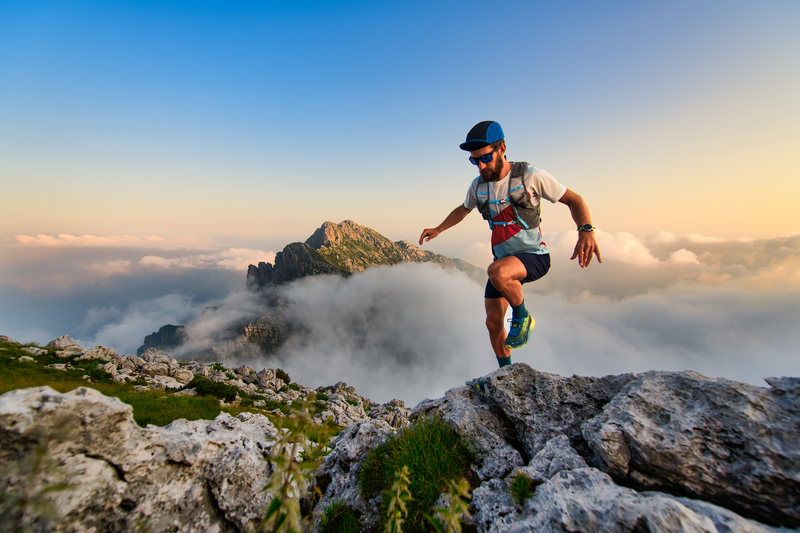
95% of researchers rate our articles as excellent or good
Learn more about the work of our research integrity team to safeguard the quality of each article we publish.
Find out more
REVIEW article
Front. Energy Res. , 22 November 2021
Sec. Wind Energy
Volume 9 - 2021 | https://doi.org/10.3389/fenrg.2021.769920
This article is part of the Research Topic Offshore Wind Energy: Modeling and Measurements View all 7 articles
With economic development and population growth, energy demand has shown an upward trend. Renewable energy is inexhaustible and causes little pollution, which has broad prospects for development. In recent years, wind energy has been developed as an essential renewable energy source. The use of wind power is very environmentally friendly and plays a critical role in economic growth. Assessing the characteristics and potential of wind energy is the first step in the effective development of wind energy. The wind speed distribution at a specific location determines the available wind energy. This paper reviews the wind speed distribution models used for wind energy assessment, and they are applicable to different wind regimes. All potential wind speed distribution models should be considered for modeling wind speed data at a particular site. Previous studies have selected several parameter estimation methods and evaluation criteria to estimate model parameters and evaluate the goodness-of-fit. This paper discusses their advantages and disadvantages. The characteristics of wind speed distribution are constantly varying geographically and temporally. Wind energy assessment should consider local geographical elements, such as local climate, topography, and thermal properties difference between the land and the sea, and focus on long-term variations in wind characteristics.
Energy is a necessary material basis for the survival and development of human society. In recent years, the energy crisis has become a worldwide problem faced by humankind. With the continuous growth of the population and the rapid development of the global economy, energy demand is also increasing. Traditional fossil fuels (such as coal, oil, natural gas, etc.) have been widely used in almost all areas of daily life. However, fossil fuel reserves are limited. Fossil fuels gradually formed in nature over millions of years may be entirely exhausted by humans within a few hundred years (Baz et al., 2021). In addition, the carbon in fossil fuels is transformed into carbon dioxide, which increases the concentration of carbon dioxide in the atmosphere, leading to aggravation of the greenhouse effect, changing the global climate, and breaking the ecological balance (Arenas-López and Badaoui, 2020). As people gradually realize the importance and urgency of environmental protection, energy conservation, emission reduction, and sustainable development, renewable energy has become the primary energy source required for future social development and has broad potential demands. Wind energy is one of the potential renewable energy sources that can be used for commercial purposes. Many countries take wind power development to improve the energy structure and protect the ecological environment. Wind energy also plays a vital role in economic growth, creating more employment opportunities, and promoting the development of science and technology (Liu et al., 2019; Kandpal and Dhingra, 2021). Wind energy has recently attracted widespread attention in the power generation industry (Jansen et al., 2020). Global wind-generated power has increased significantly from 2012 to 2017 (Figure 1) (World Bank, 2017).
FIGURE 1. Changes in wind energy output from 2012 to 2017 in the four countries with the highest wind power generation (China, the United States, Germany, and India).
At present, to meet the increasing electricity demand, researchers have focused on improving the efficiency of wind power generation (Chen and Blaabjerg, 2009). Assessing the characteristics and potential of wind energy is the first step in the effective development of wind energy. Wind energy resource assessment is an essential part of the feasibility analysis of wind farm projects. Whether the assessment is reasonable directly impacts the cost of power generation and economic benefits (Mauritzen, 2020). The wind speed distribution at a specific location determines the available wind energy and the performance of the energy conversion system. Therefore, to reduce the uncertainty of wind energy output estimation, it is necessary to accurately understand the distribution characteristics of wind speed (Celik, 2003). Two methods are generally used to determine the wind speed distribution: 1) the time series method (Morales et al., 2010; Katikas et al., 2021) and 2) the statistical analysis method (Ouarda et al., 2016; Elie Bertrand et al., 2020). The result obtained by the time series method may be more accurate because it is based on the original wind speed data. However, with an enormous amount of data, the processing process of the time series method is more complicated. When there is a lack of long-term wind speed data, it is more feasible to use statistical analysis to explain the behavior and characteristics of historical wind speed data and estimate the wind energy output. The statistical analysis method uses limited parameters to characterize wind speed distribution, which is efficient and straightforward. The process of statistical analysis to assess the potential of wind energy is shown in Figure 2. It is crucial to determine the most suitable probability density function (pdf) for historical wind speed data (Alavi et al., 2016a). The most widely used pdf in wind speed modeling is the Weibull distribution (Petković et al., 2014; Wais, 2017a; Sarkar et al., 2019). In recent years, researchers have considered several pdfs to find the most accurate model for the wind energy assessment of a particular site (Lo Brano et al., 2011; Ouarda et al., 2015; Jia et al., 2020; Wang et al., 2021). After determining the pdf, the value of each parameter in the function should be estimated. The commonly used parameter estimation methods mainly include the maximum likelihood method (Miao et al., 2019), the least squares method (Jung and Schindler, 2017), the moment method (Li and Miao, 2021), and the power density method (Akdağ and Dinler, 2009). Previous studies used different goodness-of-fit criteria to evaluate the accuracy of the model. Commonly used criteria include the coefficient of determination (ul Haq et al., 2020), the root mean square error (Guarienti et al., 2020), the Kolmogorov-Smirnov test statistic (Ouarda and Charron, 2018), the Anderson-Darling test statistic (Soukissian, 2013), and the chi-square test statistic (Wang et al., 2016).
