- 1State Grid Jiangsu Electric Power Co., Ltd., Nanjing, China
- 2Jiangsu Frontier Electric Power Technology, Co., Ltd., Nanjing, China
- 3College of Electrical and Information Engineering, Hunan University, Changsha, China
Electricity market reform provides the conditions for demand-side load resources to be incorporated into the supply–demand regulation, and the increase of residential-side electrification level makes residential load resources a high-quality resource for demand response (DR). Resident home appliances participate in the “two-way interaction” of the power grid in the form of DR, which can effectively alleviate the tension of power supply and consume clean energy, to improve the safe and stable operation of the power system. Firstly, this article summarizes the structure and functions of the home energy management system (HEMS). Secondly, it discusses the key technologies of the HEMS, starting from an advanced metering infrastructure (AMI) and DR technology. Finally, it analyzes the control strategies of the HEMS, including component models and various optimal scheduling algorithms, and describes the challenges of the HEMS.
Introduction
In May 2009, the State Grid Corporation of China proposed to build a strong smart grid with the characteristics of informatization, digitization, automation, and interaction (Liu et al., 2009). Smart grids can realize real-time monitoring and analysis of user power consumption information, and smart power services have gradually begun to realize the extensive participation of users and autonomous responses to demands (Tian et al., 2014). The reform and development of electricity marketization have gradually diversified the stakeholders of the power system, and demand-side resources have emerged as important, so demand response (DR) has emerged. Since 2011, residential household energy consumption has grown faster than that in the industrial sector, and households have become one of the most critical factors influencing the management of sustainable development. In 2019, the electricity consumption of the whole society in China was 7,225.5 billion kWh, an increase of 4.5% year-on-year, and the electricity consumption of urban and rural residents was 1,025 billion kWh, accounting for 14.2% of the total electricity consumption of the society (National Energy Administr, 2019). Residential load resources on the demand side have become important resources for DR.
Stamminger et al. (2008) pointed out that residential and commercial electricity consumption in the United States accounted for about 72%, of which at least 30% of electricity consumption can be avoided. In China, with the development and popularization of electric vehicles and distributed power generation, the energy within the family will gradually become diversified and complex in the future, and a large part of energy waste can be avoided under reasonable planning. Reasonable arrangement of the orderly work of household electrical equipment through the home energy management system (HEMS), which can assist users in controlling the energy flow and load dispatching in the system, helps in mobilizing users’ enthusiasm for participating in DR and accelerating the on-site distribution of distributed energy consumption (Zhang et al., 2021).
The development of smart grid technology and the rise of residential energy consumption provide opportunities and challenges for HEMS research, and some countries, such as the United States and Germany, have started to conduct in-depth research on intelligent power utilization and HEMS. Due to the different background environment, development technology, and storage capacity of new energy sources in each country, each country presents different development characteristics, as shown in Table 1 (Chen et al., 2019).
At present, many researchers and scientific research institutions worldwide are studying the HEMS participating in DR. This article first summarizes the structure and function of the HEMS. Second, it discusses the key technologies of the HEMS, starting from the two aspects of AMI and DR technology. The article finally analyzes the control strategies of the HEMS, including component models, various optimization scheduling algorithms, and a description of the challenges for the HEMS.
Structure and Function of HEMS
HEMS Network Structure
In the traditional power consumption mode, there is only a one-way flow from the grid to the user side, and there are disadvantages to varying degrees on both the user side and the power supply side, as shown in Figure 1. Compared with the traditional model, the HEMS is an intelligent network control system that can integrate all power generation, power consumption, and energy storage equipment in the home for control and management, which can improve the power efficiency of the user, change the power consumption habits of the user, reduce the user’s electricity bill, and realize two-way communication with the grid, two-way energy flow, etc. (Li et al., 2020; Zhang et al., 2016), and the network structure is shown in Figure 2 (Li et al., 2015a).
