- 1Faculty of Mechanical Engineering, Shahrood University of Technology, Shahrood, Iran
- 2Department of Renewable Energies, Faculty of New Science and Technologies, University of Tehran, Tehran, Iran
Utilizing the solar energy from photovoltaic panels integrated into a water pumping system to provide electricity for dispersed villages that have no access to backup systems not only reduces the electricity grid costs and environmental pollutants but also is an innovative approach in areas with sufficient solar irradiation. Therefore, identifying and studying the feasibility of stand-alone solar power plants in various locations is the first step in using this reliable source of energy. For this reason, 93 sites in the provinces of Razavi Khorasan, Northern Khorasan, and Southern Khorasan, Iran, were chosen by the renewable energy organization engineers as regions with high potential for establishing a solar water pumping station. The goals of this research were to prioritize the 93 sites and find the perfect location for the establishment of the aforementioned station. In order to investigate and prioritize these sites, a multi-criteria decision-making method, namely, TOPSIS (technique for order preference by similarity to ideal solution), has been used. Therefore, the geographical locations of the sites and the weather in these provinces have been studied; consequently, 15 important and essential criteria, including slope, solar irradiation, precipitation, wind speed, relative humidity, altitude, distance from substation, distance from grid, obtainable solar energy capacity, distance from river, population, distance from city center, distance from road, average temperature, and number of dams in the vicinity of the site, have been chosen. All the aforementioned criteria have been weighted using the Shannon entropy method, and then the sites have been prioritized using the TOPSIS method. The results indicated that Sarayan, Isk, Esfadan, and Dasht Byaz in Southern Khorasan and then Beydokht 2 in Razavi Khorasan are the top five locations and Shosef in Southern Khorasan is the least favorite location for establishing a solar pumping station. In order to achieve more reliable results, sensitivity analysis was implemented.
1 Introduction
Electricity is the most important form of used energy in the contemporary world (Modu et al., 2018). Due to the increment of population in developed countries and in developing ones, the global energy consumption is continuously increasing (Zhang et al., 2018). The important role of energy in the day-to-day activities of man cannot be neglected, and the scarcity of these resources has forced societies to change their consumption patterns, and behavior in utilizing energy and governments to search for new ways to save energy, reduce its ever increasing costs, and utilize alternative renewable energies (Yücenur et al., 2020). The price increment of fossil fuels, environmental considerations, energy supply security, petrochemical utilities, technological developments, and economic justifications are among the determining factors of the future of renewable energy resources (Das et al., 2017; Zhang et al., 2019a; Zhang et al., 2019b). Therefore, providing strategic management programs with the purpose of recognizing provincial and national potentials for renewable energies and existing industries is a necessity. Regarding the preparations of the infrastructure and the initial strategic decisions in the field of energy, the required steps include primary studies, analyses, decision-making, and operational stages in achieving energy security, obtaining reliable energy resources, reducing environmental harms, and, finally, a sustainable society.
Researchers have studied various renewable energy resources and different hybrid energy systems (Tong et al., 2016; Peng et al., 2021; Zhang et al., 2021). At present, solar energy is one of the cleanest and most attainable resources fulfilling the global energy demand as a renewable resource (Guezgouz et al., 2019a). Utilizing solar energy as a cheap resource in the agricultural sector is an innovative approach, and this rich energy resource can be utilized in greenhouses and agricultural machines and in mechanisms to reduce the problems stemming from fossil fuels (Nazari et al., 2018). Using this energy resource in countries such as Iran, which is located on the solar belt, is a viable option (Jamshidi and Askarzadeh, 2019).
To incentivize the financiers in the field of solar energy, the Ministry of Energy of Iran offers a higher price for the generated solar electricity. The government can propose plans to further the privatization of solar energy enterprises and even convert the energy resources of government buildings from fossil fuels to solar resources. It is the duty of governments to amass primary data and evaluate the potential of energy resources and the capability of a nation to utilize these resources because these strategic studies require considerable funding and can be utilized as a leverage in policy-making and managing private investors.
The agricultural development of a region requires water transmission, which is directly tied to the establishment of an energy system (Wang and Lyu, 2021). Establishing a photovoltaic (PV) power plant for pumping water is a viable method for utilizing solar energy (Zhang et al., 2019c). A solar pumping system usually consists of solar modules, a pumping system, a reservoir tank, and an inverter. Solar panels absorb solar irradiation and directly convert it into electricity. The inverter converts the output electricity from the solar panel into the electricity required to start the pump, and when the energy supply is greater than its demand, this residual energy is used to pump water to a reservoir with high gravitational potential energy (Evans et al., 2012; Chandel et al., 2015). One of the effective features of the economic analysis of combined systems is the ability to utilize the residual energy for other purposes. Solar pumping systems are among the cheapest solutions for irrigation in regions that have no access to an electricity grid or where it is difficult or expensive to supply electricity through a grid (Guezgouz et al., 2019b). The aim of this article was to study the feasibility of a PV pumping system via a multi-criteria decision-making (MCDM) method, namely, TOPSIS (technique for order preference by similarity to ideal solution), and the end goal of the MCDM method is to prioritize the candidates using different criteria (Babatunde et al., 2019).
1.1 Literature Review
Maleki et al. (2016) provided a model for the optimal electrification of a far-flung area in Namin, Ardabil Province, Iran, in accordance with minimal costs and maximal efficiency of the power supply. The results indicated that PV systems, wind turbines, and fuel cells were the more cost-effective options to supply electricity. The results of Peng et al. (2018) indicated that combined optimization methods offered the best performance, which led to reduced costs and increased reliability of the system and also provided desalinated water. Ma et al. (2014) conducted an accurate feasibility study of a solar wind energy system combined with battery storage for an island. Additionally, the effects of the size of solar panels and wind turbine on the economic performance and reliability of the system had been studied via HOMER (Hybrid Optimization of Multiple Energy Resources) software. Shezan et al. (2016) simulated a combined solar wind off-grid system for a remote village located in Selangor Province, Malaysia. Maleki et al. (2017) performed a case study in the Eastern region of Iran to discover the best location for an off-grid PV system in remote rural areas considering technical, environmental, and economic criteria using an artificial bee swarm algorithm. Their results approved the utility of the aforementioned method for off-grid electricity in remote villages. There are several research works covering this subject, which are provided in Table 1.
As is obvious from Table 1 regarding the methods chosen for decision-making, researchers have provided various novel methods to further improve the accuracy of the decision-making process (Demirel et al., 2018; Deveci et al., 2020; Deveci et al., 2021; Krishankumar et al., 2021). In a study to find the perfect location for a bread factory in Istanbul, a framework based on geographic information system (GIS) and MCDM methods was introduced (Deveci et al., 2018). In other research works on finding the perfect access mode to the newly constructed Istanbul Airport, a novel fuzzy level-based weight assessment/weighted aggregated sum product assessment/Heronian (LBWA-WASPAS-H) decision-making model was provided by Pamucar et al. (2020). In the same framework, Shahraki Shahdabadi et al. (2021) utilized an MCDM method to locate the site of a biomass-powered electricity generation system in a vast region. They implemented TOPSIS, ELECTRE (elimination et choix traduisant la realité), and simple additive weighting (SAW) to achieve their objective.