Although the research of wind speed distribution has pursued the unified modeling of existing wind regimes, previous studies have not proposed a model that can provide a sufficient description at any site. Correspondingly, different parameter estimation methods and goodness-of-fit criteria have advantages and disadvantages, which bring difficulties to wind energy potential assessment. This paper reviews wind speed models, parameter estimation methods, and goodness-of-fit criteria and briefly discusses the advantages and disadvantages. The aim is to analyze the reasons for the differences in the fitting effects of models in different geographical locations and time scales.
The kinetic energy contained in the airflow is converted into electrical energy by the wind turbines. The wind speed presents a positively skewed distribution with statistical characteristics. However, wind power has randomness, volatility, and intermittent characteristics, making the output power of wind farms fluctuate considerably. Several probability distribution models have been widely used in wind farm analysis, planning, design, construction, and operation. Wind speed distribution models can be roughly divided into two classes: parametric distribution models and nonparametric distribution models.
The theoretical average output can be calculated as follows (Masseran, 2015):
Where
The observed average output can be calculated as follows:
Where
Energy prevision bias is the percentage error between the theoretical average output and the observed average output:
The estimation of wind power mainly depends on the
The Weibull distribution was promoted by the Swedish physicist Weibull (Weibull, 1951), and it has been used in various fields, such as physics, materials science, geography, medicine, economics, etc. The pdf of the two-parameter Weibull distribution is:
where
A three-parameter Weibull distribution is proposed for wind energy evaluation as an alternative probability distribution for the two-parameter Weibull distribution (Montoya et al., 2019):
where
When the shape parameter of the two-parameter Weibull distribution is equal to 2, the Rayleigh distribution is formed:
Rayleigh distribution is used to model wind speed and evaluate the standard performance of wind turbines (Saleh et al., 2012; Valencia Ochoa et al., 2019). Compared with the Weibull distribution, because the Rayleigh distribution contains only one parameter, it is more convenient to use, and its parameters are easier to estimate. However, the Rayleigh distribution is based on the assumption that the long-term mean wind vector is zero. The vector of wind prevailing at sea, such as trade wind, deviates significantly from zero, making the applicability of the Rayleigh distribution to sea winds relatively limited (Perrin et al., 2006).
Previous studies have shown that extreme wind speeds have almost no effect on the parameter values of the Weibull distribution (Wang et al., 2015). Once the wind speed exceeds the threshold, the Weibull model is no longer applicable. There are obvious errors in the maximum annual wind speed distribution and the return period estimated by the Weibull model, which affects the risk assessment of extreme winds (Perrin et al., 2006). A practical alternative is to use extreme value distributions.
Extreme value distributions used for wind speed modeling include the Gumbel distribution, the inverse Weibull distribution, and the generalized extreme value distribution. The pdf of the Gumbel distribution is as follows:
where
The pdf of the inverse Weibull distribution is as follows:
where
The pdf of the generalized extreme value distribution is shown as follows:
where
Gumbel distribution and inverse Weibull distribution are subsets of the generalized extreme value distribution. The extreme value distribution is a heavy-tailed distribution whose right tail is thicker than the Weibull distribution. Therefore, the extreme value distribution is more accurate when estimating the occurrence probability of extreme wind speeds. However, due to the lack of historical extreme wind speed data, the traditional extreme value distribution can only estimate the annual maximum wind speed distribution and does not consider the monthly or daily extreme winds, thus reducing the reliability of quantifying extreme events (Torrielli et al., 2013). In addition, studies generally believe that there is a threshold wind speed, and the extreme value distribution is not suitable for modeling below the threshold wind speed. However, the estimation results of threshold wind speed are pretty different, so the applicable range of extreme value distribution is unclear, making it difficult to determine the most suitable type of extreme value distribution (Kang et al., 2015).
Gamma distribution is also one of the distributions widely used in wind speed distribution modeling (Aries et al., 2018). The two-parameter gamma distribution is also called the Pearson Type III distribution:
where
If the logarithm of a random variable conforms to the gamma distribution, the random variable follows the Log Pearson type III distribution:
where
The random variable that obeys the Gamma distribution takes the reciprocal to get the inverse Gamma distribution, also known as the Pearson type IV distribution (Masseran, 2015):
where
The above distributions are subsets of the generalized Gamma distribution. The generalized Gamma distribution is one of the earliest probability distribution models applied to wind speed modeling (Guedes et al., 2020), and its pdf is shown as follows:
where
where
Kiss and Jánosi (2008) proposed that the fitting effect of the generalized Gamma distribution is significantly better than that of the Weibull distribution, especially for high wind speeds, and is suitable for regions with different underlying surfaces and climatic conditions in Europe. Sarkar et al. (2017) proposed that the fitting effect of the Gamma distribution and the Weibull distribution are close, and the Gamma distribution can be used as an alternative model for the Weibull distribution in low ranges. However, the analytical expression of the wind power density function derived from the Gamma distribution is complicated, and the analytical expressions of the mean, variance, skewness, and kurtosis of the wind power density function cannot be determined, which affects the efficiency of wind energy potential assessment (Samal, 2021). Therefore, to simplify the calculation process, the Gamma distribution cannot be the first choice for the wind speed distribution model.
