- Institute of Finance and Economics Research, Shanghai University of Finance and Economics, Shanghai, China
Excessive emissions of carbon dioxide and other greenhouse gases have seriously affected the ecological environment and the normal operation of the social economy, and low-carbon city policy (LCCP) is one of China’s main policies to achieve carbon emission reduction goals. This study focused on the 280 cities in China, and used the difference-in-differences (DID) model and nighttime light data to evaluate the impact and mechanisms of LCCP on carbon emissions from 2003 to 2016. The results show that: 1) The implementation of LCCP can reduce carbon emissions by 16.2%; 2) Mechanism analysis shows that LCCP can achieve carbon reduction through the guidance of government behaviors, improvements of industrial structure and innovation capabilities, reductions of energy consumption, and the optimization of the ecological environment; 3) Heterogeneity analysis shows that the effect of the LCCP in suppressing carbon emissions is more effective in eastern cities with better economic and industrial bases, and large cities with more population. Furthermore, the effect of LCCP shows an inverted U-shaped trend as carbon emissions increase. This study enriches the research on assessing policy effects of China’s low-carbon city construction, and provides some inspiration for the goal of carbon neutrality and other developing countries with high carbon emission.
Introduction
The massive emission of greenhouse gases represented by carbon dioxide poses a serious threat to the sustainable development of global ecosystems and human society (Chu et al., 2021). After the “Paris Agreement” put forward a temperature control target of 1.5°C–2°C (Rickels et al., 2018), in order to more effectively deal with the problem of global climate change, the Glasgow Climate Convention passed in November 2021 further implements the restriction goal of global warming to 1.5°C.
As the world’s largest carbon dioxide emitting economy (Wang et al., 2021), China has demonstrated its responsibility as a responsible developing country. In order to reduce carbon emissions, the Chinese government has adjusted the economic development model from high-speed growth to high-quality growth, with a view to achieving carbon emission reduction in the process of economic development through the improvement of production efficiency. In addition, the Chinese government has also formulated climate policy goals for carbon peaking in 2030 and carbon neutrality by 2060 (Chen et al., 2022; Zhang et al., 2022). To ensure the smooth completion of these goals, the Chinese government has also implemented a series of policies such as carbon markets and low-carbon city policy (LCCP).
Unlike the carbon market policy that only targets the carbon emissions of industrial enterprises, the goal of LCCP is to reduce carbon emissions from all aspects of the social economy. The concept of China’s low-carbon cities is proposed on the basis of the “Low-carbon Economy” of the United Kingdom and the “Low-carbon Society” of Japan. The goal of low-carbon cities is to transform the production and life of cities through low-carbon concepts, low-carbon thinking, and low-carbon technologies, thereby minimizing carbon emissions and ultimately achieving sustainable development of cities (Yang and Li, 2013). Specifically, on the carbon emission side, LCCP promotes green production by enterprises and green consumption by residents to realize energy conservation and reduce carbon footprint (Yang and Li, 2013; Shen et al., 2018). On the carbon absorption side, low-carbon cities are actively carrying out tree planting and afforestation, increasing areas of green space, and enhancing the absorption of CO2 by vegetation. Therefore, LCCP is likely to become an important driving force for China to achieve carbon neutrality goals.
However, the existing literature does not have a unified conclusion on the carbon emission reduction effect of LCCP. Some literature finds that LCCP have a limited and short-term effect in reducing carbon emissions (Shin, 2018; Tang et al., 2018), Fang et al. (2017) even find that a lot of polluting projects were not stopped despite the construction of the low-carbon city. More literature has found evidence that LCCP can suppress carbon emissions through case analysis in a certain area. For example, Wang et al. (2015) analyzed specific cases of some low-carbon pilot cities and found the positive effect of LCCP on reducing carbon emissions. Yu et al. (2019) adopted the synthetic control method and drew a conclusion that the LCCP has reduced the average carbon emissions of Guangdong Province by 10%. Moreover, some studies pointed out that after the implementation of the LCCP, the annual carbon emissions of Hangzhou, Xiamen, and Shenzhen have been reduced by 20,000 tons, and the carbon emission intensity of Jingdezhen, Zunyi, and Wuhan were 24.47, 20.43, and 19.12% lower than the provincial average, respectively (Cheng et al., 2019). Another study similar to our work comes from Fu et al. (2021). They utilized DEA and PSM-DID methods to evaluate the carbon emission efficiency of the second batch of China’s low-carbon city pilots, and considered that economic growth and technological innovation are potential mechanisms. other studies, due to lack of carbon emissions data, found a positive effect of LCCP in suppressing air pollution (Gehrsitz, 2017), promoting green production efficiency (Yu, 2014), and improving ecological efficiency (Song et al., 2020). In general, there are still gaps in studies on the carbon reduction effect of LCCP: 1) the current research on the carbon emission reduction effect of LCCP does not have a unified conclusion, and the literature that believe LCCP can reduce carbon emissions is also obtained through case analysis of specific cities, which lacks rigorous empirical evidence at the national level; 2) The lack or inaccuracy of carbon emission data is an important factor hindering the smooth progress of such research; 3) The literature has not conducted in-depth research on the channels through which LCCP affects carbon emissions.
To cover these gaps, this study focused on the 280 cities in China, and used the difference-in-differences (DID) model and nighttime light data to evaluate the impact and mechanisms of LCCP on carbon emissions from 2003 to 2016. The results show that: 1) The implementation of LCCP can reduce carbon emissions by 16.2%; 2) Mechanism analysis shows that LCCP can achieve carbon reduction through the guidance of government behaviors, improvements of industrial structure and innovation capabilities, reductions of energy consumption, and the optimization of the ecological environment; 3) Heterogeneity analysis shows that the effect of the LCCP in suppressing carbon emissions is more effective in eastern cities with better economic and industrial bases, and large cities with more population. Furthermore, the effect of LCCP shows an inverted U-shaped trend as carbon emissions increase.
This paper contributes to the existing literature in the following ways. Firstly, this paper is among the first to conduct a rigorous empirical analysis of the carbon emission reduction effects of the national LCCP from the city level. Existing documents have analyzed the impact of LCCP on carbon emissions in specific provinces and cities (Cheng et al., 2019), or obtained the conclusion that LCCP can reduce carbon emissions through case analysis (Wang et al., 2015). The literature not only lacks national-level research, but the results obtained are also unconvincing.
Secondly, we use night light data to more accurately study the causal relationship between LCCP and carbon emissions. Due to the lack of city-level carbon emissions data, or the inaccurate measurement of carbon emissions, the literature can only conduct case studies on specific cities (Yu et al., 2019), or study the air pollution, building operations (Li K et al., 2022; Zhang et al., 2022), international trade (Li M et al., 2021; Liu et al., 2021), clean energy and energy efficiency (Sun et al., 2019; Zhang and Hanaoka, 2021), technological innovation and industrial development (Su et al., 2021; Tang et al., 2021), vegetation (Chen et al., 2021; Liao et al., 2021) and environmental efficiency of LCCP (Song et al., 2020). The Nighttime lighting data can not only provide a more accurate strategy for total city carbon emissions (Zhao et al., 2019; Li and Wang, 2022), but also let us more accurately assess the impact of LCCP on carbon emissions.