According to the numerous research works on solar pumping systems, there are several criteria in finding the best location for a solar pumping station required to be considered simultaneously (Zhangab et al., 2019). The most important limiting factors for implementing this technology are the differences in the elevation of the higher and lower reservoir tanks and the space limitations for the building of two big tanks (Ibrahim et al., 2008). The main drawbacks of this system are the high capital cost (Reca et al., 2016) and the alternating nature of solar energy, especially on cloudy or rainy days (Muhsen et al., 2017a; Zhangab et al., 2019). On the other hand, the carbon emissions and final energy costs of PV systems in remote areas are lower and their longer life span makes them good options (Muhsen et al., 2017b). If the solar pumping systems are properly situated, they will be superior alternatives to the diesel pump stations in far-flung regions (Yu et al., 2011).
1.2 Objectives and Novelty of the Research
Identifying and studying the feasibility of stand-alone solar power plants in various locations comprise the primary stage of utilizing solar energy. For this reason, 93 sites in three of the Eastern and North Eastern provinces of Iran were chosen by the renewable energy organization engineers as high-potential sites for establishing a solar-powered water pumping station (Table 2). The objective of this research was to prioritize these 93 sites to determine the ultimate location for the aforementioned station. Therefore, an MCDM method, namely, TOPSIS, was used. As the name suggests, an MCDM method requires multiple criteria to prioritize selected locations accordingly. In this regard, the geographical locations of the sites and the weather in these provinces have been studied. Consequently, 15 important and essential criteria, including the slope, solar irradiation, precipitation, wind speed, relative humidity, altitude, distance from the substation, distance from the grid, obtainable solar energy capacity, distance from the river, population, distance from the city center, distance from a road, average temperature, and the number of dams in the vicinity of the site, were chosen. All of the aforementioned criteria were weighted using the Shannon entropy method, and then the sites were prioritized using the TOPSIS method. Finally, to achieve more reliable results, sensitivity analysis was performed.
Considering the scarcity of research in the field of combined systems with more than one power source, such as solar pumping plants, and the fact that the Eastern provinces of Iran have not been studied before, the goal of this research was to investigate the most relevant and effective criteria in finding the optimal location for a solar pumping plant in three provinces in the east of Iran: Razavi Khorasan, Southern Khorasan, and Northern Khorasan. In this research, 15 essential criteria and parameters for a PV pumping plant have been considered for the 93 sites chosen by the engineers of the Renewable Energy and Energy Efficiency Organization of Iran (SATBA). The main difference between this research and others is that the potential of the cities in these provinces have not been investigated separately and in combination via TOPSIS and the perfect location has not been chosen. Furthermore, previous works did not include sensitivity analysis as extensively as provided in this research, and their criteria were not thoroughly assessed.
Section 2 is dedicated to the region under investigation, the selection of the criteria and decision options, and investigation of the selected criteria and decision-making matrix. Section 3 is dedicated to the methodology, indicating the weight of criteria via Shannon entropy, and the TOPSIS decision-making model. The final section includes the results and conclusion.
2 The Study Area
The main source of the supply of electricity through PV panels is solar irradiation. According to the SATBA, Razavi Khorasan, Northern Khorasan, and Southern Khorasan have approximately 300 sunny days each year, which indicates the high potential of solar energy in these regions (SATBA, 2021). Agricultural, industrial, and public demand for water has always been an issue in society, especially in rural regions. Most of the cities in the study area have access to underground water reservoirs, which require pumps to extract water. In desert or mountainous regions of the study area, which have difficulty accessing the electricity grid, extracting water from deep underground water reservoirs using solar pumping systems appears like a viable option. Therefore, establishing a solar power plant according to the geographical location and weather in these regions will be beneficial.
2.1 Selecting the Decision Options
The investigated options in this research were three provinces located in the east and northeast of Iran, namely, Razavi Khorasan, Northern Khorasan, and Southern Khorasan. Figure 1 depicts the solar atlas of Iran and the location of the three aforementioned provinces.
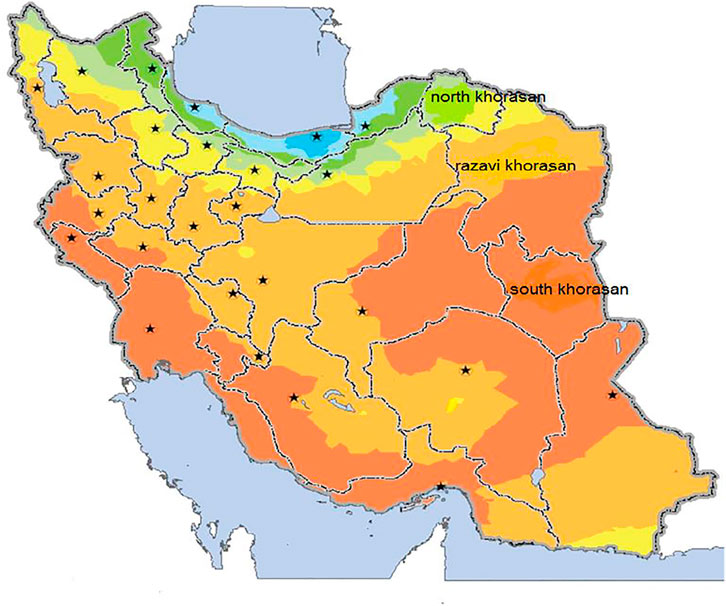
FIGURE 1. Solar atlas of Iran and the locations of the provinces of Razavi Khorasan, Northern Khorasan, and Southern Khorasan .
2.2 Criterion Selection
The primary stage of an MCDM method includes the selection of effective criteria (Kereush et al., 2017; Liu et al., 2017). In this article, the effective criteria in prioritizing the cities of the aforementioned provinces have been chosen according to the criteria governing solar pumping plants and various case studies.
The effective criteria in prioritizing the sites were chosen according to the effective criteria for establishing a solar-powered pumping station. Some data for these criteria, including solar irradiation, obtainable solar energy capacity, distance from roads, slope, distance from the substation, distance from the grid, end-user type, and accurate coordination of the site, were obtained from the SATBA website. The aforementioned data corresponded to the timeline from March 2020 to March 2021 (solar year 1399). The rest of the data were collected from weather sites and from articles and conferences.
The outcome of this research was based on data gathered from maps and atlases on energy efficiency, energy supply and demand, utilizing Google Map software. Then, all of the aforementioned data were combined, examined, and prioritized according to the electricity grid of Iran, related field data, and provincial water and wastewater corporation data, creating an integrated map. Figure 2 shows the selected criteria for assessing the potential for establishing a solar pumping plant in various regions and Table 3 indicates the positive and negative criteria.