The energy flow relationship between the various components of the HEMS and between it and the external power grid is shown in Figure 3. The HEMS components include the electricity consumption load, the energy storage device, and the distributed power supply. The collection module uploads the collected electricity consumption information to the home host.
By collecting electricity consumption information, tariff information from power-related departments, user setting information, etc., the home host realizes optimal management of the entire system operation and gives users the most economical and comfortable scheduling strategy. Users can read the power consumption of specific power-using devices, view the real-time status of distributed power and energy storage devices through cell phones, personal computers, etc., and perform relevant operations according to specific needs.
The interaction between the HEMS and power-related departments is reflected in the exchange of energy and information. Users can receive information sent by the power department through wired and wireless transmission to arrange daily electricity consumption; the HEMS can upload the user’s electricity consumption information to facilitate the relevant power departments to grasp the user’s electricity consumption information in real time and arrange reasonable electricity production and transmission through this analysis.
Functions of HEMS
The HEMS mainly includes the following five functional modules: monitoring, recording, control, management, and alarm, as shown in Figure 4, and the specific description is as follows (Son and Moon, 2010; Li, 2016).
(1) Monitoring module. It monitors the energy consumption in real time and displays the working mode and energy status of household appliances. (2) Recording module. It saves electricity consumption data such as home appliances, distributed energy, and energy storage status. (3) Control module. It is divided into direct control and remote control. Direct control includes control equipment and control system, and remote control means that users can access the usage mode and equipment status of home appliances online. (4) Alarm module. If there is an abnormal situation, an alarm will be generated and sent to the HEMS center. (5) Management module. It contains various services to improve the optimized operation and power usage efficiency of residential households.
Key Technology of HEMS
Advanced Measurement System
AMI Structure
The advanced metering infrastructure (AMI) is a set of control processing systems used to collect, measure, analyze, and store user electricity consumption information and grid electricity price information, including four main components: smart meter, communication network, meter data management system (MDMS), and home area network (HAN), and its typical structure is shown in Figure 5, which can realize two-way interaction between household energy information and the grid (Zhao et al., 2010; Yang, 2015; Peng et al., 2017).
Various components of the AMI are connected through the network to realize the two-way transmission and power control of user electricity information and electricity price information necessary in the HEMS and realize the automation and intelligence of DR.
Composition of AMI
1) Smart meter. It is equivalent to a sensor installed on the user side. Based on the measurement, communication, and calculation, it can realize not only traditional functions such as power recording but also real-time collection, measurement, display, and storage of power information, real-time bidirectional metering, automatic billing, monitoring of power quality and power supply reliability, analysis of faults for prediction, remote connection and disconnection, power theft detection, and other functions. It is also an important part of the advanced measurement system (Luan et al., 2014).
The smart meter can be used as a communication gateway between the power company and the user’s indoor network, allowing users to view electricity consumption information and receive electricity price information in near real time, which is of great significance to the HEMS. The schematic diagram of the smart meter function is shown in Figure 6, which has the following functions: two-way metering function, two-way communication function, and user load control function, in addition to anti-theft detection, dynamic display of electricity consumption information, remote time synchronization update, software upgrade, and other functions.
2) Communication network. A safe and stable communication network serves as a basic bridge for information interaction between power companies, users, and controllable power loads. The AMI uses a fixed two-way communication network, which is divided into remote channels and local channels. The remote channel realizes the transmission of residential electricity information to the power company and connects the information of the data concentrator with the data center. The commonly used methods are mainly optical fiber and telephone lines, radio waves, etc. The local channel is the communication line between the data concentrator and the smart meter, and the main methods used are power line carrier, wireless, and RS485.
3) MDMS. It is a database with analysis tools, which is the “nerve center” of AMI. It is used in conjunction with the AMI automatic data collection system through an enterprise service bus to obtain and store the metering value of the electricity meter, to realize network reconstruction, power theft analysis, and fault prediction. Stable operation, asset management, and other advanced applications provide a reliable guarantee.
