- School of Nuclear Science and Engineering, Oregon State University, Corvallis, OR, United States
This review paper highlights approaches and tools available to the nuclear industry for dynamic probabilistic risk assessment (DPRA) using dynamic event trees. DPRA is an emerging methodology that has advantages as compared to traditional, static PRA predominantly owing to the addition of time dependent modeling. Traditional PRAs predefine events and outcomes into Event Trees (ET) and Fault Trees (FT), that are coupled with various combinations of Initiating Events (IE), Top Events (TE), branches, end states and sequences. A more complete depiction of the system and accident progression behavior can be quantified using DPRA to account for dynamic events such as those involving human actions. This paper discusses the strengths and needs of existing DPRA tools to align with the risk informed methodology currently used in the nuclear industry. DPRA is evolving during an exciting time in the nuclear industry with emerging advanced reactor designs also coming on the scene. Advanced nuclear (Gen IV) designs often incorporate passively safe systems that have less readily available data for traditional PRA due to their limited operating history. DPRA is a promising methodology that can address this challenge and demonstrate to the regulatory bodies and public that advanced designs operate within safety margins. In this light, the paper considers the historical role of PRA in the nuclear industry and motivation for considering dynamic PRA models. An introduction to the differences inherent in DPRA and how it complements and enhances existing PRA approaches is discussed. Additionally, a review of research from U.S national laboratories and universities features recent DPRA tool advancements that could be applied in the nuclear industry. These DPRA approaches and tools are summarized and examined to thoughtfully provide a path forward to best leverage existing research and integrate DPRA into advanced reactor design and analysis.
Introduction
Probabilistic risk assessment (PRA) continues to play a critical role in refining and quantifying risk for the nuclear industry. As early adopters of PRA, the Nuclear Regulatory Commission (NRC) evaluates both the likelihood and consequence of possible scenarios to assess risk (US NRC, 2020a). The use of PRA techniques in the nuclear industry began back in 1977 when the “Lewis Committee” formally endorsed the risk-informed methodology of the 1975 Reactor Safety Study (WASH 1400) (Rasmussen, 1975). This initial evaluation using PRA provided a mechanism to identify and quantify risk such that the community concluded that NPP risks were tolerable. WASH 1400 was followed by the NUREG-1150 with the additional assessment of five operating reactors, in which it considered both internal and external scenarios such as fire and seismic activity (US NRC, 1990). As PRA methodology advanced and the assessment of risk refined, conservative engineering margins were allowed to be reduced while ensuring safety. It was in 1995 that the NRC issued a formal ‘PRA policy statement’ officially adopting PRA as a commitment to their risk informed approach to regulation.
Most PRAs encompass the use of Fault Trees (FT) and Event Trees (ET) to form a success/failure logic. The ET/FT are combined into a quantified probabilistic model to estimate the likelihood of an end state. In the nuclear industry, these adverse consequences are divided into three levels with different starting and end points (Murphy, 1984).
A Level 1 PRA systematically evaluates numerous sequences of events, and depicts the subsystems needed to respond to events, in order to evaluate the probability that the outcome damages the core. This process involves analyzing systems such as the steam generator, reactivity control, and plant monitoring, among others. A commonly referenced product from a Level 1 PRA is the Core Damage Frequency (CDF). Level 2 PRA extends the core damage evaluation (Level 1 PRA) to include all systems designed to mitigate, prevent, or monitor the release of radioactive material. Level 2 PRA aims to identify scenarios to prevent the unexpected release of radiological material. The final Level 3 PRA quantifies the offsite impact to the public and environment as a result of events occurring at a nuclear plant. Collectively, Levels 1–3 comprehensively evaluates the severity and probability of events that can lead to core damage, environmental release, and consequence to the general public (US NRC, 2020).
The beauty of PRA is that it is straightforward and logical in its approach, and the insights gained by constructing these detailed inter-system interactions play a key role in meeting regulatory requirements. However, with the addition of computational resources, PRA models continue to become more complex, straining the PRA tools, practitioners, and model interpretation by non-experts (Miller et al., 2020). One specific example of increased complexity is NEI’s Risk-Managed Technical Specifications Program (NEI, 2006) that relies on ‘real time’ PRA as plant conditions change. Other trends in PRA models include the consideration of multiple external hazards (i.e. flooding and seismic), human action(s) or inaction(s), and the addition of multiple sites (Miller et al., 2020).
Motivation for Dynamic Models
The demand for faster, more complex, risk analysis is advancing the development of new PRA tools to improve PRA technology and guide interpretation, including leveraging machine learning and artificial intelligence (AI). Also becoming more accessible is the ability to integrate more advanced physical models into the risk assessment process to represent a dynamic system. The concept of dynamic PRA (DPRA) has been around for decades; however, tools and computational resources have more recently matured that can more readily extend the existing PRA philosophy to account for dynamic, physics-informed models.
One limitation of traditional PRA is the need for an a priori understanding of performance so the user can assign probabilities for basic events such as pump failure, as well as define initiating events and end states. This inherently bounds PRA analysis to predefined binary failure and success rates. DPRA, in contrast, allows for a system to “play out” multiple scenarios, more accurately accounting for epistemic uncertainties. This dynamic analysis improves upon the static PRA through explicit time modeling, capturing the accident progression and system status as it occurs (Siu, 2020).
Within the nuclear industry there are several motivating factors to consider physically based DPRA models. The Light Water Reactor Sustainability (LWRS) Program has demonstrated interest in DPRA to more realistically account for aging systems structures and components (SCCs), using physics models to capture the impact of the aging process (Yadav et al., 2017). More realistically accounting for component degradation is critical for the extension of existing LWRs in the U.S. Additionally, newer advanced reactor designs will not only need to comply with the NRC’s risk-informed regulatory policy prior to licensing and construction, but are also designed with multiple passive safety features. Traditional PRA often relies on system and component operating knowledge, which is lacking in newer passively safe designs. Dynamic PRA can address this uncertainty by physically simulating these systems rather than rely on past operational data (Siu, 2020).