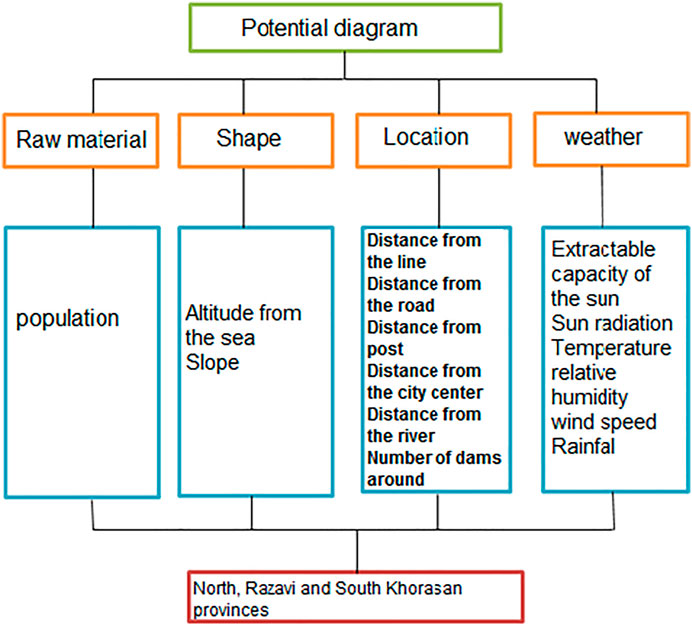
FIGURE 2. Selected criteria to assess the potential for establishing a solar pumping plant in various regions.
2.3 Investigating the Criteria
Precipitation: Rainfall has advert effects on PV panels, such as technical issues or a reduction in the effective area of panels caused by dirt. Additionally, high humidity affects the absorption or reflection of solar radiation, reducing the incident solar irradiation. Consequently, high precipitation impedes the operation of the solar panels and is considered a negative criterion (Muhsen et al., 2017b).
Altitude: Installing solar panels in regions with higher elevation increases the absorption of the solar panels due to the leaner atmosphere. In addition, the lower temperature in higher regions has a positive impact on the performance of PV panels. It must be taken into consideration that installing solar cells in highly elevated locations increases the costs and might be considered as a negative factor (Ngan and Tan, 2012).
Slope: The slope of the ground has a major impact on the installation site of solar cells. Slope is directly relative to the cost of the solar plant; therefore, higher slopes translate into greater costs in the construction and structure of solar panels. The optimal slope for installing solar panels from an economic point of view is 0–3. Solar plants installed on ground with a higher inclination are more susceptible to earthquakes as well (Horner and Clark, 2013).
Average annual temperature: Considering the effect of temperature on the process of choosing the proper location for the installation of solar panels will lead to a more cost-effective system with higher performance. This is due to the inverse relation between the temperature and the performance of solar panels. Furthermore, extremely high or low temperatures have adverse effects on the performance of a solar plant (Suresh et al., 2018).
Distance from the road: Access to roads facilitates the transportation of human resources and equipment. Therefore, distance from roads plays a major role in establishing power plants.
Distance from the grid line: Electricity transmission is one of the main elements of any power generation system. As the distance from the grid increases, so do the electrical resistance and the cost of transmission. Therefore, the ultimate location for a power plant is within the vicinity of the grid.
Distance from the substation: A substation is a place where the transmission equipment are installed and where voltage conversion occurs. Farther distance from a substation results in more wasted energy, reduced efficiency, and increased costs. In addition, the electromagnetic radiation emitted from substations can threaten the health of those who live nearby.
Relative humidity: High humidity leads to the oxidization of equipment and reduces the life span of the system (Suresh et al., 2018). Vapor and CO2 are the most important absorbers of solar radiation in the atmosphere. Regions with high humidity are not appropriate for installing a solar power plant.
Population: Highly populated areas have greater service and energy requirements and, thus, have a higher priority (Padhy et al., 2021).
Distance from the city center: Construction of a power plant in the vicinity of residential areas has a negative impact on population growth, so it is better to construct them a safe distance from the city center. Additionally, to increase investments, more agricultural lands are required. Therefore, distance from the city center is an important criterion for the inhabitants of that region.
Distance from the river: In order to protect the ecological environment of rivers, lakes, and other water resources, it is necessary to construct the power plant away from these areas. Contrary to other power plants, PV plants have the least amount of pollutants, and according to Bolin’s model, the minimum distance of a PV plant from the river or lake should be 200 m. This distance will alleviate any damage to the power plant in the event of flooding.
Wind speed: The speed of wind can obscure the solar panels with small particles or clear the surface of solar panels. Wind might cause damage to the solar plant.
Number of dams in the vicinity: Dams are usually established where the river’s water is gathered. Dams have negative impacts on the environment and can disrupt agricultural activities. Additionally, following the required distance considerations leads to the safety of the plant in the event of flooding or an earthquake.
2.4 Decision-Making Matrix
The primary stage in any MCDM method is the formation of a decision-making matrix. This matrix is created to assess a number of options considering a number of criteria, and each option is awarded a point according to a number of criteria. The decision-making matrix of this study is a 15 × 93 consisting of 15 criteria and 93 options. The 93 options are the sites located in Razavi Khorasan, Northern Khorasan, and Southern Khorasan, chosen by SATBA engineers. Table 4 indicates the decision-making matrix for this research.
The outcome of this research was based on data gathered from maps and atlases on energy efficiency, energy supply and demand, utilizing Google Map software. Then, all of the aforementioned data were combined, examined, and prioritized according to the electricity grid of Iran, related field data, and provincial water and wastewater corporation data, creating an integrated map.
3 Methodology
Access to computational device and decision-making systems in recent years has paved the way for more accurate decision-making and analysis of several quantitative and qualitative parameters and their effects on each other. An MCDM method has been utilized in this study, which is explained in this section.
3.1 Weighting of Criteria
Knowing the relative weights of the criteria in MCDM problems is an important step forward and is a requirement. After creating the non-dimensional criteria, it is necessary to determine the relative importance of these criteria. There are various methods for weighting the criteria, such as linear programming technique for multidimensional analysis of preferences (LINMAP), least squares, the eigenvector method, and Shannon entropy. The Shannon entropy method was utilized in this research.
3.1.1 Shannon Entropy Method for Weighting the Criteria
The Shannon entropy method is a decision-making method for calculating the weights of the criteria. This method requires a criteria–option matrix, which was introduced by Shannon in 1974. This matrix is indicative of the amount of uncertainty in a continuous probability distribution. The main idea of this method is that, if the dispersion of a criterion is high, that criterion has a high degree of importance. The steps of this method include calculating the Pij, Ej, K, and dj and, finally, wj using relations 1–5 (Yunna and Geng, 2014).
Step 1: Calculating Pij
Step 2: Calculating the entropy Ej
Step 3: Calculating the uncertainty dj
Step 4: Calculating the weight wj
3.2 Decision-Making Models
To prioritize the locations in the three provinces via the 15 aforementioned criteria, the TOPSIS MCDM method was used in the provided research.