Several probability distribution models containing more than three parameters have been proposed to characterize the wind speed distribution to pursue higher fitting accuracy. Commonly used multi-parameter probability distributions include the four-parameter Burr distribution, Johnson SB distribution, Kappa distribution, and the five-parameter Wakeby distribution. The pdf of Burr distribution is as follows:
where
The pdf of Johnson SB distribution is shown as follows:
where
The pdf of Kappa distribution is shown as follows:
where
The pdf of Wakeby distribution is as follows:
where
Lo Brano et al. (2011) proposed that the Burr distribution provides high fitting accuracy for wind speed data in southern Italy. The results of Jung and Schindler (2017) showed that Wakeby distribution and Kappa distribution are suitable choices for onshore and offshore wind speed distribution models, respectively. Soukissian (2013) proposed that Johnson SB fits the wind speed data measured in the Mediterranean Sea well. Because the multi-parameter probability distribution contains more parameters, it is more flexible, more adaptable, and has higher fitting accuracy, which reduces the error of wind energy estimation. However, compared with the two-parameter or three-parameter probability distribution models, its complexity is also greatly improved. Therefore, if the multi-parameter probability distribution model does not significantly improve the estimation accuracy, it is not recommended to prioritize this type of model in most cases.
The above models are all single wind speed distributions. The single distribution cannot describe complex wind regimes, especially the wind speed distribution with bimodal or multimodal characteristics (Santos et al., 2021). Therefore, previous studies tend to use mixture distributions to assess the wind energy potential under complex wind regimes. The pdf of the mixture distributions are as follows:
Generally, for special wind regimes, the fitting effect of mixture distributions is better than that of single wind speed distributions. Carta and Ramírez (2007) used a two-component mixture Weibull distribution to describe the wind regimes at weather stations in Spain. Ouarda et al. (2015) proposed that mixed distributions, such as mixed Weibull and Gamma distributions, fit bimodal wind speed regimes better than single distributions. However, there are still some difficulties in using mixture distributions appropriately. Firstly, it is difficult to determine which one or several single distribution models are used to construct a mixed distribution. The fitting effects of mixed distributions constructed from different single wind speed distributions are significantly different (Hu et al., 2016). Secondly, the optimal number of single distributions to form a mixture distribution cannot be calculated, and the researcher often subjectively determines it (Ouarda and Charron, 2018). In addition, the parameter estimation process of the mixture distribution is more complicated, which leads to over-parameterization. Therefore, the use of mixture distributions has limitations.
Although parametric distributions have certain advantages in wind speed modeling, choosing a qualified distribution is still challenging. The theoretical probability distribution model may not describe the actual wind regimes, and the estimated parameter values may not pass statistical tests (Xu et al., 2015). The nonparametric model does not need to make any assumptions about the theoretical distribution of wind speed, nor does it need to estimate the parameters of any distribution. Its parameters can be automatically learned from the historical data (Qin et al., 2011). Commonly used nonparametric models include kernel density estimation (KDE) and maximum entropy principle (MEP).
KDE can get the pdf from the sample data:
where
where
KDE models have substantial flexibility and stability. Han et al. (2019) used wind speed data from a total of 698 wind stations across China, and the test results showed that the effect of the KDE model is better than that of the Weibull distribution and other 18 commonly used parametric distribution models. The fitting results of historical wind speed data from four wind sites in central China showed that KDE has higher accuracy (Han et al., 2018). KDE model needs to select an appropriate bandwidth. Otherwise, there will be over-fitting or under-fitting, which will significantly affect the estimated value. Although several methods for selecting bandwidth, determining the best bandwidth is still challenging (Tenreiro, 2011).
The content of MEP theory is that under some constraints, the distribution model should reach the maximum remaining uncertainty (i.e., maximum entropy):
where
where
where
where
MEP has strong flexibility and can describe complex wind regimes with a large proportion of null wind and a bimodal distribution (Zhou et al., 2010). In some cases, MEP can more accurately characterize the wind speed distribution than parametric distributions, and the estimation error of wind power density is minor (Chellali et al., 2012). However, MEP has limitations in some cases, such as the difficulty of selecting constraint conditions (Zhang et al., 2014).
Particular parameters define all single distribution models and mixed models. Determining the best value of the parameter has a significant impact on the effect of models in fitting wind speed data. Previous studies have used different methods to estimate the parameters of the wind speed distribution models, but calculating the best value of the parameters is still a challenging task.
MLM needs first to construct a likelihood function or a log-likelihood function and then seek the parameter value that makes the function reach the maximum value. MLM estimates the parameter values of the probability distribution through numerical iteration, and the most commonly used method is Newton’s method (Tosunoğlu, 2018). The maximum likelihood estimator is asymptotically unbiased, consistent, and asymptotically effective, and it can reach the minimum variance. In addition, MLM can estimate sample variance to construct confidence intervals and perform hypothesis testing, suitable for analyzing wind speed data in the form of time series (Ramírez and Carta, 2005). However, MLM is more sensitive to the initial value. When the upper and lower limits of the parameters are unknown, its effect is poor (Flynn, 2006). The numerical iterative method requires a large amount of calculation, especially when estimating the parameters of a multi-parameter model or a mixture model, the efficiency of MLM is low (Seo et al., 2019). Some studies have applied improved MLM, called the alternative maximum likelihood method (AMLM), to modeling wind speed distribution (Akdağ and Güler, 2015). AMLM is based on the idea of linearizing the nonlinear term in the likelihood equation through Taylor series expansion and derives the parameter estimator in a non-iterative manner. MLM and AMLM are often used to estimate the shape parameter and scale parameter of the Weibull distribution. Previous studies have shown that the accuracy of the two methods will show differences due to the different geographical locations of wind speed observations (Chaurasiya et al., 2018).
LSM is known as the graphic method. The parameter value estimated by LSM minimizes the sum of squares of the deviations between the empirical cdf and the cdf of the model
where
Some studies have proposed that the accuracy and robustness of LSM are lower than other parameter estimation methods, which may be related to the error in the definition of the cdf (Chang, 2011). Deep et al. (2020) used the modified LSM for parameter estimation, and the results show that its effect is not inferior to other methods. LSM needs to linearize the objective function, and the logarithmic transformation is the basis of LSM. The cdf of the Weibull and Rayleigh distributions contains the exponential term, which is easy to perform the logarithmic transformation, so it is more suitable to use LSM to estimate its parameters. However, linearization of Lognormal and Gamma distribution is more complicated, making LSM unsuitable for these two models (Alrashidi et al., 2020).