Thirdly, we further supplement the research field of potential mechanisms for LCCP to reduce carbon emissions. Previous studies focus on how to reduce carbon emissions in the industrial sector, such as industrial upgrading, energy intensity, and technological innovation (Song et al., 2020; Fu et al., 2021). On the basis of these studies, we have innovatively increased the investigation of the influence channels of government and residential departments, as well as the investigation of carbon absorption from the perspective of carbon sequestration by vegetation. Additionally, we provide evidence that LCCP has a better effect of suppressing carbon emissions in the more industrialized central and eastern regions and larger cities. Quantile regression implies that the effect of LCCP shows an inverted U-shaped trend with increasing carbon emissions. Taken together, our study provides some enlightenment how different cities use the effects of LCCP to promote low carbon development and achieve carbon neutrality goals.
The remainder of this paper is organized as follows. The LCCP in China section briefly describes the institutional background of LCCP in China. Theoretical Mechanism section outlines the potential theoretical mechanisms. Methodology and Variables section explains the methodology and variables. In Results section, five questions are discussed: Baseline Results section conducted a baseline regression to study the causal relationship between LCCP and carbon emissions. Parallel Trend Tests section Testes the parallel trend between low-carbon city pilots and non-low-carbon city pilots; Mechanism Analysis section analyzes the mechanisms of LCCP affecting carbon emissions; Robustness Tests section tests the robustness of LCCP in reducing carbon emissions; Heterogeneity Analysis section explores the heterogeneity of carbon reduction effect of LCCP in different cities. Conclusion section concludes and discusses.
The LCCP in China
China’s low-carbon city pilot program started in 2010. During China’s “Two Sessions” in March 2010, the Chinese government identified the low-carbon development path as a major strategy for economic and social development and further included the low-carbon strategy in the “Twelfth Five-Year Plan.” In July 2010, the National Development and Reform Commission issued the “Notice on Carrying out Low-Carbon Provinces and Low-Carbon City Pilot Work.” The first batch of low-carbon city pilots began to be set up, including five provinces and eight prefecture-level and above cities, a total of 82 low-carbon cities. In 2012, in order to implement the goal of “Building a Beautiful China” in the report of the 18th National Congress of the Communist Party of China, the Chinese government continued to launch low-carbon pilot projects to further expand the scope of low-carbon pilots. Specifically, the second batch of pilot projects implemented in 2013 will include 33 cities like Beijing, Shanghai, Wuhan, and Suzhou.
In addition, it needs to be mentioned that the third batch of pilot projects have been implemented in 2017. Reference to previous literature, the third batch have not been included due to the data availability and method limitations (Song et al., 2020). As shown in Figure 1, China’s low-carbon city pilots have spread across 31 provinces across the country.
Although the specific LCCP in various cities may not be uniform, they are generally summarized in the following four aspects. First, local governments provide special funds and financial incentives, and develop a low-carbon transportation system. Second, adjust the industrial structure and encourage technological innovation, actively develop a circular economy to promote the low-carbon industries. Third, replace coal with clean energy, such as solar and wind energy, and improve energy efficiency. Fourth, establish a data statistics and management system for greenhouse gas emissions, strengthen carbon emissions statistics, and set up a complete data collection and accounting system.
Theoretical Mechanism
In this section, we discuss in detail the theoretical mechanisms of the LCCP on city carbon emissions. We classify possible channels into the following categories: government behavior, industrial structure and innovation, energy consumption, and carbon absorption.
Government Behaviors
As a command-and-control environmental regulation tool, LCCP can impose mandatory interventions and constraints on projects and enterprises that affect the city carbon emissions to ensure the achievement of emission reduction targets in pilot cities (Yu, 2014). At present, China’s LCCP implements the main leadership responsibility system (Lo, 2014; Tang et al., 2018). Pilot cities formulate carbon emission targets and implementation plans, and delegate them to specific industries and key industries (Wang et al., 2015; Shin, 2018). In the process of policy implementation, the National Development and Reform Commission and the Provincial Development and Reform Commission established a contact mechanism to regularly supervise the implementation of plans by local governments in pilot cities. This top-down supervision mechanism may ensure the effectiveness of policy implementation (Liu and Ravenscroft, 2017).
In addition, the LCCP package includes some special funds and fiscal stimulus plans (Wang et al., 2015; Qu and Liu, 2017), which helps to combine market incentive tools to achieve emission reduction targets, and to maximize social and economic effects. Meanwhile, the implementation of market tools and funding plans can make up for the mechanical and compulsory nature of traditional environmental regulatory tools, which is more conducive to solving the problem of government environmental governance failures (van der Vlist et al., 2007; Wirth et al., 2013). Moreover, governments in low-carbon cities may also affect carbon emissions by changing the city transportation structure and network (Yang and Li, 2013). For example, they may change the public transportation network to ensure the smoothness and convenience of public transportation, thereby reducing dependence on private cars and reducing carbon emissions.
In view of the fact that the role of Chinese environmental regulation in reducing carbon emissions has been widely confirmed in previous studies (e.g., Feng and Li, 2019; Wang et al., 2019), the low-carbon policy through changing government behavior to achieve low-carbon goals will not be questioned.
Industrial Structure and Innovation
The LCCP is likely to improve the city industrial structure and innovation capabilities in many ways. First, from the perspective of macroscopic effects, living spaces of high-energy-consuming and high-polluting industries has been compressed under the guidance of LCCP, and the new energy, low-energy, and environmentally friendly emerging industries have been replaced (Song et al., 2020). With the dual impact of policy support and industrial migration effects, the resources of polluting industries may gradually flow to green industries forming a cluster of green industries (Cheng et al., 2019; Sun et al., 2021a). In view of the high-tech nature of the green industry, the LCCP can effectively promote industrial upgrading while promoting urban innovation capabilities.
Second, in the microscopic effects, the implementation of LCCP makes environmental friendliness an important factor that affects the internal decision-making of enterprises, which is likely to change the distribution of factors and production methods of enterprises (Hu et al., 2020). There is no doubt that the cost of environmental regulations may affect the production efficiency of enterprises in the early stage, but the government can effectively guide enterprises to reduce resource consumption and carbon emissions through subsidies to green enterprises (Qiu et al., 2021). Taking into account the comparative advantages and long-term economic benefits brought by low-carbon technologies, companies tend to actively change the original resource allocation model, such as actively increasing investment in green elements (Boyd and McClelland, 1999; Chakraborty and Chatterjee, 2017), increasing green innovation and investing in environmental protection industries. Through the impact on the micro-behavior of enterprises, LCCP may achieve the goals of technological innovation and industrial upgrading.
In addition, promoting technological innovation and industrial structure upgrading has become an important way for countries to reduce carbon emissions (e.g., Hu et al., 2020; Fu et al., 2021). Therefore, we consider that LCCP can reduce carbon emissions through technological innovation and industrial upgrading.
Energy Consumption
The third way we consider LCCP to reduce carbon emissions is increasing urban energy consumption. First, LCCP requires not only low energy consumption and low pollution for enterprises, but also low-carbon concept in government affairs and residents’ daily life (Yang and Li, 2013), such as using green energy for heating in winter, advocating green and low-carbon concepts, and developing low-carbon transportation systems. Second, several local governments are gradually establishing a complete carbon emission data accounting system and low-carbon assessment mechanism, and the economic development model based on low-carbon is taking shape (Sun et al., 2021b), which helps to further improve energy efficiency. Third, some low-carbon cities also advocate building energy conservation, and promote the use of renewable energy such as solar energy and geothermal energy in buildings on a large scale (van der Heijden, 2016; Li K et al., 2022). Residential energy supply should also use renewable resources as much as possible to achieve zero emissions from residential buildings (Chen et al., 2022).