3.2.1 TOPSIS
TOPSIS is one of the MCDM methods that aim to rank choices. Decision-making with multiple criteria tries to decide the best option in the presence of various and sometimes opposing criteria (San Cristóbal, 2011). The solution to this method would mean the design or selection of the best option among other options (Yang et al., 2018). In this method, the concepts of “ideal solution” and “semi-ideal solution” are used. The ideal solution is the best answer from all aspects, and such a solution does not exist in reality; the goal is to get as close to it as possible. In order to measure the similarity of an idea or option to the ideal solution and the anti-ideal solution, the distances between that idea or option from the ideal and anti-ideal solutions are measured. Then, the options are ranked according to the ratio of the distance from the anti-ideal solution to the sum of the distances from the ideal and anti-ideal solutions (Ren and Sovacool, 2015). TOPSIS chooses the solution with the minimum distance from the best option and the maximum distance from the worst option as the optimum option, and therefore its mathematical basis is superior to that of other decision-making methods (Sadeghzadeh and Salehi, 2011). It is possible to perform the process of decision-making in the presence of positive, negative, and a combination of positive and negative criteria. In order to choose the best option, a vast number of criteria can be considered; other methods such as the analytic hierarchical process (AHP) and the analytic network process (ANP) have intrinsic limitations in this regard (Falqi et al., 2019). Another feature that makes TOPSIS superior to other methods is that it is a compensational method, which means that the weights of all of the criteria and options are considered in the decision-making process and nothing is neglected. This simple method has an acceptable response time, and it is a viable candidate for solving the problem of a vast number of criteria and options (Şengül et al., 2015).
Step 1: Forming the decision-making matrix and non-dimensionalizing it via the norm method
Step 2: Calculating the non-dimensionalized weighted matrix
Step 3: Calculating the positive and negative ideal solutions
Step 4: Calculating the distance of each option from the negative and positive ideals
Step 5: Calculating the ith relative distance
Step 6: Choosing the best option
Figure 3 displays the TOPSIS flowchart. In this figure, all the stages of the TOPSIS decision-making have been illustrated. As can be seen, this method has six steps and is short and quick.
4 Results
Figure 4 demonstrates the weighted criterion according to the Shannon entropy method. Information theorem introduces entropy as an uncertainty criterion that is expressed with a particular probability distribution. The entropy method can be used to assess the criterion weights.
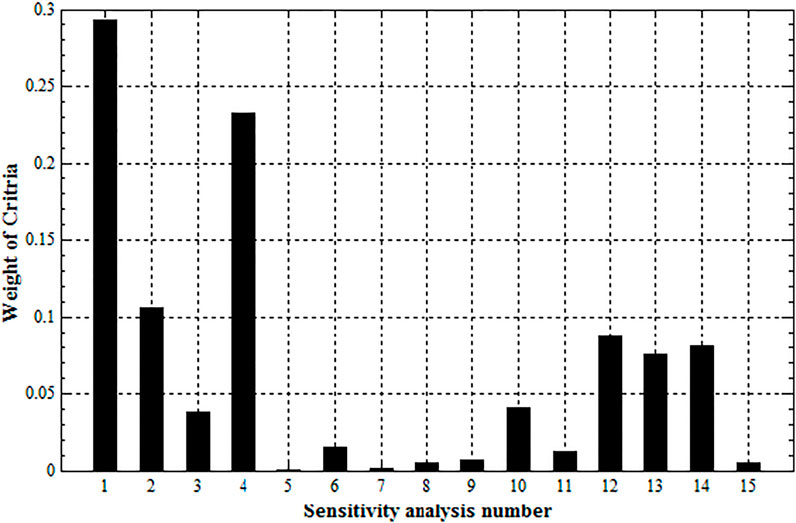
FIGURE 4. Criteria weights. (1-Distance from grid line, 2-Distance from the substation, 3-Slope, 4-Distance from the road, 5-Solar irradiation, 6-Obtainable solar energy capacity, 7-Temperature, 8-Relative humidity, 9-Altitude, 10-Distance from the city center, 11-Rainfall, 12-Population, 13-Distance from the river, 14-Number of dams in the vicinity, 15-Wind speed).
The aforementioned method indicates that the higher the dispersion of a criterion, the more important is the said criterion. Solar irradiation is almost the same in the provinces under investigation; therefore, solar irradiation is among the criteria with low weights.
The results of this research indicated that the distance from the grid had the greatest weight (equal to 0.29) due to the high dispersion and the distance from the substation ranking second, with a weight of 0.23. Additionally, solar irradiation and relative humidity had the least weights, equal to 0 and 0.1, respectively.
The TOPSIS method can utilize both the quantitative and qualitative criteria simultaneously and prioritize the options as a quantitative outcome. Therefore, all of the criteria have been weighted and assessed using the TOPSIS method. Figure 5 depicts the TOPSIS prioritization results. It can be observed that the percentages of the cities varied from 43.95% to 93.87%. The Sarayan site is located in a perfect spot in terms of solar irradiation and can easily utilize this energy to power the solar plant. The other advantages of this site included the proper distance from the grid, the elevation from the sea level, and the ideal slope. Figure 6 shows a satellite image of Sarayan in Southern Khorasan. With an area of 230 ha, the latitude and longitude of this site are 33.826,739°N and 58.534,629°E, respectively. Most of the areas of this region are flat lands, with a slope of 2.3% and distance from the road of 150 m. Grid lines pass through this site, and the distance from the substation is 5 km. The solar radiation in this region is 1,800 kWh/kWp, and the obtainable solar capacity is estimated to be 115 MW. Figure 7 shows an enlarged view of the top 10 sites, which included Sarayan in Southern Khorasan, Isk in Southern Khorasan, Esfadan in Southern Khorasan, Dasht Byaz in Southern Khorasan, Beydokht 2 in Razavi Khorasan, Shahr Jangal in Razavi Khorasan, Hajabad in Razavi Khorasan, Erinshahr in Southern Khorasan, Sardab in Razavi Khorasan, and Garmab in Southern Khorasan, with values of 93.87%, 93.82%, 93.80%, 93.50%, 93.31%, 92.73%, 92.13%, 91.75%, 91.64%, and 91.55%, respectively. Figure 8 depicts an enlarged view of the bottom 10 sites, Sindar, Safiabad, Sarand, Momenabad, Hajiabad, Aliabadalo, Pedran, Seivjan, Ghasemkhan, and Shosef, with values of 75.73%, 73.33%, 71.18%, 70.47%, 65.68%, 63.66%, 63.04%, 63.01%, and 43.95%, respectively.
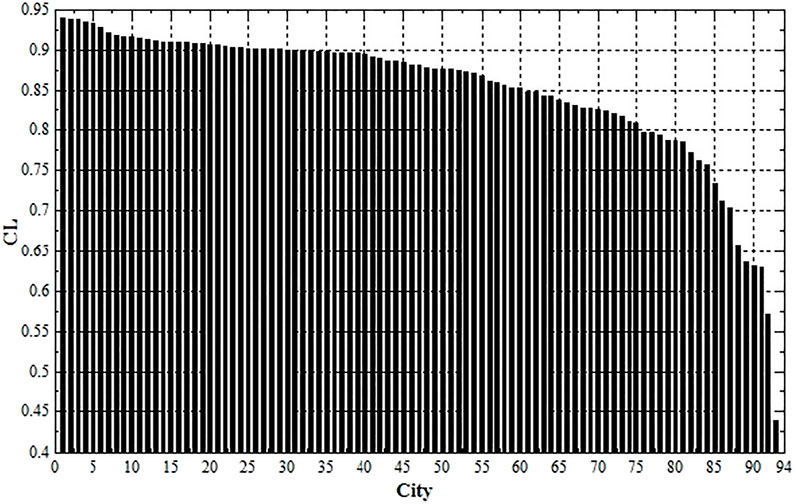
FIGURE 5. Assessment of priority options for establishing a solar plant via the TOPSIS (technique for order preference by similarity to ideal solution) method.
