. (2021). Environmental Regulation, Government Subsidies, and Green Technology Innovation-A Provincial Panel Data Analysis from China. Ijerph 18, 11991. doi:10.3390/ijerph182211991
Wang, Y., Sun, X., and Guo, X. (2019). Environmental Regulation and Green Productivity Growth: Empirical Evidence on the Porter Hypothesis from OECD Industrial Sectors. Energy Policy 132, 611–619. doi:10.1016/j.enpol.2019.06.016
Wu, X., Gao, M., Guo, S., and Maqbool, R. (2019). Environmental and Economic Effects of Sulfur Dioxide Emissions Trading Pilot Scheme in China: A Quasi-Experiment. Energ. Environ. 30, 1255–1274. doi:10.1177/0958305X19843104
Yu, M. J., Jin, Y., and Zhang, R. (2018). Capacity Utilization Rate Measurement and Productivity Estimation for Industrial Firms. Econ. Res. J. 53, 56–71.
Yuan, B., and Xiang, Q. (2018). Environmental Regulation, Industrial Innovation and Green Development of Chinese Manufacturing: Based on an Extended CDM Model. J. Clean. Prod. 176, 895–908. doi:10.1016/j.jclepro.2017.12.034
Zhang, B., Chen, X., and Guo, H. (2018). Does Central Supervision Enhance Local Environmental Enforcement? Quasi-Experimental Evidence from China. J. Public Econ. 164, 70–90. doi:10.1016/j.jpubeco.2018.05.009
Zhang, P., and Hao, Y. (2020). Rethinking China's Environmental Target Responsibility System: Province-Level Convergence Analysis of Pollutant Emission Intensities in China. J. Clean. Prod. 242, 118472. doi:10.1016/j.jclepro.2019.118472
Zhang, P., Yu, T., Lu, S., and Huang, R. (2021). How Does Target-Based Performance Evaluation Affect the Accuracy of Energy-Saving Data: Evidence from China. Front. Energ. Res. 9, 704317. doi:10.3389/fenrg.2021.704317
Zhao, X., Ma, Q., and Yang, R. (2013). Factors Influencing CO2 Emissions in China's Power Industry: Co-integration Analysis. Energy Policy 57, 89–98. doi:10.1016/j.enpol.2012.11.037
Zheng, Y., Sun, X., Zhang, C., Wang, D., and Mao, J. (2021). Can Emission Trading Scheme Improve Carbon Emission Performance? Evidence from China. Front. Energ. Res. 9, 759572. doi:10.3389/fenrg.2021.759572
Keywords: target responsibility system, APCMS, pollutant emission, TFP, innovation
Citation: Wang J, Xu H and Xu J (2022) Can the Target Responsibility System of Air Pollution Control Achieve a Win-Win Situation of Pollution Reduction and Efficiency Enhancement?. Front. Energy Res. 9:821686. doi: 10.3389/fenrg.2021.821686
Received: 24 November 2021; Accepted: 13 December 2021;
Published: 07 January 2022.
Edited by:
Kangyin Dong, University of International Business and Economics, ChinaReviewed by:
Jian Yu, Central University of Finance and Economics, ChinaCopyright © 2022 Wang, Xu and Xu. This is an open-access article distributed under the terms of the Creative Commons Attribution License (CC BY). The use, distribution or reproduction in other forums is permitted, provided the original author(s) and the copyright owner(s) are credited and that the original publication in this journal is cited, in accordance with accepted academic practice. No use, distribution or reproduction is permitted which does not comply with these terms.
*Correspondence: Hao Xu, eGgxMzc0MDc4MTYzQDE2My5jb20=; Jingxuan Xu, eHVqaW5neHVhbkBidGJ1LmVkdS5jbg==
Disclaimer: All claims expressed in this article are solely those of the authors and do not necessarily represent those of their affiliated organizations, or those of the publisher, the editors and the reviewers. Any product that may be evaluated in this article or claim that may be made by its manufacturer is not guaranteed or endorsed by the publisher.
Research integrity at Frontiers
Learn more about the work of our research integrity team to safeguard the quality of each article we publish.