4) HAN. It is a bridge that realizes the information interaction between indoor intelligent interactive terminals, intelligent electrical energy meters, and household electrical equipment, making the entire HEMS into a whole. Due to the relatively random placement of indoor equipment, which is not suitable for wired communication, and the variety of household loads and the wide range of residents’ lives, it is more appropriate to use a wireless network in the HEMS. The communication technology suitable for the HAN is very large. The commonly used wireless communication methods include ZigBee, WiFi, and Bluetooth. Table 2 shows a comparison of representative communication technologies (Wu et al., 2011; Ji, 2017). ZigBee has greater advantages in power consumption, cost, and networking, and its market acceptance is higher.
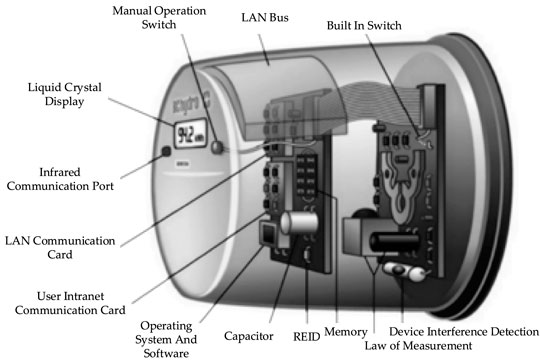
FIGURE 6. Functional schematic diagram of a smart meter (Luan, 2009).
Demand Response
DR means that electricity users respond to electricity price guidance or incentive mechanisms and adjust electricity consumption methods to achieve peak shaving and valley filling, so that the load presents flexible characteristics, to promote the optimal allocation of power resources (Li et al., 2005; Yang et al., 2014; Yang et al., 2016). The Federal Energy Regulatory Commission classifies DR into two types: price-based demand response (PDR) and incentive-based demand response (IDR), as shown in Figure 7.
Due to the huge impact of price on consumer behavior, the price mechanism is the most sensitive mechanism in the market. The market side publishes the electricity price, and the end-user side responds to the electricity price to change the time and electricity consumption of household loads to obtain economic benefits. PDR technology has three types: time-of-use pricing (TOU), real-time pricing (RTP), and critical peak pricing (CPP).
IDR usually refers to an incentive measure formulated by independent operators or power companies to incentivize power users under power shortage or other special circumstances to reduce the operation of home appliances, thereby reducing the operating pressure of the power system. This type of compensation is usually implemented utilizing direct economic compensation or preferential electricity prices. Therefore, this kind of control measure avoids the possibility of large-scale power outages and brings economic benefits to power users.
IDR strategies are divided into plan-based incentives and market-based incentives. Plan-based incentives can be subdivided into direct load control (DLC) and interruptible load (IL); market-based incentives can be further subdivided into demand side bidding (DSB), emergency demand response (EDR), capacity market program (CMP), and ancillary service market program (ASMP). Due to the United States’ advantages in mechanism, technology, and implementation time, all types of DR projects have been implemented in the United States.
HEMS Control Strategy
Equivalent Model of HEMS
Photovoltaic Cell Model
The output power of photovoltaic cells is related to solar irradiance and temperature:
where PPV(t) is the photovoltaic output power; Pmax is the maximum output power under standard test conditions; G(t) is the current solar irradiance; GSTC is the rated solar irradiance; k is the temperature coefficient; T(t) is the temperature of the battery assembly at the current moment; Tair(t) is the ambient temperature; TSTC is the rated reference temperature; and VW is the current wind speed.
Energy Storage Device and Electric Vehicle Model
The energy storage device participates in scheduling through charging and discharging, and the change in the state of charge is used to reflect the remaining capacity of the energy storage device:
where SOCB(t) is the state of charge of the energy storage device during the t period; ηBc, PBc(t) are the charging efficiency and power of the energy storage device, respectively; ηBd, PBd(t) are the discharge efficiency and power of the energy storage device, respectively; and SB(t) and EB are the switching state and rated capacity of the energy storage device, respectively. The electric vehicle (EV) model is similar to the battery, so we will not repeat it here.