Since fossil energy consumption is the main source of excessive carbon emissions in Chinese cities, the establishment of LCCP can reduce energy consumption at the source and achieve low-carbon development.
Ecological Optimization
The ultimate goal of low-carbon city construction is to achieve carbon neutrality, that is, relative “zero” carbon emissions (Opschoor, 2011). In addition to the energy saving and emission reduction mentioned in the previous mechanisms, improving ecology environment to increase carbon absorption is also an important aspect. It is the choice of many low-carbon cities in China to achieve carbon absorption by planting a large number of carbon sequestering plants and ecological parks. For example, Chengdu has planted nearly 4,500 hectares of trees after becoming a low-carbon city. More importantly, the carbon sequestration effect of planted forests is more significant (Liao et al., 2021). Taking the planted eucalyptus forests that can become lumber within 5–7 years as an example, the solid level is equivalent to 20 to 30 times that of natural coniferous forests.
In addition, the increase in vegetation brought about by low-carbon cities may adjust the urban climate, reduce the heat island effect (Wilmers, 1990; Zhang et al., 2010), and reduce energy consumption due to high-power electrical appliances, which can indirectly reduce carbon emissions. Taken together, increasing carbon absorption by increasing vegetation and green areas may be the fourth mechanism of LCCP worth considering. The mechanism of LCCP affecting carbon emissions is shown in Figure 2.
Methodology and Variables
Empirical Strategy
Similar to the existing literature (e.g., Sun et al., 2021b), we employ the widely used DID method in policy evaluation to assess the impact of LCCP on carbon emissions. Considering that the pilot projects in low-carbon cities are conducted in batches, we adopt the time-varying DID approach to examine the causal relationship between the LCCP and carbon emissions in Chinese cities. The model settings are as follows:
where the subscript i accounts for a prefecture-level city, and t refers to the year.
Variables
CO2 Emissions
The explained variable in this paper is the city’s carbon emissions. As mentioned above, the data comes from two sets of nighttime light data provided by the National Geophysical Data Center, and Chen et al. (2020) used them to calculate the CO2 emissions of 2,735 counties in China from 1997 to 2017. Since DMPP/OLS and NPP/VIIRS images come from two types of satellites, there are differences in sensors, spatial resolution, and propagation function. Therefore, they adopt the particle swarm optimization-back propagation (PSO-BP) algorithm for inter-calibration.
Furthermore, they established the relationship between provincial CO2 emissions and nighttime light data to get the sum of DN values to represent county-level carbon emissions. Through some econometric tests, the CO2 emission data calibrated by satellite nighttime light data show good validity and higher reliability than previous calculations (Lv et al., 2020). Additionally, since the LCCP is a city-level environmental policy, we obtain city annual CO2 emissions by adding up the values of all counties in the same city.
Figure 3 shows the carbon emissions of each city in 2003, 2010, 2016, and the average carbon emissions during the sample period. Compared with 2003, the carbon emissions of each city in 2010 have increased significantly. In 2016, the carbon emissions of prefecture-level cities also increased compared with 2010, but the increasing trend of carbon emissions was significantly weakened. Additionally, the average carbon emissions of prefecture-level cities from 2003 to 2016 is similar, or even lower than 2010. Overall, northern and eastern cities emit more CO2.
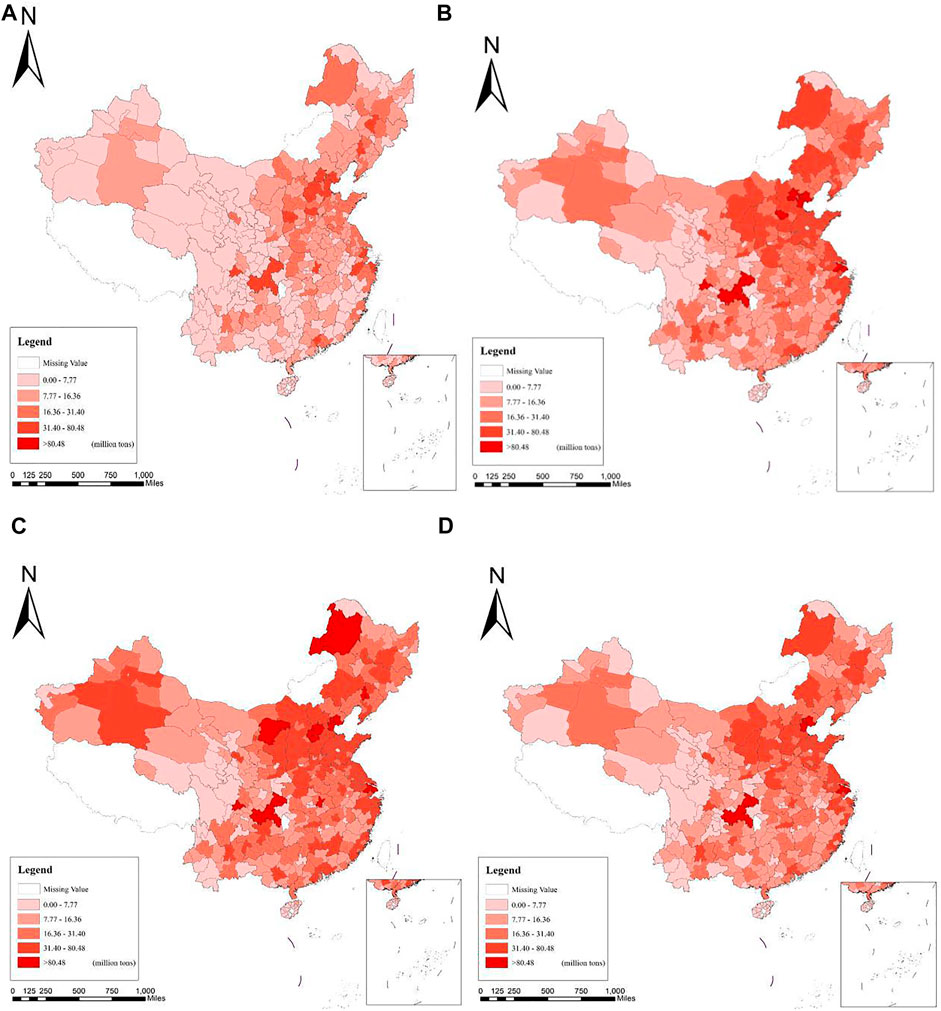
FIGURE 3. Carbon emissions in Chinese cities based on the nighttime light data. (A) the carbon emissions in 2003; (B) the carbon emissions in 2010; (C) the carbon emissions in 2016; (D) the average carbon emissions from 2003 to 2016.
The LCCP
The explanatory variable is whether a city becomes a low-carbon city pilot. As noted in The LCCP in China section, the National Reform Commission of China issued the “Notice on Pilot Work for Carbon Provinces and Low-Carbon Cities” on July 19, 2010 and November 26, 2012. These two batches of low-carbon city pilots involved 115 Chinese cities at prefecture-level and above. If a city becomes a low-carbon city according to these two “Notices,” the value of the LCCP is set to 1, and 0 otherwise.