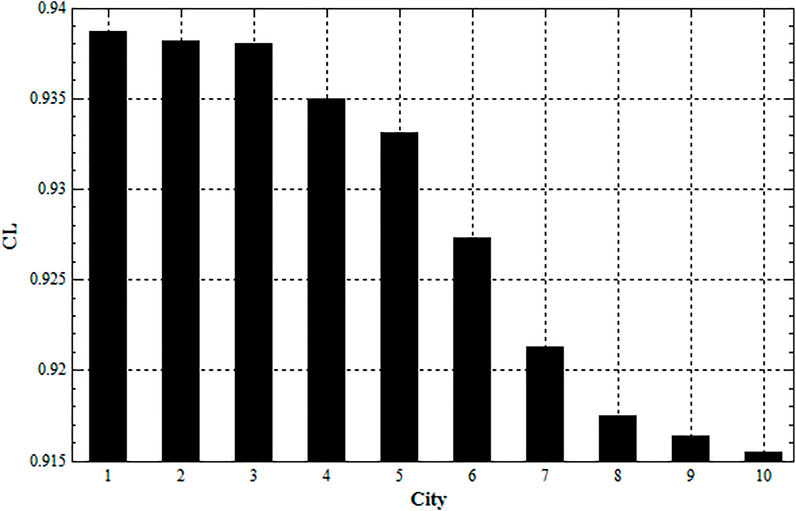
FIGURE 6. Top 10 sites for the establishment of a solar plant according to the TOPSIS (technique for order preference by similarity to ideal solution) method.
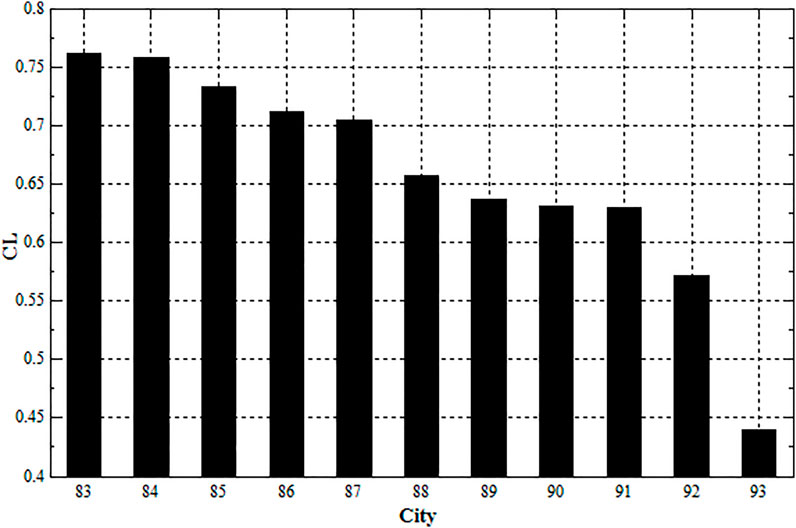
FIGURE 7. Bottom 10 sites for the establishment of a solar plant according to the TOPSIS (technique for order preference by similarity to ideal solution) method.
5 Sensitivity Analysis
Assumptions and known values of each technical or economic model are subject to change and error. Sensitivity analysis investigates these errors and changes and their effects on the model. Sensitivity analysis methods can be divided into three groups: statistical, mathematical, and graphical. In order to investigate the response of the various criteria on the outcome, sensitivity analysis was conducted in this article. Investigating the effect of criterion weight variations on the outcome of the model is of utmost importance. Henceforth, 30 mods of sensitivity analysis have been performed on the 15 criteria (Table 5). In the first mod, the weight of criterion 1 was increased by 10%, and then in the second mod, the first criterion was subjected to a 10% weight reduction. The same process was applied to criteria 2–15. Consequently, 30 mods for sensitivity analysis were produced for the 15 aforementioned criteria, and the effect of each one was investigated. The results of the sensitivity analysis indicated that, out of the 30 mods, Sarayan in Southern Khorasan came out on top in 28 of them, closely followed by Isk in the same province with a 0.05% margin. On the other hand, Shosef, located in Southern Khorasan, was the worst option among all 30 mods of sensitivity analysis.
Figure 9A demonstrates the results of the sensitivity analysis for the MCDM method TOPSIS. Figure 9B shows an enlarged view of the top 10 choices from the above calculations. Figure 9C showcases the top 5 sites in a larger view. Figure 9D is a demonstration of the top 3 sites from the sensitivity analysis. Consequently, according to the weather and geographical conditions, Sarayan, located in Southern Khorasan, is the best site for a solar plant, followed by Isk, Esfadan, Dasht Byaz, Beydokht 2, Shahr Jangal, Hajabad, Erinshahr, Sardab, and Garmab.
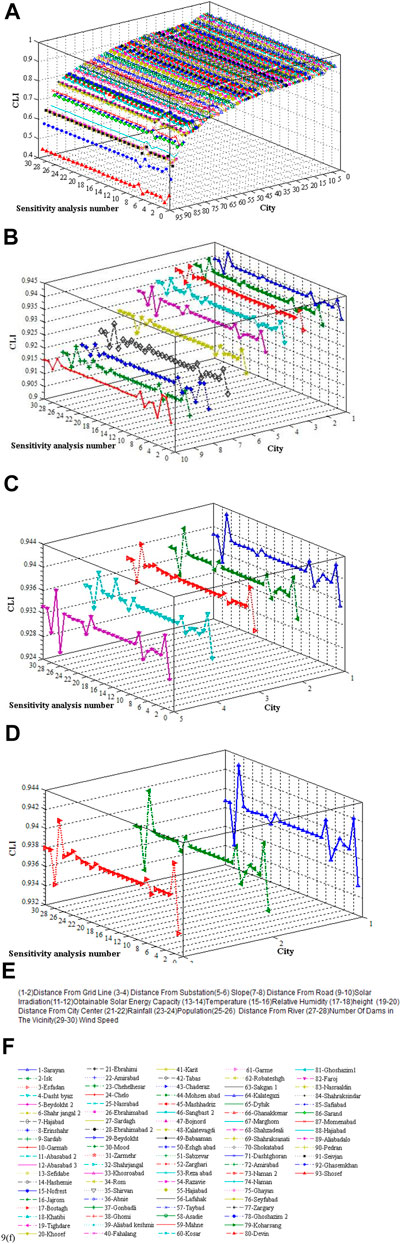
FIGURE 9. (A) Sensitivity analysis results via the TOPSIS (technique for order preference by similarity to ideal solution) multi-criteria decision-making method. (B) Top 10 results in an enlarged view. (C) Top 5 sites enlarged. (D) Top 3 results in a large view mode. (E) Determining the sensitivity analysis numbers. (F) Sites included in the sensitivity analysis.