Load Model
Resident loads can be divided into flexible loads and rigid loads. The flexible load refers to the load that can change their own electricity consumption behavior according to the needs of the grid within a certain range (Li et al., 2015b). The rigid load is an uncontrollable load. Flexible load resources usually adopt load dispatching methods of peak shifting and peak avoiding. According to load response characteristics, the peak shifting load includes the shiftable load and transferable load. Users stagger their own power consumption peak from the peak of the power grid load, to realize peak cutting and valley filling. The peak avoiding load is a load that can be reduced to reduce power consumption during peak hours.
Air conditioners and water heaters are typical flexible load resources. As far as the air conditioner load is concerned, the time-varying model equation of room temperature can be obtained by deriving and solving the first-order equivalent thermal parameter model:
where Tin(t+1) is the indoor temperature at time t+1, °C; Tin(t) is the indoor temperature at time t, °C; Tout(t+1) is the outdoor temperature at time t+1, °C; R is the equivalent thermal resistance, °C/kW; C is the equivalent specific heat capacity, J/°C; ∆t is the time period, h; and
If the air conditioner works in the cooling mode, the on–off state of the air conditioner at time t can be expressed as
where ∆TAC is the temperature setting range of the air conditioner; SAC (t-1) is the on/off state of the air conditioner at t-1; and Ts(t) is the temperature value set by the user.
As far as the water heater is concerned, consider all the water in the tank as a single unit with a uniform temperature. The temperature change model of the water heater is as follows:
where c is the specific heat capacity of water, J/kg·°C; ρ is the density of water, kg/m3; Vtank is the volume of the water heater tank, mL; Tinside(t) is the water temperature in the water tank at time t, °C; Tinlet is the temperature of cold water flowing into the water tank, °C; fhot is the hot water outflow rate in the water tank, mL/s; ∆t is the duration of each time slot, min; PEWH(t) is the operating power of the water heater at time t; ηEWH is the operating efficiency of the water heater; SEWH(t) is the on–off state of the water heater at time t, whose value is 0 when it is closed and 1 when it is open; Atank is the surface area of the water heater, m2; REWH is the thermal resistance of the water heater, m2·°C /W; and Tamb is the indoor ambient temperature, °C.
The on–off state of the water heater at time t can be expressed as
where D is the heat preservation interval of the water heater; SEWH(t-1) is the on–off state of the water heater at t-1; and Tset(t) is the temperature value set by the user, Tmin ≤ Tset(t)-D&Tset(t)≤Tmax, with Tmin and Tmax being the upper and lower temperature limits of the water heater.
Optimal Scheduling Algorithm of HEMS
The family energy management strategy aims to optimize the load usage in the family. Household energy management is a mixed-integer non-linear programming problem. Different mathematical methods or intelligent algorithms can be used to solve household energy scheduling problems, such as the artificial bee colony algorithm, genetic algorithm, particle swarm optimization algorithm, dragonfly optimization algorithm, simulated annealing algorithm, and tabu search algorithm. Optimal scheduling objectives can be divided into two aspects. On the one hand, it is considered from the perspective of users. The main purpose is to reduce user electricity costs and improve user comfort. On the other hand, it is considered from the power system, including increasing the utilization rate of renewable energy and reducing the peak ratio during peak electricity consumption.
Reducing Residential Electricity Costs
1) Predicted dynamic electricity prices and residential electricity consumption habits are used as indicators to adjust the operation of electricity-using equipment to reduce customers’ electricity costs. Kim and Poor (2011) formulated the dispatching problem as a Markov decision process based on past and current electricity prices so as to derive the optimal strategy and used algorithms to derive price thresholds for each time period that could bring economic benefits to electricity consumers. Lee et al. (2013) predicted the user’s home appliance usage habits through the user’s power consumption data and Bayesian theorem, so that the user can reasonably reduce the electrical appliance energy consumption, so as to reduce the power consumption cost.