City Characteristic Variables
In order to eliminate the influence of other factors and get a more accurate results, we control a series of city characteristic variables. First, considering that the level of economic development is closely related to carbon emissions (Lozano and Gutiérrez, 2008), we use the total GDP of the city to measure economic development. Second, since the second industry is the main sector of China’s carbon consumption (Chen et al., 2018; Shen et al., 2018), we control the secondary industry development (SEC) and industrial agglomeration level (AGG) of the city. Meanwhile, technological progress (TEC) may improve traditional production processes and increase production efficiency, and ultimately reduce industrial carbon emissions. We use the number of regional patent applications to indicate the level of technological progress. Third, China is a government-oriented society, and government intervention (GOV) and fixed asset investment (FAI) are regarded as important factors affecting city carbon emissions (Kennedy and Corfee-Morlot, 2013; Ma et al., 2019). Fourth, Cities with higher population density could emit more CO2 by people’s daily activities and carbon footprint (Aichele and Felbermayr, 2012). Lastly, energy consumption (ENE) directly affects the level of CO2 emissions (Bloch et al., 2012), therefore, it is necessary to control city’s energy consumption. It should be mentioned that the patent data comes from the State Intellectual Property Office, and other city characteristic variables are all from the China City Statistical Yearbook.
Weather Variables
Weather conditions may also affect carbon emissions (Kaufmann et al., 2013; Wu et al., 2021). For example, the CO2 content in the air is lower in areas with heavy rainfall or strong winds. Therefore, we control for city’s weather variables including average temperature (TEM), relative humidity (HUM), total amount of precipitation (PRE), and sunshine hours (SUN). Our data on weather variables comes from 331 weather stations in China. These weather stations recorded the temperature of local cities on daily basis from 1984 onwards. We add up or average these daily data to get annual weather data of each city, and then match them with the other city-level data.
Summary Statistics
Table 1 presents the definition and descriptive statistics of our main variables, and all continuous variables are winsorized by replacing samples among the top 1% and the bottom 1%. In our samples, the average value of carbon emissions is close to the standard deviation, suggesting that city CO2 emissions are likely to be relatively balanced in different Chinese cities. The results of descriptive statistics also suggest that 803 samples became low-carbon cities among all the samples, which provides sufficient samples and heterogeneity for the analysis using the DID model in this paper.
Results
Baseline Results
Based on the quasi-natural experiment of the LCCP, we use the time-varying DID method to examine the impact of the implementation of LCCP on CO2 emissions in Chinese cities. The baseline results are shown in Table 2. In column (1), we control the city and year fixed effects without any city characteristic variables and weather variables, and we find that the coefficient on LCCP is negative at the 1% significance level. In column (2), we add the city characteristic variables and the result remains unchanged. In column (3), we control weather variables and fixed effects, and the result show that there is no association between the LCCP and city CO2 emissions. Column (4) reports the result with controlling all control variables and fixed effects. It is clear that the implementation of LCCP has a significant inhibitory effect on CO2 emissions.
In general, our results are consistent with some previously related studies that confirmed the positive effects of the LCCP (Yu, 2014; Cheng et al., 2019; Qiu et al., 2021). By using the nighttime light data from Chen et al. (2020), we further proved the advantages of LCCP in reducing city carbon emissions.
Parallel Trend Tests
An important prerequisite for the effectiveness of DID is the parallel trend hypothesis, that is, before the LCCP takes place, the changing trends of the CO2 of the experimental group and control group should be parallel and should not be significantly different. This paper follows the approach of Jacobson et al. (1993) to further investigate the changing trends between experimental group and control group. Specifically, we use the following equation to do the parallel trend test:
Where
Figure 4 represents parallel trend tests of the impact of the LCCP on carbon emissions. We find that before the implementation of LCCP, the coefficients between experimental group and control group have no different from 0 at the 5% significance level. This shows that there is no significant difference between the treatment group and the control group on CO2 emissions before the implementation of LCCP. However, after the implementation of LCCP, cities with the LCCP can significantly reduce CO2 emissions compared with cities who is not. In addition, we can see that after the implementation of the LCCP, coefficients turned from positive to negative, and showed a downward trend, indicating that in the medium and long term, low-carbon city construction may significantly reduce carbon emissions. Taken together, the results in Figure 4 suggests that the parallel trend tests are satisfied.
Mechanism Analysis
According to Theoretical Mechanism section, the LCCP may affect city’s CO2 emissions through channels such as government behaviors, industrial structure and innovation, energy consumption, and ecological optimization. We test the above mechanisms in turn through empirical analysis in this subsection.
Government Behaviors
As noted above, the LCCP can reduce CO2 emissions by improving government behaviors. One of the goals of LCCP is to encourage government departments to build a low-carbon society, therefore, strengthening government intervention and increasing fixed assets investments are feasible ways (Qiu et al., 2021). In the first two columns of Table 3, the estimation results from the DID models show that the coefficients on LCCP are both positive at the 5% significance level, indicating that governments of low-carbon city pilots may increase investment and intervention to guide the city’s low-carbon transformation by providing subsidies for low-carbon technologies and green industries.
In addition, building a green transportation system is the choice of some low-carbon cities in China. Since public transportation provided by government sectors can replace private cars and reduce carbon emissions (Jiang et al., 2019), we use the number of public transport as a proxy indicator of city’s green transportation. Column (3) of Table 3 shows that the low-carbon cities were more likely to have an environmentally friendly public transportation mode. All in all, these results provide supportive evidence that low-carbon cities may reduce carbon emissions by changing government behaviors, including strengthening government intervention, increasing fixed assets investments, and building greener transportation systems.
Industrial Structure and Innovation
As highlighted in Theoretical Mechanism section, the LCCP helps promote the improvement of city’s industrial structure and innovation capabilities. We measure the industrial structure by using the ratio of the employed population in the secondary to the tertiary industries. In column (1) of Table 4, the DID estimate shows a negative association between the LCCP and industrial structure, which implies that compared with the secondary industry with more carbon emissions, the LCCP is more beneficial to the development of the tertiary industry, and further promote the transformation and upgrading of the secondary industry to the tertiary industry.
Next, we use the number of green patent applications and city innovation index to measure innovation ability1. In column (2) and (3) of Table 4, the estimated coefficient on LCCP is positive at the 5% significant level, suggesting that the implementation of LCCP may contribute to improving the city’s innovation level and green patent applications, and finally reducing carbon emissions. Our estimates confirm that the LCCP plays a role in industrial structure and innovation capabilities. As discussed in Theoretical Mechanism section, the literature suggests that industrial upgrading and innovation can effectively improve production processes and increase efficiency, realizing low energy consumption and green production (Wang et al., 2020).
Energy Consumption
As discussed in Theoretical Mechanism section, the LCCP may bring about low energy consumption to reduce carbon emissions. Column (4) of Table 4 reports the estimation result of the impact of the LCCP on electricity consumption. We can see that the LCCP has inhibited the industrial energy consumption, which is significant at the 1% level. In addition, considering that another emission of industrial energy consumption is SO2, we use the total industrial SO2 emissions as a proxy of energy consumption2. In column (5), we find that the LCCP can suppress industrial SO2 emissions, which is consistent with the previous result. These findings indicate that the implementation of the LCCP may reduce industrial energy consumption and promote green and low-carbon production of enterprises.
Ecological Optimization
In Theoretical Mechanism section, we consider that the construction of low-carbon cities helps improve ecological environment and increase carbon absorption. We construct three ecological variables, namely vegetation carbon sequestration, garden green areas, and green coverage areas. Among them, the vegetation carbon sequestration is also from Chen et al. (2020), and calculated by satellite nighttime light data3. Garden green areas is measured by the area of garden green spaces in the urban areas, and green coverage areas is measured by coverage rate of green space in city’s built-up areas. The original data on these two variables also comes from China City Statistical Yearbook.