6 Conclusion
An MCDM method via the TOPSIS mathematical model was used in a feasibility study of three eastern and northeastern provinces of Iran, namely, Northern Khorasan, Razavi Khorasan, and Southern Khorasan. The objective of this article was to find the best location for the establishment of a PV pumping plant. Therefore, a total of 93 sites, including 43 sites in Southern Khorasan, 33 sites in Razavi Khorasan, and 17 sites in Northern Khorasan, were chosen. Additionally, 15 criteria that had the highest impacts on the problem were selected, such as solar irradiation, precipitation, wind speed, relative humidity, slope, altitude from sea level, distance from the substation, distance from the grid line, obtainable solar energy capacity, distance from the river, population, distance from the city center, distance from the road, average temperature, and the number of dams in the vicinity. Using the Shannon entropy method, each of the criteria was weighted; consequently, the distance from the grid line had the highest weight equal to 0.29. Additionally, sites were prioritized using the TOPSIS method. The prioritization results showed various percentages ranging from the minimum of 43.95%, corresponding to Shosef, to the maximum of 93.87%, corresponding to Sarayan. Closely following Sarayan were Isk, Esfadan, and Dasht Byaz in Southern Khorasan and Beydokht 2, Shahr Jangal, and Hajabad in Razavi Khorasan. Shosef, Ghasemkhan, and Seivjan obtained the lowest points equal to 43.95, 57.11, and 63.01, respectively. In order to investigate the problem extensively, sensitivity analysis was implemented. To change the weights of the criteria, 30 sensitivity analysis mods were introduced for the 15 criteria. It was observed that, in 28 of the 30 mods, Sarayan was the first option, followed by Isk with a margin of 0.05%; Shosef in Southern Khorasan obtained the lowest score in all mods. The priority of the other sites varied depending on the various criteria; therefore, no accurate prioritization could be achieved.
This research provides a viable framework for future research works in other areas of the world. Utilizing novel and hybrid MCDM methods based on TOPSIS to provide greater accuracy in the selection of desirable sites and comparing the results of these methods would be a step forward in the right direction.
Data Availability Statement
The original contributions presented in the study are included in the article/supplementary material. Further inquiries can be directed to the corresponding author.
Author Contributions
FH curated the data, prepared the draft, and performed the methodology. AM contributed to the supervision, visualization, methodology, and investigation. AJ helped with supervision, investigation, and editing. SH contributed to the investigation and reviewed and edited the manuscript.
Conflict of Interest
The authors declare that the research was conducted in the absence of any commercial or financial relationships that could be construed as a potential conflict of interest.
Publisher’s Note
All claims expressed in this article are solely those of the authors and do not necessarily represent those of their affiliated organizations or those of the publisher, the editors, and the reviewers. Any product that may be evaluated in this article, or claim that may be made by its manufacturer, is not guaranteed or endorsed by the publisher.
References
Ali, S., Taweekun, J., Techato, K., Waewsak, J., and Gyawali, S. (2019). GIS Based Site Suitability Assessment for Wind and Solar Farms in Songkhla, Thailand. Renew. Energ. 132, 1360–1372. doi:10.1016/j.renene.2018.09.035
Ayodele, T. R., Ogunjuyigbe, A. S. O., Odigie, O., and Munda, J. L. (2018). A Multi-Criteria GIS Based Model for Wind Farm Site Selection Using Interval Type-2 Fuzzy Analytic Hierarchy Process: The Case Study of Nigeria. Appl. Energ. 228, 1853–1869. doi:10.1016/j.apenergy.2018.07.051
Babatunde, O. M., Munda, J. L., and Hamam, Y. (2019). Selection of a Hybrid Renewable Energy Systems for a Low-Income Household. Sustainability 11, 4282. doi:10.3390/su11164282
Büyüközkan, G., and Karabulut, Y. (2017). Energy Project Performance Evaluation with Sustainability Perspective. Energy 119, 549–560. doi:10.1016/j.energy.2016.12.087
Chandel, S. S., Nagaraju Naik, M., and Chandel, R. (2015). Review of Solar Photovoltaic Water Pumping System Technology for Irrigation and Community Drinking Water Supplies. Renew. Sust. Energ. Rev. 49, 1084–1099. doi:10.1016/j.rser.2015.04.083
Das, H. S., Tan, C. W., Yatim, A. H. M., and Lau, K. Y. (2017). Feasibility Analysis of Hybrid Photovoltaic/battery/fuel Cell Energy System for an Indigenous Residence in East Malaysia. Renew. Sust. Energ. Rev. 76, 1332–1347. doi:10.1016/j.rser.2017.01.174
Demirel, T., Öner, S. C., Tüzün, S., Deveci, M., Öner, M., and Demirel, N. Ç. (2018). Choquet Integral-Based Hesitant Fuzzy Decision-Making to Prevent Soil Erosion. Geoderma 313, 276–289. doi:10.1016/j.geoderma.2017.10.054
Deveci, M., Akyurt, I. Z., and Yavuz, S. (2018). A GIS-Based Interval Type-2 Fuzzy Set for Public Bread Factory Site Selection. J. Enterprise Inf. Manage. 31, 820–847. doi:10.1108/jeim-02-2018-0029
Deveci, M., Pekaslan, D., and Canıtez, F. (2020). The Assessment of Smart City Projects Using zSlice Type-2 Fuzzy Sets Based Interval Agreement Method. Sust. cities Soc. 53, 101889. doi:10.1016/j.scs.2019.101889
Deveci, M., Simic, V., and Torkayesh, A. E. (2021). Remanufacturing Facility Location for Automotive Lithium-Ion Batteries: an Integrated Neutrosophic Decision-Making Model. J. Clean. Prod. 317, 128438. doi:10.1016/j.jclepro.2021.128438
Evans, A., Strezov, V., and Evans, T. J. (2012). Assessment of Utility Energy Storage Options for Increased Renewable Energy Penetration. Renew. Sust. Energ. Rev. 16 (6), 4141–4147. doi:10.1016/j.rser.2012.03.048
Falqi, I. I., Ahmed, M., and Mallick, J. (2019). Siliceous Concrete Materials Management for Sustainability Using Fuzzy-TOPSIS Approach. Appl. Sci. 9, 3457. doi:10.3390/app9173457
Fetanat, A., and Khorasaninejad, E. (2015). A Novel Hybrid MCDM Approach for Offshore Wind Farm Site Selection: A Case Study of Iran. Ocean Coastal Manage. 109, 17–28. doi:10.1016/j.ocecoaman.2015.02.005
Guezgouz, M., Jurasz, J., Bekkouche, B., Ma, T., Javed, M. S., and Kies, A. (2019). Optimal Hybrid Pumped Hydro-Battery Storage Scheme for Off-Grid Renewable Energy Systems. Energ. Convers. Manage. 199, 112046. doi:10.1016/j.enconman.2019.112046