The first step of MOM is generally to calculate the first four moments of a random variable:
where
Some studies have proposed the linear moment estimation method (LMOM) for parameter estimation based on MOM. LMOM needs to calculate the probability weighted moments:
where
LMOM estimator is unbiased, it is more stable for samples with abnormal data, and better results will be obtained with a smaller sample size (Soukissian and Tsalis, 2018). However, LMOM, like MOM, cannot fully utilize all the valid information in the sample. When other parameter estimation methods are difficult to calculate parameters, MOM and LMOM are effective alternative methods.
Akdağ and Dinler (2009) proposed a new method for calculating the shape and scale parameters of the Weibull distribution. The relationship between the scale parameter
where
As long as the average wind speed value is obtained, PDM is applicable and does not require complete historical wind speed data. PDM does not need to calculate complicated iterative equations, so the numerical solution of the parameters can be obtained easily. In addition, the wind power density can be directly estimated by this method. The results of Shu et al. (2015) showed that the accuracy of PDM is not worse than other traditional parameter estimation methods. However, PDM is currently only suitable for estimating the parameters of the Weibull distribution. Other probability distribution models may not be able to derive the power density expression from Eq. 1, or the expression may be too complicated to perform the next step. Therefore, the scope of the application of PDM is minimal.
After the distribution model and the parameter values are determined, it is necessary to evaluate the goodness-of-fit of the model. The goodness-of-fit criteria reflect how well the selected model fits the wind speed data so that the applicability of the model to the sample can be evaluated. Choosing different goodness-of-fit criteria may lead to different results.
There are several commonly used variants of
where
The second variant is
where
The third variation is the adjusted coefficient of determination (
where
The closer the value of
The closer the
The KS and AD tests are used to determine whether a given probability distribution model is suitable for a set of wind speed observation data. They are also used to compare the goodness-of-fit of different models to the same set of data. Both the KS and AD tests compare the empirical cumulative probability with the estimated cumulative probability distribution. Specifically, the KS test calculates the maximum difference between the two:
The KS test is an accurate nonparametric test suitable for a continuous distribution. It is more sensitive to the middle part of the distribution.
AD test is improved based on KS test:
The AD test is related to the weight function and is more sensitive to the tail of the distribution. Both the KS and AD tests have a critical value. If these two test statistics are lower than the critical value, the assumed distribution is accepted. The critical value of the KS test is independent of the selected model, and the critical value of the AD test varies with the model, so the AD test is more accurate (Saeed et al., 2021).
χ2 can verify whether the measured wind speed data frequency is consistent with the frequency obtained from the assumed model. It is often used to compare the goodness-of-fit of different models. The chi-square test needs to divide the observation data into several groups, and the frequency of each group is expressed in the form of histograms. Then calculate the test statistics:
where,
If the value of
The fitting effect of the wind speed distribution model usually varies according to the location of different sites (Ouarda et al., 2016). This section uses a case study to compare the fitting effects of the two-parameter Weibull distribution and the three-parameter Weibull distribution at different sites. Two sites are selected in this study. Shengsi is located in the southeast of the Yangtze River estuary and is an island on the East China Sea. Xianyang is a city located in the Guanzhong Basin, surrounded by mountains (Figure 3). Table 1 introduces the altitude, geographic coordinates, period of record, maximum wind speed, and the probability of null wind speed of the two sites. The wind speed data are obtained from the Integrated Surface Database of the National Centers for Environmental Information (www.ncei.noaa.gov/maps/hourly/). The resolution of wind speed data is 1 m/s, and it is recorded every three hours. Previous studies have shown that the wind direction has almost no influence on the wind energy output, and the output of each direction is not distinguished (Kiss and Jánosi, 2008). Therefore, this case study uses wind speed data aggregated from all directions.
TABLE 1. The altitude, geographic coordinates, period of record, maximum wind speed, and the probability of null wind speed of the two sites in Shengsi and Xianyang.
The two-parameter Weibull distribution and the three-parameter Weibull distribution are used to fit the wind speed observation data of the two sites, respectively. To ensure that the fitting effects are comparable, the Least Squares Method is used to estimate the parameter values, and
FIGURE 4. Fitting curves of two-parameter Weibull distribution and three-parameter Weibull distribution at Shengsi and Xianyang sites.
The wind speed distribution characteristics of different sites determine the applicability of the models. Shengsi is located in the coastal area of eastern China, where there is a thermal difference between land and sea, coupled with the influence of the East Asian monsoon and tropical storms, resulting in strong winds throughout the year. Xianyang is located in the inland area, affected by the continental climate, causing a large proportion of null wind. In addition, the topography also affects the characteristics of wind speed distribution (Kim and Lim, 2017). The mountains around Xianyang block the airflow movement, while the terrain of Shengsi is flat, and the airflow is less obstructed.
For a specific site, the wind speed distribution may have different characteristics over time. Xiao et al. (2021) proposed that the wind speed in the Badain Jaran Desert in China reached the highest in April, followed by November, which was much higher than the wind speed in other months. Usta and Kantar (2012) proposed that the statistical characteristics of monthly, seasonal, and yearly wind speed data, such as mean, variance, skewness, and kurtosis, are significantly different. Therefore, the optimal wind speed distribution model under different time scales of wind speed data may be different. Some studies have conducted wind energy assessments on longer time scales. Gao et al. (2018) proposed that climate warming has led to a gradual decline in the long-term wind energy potential of the Indian Ocean, which will affect the economic income of wind farms. Shu et al. (2015) proposed that long-term temperature variations will lead to variations in wind characteristics, which will change the energy output. However, on the one hand, the trend of climate change is very complex and has not been fully understood, making it difficult to predict long-term variations in wind characteristics. On the other hand, the location of wind farms is often determined only by wind speed observation data within 1–2 years or even less (Chen and Blaabjerg, 2009). If the wind energy potential of the area decreases year by year due to rising temperatures, the output of wind farms may not meet expectations. Therefore, wind energy assessment should consider the impact of long-term climate change rather than focusing only on the current output of the site.
Previous studies have proposed a variety of probability distribution models for the modeling of wind speed distribution. In different sites, the models have shown particular applicability, but a specific model has not been found to have the best fitting effect on the wind speed data recorded at all sites. In addition, the selection of parameter estimation methods and the setting of goodness-of-fit test statistics are not unified. They have their advantages and disadvantages and are suitable for different observation data and wind speed distribution models. Therefore, all potential wind speed distribution models should be considered for selecting the wind speed distribution model for a particular site. According to the chosen model and the characteristics of the wind speed observation data, the parameter estimation method and the goodness-of-fit test statistics are determined.