2) According to the output status of renewable energy power generation, coordinated control of electrical equipment increases the utilization of low-grade renewable energy and reduces residential electricity costs. Wang et al. (2015) proposed a household energy–coordinated scheduling strategy including photovoltaic and energy storage equipment, including photovoltaic forecasting and household load forecasting, and the use of particle swarm algorithms to optimize the energy dispatch of users with the goal of maximum household energy profitability. Table 3 shows that this strategy increases the proportion of local photovoltaic consumption while increasing user revenue. Xu et al. (2017) considered the power grid, photovoltaic power generation, and energy storage as energy sources, established a multi-objective mixed-integer non-linear optimization model, and proposed an intelligent solution method based on the adaptive particle swarm optimization algorithm (APSOA), which can minimize household power consumption.
3) Other situations include collaborative scheduling of EVs and energy storage equipment. Yao et al. (2020) proposed two modes of ground control strategies for cooperative dispatching of EVs and energy storage devices. Mode 1 is a charging-only storage device for EVs; mode 2 adopts the control strategy of “charging before discharging” for EVs, which further improves the economy and flexibility. In addition, the HEMS can also improve the economy of user energy consumption by reducing the idle loss of load and transfer the working time of some loads from a “high price period” to a “low price period.”

TABLE 3. Electricity consumption model revenue data (Wang et al., 2015).
Improving User Comfort
Chen et al. (2012) proposed the concept of operational comfort level (OCL) and developed a minimum load scheduling algorithm, which can achieve peak load transfer and maximize the residents’ OCL. Liu et al. (2015) proposed an optimal scheduling model for home appliances based on real-time electricity prices, which can achieve scheduling including satisfaction and economy, as well as scheduling with the least carbon dioxide emissions. Nguyen and Le (2014) discussed the energy dispatch optimization problem of household appliances such as EVs, air conditioners, and water heaters, which can realize the integration of user comfort and electricity cost.
Improving the Utilization of Renewable Energy
Huang et al. (2015) researched household users including wind power and photovoltaic power generation. Based on TOU, fully considering the economy of electricity consumption and user comfort, a demand-side response method was proposed, which greatly improved the utilization rate of wind power. Zong et al. (2013) proposed a distributed active demand-side management controller based on an artificial neural network. The system includes photovoltaic, energy storage, and home automation systems, which can coordinate user preferences and predict power generation. Therefore, the operation of home appliances can be arranged reasonably, and the utilization rate of photovoltaic energy can be improved.
Reducing Peak Ratios During Peak Electricity Consumption Periods
Latif et al. (2018) proposed a bat genetic algorithm (BGA) based on TOU, which shifts household appliances from the period of high peak electricity prices to the period of low electricity prices; it has an obvious effect on reducing the peak ratio and residential power cost in the peak period. Tu et al. (2019) took the incentive mechanism of residents’ load participating in power grid peak shaving as the starting point and designed the incentive model and optimization strategy of peak shaving and valley filling, which can reduce the peak ratio in the peak period while considering the user’s power consumption cost and realize the friendly interaction of “network load.”
Challenges for HEMS
At present, there have been many studies on HEMS control strategies, but the application and promotion of the HEMS are still in the process of exploration:
1) The penetration rate of energy equipment in households is low, and how to promote household energy equipment and transform the existing household power supply system on a large scale is also a problem to be solved.
2) Household energy consumption information is lacking, and DR is insufficient. In the residential DR, accurate demand response and behavior analysis are still issues that need attention. On the premise of ensuring the comfort of power consumption, providing users with power-saving measures and effective energy consumption suggestions will help mobilize the enthusiasm of residential users to participate in the demand-side response and enhance the friendly interaction between the power grid and users, which is of great significance.
3) A reasonable incentive mechanism is lacking. Although there are many incentive mechanisms in China, including energy efficiency subsidies, differential tariffs, tiered tariffs, peak and valley tariffs, and temporary incentives for peak power load reduction, the formulation of subsidies lacks a market-based mechanism, and it is difficult to optimize the allocation of resources when subsidy standards are given. The development of the HEMS in China is still in the primary stage, and more policies, software and hardware facilities, and related supporting technologies are needed to promote the establishment of home intelligent networks.