Guezgouz, M., Jurasz, J., and Bekkouche, B. (2019). Techno-Economic and Environmental Analysis of a Hybrid PV-WT-PSH/BB Standalone System Supplying Various Loads. Energies 12 (3), 514. Available at: https://www.mdpi.com/1996-1073/12/3/514. doi:10.3390/en12030514
Horner, R. M., and Clark, C. E. (2013). Characterizing Variability and Reducing Uncertainty in Estimates of Solar Land Use Energy Intensity. Renew. Sust. Energ. Rev. 23, 129–137. doi:10.1016/j.rser.2013.01.014
Ibrahim, H., Ilinca, A., and Perron, J. (2008). Energy Storage Systems-Characteristics and Comparisons. Renew. Sust. Energ. Rev. 12 (5), 1221–1250. doi:10.1016/j.rser.2007.01.023
Jamshidi, M., and Askarzadeh, A. (2019). Techno-economic Analysis and Size Optimization of an Off-Grid Hybrid Photovoltaic, Fuel Cell and Diesel Generator System. Sust. Cities Soc. 44, 310–320. doi:10.1016/j.scs.2018.10.021
Kereush, D., Perovych, I., and Perovych, I. (2017). Determining Criteria for Optimal Site Selection for Solar Power Plants. Gll 4, 39–54. doi:10.15576/GLL/2017.4.39
Krishankumar, R., Pamucar, D., Deveci, M., and Ravichandran, K. S. (2021). Prioritization of Zero-Carbon Measures for Sustainable Urban Mobility Using Integrated Double Hierarchy Decision Framework and EDAS Approach. Sci. Total Environ. 797, 149068. doi:10.1016/j.scitotenv.2021.149068
Lee, H.-C., and Chang, C.-T. (2018). Comparative Analysis of MCDM Methods for Ranking Renewable Energy Sources in Taiwan. Renew. Sust. Energ. Rev. 92, 883–896. doi:10.1016/j.rser.2018.05.007
Liu, J., Xu, F., and Lin, S. (2017). Site Selection of Photovoltaic Power Plants in a Value Chain Based on Grey Cumulative prospect Theory for Sustainability: A Case Study in Northwest China. J. Clean. Prod. 148, 386–397. doi:10.1016/j.jclepro.2017.02.012
Ma, T., Yang, H., and Lu, L. (2014). A Feasibility Study of a Stand-Alone Hybrid Solar-Wind-Battery System for a Remote Island. Appl. Energ. 121, 149–158. doi:10.1016/j.apenergy.2014.01.090
Machiwal, D., Jha, M. K., and Mal, B. C. (2011). Assessment of Groundwater Potential in a Semi-arid Region of India Using Remote Sensing, GIS and MCDM Techniques. Water Resour. Manage. 25 (5), 1359–1386. doi:10.1007/s11269-010-9749-y
Maleki, A., Pourfayaz, F., Hafeznia, H., and Rosen, M. A. (2017). A Novel Framework for Optimal Photovoltaic Size and Location in Remote Areas Using a Hybrid Method: A Case Study of Eastern Iran. Energ. Convers. Manage. 153, 129–143. doi:10.1016/j.enconman.2017.09.061
Maleki, A., Pourfayaz, F., and Rosen, M. A. (2016). A Novel Framework for Optimal Design of Hybrid Renewable Energy-Based Autonomous Energy Systems: A Case Study for Namin, Iran. Energy 98, 168–180. doi:10.1016/j.energy.2015.12.133
Modu, B., Aliyu, A., Bukar, A. L., Abdulkadir, M., Gwoma, Z., and Mustapha, M. (2018). Techno-Economic Analysis of Off-Grid Hybrid PV-Diesel-Battery System in Katsina State, Nigeria. Arid Zone J. Eng. Tech. Environ. 14, 317–324.
Moradi, S., Yousefi, H., Noorollahi, Y., and Rosso, D. (2020). Multi-criteria Decision Support System for Wind Farm Site Selection and Sensitivity Analysis: Case Study of Alborz Province, Iran. Energ. Strategy Rev. 29, 100478. doi:10.1016/j.esr.2020.100478
Muhsen, D. H., Khatib, T., and Haider, H. T. (2017). A Feasibility and Load Sensitivity Analysis of Photovoltaic Water Pumping System with Battery and Diesel Generator. Energ. Convers. Manage. 148, 287–304. doi:10.1016/j.enconman.2017.06.007
Muhsen, D. H., Khatib, T., and Nagi, F. (2017). A Review of Photovoltaic Water Pumping System Designing Methods, Control Strategies and Field Performance. Renew. Sust. Energ. Rev. 68, 70–86. doi:10.1016/j.rser.2016.09.129
Nazari, M. A., Aslani, A., and Ghasempour, R. (2018). Analysis of Solar Farm Site Selection Based on TOPSIS Approach. Int. J. Soc. Ecol. Sust. Dev. 9, 12–25. doi:10.4018/IJSESD.2018010102
Neves, D., Baptista, P., Simões, M., Silva, C. A., and Figueira, J. R. (2018). Designing a Municipal Sustainable Energy Strategy Using Multi-Criteria Decision Analysis. J. Clean. Prod. 176, 251–260. doi:10.1016/j.jclepro.2017.12.114
Ngan, M. S., and Tan, C. W. (2012). Assessment of Economic Viability for PV/wind/diesel Hybrid Energy System in Southern Peninsular Malaysia. Renew. Sust. Energ. Rev. 16 (1), 634–647. doi:10.1016/j.rser.2011.08.028
Padhy, A., Vishal, B., Verma, P., Dwivedi, G., and Behura, A. K. (2021). Fabrication of Parabolic Trough Hybrid Solar PV-T Collector Using A-Si Thin Film Solar Cells in Indian Perspective. Mater. Today Proc. 38, 56–62. doi:10.1016/j.matpr.2020.05.652
Pamucar, D., Deveci, M., Canıtez, F., and Lukovac, V. (2020). Selecting an Airport Ground Access Mode Using Novel Fuzzy LBWA-WASPAS-H Decision Making Model. Eng. Appl. Artif. Intelligence 93, 103703. doi:10.1016/j.engappai.2020.103703
Peng, W., Maleki, A., Rosen, M. A., and Azarikhah, P. (2018). Optimization of a Hybrid System for Solar-Wind-Based Water Desalination by Reverse Osmosis: Comparison of Approaches. Desalination 442, 16–31. doi:10.1016/j.desal.2018.03.021
Peng, X., Liu, Z., and Jiang, D. (2021). A Review of Multiphase Energy Conversion in Wind Power Generation. Renew. Sust. Energ. Rev. 147, 111172. doi:10.1016/j.rser.2021.111172
Reca, J., Torrente, C., López-Luque, R., and Martínez, J. (2016). Feasibility Analysis of a Standalone Direct Pumping Photovoltaic System for Irrigation in Mediterranean Greenhouses. Renew. Energ. 85, 1143–1154. doi:10.1016/j.renene.2015.07.056
Ren, J., and Sovacool, B. K. (2015). Prioritizing Low-Carbon Energy Sources to Enhance China's Energy Security. Energ. Convers. Manage. 92, 129–136. doi:10.1016/j.enconman.2014.12.044
Sadeghzadeh, K., and Salehi, M. B. (2011). Mathematical Analysis of Fuel Cell Strategic Technologies Development Solutions in the Automotive Industry by the TOPSIS Multi-Criteria Decision Making Method. Int. J. Hydrogen Energ. 36 (20), 13272–13280. doi:10.1016/j.ijhydene.2010.07.064
San Cristóbal, J. R. (2011). Multi-criteria Decision-Making in the Selection of a Renewable Energy Project in spain: The Vikor Method. Renew. Energ. 36 (2), 498–502. doi:10.1016/j.renene.2010.07.031
Sánchez-Lozano, J. M., Teruel-Solano, J., Soto-Elvira, P. L., and Socorro García-Cascales, M. (2013). Geographical Information Systems (GIS) and Multi-Criteria Decision Making (MCDM) Methods for the Evaluation of Solar Farms Locations: Case Study in South-Eastern Spain. Renew. Sust. Energ. Rev. 24, 544–556. doi:10.1016/j.rser.2013.03.019
SATBA (2021). Renewable Energy and Energy Efficiency Organization (SATBA). Available at: http://www.satba.gov.ir/en/home (Accessed May, 2021).