Wind characteristics determine the wind energy output of a wind farm. The characteristics of wind speed distribution are constantly varying geographically and temporally. The wind characteristics at different locations are affected by various geographical elements, such as local climate, topography, and thermal properties difference between the land and the sea. The variations in these geographical elements make the applicability of a model in different regions may change significantly. The wind speed distribution in different months, seasons, and years may have different characteristics for a specific site. Long-term variations in wind characteristics are a topic of great concern because they will lead to gradual variations in wind energy potential and affect the economic benefits of wind farms. We suggest that wind energy assessment consider the long-term variations in local wind characteristics instead of just focusing on the current energy output.
SH contributed to conceptualization. DZ contributed to funding acquisition. XN contributed to methodology. HQ contributed to formal analysis. All authors contributed to the article and approved the submitted version.
This work was supported by the National Natural Science Foundation of China (grant numbers 41871008, 41930641, 41871011, and 41571008).
The authors declare that the research was conducted in the absence of any commercial or financial relationships that could be construed as a potential conflict of interest.
All claims expressed in this article are solely those of the authors and do not necessarily represent those of their affiliated organizations or those of the publisher, the editors, and the reviewers. Any product that may be evaluated in this article, or claim that may be made by its manufacturer, is not guaranteed or endorsed by the publisher.
Akdağ, S. A., Bagiorgas, H. S., and Mihalakakou, G. (2010). Use of Two-Component Weibull Mixtures in the Analysis of Wind Speed in the Eastern Mediterranean. Appl. Energ. 87 (8), 2566–2573. doi:10.1016/j.apenergy.2010.02.033
Akdağ, S. A., and Dinler, A. (2009). A New Method to Estimate Weibull Parameters for Wind Energy Applications. Energy Convers. Manag. 50 (7), 1761–1766. doi:10.1016/j.enconman.2009.03.020
Akdağ, S. A., and Güler, Ö. (2015). A Novel Energy Pattern Factor Method for Wind Speed Distribution Parameter Estimation. Energ. Convers. Manag. 106, 1124–1133. doi:10.1016/j.enconman.2015.10.042
Akgül, F. G., Şenoğlu, B., and Arslan, T. (2016). An Alternative Distribution to Weibull for Modeling the Wind Speed Data: Inverse Weibull Distribution. Energ. Convers. Manag. 114, 234–240. doi:10.1016/j.enconman.2016.02.026
Alavi, O., Mohammadi, K., and Mostafaeipour, A. (2016a). Evaluating the Suitability of Wind Speed Probability Distribution Models: A Case of Study of East and Southeast Parts of Iran. Energ. Convers. Manag. 119, 101–108. doi:10.1016/j.enconman.2016.04.039
Alavi, O., Sedaghat, A., and Mostafaeipour, A. (2016b). Sensitivity Analysis of Different Wind Speed Distribution Models with Actual and Truncated Wind Data: A Case Study for Kerman, Iran. Energ. Convers. Manag. 120, 51–61. doi:10.1016/j.enconman.2016.04.078
Alrashidi, M., Rahman, S., and Pipattanasomporn, M. (2020). Metaheuristic Optimization Algorithms to Estimate Statistical Distribution Parameters for Characterizing Wind Speeds. Renew. Energ. 149, 664–681. doi:10.1016/j.renene.2019.12.048
Arenas-López, J. P., and Badaoui, M. (2020). A Fokker–Planck Equation Based Approach for Modelling Wind Speed and its Power Output. Energ. Convers. Manag. 222, 113152. doi:10.1016/j.enconman.2020.113152
Aries, N., Boudia, S. M., and Ounis, H. (2018). Deep Assessment of Wind Speed Distribution Models: A Case Study of Four Sites in Algeria. Energ. Convers. Manag. 155, 78–90. doi:10.1016/j.enconman.2017.10.082
Baz, K., Cheng, J., Xu, D., Abbas, K., Ali, I., Ali, H., et al. (2021). Asymmetric Impact of Fossil Fuel and Renewable Energy Consumption on Economic Growth: A Nonlinear Technique. Energy 226, 120357. doi:10.1016/j.energy.2021.120357
Bilir, L., İmir, M., Devrim, Y., and Albostan, A. (2015). An Investigation on Wind Energy Potential and Small Scale Wind Turbine Performance at İncek Region – Ankara, Turkey. Energ. Convers. Manag. 103, 910–923. doi:10.1016/j.enconman.2015.07.017
Carta, J. A., and Ramírez, P. (2007). Analysis of Two-Component Mixture Weibull Statistics for Estimation of Wind Speed Distributions. Renew. Energ. 32 (3), 518–531. doi:10.1016/j.renene.2006.05.005
Carta, J. A., Ramírez, P., and Velázquez, S. (2009). A Review of Wind Speed Probability Distributions Used in Wind Energy Analysis. Renew. Sustain. Energ. Rev 13 (5), 933–955. doi:10.1016/j.rser.2008.05.005
Celik, A. N. (2006). A Simplified Model for Estimating Yearly Wind Fraction in Hybrid-Wind Energy Systems. Renew. Energ. 31 (1), 105–118. doi:10.1016/j.renene.2005.03.006
Celik, A. N. (2003). Energy Output Estimation for Small-Scale Wind Power Generators Using Weibull-Representative Wind Data. J. Wind Eng. Ind. Aerod 91 (5), 693–707. doi:10.1016/s0167-6105(02)00471-3