In column (1) of Table 5, we find that the LCCP can increase the carbon sequestration of vegetation with statistical significance at the 1% level, suggesting that low-carbon cities are likely to create a better ecological environment and increase carbon absorption through tree planting and afforestation. Columns (2) and (3) report the DID estimation results on garden green areas and green coverage areas, and we find that the coefficients of LCCP are both significant and positive, suggesting that the LCCP can effectively increase the urban green areas and improve the ecology. In summary, our findings provide suggestive evidence that ecological optimization is one of the important channels for LCCP to reduce carbon emissions and achieve carbon neutral.
Robustness Tests
To get a more accurate estimation result, we conduct several robustness checks in this subsection. First, we employ placebo tests and results are presented in Table 6. We assume that the implementation time of the policy is 1–3 years earlier than the real time. We find that the inhibitory effect of LCCP on carbon emissions is insignificant in columns (1) to (3). This result shows that fictional effects are not valid and the decrease in carbon emissions is indeed caused by the LCCP, indicating that the baseline regression results are convincing.
To alleviate the bias caused by the model setting and the differences in identification methods of policy implementation time, we do robustness tests through replacing estimation approaches and key variables. First, the first batch of LCCP was implemented in July 2010, and the second batch in November 2012. In the main specification, we regard 2010 and 2012 as the starting time of LCCP, respectively. However, some studies believe that it will take some time for the policy to be truly implemented, especially for the policies announce in the second half of the year (Lipp, 2007). Due to time delays, policies often work in the second year after promulgation. Therefore, we postpone the year of policy implementation by 1 year, and the results are shown in column (1) of Table 7. It is clear that the DID estimated result remains unchanged.
Second, there is a proportional relationship between CO2 and PM2.5 since they have the same homology and the synchronization of pollution (Li X et al., 2022). In order to overcome the bias in the use of satellites to collect CO2 data, we replace CO2 with PM2.5 and re-estimate. The data of PM2.5 comes from the annual World PM2.5 density map published by Columbia University (Zhao et al., 2021). The result in column (2) of Table 5 indicates that the LCCP still reduces the concentration of PM2.5.
Third, in order to alleviate the bias caused by the model settings, we adopt the PSM-DID method for robustness checks (Wang et al., 2019; Fu et al., 2021). The results in columns (3) and (4) show that no matter what methods are used for regression, the similar effect of the LCCP curbing carbon emissions remains unchallenged.
Furthermore, we try to eliminate some interfering factors. First, in 2013, China established seven carbon market pilots, which had a significant inhibitory effect on carbon emissions (Fan et al., 2016), and the carbon market will cause an overestimation of the LCCP’s inhibitory effect on carbon emissions. Due to the similarities of policy effects, we deleted pilot cities of carbon trading markets4, and the result are represented in column (1) of Table 8. Clearly, The LCCP can still significantly reduce city’s carbon emissions.
Second, the LCCP is not only implemented in prefecture-level cities, but also implemented at the provincial level, like Guangdong, Hubei, and Hainan. Compared with municipal-level pilots, provincial-level pilots have a higher administrative level, stronger government control and stricter policy implementation methods, which will cause an overestimation of the effect of LCCP on carbon emissions suppression. Some previous studies directly delete the provincial-level pilots when evaluating the effectiveness of LCCP (Cheng et al., 2019; Qiu et al., 2021). In column (2) of Table 8, we delete the sample of provincial-level pilots, and find that the DID estimates are similar to the main estimates in Table 2.
Third, considering that the differences in the administrative level may affect the reliability of the results, we remove the sample data of Beijing, Shanghai, Tianjin, and Chongqing as well as all provincial capital cities. In column (3) of Table 8, we find that this change has little effect on the results.
Lastly, the implementation of more stringent environmental regulations such as the Ten Atmosphere Regulations has resulted in technological progress and industrial structure optimization and upgrading in areas with severe air pollution (Feng and Li, 2019; Cheng et al., 2020; Li X. et al., 2021), which may reduce carbon emissions and make the effect of LCCP on carbon emissions overestimated. After excluding the “2 + 26” areas5 with particularly serious pollution in the ten atmospheric articles in column (4) of Table 8, the inhibitory effect of LCCP on carbon emissions is still significant.
Heterogeneity Analysis
The influence of the LCCP on carbon emissions may be heterogeneous. First, due to the differences in economic development, degree of industrialization, and technological innovation among various regions in China, we divided into three sub-samples of the eastern, central, and western to analyze the heterogeneity according to the previous literature (Yao and Zhang, 2001; Fan et al., 2016)6. In the first three columns of Table 9, the DID estimation results show that the LCCP has the strongest inhibitory effect on carbon emissions in the eastern region with the highest degree of industrialization. According to Shen et al. (2018), since the industrial sector accounts for a high proportion of CO2 emissions, the effects of LCCP can reduce CO2 emissions by promoting regional industrial structure. In contrast, in the western region, industrial sectors are still needed for city’s economic development, and has not met the requirements of transformation and upgrading, so the LCCP has a weaker suppression effect.
Second, we divide cities into large-scale cities and small and medium-sized cities according to whether the permanent population of the city exceeds one million (Zhao et al., 2021). By comparing the results in columns (4) and (5) of Table 9, the LCCP in large cities has a stronger suppression effect on carbon emissions, while weaker in small and medium-sized cities. The possible reason is that the population quality of Chinese large cities is higher, and they are more able to practice the concept of low-carbon life, by reducing carbon footprint and carrying out environmental protection activities to help build low-carbon cities and reduce carbon emissions.
Third, considering that under different carbon emission levels, the effects of LCCP may be inconsistent, we perform the quantile regression for heterogeneity analysis. In Table 10, we can see that the coefficients on LCCP are significantly negative in all column, indicating that under different levels of carbon emissions, there was no obvious difference in the effect of the LCCP. Furthermore, with the increase in the quantile, the absolute value of the coefficients of LCCP increase first and then decrease, indicating that the LCCP has more significant impact in the middle part of the conditional distribution of city’s carbon emissions than on both ends.
Conclusion
With China’s transformation and development, the LCCP has become the key measure for achieving carbon peaks in 2030 and carbon neutral targets in 2060. By using the latest comprehensive CO2 emission data calculated based on nighttime light data from Chen et al. (2020) and the panel data of Chinese cities from 2003 to 2016, we employ the time-varying DID approach to examine the impact and mechanism of the LCCP on carbon emissions. The findings are summarized as follows. 1) The LCCP could significantly curb carbon emissions. 2) The negative impact of the LCCP on carbon emission could be explained by the guidance of government behaviors, improvements of industrial structure and innovation capabilities, reductions of energy consumption, and the optimization of the ecological environment. 3) The effect of the LCCP in suppressing carbon emissions is more effective in eastern cities with better economic and industrial bases and large cities with more population. 4) LCCP can significantly reduce carbon emissions, regardless of the level of carbon emissions, and the effect of LCCP shows an inverted U-shaped trend as urban carbon emissions increase.
We put forward the following policy recommendations in response to the above findings. First, the central government could appropriately strengthen the LCCP. On the one hand, the central government could appropriately improve the LCCP evaluation mechanism and evaluation standards, so that cities can further play the role of LCCP in promoting carbon emission reduction in the process of obtaining or maintaining the honor of “Low-carbon Pilot City.” On the other hand, in order to achieve carbon emission reduction targets, local governments should also strengthen their intervention in carbon emission reduction. Such as planting trees, increasing the area of parks and green spaces, and advocating the residents’ departments to pay attention to the carbon footprint.