Conclusion
The conclusions are drawn as follows:
1) The HEMS integrates all power generation, electricity consumption, and energy storage equipment in the home. The core equipment in the HEMS structure is the home host, which is the key to realizing the two-way interaction between the residential load and the power grid. It includes five functional modules: monitoring, recording, control, management, and alarm.
2) The AMI and DR technology in the smart grid are the core technology and foundation of the HEMS. The HEMS connects various home appliances, distributed power sources, energy storage, and other equipment through the AMI to monitor and manage the use of various electrical equipment and realizes the optimal management of electrical equipment in combination with DR technology. The development and implementation of these technologies have made household energy management on the residential side become of great significance, helping to save energy, reduce emissions, and cut peaks and fill valleys.
3) The component model of the HEMS is established. From the perspective of residents and the power grid, it discussed household energy optimization for the purposes of reducing user electricity costs, improving user comfort, improving renewable energy utilization, and reducing peak power consumption during peak periods. Finally, the challenges to the development of the HEMS are pointed out.
Data Availability Statement
The original contributions presented in the study are included in the article/Supplementary Material, and further inquiries can be directed to the corresponding author.
Author Contributions
YM and XC proposed the concepts and ideas. LW analyzed the results. JY wrote this paper and revised the contents of this manuscript.
Funding
This work was financially supported by the Jiangsu Electric Power Company Research Foundation under Grant No. SGJS0000YXJS2001039.
Conflict of Interest
The authors YM and XC were employed by the company State Grid Jiangsu Electric Power Co., Ltd. The author LW was employed by the company Jiangsu Frontier Electric Power Technology Co., Ltd.
The remaining author declares that the research was conducted in the absence of any commercial or financial relationships that could be construed as a potential conflict of interest.
Publisher’s Note
All claims expressed in this article are solely those of the authors and do not necessarily represent those of their affiliated organizations, or those of the publisher, the editors, and the reviewers. Any product that may be evaluated in this article, or claim that may be made by its manufacturer, is not guaranteed or endorsed by the publisher.
References
Chen, M., Guan, X., Meng, J., and Wu, W. X. (2019). Review on Home Energy Management System for Smart Grid. Building Energy Efficiency 47 (10), 117–121. doi:10.3969/j.issn.1673-7237.2019.10.023j
Chen, Y., Liu, R. P., Wang, C., de Groot, M., and Zeng, Z. (2012). IEEE, 1–6.Consumer Operational Comfort Level Based Power Demand Management in the Smart Grid, 2012 3rd IEEE PES Innovative Smart Grid Technologies Europe (ISGT Europe), 14 Oct. 2012, Berlin, Germany.
Huang, L., Wei, Z. N., Yan, Y. F., Sun, G. Q., Sun, Y. H., Liu, J. S., et al. (2013). A Survey on Interactive System and Operation Patterns of Intelligent Power Utilization. Power Syst. Technol. 37 (18), 2230–2237. doi:10.13335/j.1000-3673.pst.2013.08.005
Huang, T., Ma, X. Y., Lei, J. Y., Xu, A. D., Guo, X. B., Li, P., et al. (2015). Optimal Operation of Household User-Side Microgrid Considering Time-Of-Use Price and Demand Response. South. Power Syst. Technol. 9 (4), 47–53. doi:10.13648/j.cnki.issn1674-0629.2015.04.008
Ji, S. Y. (2017). Research on Home Energy Management for Intelligent Power Utilization. Hefei: Hefei University of Technology.