Chang, T. P. (2011). Performance Comparison of Six Numerical Methods in Estimating Weibull Parameters for Wind Energy Application. Appl. Energ. 88 (1), 272–282. doi:10.1016/j.apenergy.2010.06.018
Chaurasiya, P. K., Ahmed, S., and Warudkar, V. (2018). Study of Different Parameters Estimation Methods of Weibull Distribution to Determine Wind Power Density Using Ground Based Doppler SODAR Instrument. ALEX ENG. J. 57 (4), 2299–2311. doi:10.1016/j.aej.2017.08.008
Chellali, F., Khellaf, A., Belouchrani, A., and Khanniche, R. (2012). A Comparison between Wind Speed Distributions Derived from the Maximum Entropy Principle and Weibull Distribution. Case of Study; Six Regions of Algeria. Renew. Sustain. Energ. Rev 16 (1), 379–385. doi:10.1016/j.rser.2011.08.002
Chen, Z., and Blaabjerg, F. (2009). Wind Farm—A Power Source in Future Power Systems. Renew. Sustain. Energ. Rev 13 (6-7), 1288–1300. doi:10.1016/j.rser.2008.09.010
Deep, S., Sarkar, A., Ghawat, M., and Rajak, M. K. (2020). Estimation of the Wind Energy Potential for Coastal Locations in India Using the Weibull Model. Renew. Energ. 161, 319–339. doi:10.1016/j.renene.2020.07.054
Elie Bertrand, K. S., Abraham, K., and Lucien, M. A. (2020). Sustainable Energy through Wind Speed and Power Density Analysis in Ambam, South Region of Cameroon. Front Energ. Res 8, 176. doi:10.3389/fenrg.2020.00176
Flynn, M. R. (2006). Fitting Human Exposure Data with the Johnson S(B) Distribution. J. Expo. Sci. Environ. Epidemiol. 16 (1), 56–62. doi:10.1038/sj.jea.7500437
Gao, M., Ding, Y., Song, S., Lu, X., Chen, X., and McElroy, M. B. (2018). Secular Decrease of Wind Power Potential in India Associated with Warming in the Indian Ocean. Sci. Adv. 4 (12), eaat5256. doi:10.1126/sciadv.aat5256
Guarienti, J. A., Kaufmann Almeida, A., Menegati Neto, A., de Oliveira Ferreira, A. R., Ottonelli, J. P., and Kaufmann de Almeida, I. (2020). Performance Analysis of Numerical Methods for Determining Weibull Distribution Parameters Applied to Wind Speed in Mato Grosso Do Sul, Brazil. Sustain. Energ. Techn 42, 100854. doi:10.1016/j.seta.2020.100854
Guedes, K. S., de Andrade, C. F., Rocha, P. A. C., Mangueira, R. d. S., and de Moura, E. P. (2020). Performance Analysis of Metaheuristic Optimization Algorithms in Estimating the Parameters of Several Wind Speed Distributions. Appl. Energ. 268, 114952. doi:10.1016/j.apenergy.2020.114952
Han, Q., Hao, Z., Hu, T., and Chu, F. (2018). Non-parametric Models for Joint Probabilistic Distributions of Wind Speed and Direction Data. Renew. Energ. 126, 1032–1042. doi:10.1016/j.renene.2018.04.026
Han, Q., Ma, S., Wang, T., and Chu, F. (2019). Kernel Density Estimation Model for Wind Speed Probability Distribution with Applicability to Wind Energy Assessment in China. Renew. Sustain. Energ. Rev 115, 109387. doi:10.1016/j.rser.2019.109387
Harris, R. I., and Cook, N. J. (2014). The Parent Wind Speed Distribution: Why Weibull?. J. Wind Eng. Ind. Aerod 131, 72–87. doi:10.1016/j.jweia.2014.05.005
Hossain, J., Sharma, S., and Kishore, V. V. N. (2014). Multi-peak Gaussian Fit Applicability to Wind Speed Distribution. Renew. Sustain. Energ. Rev 34, 483–490. doi:10.1016/j.rser.2014.03.026
Hu, Q., Wang, Y., Xie, Z., Zhu, P., and Yu, D. (2016). On Estimating Uncertainty of Wind Energy with Mixture of Distributions. Energy 112, 935–962. doi:10.1016/j.energy.2016.06.112
Jansen, M., Staffell, I., Kitzing, L., Quoilin, S., Wiggelinkhuizen, E., Bulder, B., et al. (2020). Offshore Wind Competitiveness in Mature Markets without Subsidy. Nat. Energ. 5 (8), 614–622. doi:10.1038/s41560-020-0661-2
Jia, J., Yan, Z., Peng, X., and An, X. (2020). A New Distribution for Modeling the Wind Speed Data in Inner Mongolia of China. Renew. Energ. 162, 1979–1991. doi:10.1016/j.renene.2020.10.019
Jin, X., Chen, Y., Wang, L., Han, H., and Chen, P. (2021). Failure Prediction, Monitoring and Diagnosis Methods for Slewing Bearings of Large-Scale Wind Turbine: A Review. Measurement 172, 108855. doi:10.1016/j.measurement.2020.108855
Jung, C., and Schindler, D. (2017). Global Comparison of the Goodness-Of-Fit of Wind Speed Distributions. Energy Convers. Manag 133, 216–234. doi:10.1016/j.enconman.2016.12.006
Kandpal, D., and Dhingra, T. (2021). Migrating to Reverse Auction Mechanisms in Wind Energy Sector: Status and Challenges. Energ Policy 156, 112352. doi:10.1016/j.enpol.2021.112352
Kang, D., Ko, K., and Huh, J. (2015). Determination of Extreme Wind Values Using the Gumbel Distribution. Energy 86, 51–58. doi:10.1016/j.energy.2015.03.126
Kaplan, O., and Temiz, M. (2017). A Novel Method Based on Weibull Distribution for Short-Term Wind Speed Prediction. Int. J. Hydrogen Energ 42 (28), 17793–17800. doi:10.1016/j.ijhydene.2017.03.006
Katikas, L., Dimitriadis, P., Koutsoyiannis, D., Kontos, T., and Kyriakidis, P. (2021). A Stochastic Simulation Scheme for the Long-Term Persistence, Heavy-Tailed and Double Periodic Behavior of Observational and Reanalysis Wind Time-Series. Appl. Energ. 295, 116873. doi:10.1016/j.apenergy.2021.116873
Kim, Y., and Lim, H. (2017). Effect of Island Topography and Surface Roughness on the Estimation of Annual Energy Production of Offshore Wind Farms. Renew. Energ. 103, 106–114. doi:10.1016/j.renene.2016.11.020