Second, government departments should issue relevant policies or supporting measures to assist LCCP in achieving carbon emission reductions more efficiently. As the industrial sector is an important source of carbon emissions, technological innovation and industrial structure upgrades by industrial enterprises could reduce carbon emissions to a large extent. Therefore, government departments should implement preferential tax policies or innovation subsidy policies for industrial enterprises engaged in R&D and innovation to ensure or promote the motivation of industrial enterprises to carry out technological innovation. On the other hand, the government should also issue relevant industrial policies to ensure the transformation and upgrading of the industrial structure from high-carbon to low-carbon.
Third, local governments should also implement LCCP based on local conditions. Since different cities in China have great differences in the level of economic development, development stage, and resource endowment, each local government should formulate relevant policy standards according to its own development. For example, economically developed regions could set stricter carbon emission reduction targets, while regions with relatively backward economies and developed heavy industries should moderately reduce carbon emissions under the premise of ensuring economic growth, so as to achieve economic and environmental coordination, green and sustainable development.
Similar to China, some developing countries also face the dilemma of high energy consumption, high carbon emissions, and environmental degradation, such as India, Russia, Iran, Mexico, and Indonesia. Although the LCCP originated in developed countries, China’s successful experience may provide a good template for these developing countries that are under pressure to reduce CO2 emissions. The evidence from Chinese cities we offer in this paper shows that the LCCP plays a significant role in reducing carbon emissions. Therefore, we believe that the LCCP provides good enlightenment for these developing countries to mitigate the problem high carbon emissions and achieve sustainable development.
Data Availability Statement
The original contributions presented in the study are included in the article/supplementary material, further inquiries can be directed to the corresponding author.
Author Contributions
XL: Data curation, formal analysis, methodology, software, writing—original draft, validation, resources; CZ: conceptualization, data curation, formal analysis, visualization, writing—original draft, funding acquisition; MH: formal analysis, writing—review and editing, supervision, resources.
Funding
This work was supported by the Shanghai Philosophy and Social Science Planning Annual Project (2021BJB003), the Fundamental Research Funds for the Central Universities (CXJJ-2020-310), and the Fundamental Research Funds for the Central Universities (No. QCDC-2020-21).
Conflict of Interest
The authors declare that the research was conducted in the absence of any commercial or financial relationships that could be construed as a potential conflict of interest.
Publisher’s Note
All claims expressed in this article are solely those of the authors and do not necessarily represent those of their affiliated organizations, or those of the publisher, the editors and the reviewers. Any product that may be evaluated in this article, or claim that may be made by its manufacturer, is not guaranteed or endorsed by the publisher.
Footnotes
1The original data of city innovation index comes from Kou and Liu (2017), and the data related to green patents are compiled in accordance with the “Green List of International Patent Classification” issued by the World Intellectual Property Office.
2The unit of total industrial SO2 emissions is ton.
3As before, the unit of vegetation carbon sequestration is million tons.
4Carbon trading pilots include Beijing, Shanghai, Shenzhen, Tianjin, and Chongqing, Hubei province and Guangdong province.
5These 28 cities are Beijing, Tianjin, Shijiazhuang, Tangshan, Langfang, Baoding, Cangzhou, Hengshui, Xingtai, Handan, Taiyuan, Yangquan, Changzhi, Jincheng, Jinan, Zibo, Jining, Dezhou, Liaocheng, Binzhou, Heze, Zhengzhou, Kaifeng, Anyang, Hebi, Xinxiang, Jiaozuo, and Puyang.
6The eastern region includes eight provinces, namely Hebei, Shandong, Liaoning, Jiangsu, Zhejiang, Fujian, Guangdong, and Hainan. There are eight provinces in the central region, and they are Heilongjiang, Jilin, Shanxi, Jiangxi, Anhui, Henan, Hubei, and Hunan. The remaining 11 provinces or autonomous regions belong to the western region, namely Xinjiang, Inner Mongolia, Ningxia, Gansu, Qinghai, Tibet, Yunnan, Guizhou, Sichuan, Guangxi, and Shaanxi.
References
Aichele, R., and Felbermayr, G. (2012). Kyoto and the Carbon Footprint of Nations. J. Environ. Econ. Manage. 63, 336–354. doi:10.1016/j.jeem.2011.10.005
Bloch, H., Rafiq, S., and Salim, R. (2012). Coal Consumption, CO2 Emission and Economic Growth in China: Empirical Evidence and Policy Responses. Energ. Econ. 34, 518–528. doi:10.1016/j.eneco.2011.07.014
Boyd, G. A., and McClelland, J. D. (1999). The Impact of Environmental Constraints on Productivity Improvement in Integrated Paper Plants. J. Environ. Econ. Manage. 38, 121–142. doi:10.1006/jeem.1999.1082
Chakraborty, P., and Chatterjee, C. (2017). Does Environmental Regulation Indirectly Induce Upstream Innovation? New Evidence from India. Res. Pol. 46, 939–955. doi:10.1016/j.respol.2017.03.004
Chen, J., Gao, M., Cheng, S., Hou, W., Song, M., Liu, X., et al. (2020). County-level CO2 Emissions and Sequestration in China during 1997-2017. Sci. Data 7, 391. doi:10.1038/s41597-020-00736-3
Chen, J., Xu, C., Wang, Y., Li, D., and Song, M. (2021). Carbon Neutrality Based on Vegetation Carbon Sequestration for China's Cities and Counties: Trend, Inequality and Driver. Resour. Pol. 74, 102403. doi:10.1016/j.resourpol.2021.102403
Chen, M., Ma, M., Lin, Y., Ma, Z., and Li, K. (2022). Carbon Kuznets Curve in China's Building Operations: Retrospective and Prospective Trajectories. Sci. Total Environ. 803, 150104. doi:10.1016/j.scitotenv.2021.150104
Chen, Y. J., Li, P., and Lu, Y. (2018). Career Concerns and Multitasking Local Bureaucrats: Evidence of a Target-Based Performance Evaluation System in China. J. Dev. Econ. 133, 84–101. doi:10.1016/j.jdeveco.2018.02.001
Cheng, J., Yi, J., Dai, S., and Xiong, Y. (2019). Can Low-Carbon City Construction Facilitate green Growth? Evidence from China's Pilot Low-Carbon City Initiative. J. Clean. Prod. 231, 1158–1170. doi:10.1016/j.jclepro.2019.05.327
Cheng, S., Fan, W., Meng, F., Chen, J., Cai, B., Liu, G., et al. (2020). Toward Low-Carbon Development: Assessing Emissions-Reduction Pressure Among Chinese Cities. J. Environ. Manage. 271, 111036. doi:10.1016/j.jenvman.2020.111036
Chu, J., Shao, C., Emrouznejad, A., Wu, J., and Yuan, Z. (2021). Performance Evaluation of Organizations Considering Economic Incentives for Emission Reduction: A Carbon Emission Permit Trading Approach. Energ. Econ. 101, 105398. doi:10.1016/j.eneco.2021.105398
Fan, Y., Wu, J., Xia, Y., and Liu, J.-Y. (2016). How Will a Nationwide Carbon Market Affect Regional Economies and Efficiency of CO2 Emission Reduction in China? China Econ. Rev. 38, 151–166. doi:10.1016/j.chieco.2015.12.011
Fang, K., Dong, L., Ren, J., Zhang, Q., Han, L., and Fu, H. (2017). Carbon Footprints of Urban Transition: Tracking Circular Economy Promotions in Guiyang, China. Ecol. Model. 365, 30–44. doi:10.1016/j.ecolmodel.2017.09.024
Feng, M., and Li, X. (2019). Technological Innovation Threshold Characteristic of the Impact of Environmental Regulation on Carbon Emission Based on Chinese Provincial Panel Data. J. Scientific Ind. Res. 78, 274–277.