Kim, T. T., and Poor, H. V. (2011). Scheduling Power Consumption with Price Uncertainty. IEEE Trans. Smart Grid 2 (3), 519–527. doi:10.1109/tsg.2011.2159279
Latif, U., Javaid, N., Zarin, S. S., Naz, M., Jamal, A., and Mateen, A. (2018)., 667–677. Cost Optimization in Home Energy Management System Using Genetic Algorithm, Bat Algorithm and Hybrid Bat Genetic Algorithm, 2018 IEEE 32nd International Conference on Advanced Information Networking and Applications (AINA), IEEE, 16 May 2018. Krakow, Poland.
Lee, S., Ryu, G., Chon, Y., Ha, R., and Cha, H. (2013). Automatic Standby Power Management Using Usage Profiling and Prediction. IEEE Trans. Human-mach. Syst. 43 (6), 535–546. doi:10.1109/thms.2013.2285921
Li, Q., Wang, Z. X., Yan, S., Wang, C. M., Bao, L. X., and Qin, H. (2020). Optimal Operation of Home Energy Management System Considering User Comfort Preference. Acta Energiae Solaris Sinica 41 (10), 51–58.
Li, W. T. (2016). Research on Load Power Strategy of Home Energy Management Systems. Changsha: Hunan Unversity.
Li, Y. P., Zhou, J., Ju, P., and Ding, M. (2015). Quantitative Assessment Method for Interactive Impact of Flexible Load. Automation Electric Power Syst. 39 (17), 26–32+67. doi:10.7500/AEPS20140417002
Li, Y., Wang, B. B., and Li, F. X. (2015). Outlook and Thinking of Flexible and Interactive Utilization of Intelligent Power. Automation Electric Power Syst. 39 (17), 2–9. doi:10.7500/AEPS20150730004
Li, Y., Wang, B. B., and Song, H. K. (2005). Demand Side Response and its Application. Power DSM 7 (6), 13–15+18. doi:10.3969/j.issn.1009-1831.2005.06.008
Liu, J. H., He, R., and Li, R. F. (2015). Optimal Scheduling Model for home Energy Management System Based on Real-Time Electricity Pricing. Appl. Res. Comput. 32 (1), 132–137+160. doi:10.3969/j.issn.1001-3695.2015.01.030
Liu, Z. Z., Wang, M. J., and Yang, X. S. (2009). Research Status and Development Trend of Smart Grid. Power Syst. Technol. 33 (13), 1–11.
Luan, W. P., Wang, G., and Xu, D. Q. (2014). Advanced Metering Infrastructure Solution Supporting Multiple Services and Business Integration. Proc. CSEE 34 (29), 5088–5095.
National Energy Administration, National Energy Administration Releases Total Electricity Consumption in 2019. Available at: http://www.nea.gov.cn/2020-01/20/c_138720877.htm.
Nguyen, D. T., and Le, L. B. (2014). Joint Optimization of Electric Vehicle and Home Energy Scheduling Considering User Comfort Preference. IEEE Trans. Smart Grid 5 (1), 188–199. doi:10.1109/tsg.2013.2274521
Peng, X. G., Li, Z. M., Deng, X. K., and Liu, Y. (2017). Research on Advanced Metering Infrastructure under Smart Grid Framework. Guangdong Electric Power 30 (12), 7–14.
Son, Y., and Moon, K. (2010).Home Energy Management System Based on Power Line Communication, 2010 Digest of Technical Papers International Conference on Consumer Electronics (ICCE), IEEE, 9 Jan 2010, Las Vegas, NV, USA, 115–116 .
Stamminger, R., Broil, G., Pakula, C., Jungbecker, C., Braun, M., Ruidenauer, L., et al. (2008). Synergy Potential of Smart Appliances. Europe: Report of the Smart-A project.
Tang, Y., Pipattanasomporn, M., Shao, S. N., Liu, H. M., and Rahman, S. (2009). Comparative Study on Smart Grid Related R&D in China, the United States and the European Union. Power Syst. Technol.
Tian, S. M., Wang, B. B., and Zhang, J. (2014). Key Technologies for Demand Response in Smart Grid. Proc. CSEE 34 (22), 3576–3589.