Kiss, P., and Jánosi, I. M. (2008). Comprehensive Empirical Analysis of ERA-40 Surface Wind Speed Distribution over Europe. Energ. Convers. Manag. 49 (8), 2142–2151. doi:10.1016/j.enconman.2008.02.003
Li, D., and Miao, S. (2021). Fitting the Wind Speed Probability Distribution with Maxwell and Power Maxwell Distributions: A Case Study of North Dakota Sites. Sustain. Energ. Techn 47, 101446. doi:10.1016/j.seta.2021.101446
Liu, G., Zhou, J., Jia, B., He, F., Yang, Y., and Sun, N. (2019). Advance Short-Term Wind Energy Quality Assessment Based on Instantaneous Standard Deviation and Variogram of Wind Speed by a Hybrid Method. Appl. Energ. 238, 643–667. doi:10.1016/j.apenergy.2019.01.105
Liu, M., Li, W., Billinton, R., Wang, C., and Yu, J. (2015). Modeling Tidal Current Speed Using a Wakeby Distribution. Electr. Pow Syst. Res. 127, 240–248. doi:10.1016/j.epsr.2015.06.014
Lo Brano, V., Orioli, A., Ciulla, G., and Culotta, S. (2011). Quality of Wind Speed Fitting Distributions for the Urban Area of Palermo, Italy. Renew. Energ. 36 (3), 1026–1039. doi:10.1016/j.renene.2010.09.009
Masseran, N. (2015). Evaluating Wind Power Density Models and Their Statistical Properties. Energy 84, 533–541. doi:10.1016/j.energy.2015.03.018
Mert, İ., and KarakuŞ, C. (2015). A Statistical Analysis of Wind Speed Data Using Burr, Generalized Gamma, and Weibull Distributions in Antakya, Turkey. Turk J. Electr. Eng. Co 23, 1571–1586. doi:10.3906/elk-1402-66
Miao, S., Gu, Y., Li, D., and Li, H. (2019). Determining Suitable Region Wind Speed Probability Distribution Using Optimal Score-Radar Map. Energ. Convers. Manag. 183, 590–603. doi:10.1016/j.enconman.2019.01.001
Montoya, J. A., Díaz-Francés, E., and P, G. F. (2019). Estimation of the Reliability Parameter for Three-Parameter Weibull Models. Appl. Math. Model. 67, 621–633. doi:10.1016/j.apm.2018.11.043
Morales, J. M., Mínguez, R., and Conejo, A. J. (2010). A Methodology to Generate Statistically Dependent Wind Speed Scenarios. Appl. Energ. 87 (3), 843–855. doi:10.1016/j.apenergy.2009.09.022
Ouarda, T. B. M. J., Charron, C., and Chebana, F. (2016). Review of Criteria for the Selection of Probability Distributions for Wind Speed Data and Introduction of the Moment and L-Moment Ratio Diagram Methods, with a Case Study. Energ. Convers. Manag. 124, 247–265. doi:10.1016/j.enconman.2016.07.012
Ouarda, T. B. M. J., and Charron, C. (2018). On the Mixture of Wind Speed Distribution in a Nordic Region. Energ. Convers. Manag. 174, 33–44. doi:10.1016/j.enconman.2018.08.007
Ouarda, T. B. M. J., Charron, C., Shin, J. Y., Marpu, P. R., Al-Mandoos, A. H., Al-Tamimi, M. H., et al. (2015). Probability Distributions of Wind Speed in the UAE. Energ. Convers. Manag. 93, 414–434. doi:10.1016/j.enconman.2015.01.036
Perrin, O., Rootzén, H., and Taesler, R. (2006). A Discussion of Statistical Methods Used to Estimate Extreme Wind Speeds. Theor. Appl. Climatol 85 (3-4), 203–215. doi:10.1007/s00704-005-0187-3
Petković, D., Shamshirband, S., Anuar, N. B., Saboohi, H., Abdul Wahab, A. W., Protić, M., et al. (2014). An Appraisal of Wind Speed Distribution Prediction by Soft Computing Methodologies: A Comparative Study. Energ. Convers. Manag. 84, 133–139. doi:10.1016/j.enconman.2014.04.010
Qin, Z., Li, W., and Xiong, X. (2011). Estimating Wind Speed Probability Distribution Using Kernel Density Method. Electr. Pow Syst. Res. 81 (12), 2139–2146. doi:10.1016/j.epsr.2011.08.009
Ramírez, P., and Carta, J. A. (2005). Influence of the Data Sampling Interval in the Estimation of the Parameters of the Weibull Wind Speed Probability Density Distribution: a Case Study. Energ. Convers. Manag. 46 (15-16), 2419–2438. doi:10.1016/j.enconman.2004.11.004
Saeed, M. A., Ahmed, Z., and Zhang, W. (2021). Optimal Approach for Wind Resource Assessment Using Kolmogorov–Smirnov Statistic: A Case Study for Large-Scale Wind Farm in Pakistan. Renew. Energ. 168, 1229–1248. doi:10.1016/j.renene.2021.01.008
Saleh, H., Abou El-Azm Aly, A., and Abdel-Hady, S. (2012). Assessment of Different Methods Used to Estimate Weibull Distribution Parameters for Wind Speed in Zafarana Wind Farm, Suez Gulf, Egypt. Energy 44 (1), 710–719. doi:10.1016/j.energy.2012.05.021
Samal, R. K. (2021). Assessment of Wind Energy Potential Using Reanalysis Data: A Comparison with Mast Measurements. J. Clean. Prod. 313, 127933. doi:10.1016/j.jclepro.2021.127933
Santos, F. S. d., Nascimento, K. K. F. d., Jale, J. d. S., Stosic, T., Marinho, M. H. N., and Ferreira, T. A. E. (2021). Mixture Distribution and Multifractal Analysis Applied to Wind Speed in the Brazilian Northeast Region. Chaos Soliton Fract 144, 110651. doi:10.1016/j.chaos.2021.110651
Sarkar, A., Deep, S., Datta, D., Vijaywargiya, A., Roy, R., and Phanikanth, V. S. (2019). Weibull and Generalized Extreme Value Distributions for Wind Speed Data Analysis of Some Locations in India. Ksce J. Civ Eng. 23 (8), 3476–3492. doi:10.1007/s12205-019-1538-4
Sarkar, A., Gugliani, G., and Deep, S. (2017). Weibull Model for Wind Speed Data Analysis of Different Locations in India. Ksce J. Civ Eng. 21 (7), 2764–2776. doi:10.1007/s12205-017-0538-5