Fu, Y., He, C., and Luo, L. (2021). Does the Low-Carbon City Policy Make a Difference? Empirical Evidence of the Pilot Scheme in China with DEA and PSM-DID. Ecol. Indicators 122, 107238. doi:10.1016/j.ecolind.2020.107238
Gehrsitz, M. (2017). The Effect of Low Emission Zones on Air Pollution and Infant Health. J. Environ. Econ. Manage. 83, 121–144. doi:10.1016/j.jeem.2017.02.003
Hu, J., Pan, X., and Huang, Q. (2020). Quantity or Quality? the Impacts of Environmental Regulation on Firms' Innovation-Quasi-Natural experiment Based on China's Carbon Emissions Trading Pilot. Technol. Forecast. Soc. Change 158, 120122. doi:10.1016/j.techfore.2020.120122
Jacobson, L. S., LaLonde, R. J., and Sullivan, D. G. (1993). Earnings Losses of Displaced Workers. Am. Econ. Rev. 83, 685–709.
Jiang, Y., Zhou, Z., and Liu, C. (2019). The Impact of Public Transportation on Carbon Emissions: a Panel Quantile Analysis Based on Chinese Provincial Data. Environ. Sci. Pollut. Res. 26 (4), 4000–4012. doi:10.1007/s11356-018-3921-y
Kaufmann, R. K., Gopal, S., Tang, X., Raciti, S. M., Lyons, P. E., Geron, N., et al. (2013). Revisiting the Weather Effect on Energy Consumption: Implications for the Impact of Climate Change. Energy Policy 62, 1377–1384. doi:10.1016/j.enpol.2013.07.056
Kou, Z. L., and Liu, X. Y. (2017). FIND Report on City and Industrial Innovation in China Fudan Institute of Industrial Development.
Kennedy, C., and Corfee-Morlot, J. (2013). Past Performance and Future Needs for Low Carbon Climate Resilient Infrastructure- an Investment Perspective. Energy Policy 59, 773–783. doi:10.1016/j.enpol.2013.04.031
Li, K., Ma, M., Xiang, X., Feng, W., Ma, Z., Cai, W., et al. (2022). Carbon Reduction in Commercial Building Operations: A Provincial Retrospection in China. Appl. Energ. 306, 118098. doi:10.1016/j.apenergy.2021.118098
Li, M., Ahmad, M., Fareed, Z., Hassan, T., and Kirikkaleli, D. (2021). Role of Trade Openness, export Diversification, and Renewable Electricity Output in Realizing Carbon Neutrality Dream of China. J. Environ. Manage. 297, 113419. doi:10.1016/j.jenvman.2021.113419
Li, X., Hu, Z., and Cao, J. (2021). The Impact of Carbon Market Pilots on Air Pollution: Evidence from China. Environ. Sci. Pollut. Res. 28, 62274–62291. doi:10.1007/s11356-021-14995-z
Li, X., Hu, Z., Cao, J., and Xu, X. (2022). The Impact of Environmental Accountability on Air Pollution: A Public Attention Perspective. Energy Policy 161, 112733. doi:10.1016/j.enpol.2021.112733
Li, X., and Wang, D. (2022). Does Transfer Payments Promote Low‐Carbon Development of Resource‐Exhausted Cities in China? Earth's Future 10, 1–20. doi:10.1029/2021EF002339
Liao, L., Zhao, C., Li, X., and Qin, J. (2021). Towards Low Carbon Development: The Role of forest city Constructions in China. Ecol. Indicators 131, 108199. doi:10.1016/j.ecolind.2021.108199
Lipp, J. (2007). Lessons for Effective Renewable Electricity Policy from Denmark, Germany and the United Kingdom. Energy Policy 35, 5481–5495. doi:10.1016/j.enpol.2007.05.015
Liu, P., and Ravenscroft, N. (2017). Collective Action in Implementing Top-Down Land Policy: The Case of Chengdu, China. Land Use Policy 65, 45–52. doi:10.1016/j.landusepol.2017.03.031
Liu, X., Wahab, S., Hussain, M., Sun, Y., and Kirikkaleli, D. (2021). China Carbon Neutrality Target: Revisiting FDI-Trade-Innovation Nexus with Carbon Emissions. J. Environ. Manage. 294, 113043. doi:10.1016/j.jenvman.2021.113043
Lo, K. (2014). China's Low-Carbon City Initiatives: The Implementation gap and the Limits of the Target Responsibility System. Habitat Int. 42, 236–244. doi:10.1016/j.habitatint.2014.01.007
Lozano, S., and Gutiérrez, E. (2008). Non-parametric Frontier Approach to Modelling the Relationships Among Population, GDP, Energy Consumption and CO2 Emissions. Ecol. Econ. 66, 687–699. doi:10.1016/j.ecolecon.2007.11.003
Lv, Q., Liu, H., Wang, J., Liu, H., and Shang, Y. (2020). Multiscale Analysis on Spatiotemporal Dynamics of Energy Consumption CO2 Emissions in China: Utilizing the Integrated of DMSP-OLS and NPP-VIIRS Nighttime Light Datasets. Sci. Total Environ. 703, 134394. doi:10.1016/j.scitotenv.2019.134394
Ma, D., Fei, R., and Yu, Y. (2019). How Government Regulation Impacts on Energy and CO2 Emissions Performance in China's Mining Industry. Resour. Pol. 62, 651–663. doi:10.1016/j.resourpol.2018.11.013
Opschoor, H. (2011). Local Sustainable Development and Carbon Neutrality in Cities in Developing and Emerging Countries. Int. J. Sustain. Dev. World Ecol. 18, 190–200. doi:10.1080/13504509.2011.570800
Qiu, S., Wang, Z., and Liu, S. (2021). The Policy Outcomes of Low-Carbon City Construction on Urban green Development: Evidence from a Quasi-Natural experiment Conducted in China. Sustain. Cities Soc. 66, 102699. doi:10.1016/j.scs.2020.102699
Qu, Y., and Liu, Y. (2017). Evaluating the Low-Carbon Development of Urban China. Environ. Dev. Sustain. 19, 939–953. doi:10.1007/s10668-016-9777-8
Rickels, W., Reith, F., Keller, D., Oschlies, A., and Quaas, M. F. (2018). Integrated Assessment of Carbon Dioxide Removal. Earth's Future 6, 565–582. doi:10.1002/2017ef000724
Shen, L., Wu, Y., Lou, Y., Zeng, D., Shuai, C., and Song, X. (2018). What Drives the Carbon Emission in the Chinese Cities? A Case of Pilot Low Carbon City of Beijing. J. Clean. Prod. 174, 343–354. doi:10.1016/j.jclepro.2017.10.333
Shin, K. (2018). Environmental Policy Innovations in China: a Critical Analysis from a Low-Carbon City. Environ. Polit. 27, 830–851. doi:10.1080/09644016.2018.1449573
Song, M., Zhao, X., and Shang, Y. (2020). The Impact of Low-Carbon City Construction on Ecological Efficiency: Empirical Evidence from Quasi-Natural Experiments. Resour. Conservation Recycling 157, 104777. doi:10.1016/j.resconrec.2020.104777