Tu, J., Zhou, M., Song, X. F., Luan, K. M., and Li, G. Y. (2019). Research on Incentive Mechanism and Optimal Power Consumption Strategy for Residential Users’ Participation in Peak Shaving of Power Grid”. Power Syst. Technol. 43 (2), 443–453.
Wang, S. X., Sun, Z. Q., and Liu, Z. (2015). Co-scheduling Strategy of Home Energy for Smart Power Utilization. Automation Electric Power Syst. 39 (17), 108–113.
Wu, L., Xin, J. Q., and Wang, S. (2011). Advanced Metering Infrastructure and its Application in Demand Response. Water Resour. Power 29 (12), 170–173+216.
Xu, J. J., Wang, B. E., Yan, L. M., and Li, Z. (2017). The Strategy of the Smart Home Energy Optimization Control of the Hybrid Energy Coordinated Control. Trans. China Electrotechnical Soc. 32 (12), 214–223. doi:10.1109/tec.2017.2696979
Yang, C. S. (2015). Design on Communications and Information System of Grid and Users Bidirectional Interaction. Beijing: School of Electrical and Electronic Engineering.
Yang, S. C., Liu, J. T., Yao, Y. G., Ding, H. F., Wang, K., and Li, Y. P. (2014). Model and Strategy for Multi-Time Scale Coordinated Flexible Load Interactive Scheduling. Proc. CSEE 34 (22), 3664–3673.
Yang, X. Y., Zhou, M., and Li, G. Y. (2016). Survey on Demand Response Mechanism and Modeling in Smart Grid. Power Syst. Technol. 40 (1), 220–226.
Yao, G., Mao, Z. L., Zhou, L. D., and Li, D. (2020). Home Energy Management Strategy for Co-scheduling of Electric Vehicle and Energy Storage Device. Proc. CSU-EPSA 32 (4), 35–41+50.
Zhang, K., Zhou, B., Or, S. W., Li, C., Chung, C. Y., and Voropai, N. I. (2021). Optimal Coordinated Control of Multi-Renewable-To-Hydrogen Production System for Hydrogen Fueling Stations. IEEE Trans. Ind. Applicat., 1. doi:10.1109/TIA.2021.3093841
Zhang, Y. Y., Zeng, P., and Zang, C. Z. (2016). A Scheduling Algorithm for home Energy Management System in Smart Grid. Power Syst. Prot. Control. 44 (2), 18–26.
Zhao, H. T., Zhou, J. Y., and Y, E. K. (2010). Advanced Metering Infrastructure Supporting Effective Demand Response. Power Syst. Technol. 34 (9), 13–20.
Zong, Y., Mihetpopa, L., Kullmann, D., Thavlov, A., Gehrke, O., and Bindner, H. W. (2013). Model Predictive Controller for Active Demand Side Management with PV Self-Consumption in an Intelligent Building, IEEE PES International Conference & Exhibition on Innovative Smart Grid Technologies, IEEE, 14 Oct. 2012, Berlin, Germany.
Keywords: home energy management system, demand response, residential load, control strategy, advanced metering infrastructure
Citation: Ma Y, Chen X, Wang L and Yang J (2021) Investigation of Smart Home Energy Management System for Demand Response Application. Front. Energy Res. 9:772027. doi: 10.3389/fenrg.2021.772027
Received: 07 September 2021; Accepted: 28 September 2021;
Published: 16 November 2021.
Edited by:
Jian Zhao, Shanghai University of Electric Power, ChinaReviewed by:
Changyun Li, Shandong University of Science and Technology, ChinaHongshun Liu, Shandong University, China
Copyright © 2021 Ma, Chen, Wang and Yang. This is an open-access article distributed under the terms of the Creative Commons Attribution License (CC BY). The use, distribution or reproduction in other forums is permitted, provided the original author(s) and the copyright owner(s) are credited and that the original publication in this journal is cited, in accordance with accepted academic practice. No use, distribution or reproduction is permitted which does not comply with these terms.
*Correspondence: Jianlan Yang, Yangjianlan@hnu.edu.cn