Seo, S., Oh, S.-D., and Kwak, H.-Y. (2019). Wind Turbine Power Curve Modeling Using Maximum Likelihood Estimation Method. Renew. Energ. 136, 1164–1169. doi:10.1016/j.renene.2018.09.087
Shu, Z. R., Li, Q. S., and Chan, P. W. (2015). Statistical Analysis of Wind Characteristics and Wind Energy Potential in Hong Kong. Energ. Convers. Manag. 101, 644–657. doi:10.1016/j.enconman.2015.05.070
Soukissian, T. H., and Tsalis, C. (2018). Effects of Parameter Estimation Method and Sample Size in Metocean Design Conditions. Ocean Eng. 169, 19–37. doi:10.1016/j.oceaneng.2018.09.017
Soukissian, T. (2013). Use of Multi-Parameter Distributions for Offshore Wind Speed Modeling: The Johnson SB Distribution. Appl. Energ. 111, 982–1000. doi:10.1016/j.apenergy.2013.06.050
Tenreiro, C. (2011). Fourier Series-Based Direct Plug-In Bandwidth Selectors for Kernel Density Estimation. J. Nonparametr Stat. 23, 533–545. doi:10.1080/10485252.2010.537337
Torrielli, A., Repetto, M. P., and Solari, G. (2013). Extreme Wind Speeds from Long-Term Synthetic Records. J. Wind Eng. Ind. Aerod 115, 22–38. doi:10.1016/j.jweia.2012.12.008
Tosunoğlu, F. (2018). Accurate Estimation of T Year Extreme Wind Speeds by Considering Different Model Selection Criterions and Different Parameter Estimation Methods. Energy 162, 813–824. doi:10.1016/j.energy.2018.08.074
ul Haq, M. A., Rao, G. S., Albassam, M., and Aslam, M. (2020). Marshall–Olkin Power Lomax Distribution for Modeling of Wind Speed Data. Energy Rep 6, 1118–1123. doi:10.1016/j.egyr.2020.04.033
Usta, I., and Kantar, Y. M. (2012). Analysis of Some Flexible Families of Distributions for Estimation of Wind Speed Distributions. Appl. Energ. 89 (1), 355–367. doi:10.1016/j.apenergy.2011.07.045
Valencia Ochoa, G., Núñez Alvarez, J., and Vanegas Chamorro, M. (2019). Data Set on Wind Speed, Wind Direction and Wind Probability Distributions in Puerto Bolivar - Colombia. Data Brief 27, 104753. doi:10.1016/j.dib.2019.104753
Wais, P. (2017a). A Review of Weibull Functions in Wind Sector. Renew. Sustain. Energ. Rev 70, 1099–1107. doi:10.1016/j.rser.2016.12.014
Wais, P. (2017b). Two and Three-Parameter Weibull Distribution in Available Wind Power Analysis. Renew. Energ. 103, 15–29. doi:10.1016/j.renene.2016.10.041
Wang, J., Hu, J., and Ma, K. (2016). Wind Speed Probability Distribution Estimation and Wind Energy Assessment. Renew. Sustain. Energ. Rev 60, 881–899. doi:10.1016/j.rser.2016.01.057
Wang, J., Qin, S., Jin, S., and Wu, J. (2015). Estimation Methods Review and Analysis of Offshore Extreme Wind Speeds and Wind Energy Resources. Renew. Sustain. Energ. Rev 42, 26–42. doi:10.1016/j.rser.2014.09.042
Wang, Y., Li, Y., Zou, R., and Song, D. (2021). Bayesian Infinite Mixture Models for Wind Speed Distribution Estimation. Energ. Convers. Manag. 236, 113946. doi:10.1016/j.enconman.2021.113946
Weibull, W. (1951). A Statistical Distribution Function of Wide Applicability. J. Appl. Mech. 18, 293–297. doi:10.1115/1.4010337
Xiao, N., Dong, Z., Xiao, S., Wang, J., and Liu, Z. (2021). An Improved Approach to Estimate Sand-Driving Winds. J. Clean. Prod. 285, 124820. doi:10.1016/j.jclepro.2020.124820
Xu, X., Yan, Z., and Xu, S. (2015). Estimating Wind Speed Probability Distribution by Diffusion-Based Kernel Density Method. Electr. Pow Syst. Res. 121, 28–37. doi:10.1016/j.epsr.2014.11.029
Zhang, H., Yu, Y.-J., and Liu, Z.-Y. (2014). Study on the Maximum Entropy Principle Applied to the Annual Wind Speed Probability Distribution: A Case Study for Observations of Intertidal Zone Anemometer Towers of Rudong in East China Sea. Appl. Energ. 114, 931–938. doi:10.1016/j.apenergy.2013.07.040
Keywords: renewable energy, wind energy assessment, wind speed distribution, parameter estimation method, goodness-of-fit criteria
Citation: Shi H, Dong Z, Xiao N and Huang Q (2021) Wind Speed Distributions Used in Wind Energy Assessment: A Review. Front. Energy Res. 9:769920. doi: 10.3389/fenrg.2021.769920
Received: 02 September 2021; Accepted: 04 November 2021;
Published: 22 November 2021.
Edited by:
Joanna Staneva, Institute of Coastal Systems Helmholtz Centre Hereon, GermanyCopyright © 2021 Shi, Dong, Xiao and Huang. This is an open-access article distributed under the terms of the Creative Commons Attribution License (CC BY). The use, distribution or reproduction in other forums is permitted, provided the original author(s) and the copyright owner(s) are credited and that the original publication in this journal is cited, in accordance with accepted academic practice. No use, distribution or reproduction is permitted which does not comply with these terms.
*Correspondence: Zhibao Dong, emJkb25nQHNubnUuZWR1LmNu; Nan Xiao, bmFueGlhbzE5OTJAb3V0bG9vay5jb20=
Disclaimer: All claims expressed in this article are solely those of the authors and do not necessarily represent those of their affiliated organizations, or those of the publisher, the editors and the reviewers. Any product that may be evaluated in this article or claim that may be made by its manufacturer is not guaranteed or endorsed by the publisher.
Research integrity at Frontiers
Learn more about the work of our research integrity team to safeguard the quality of each article we publish.