Su, C.-W., Yuan, X., Tao, R., and Umar, M. (2021). Can New Energy Vehicles Help to Achieve Carbon Neutrality Targets? J. Environ. Manage. 297, 113348. doi:10.1016/j.jenvman.2021.113348
Sun, H., Edziah, B. K., Kporsu, A. K., Sarkodie, S. A., and Taghizadeh-Hesary, F. (2021a). Energy Efficiency: The Role of Technological Innovation and Knowledge Spillover. Technol. Forecast. Soc. Change 167, 120659. doi:10.1016/j.techfore.2021.120659
Sun, H., Edziah, B. K., Sun, C., and Kporsu, A. K. (2021b). Institutional Quality and its Spatial Spillover Effects on Energy Efficiency. Socio-Economic Plann. Sci. 261, 101023. doi:10.1016/j.seps.2021.101023
Sun, H., Edziah, B. K., Sun, C., and Kporsu, A. K. (2019). Institutional Quality, green Innovation and Energy Efficiency. Energy Policy 135, 111002. doi:10.1016/j.enpol.2019.111002
Tang, H., Zhang, S., and Chen, W. (2021). Assessing Representative CCUS Layouts for China's Power Sector toward Carbon Neutrality. Environ. Sci. Technol. 55 (16), 11225–11235. doi:10.1021/acs.est.1c03401
Tang, P., Yang, S., Shen, J., and Fu, S. (2018). Does China's Low-Carbon Pilot Programme Really Take off? Evidence from Land Transfer of Energy-Intensive Industry. Energy Policy 114, 482–491. doi:10.1016/j.enpol.2017.12.032
van der Heijden, J. (2016). Experimental Governance for Low-Carbon Buildings and Cities: Value and Limits of Local Action Networks. Cities 53, 1–7. doi:10.1016/j.cities.2015.12.008
van der Vlist, A. J., Withagen, C., and Folmer, H. (2007). Technical Efficiency under Alternative Environmental Regulatory Regimes: The Case of Dutch Horticulture. Ecol. Econ. 63, 165–173. doi:10.1016/j.ecolecon.2006.10.013
Wang, H., Chen, Z., Wu, X., and Nie, X. (2019). Can a Carbon Trading System Promote the Transformation of a Low-Carbon Economy under the Framework of the porter Hypothesis? Empirical Analysis Based on the PSM-DID Method. Energy Policy 129, 930–938. doi:10.1016/j.enpol.2019.03.007
Wang, L., Chang, H.-L., Rizvi, S. K. A., and Sari, A. (2020). Are Eco-Innovation and export Diversification Mutually Exclusive to Control Carbon Emissions in G-7 Countries? J. Environ. Manage. 270, 110829. doi:10.1016/j.jenvman.2020.110829
Wang, Y., Fang, X., Yin, S., and Chen, W. (2021). Low-carbon Development Quality of Cities in China: Evaluation and Obstacle Analysis. Sustain. Cities Soc. 64, 102553. doi:10.1016/j.scs.2020.102553
Wang, Y., Song, Q., He, J., and Qi, Y. (2015). Developing Low-Carbon Cities through Pilots. Clim. Pol. 15, S81–S103. doi:10.1080/14693062.2015.1050347
Wilmers, F. (1990). Effects of Vegetation on Urban Climate and Buildings. Energy and Buildings 15, 507–514. doi:10.1016/0378-7788(90)90028-H
Wirth, C., Chi, J., and Young, M. (2013). The Economic Impact of Capital Expenditures: Environmental Regulatory Delay as a Source of Competitive Advantage? J. Business Finance Account. 40, 115–141. doi:10.1111/jbfa.12009
Wu, P., Guo, F., Cai, B., Wang, C., Lv, C., Liu, H., et al. (2021). Co-benefits of Peaking Carbon Dioxide Emissions on Air Quality and Health, a Case of Guangzhou, China. J. Environ. Manage. 282, 111796. doi:10.1016/j.jenvman.2020.111796
Yang, L., and Li, Y. (2013). Low-carbon City in China. Sustain. Cities Soc. 9, 62–66. doi:10.1016/j.scs.2013.03.001
Yao, S., and Zhang, Z. (2001). On Regional Inequality and Diverging Clubs: A Case Study of Contemporary China. J. Comp. Econ. 29, 466–484. doi:10.1006/jcec.2001.1726
Yu, L. (2014). Low Carbon Eco-City: New Approach for Chinese Urbanisation. Habitat Int. 44, 102–110. doi:10.1016/j.habitatint.2014.05.004
Yu, X., Shen, M., Wang, D., and Imwa, B. T. (2019). Does the Low-Carbon Pilot Initiative Reduce Carbon Emissions? Evidence from the Application of the Synthetic Control Method in Guangdong Province. Sustainability 11, 3979. doi:10.3390/su11143979
Zhang, R., and Hanaoka, T. (2021). Deployment of Electric Vehicles in China to Meet the Carbon Neutral Target by 2060: Provincial Disparities in Energy Systems, CO2 Emissions, and Cost Effectiveness. Resour. Conservation Recycling 170, 105622. doi:10.1016/j.resconrec.2021.105622
Zhang, S., Ma, M., Li, K., Ma, Z., Feng, W., and Cai, W. (2022). Historical Carbon Abatement in the Commercial Building Operation: China versus the US. Energ. Econ. 105, 105712. doi:10.1016/j.eneco.2021.105712
Zhang, X. X., Wu, P. F., and Chen, B. (2010). Relationship between Vegetation Greenness and Urban Heat Island Effect in Beijing City of China. Proced. Environ. Sci. 2, 1438–1450. doi:10.1016/j.proenv.2010.10.157
Zhao, C., Deng, M., and Cao, X. (2021). Does Haze Pollution Damage Urban Innovation? Empirical Evidence from China. Environ. Sci. Pollut. Res. 28 (13), 16334–16349. doi:10.1007/s11356-020-11874-x
Keywords: low-carbon city policy, carbon emission, carbon neutrality, Government behaviors, nighttime light data, difference-in-differences model
Citation: Li X, Zhao C and Huang M (2022) Reassessing the Effect of Low-Carbon City Policy in China: New Evidence From the Nighttime Light Data. Front. Energy Res. 9:798448. doi: 10.3389/fenrg.2021.798448
Received: 21 October 2021; Accepted: 31 December 2021;
Published: 24 January 2022.
Edited by:
Fateh Belaid, King Abdullah Petroleum Studies and Research Center (KAPSARC), Saudi ArabiaCopyright © 2022 Li, Zhao and Huang. This is an open-access article distributed under the terms of the Creative Commons Attribution License (CC BY). The use, distribution or reproduction in other forums is permitted, provided the original author(s) and the copyright owner(s) are credited and that the original publication in this journal is cited, in accordance with accepted academic practice. No use, distribution or reproduction is permitted which does not comply with these terms.
*Correspondence: Chunkai Zhao, Y2t6X3poYW9AMTYzLmNvbQ==
†ORCID: Xing Li, orcid.org/0000-0002-3428-983X; Chunkai Zhao, orcid.org/0000-0003-1918-9598