Şengül, Ü., Eren, M., Eslamian Shiraz, S., Gezder, V., and Şengül, A. B. (2015). Fuzzy TOPSIS Method for Ranking Renewable Energy Supply Systems in Turkey. Renew. Energ. 75, 617–625. doi:10.1016/j.renene.2014.10.045
Shahraki Shahdabadi, R., Maleki, A., Haghighat, S., and Ghalandari, M. (2021). Using Multi-Criteria Decision-Making Methods to Select the Best Location for the Construction of a Biomass Power Plant in Iran. J. Therm. Anal. Calorim. 145 (4), 2105–2122. doi:10.1007/s10973-020-10281-1
Shezan, S. A., Julai, S., Kibria, M. A., Ullah, K. R., Saidur, R., Chong, W. T., et al. (2016). Performance Analysis of an Off-Grid Wind-PV (Photovoltaic)-diesel-battery Hybrid Energy System Feasible for Remote Areas. J. Clean. Prod. 125, 121–132. doi:10.1016/j.jclepro.2016.03.014
Suresh, A. K., Khurana, S., Nandan, G., Dwivedi, G., and Kumar, S. (2018). Role on Nanofluids in Cooling Solar Photovoltaic Cell to Enhance Overall Efficiency. Mater. Today Proc. 5 (9), 20614–20620. doi:10.1016/j.matpr.2018.06.442
Tong, X., Zhang, F., Ji, B., Sheng, M., and Tang, Y. (2016). Carbon-Coated Porous Aluminum Foil Anode for High-Rate, Long-Term Cycling Stability, and High Energy Density Dual-Ion Batteries. Adv. Mater. 28 (45), 9979–9985. doi:10.1002/adma.201603735
Wang, X., and Lyu, X. (2021). Experimental Study on Vertical Water Entry of Twin Spheres Side-By-Side. Ocean Eng. 221, 108508. doi:10.1016/j.oceaneng.2020.108508
Watson, J. J. W., and Hudson, M. D. (2015). Regional Scale Wind Farm and Solar Farm Suitability Assessment Using GIS-Assisted Multi-Criteria Evaluation. Landscape Urban Plann. 138, 20–31. doi:10.1016/j.landurbplan.2015.02.001
Yang, Y., Ren, J., Solgaard, H. S., Xu, D., and Nguyen, T. T. (2018). Using Multi‐criteria Analysis to Prioritize Renewable Energy home Heating Technologies. Sustainable Energ. Tech. Assessments 29, 36–43. doi:10.1016/j.seta.2018.06.005
Yu, Y., Liu, J., Wang, H., and Liu, M. (2011). Assess the Potential of Solar Irrigation Systems for Sustaining Pasture Lands in Arid Regions - A Case Study in Northwestern China. Appl. Energ. 88 (9), 3176–3182. doi:10.1016/j.apenergy.2011.02.028
Yücenur, G. N., Çaylak, Ş., Gönül, G., and Postalcıoğlu, M. (2020). An Integrated Solution with SWARA&COPRAS Methods in Renewable Energy Production: City Selection for Biogas Facility. Renew. Energ. 145, 2587–2597. doi:10.1016/j.renene.2019.08.011
Yunna, W., and Geng, S. (2014). Multi-criteria Decision Making on Selection of Solar-Wind Hybrid Power Station Location: A Case of China. Energ. Convers. Manage. 81, 527–533. doi:10.1016/j.enconman.2014.02.056
Zhang, G., Wu, B., Maleki, A., and Zhang, W. (2018). Simulated Annealing-Chaotic Search Algorithm Based Optimization of Reverse Osmosis Hybrid Desalination System Driven by Wind and Solar Energies. Solar Energy 173, 964–975. doi:10.1016/j.solener.2018.07.094
Zhang, K., Ali, A., Antonarakis, A., Moghaddam, M., Saatchi, S., Tabatabaeenejad, A., et al. (2019). The Sensitivity of North American Terrestrial Carbon Fluxes to Spatial and Temporal Variation in Soil Moisture: An Analysis Using Radar‐Derived Estimates of Root‐Zone Soil Moisture. J. Geophys. Res. Biogeosci. 124 (11), 3208–3231. doi:10.1029/2018JG004589
Zhang, K., Wang, Q., Chao, L., Ye, J., Li, Z., Yu, Z., et al. (2019). Ground Observation-Based Analysis of Soil Moisture Spatiotemporal Variability across a Humid to Semi-humid Transitional Zone in China. J. Hydrol. 574, 903–914. doi:10.1016/j.jhydrol.2019.04.087
Zhang, L., Wang, X., Zhang, Z., Cui, Y., Ling, L., and Cai, G. (2021). An Adaptative Control Strategy for Interfacing Converter of Hybrid Microgrid Based on Improved Virtual Synchronous Generator. IET Renew. Power Generation, 1–13. doi:10.1049/rpg2.12293
Zhang, W., Maleki, A., and Rosen, M. A. (2019). A Heuristic-Based Approach for Optimizing a Small Independent Solar and Wind Hybrid Power Scheme Incorporating Load Forecasting. J. Clean. Prod. 241, 117920. doi:10.1016/j.jclepro.2019.117920
Zhangab, W., Maleki, A., Rosen, M. A., and Liu, J. (2019). Sizing a Stand-Alone Solar-Wind-Hydrogen Energy System Using Weather Forecasting and a Hybrid Search Optimization Algorithm. Energy Convers. Manag. 180, 609–621. doi:10.1016/j.enconman.2018.08.102
Keywords: obtainable solar energy capacity, solar pumping system, multi-criteria decision-making method, feasibility study, TOPSIS
Citation: Heydari F, Maleki A, Jabari Moghadam A and Haghighat S (2021) Emplacement of the Photovoltaic Water Pumping System in Remote Areas by a Multi-Criteria Decision-Making Method: A Case Study. Front. Energy Res. 9:770981. doi: 10.3389/fenrg.2021.770981
Received: 05 September 2021; Accepted: 04 November 2021;
Published: 13 December 2021.
Edited by:
Enhua Wang, Beijing Institute of Technology, ChinaReviewed by:
Muhammet Deveci, National Defense University, TurkeyChristian Wagner, Georgetown University, United States
Copyright © 2021 Heydari, Maleki, Jabari Moghadam and Haghighat. This is an open-access article distributed under the terms of the Creative Commons Attribution License (CC BY). The use, distribution or reproduction in other forums is permitted, provided the original author(s) and the copyright owner(s) are credited and that the original publication in this journal is cited, in accordance with accepted academic practice. No use, distribution or reproduction is permitted which does not comply with these terms.
*Correspondence: Akbar Maleki, YWtiYXIubWFsZWtpMjBAeWFob28uY29t