Another advantage of dynamically integrating physics-based models into the risk assessment process is the ability to depict time-dependent system interactions, including aspects of human behavior (adversarial or otherwise). While some PRA evaluations attempt to capture the impact of human behavior, such as EPRI’s Human Reliability Assessment (HRA) Calculator, human failure is constrained to a single event (EPRI, 2012). However, in DPRA the human action modifies the decision points of the DPRA analysis to create additional branches as part of a sequence. Dynamic PRA allows for human behaviors in the form of repeated fault trees that both accommodate the uncertainty of the timing of human factors and allows the consequence(s) to progress in time more realistically. For example, Sandia National Laboratories (SNL), in support of the LWRS Program, coupled a force-on-force physical security model with a generic MELCOR reactor system model via DPRA methodology (Osborn et al., 2019). This expansion of PRA philosophy incorporates an additional level of realism reflecting adversarial human actions at a nuclear power plant and offering a new risk-informed assessment of physical security.
This paper highlights the extension of risk assessment to dynamic (time -dependent) events, several available tools developed for DPRA analysis, and opportunities and challenges for DPRA in the nuclear industry.
Dynamic PRA
Dynamic PRA shares many characteristics with traditional, static PRA. The basic Event Tree/Fault Tree structure is retained, with basic events connected through Boolean logic to form fault trees for specific Top Events that combine to create Event Trees that represent system wide event probabilities. Dynamic PRA primarily differs in its modeling of any given system over time. Introducing the element of time to traditional PRA can complicate ET/FT analyses. PRA analyses rely heavily on knowledgeable analysts that can build ET/FTs that are realistic. This means there is not really any accounting for time dependent effects, such as when during an accident progression a control room operator takes certain actions (Siu, 2020). This will certainly affect the outcome of any analysis, and so new tools that can appropriately handle the element of time will be a considerable boon to PRA analyses in the nuclear industry.
The addition of time dependency modifies traditional Event Trees into Dynamic Event Trees (DET). A simple example DET is shown in Figure 1. Key differences between a DET and a traditional ET are the differentiation of “time-dependent branches” and “parameter-dependent branches,” the ability for new branches to occur while the problem is ongoing (traditional PRA branches are defined a priori), as well as the ability to accommodate the same branching event occurring more than once, at multiple times within the overall problem (Jankovsky et al., 2018 b.) (Martin et al., 2016). These added features allow for many simplifications and assumptions that would be made in a comparable traditional PRA problem to be eliminated. A more robust and realistic probabilistic risk assessment can now be conducted.
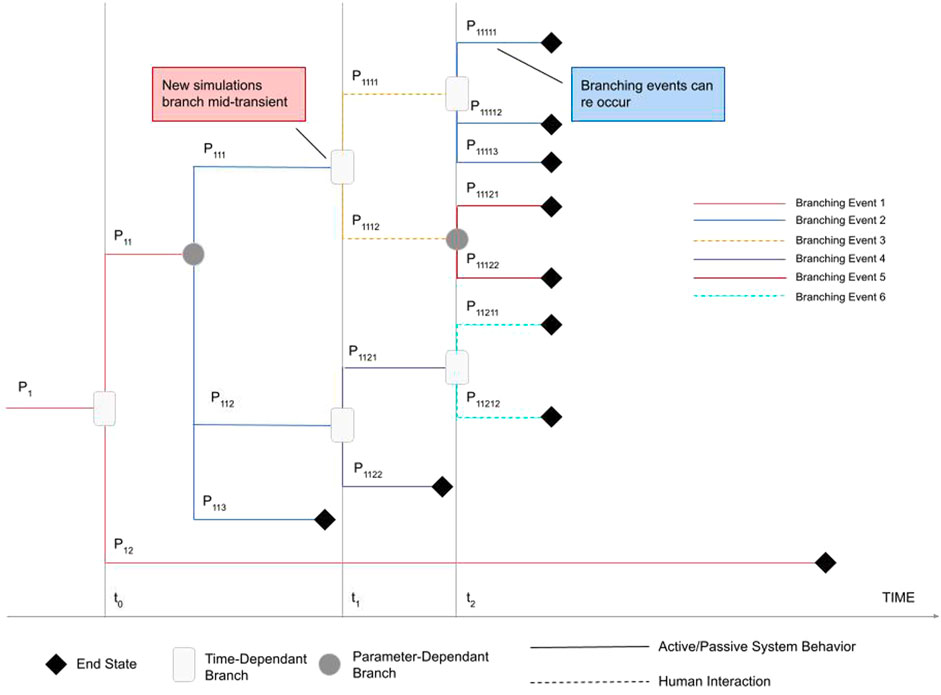
FIGURE 1. Example dynamic event tree (Martin et al., 2016).
At the highest level, dynamic risk assessment methodologies can be split into three groups; continuous-time methods, discrete-time methods, and methods that take advantage of graphical user interfaces (GUIs) (Aldemir, 2013). It is important to recognize that any method that uses a GUI, by definition, is also a method that uses either continuous or discrete timing methods. Every method requires similar inputs in the form of a time-dependent model (such as a RELAP model) that can capture both normal and abnormal system configurations, and configuration transition probabilities that help determine the evolution of the system over time (referred to as branching conditions for the tools described below). Dynamic event trees exist within the discrete timing methods group and are the primary focus of this review paper.
DPRA Tools
Currently, several DPRA tools are available to the nuclear industry with publications surfacing in the 1980s, however the number of practitioners remain few (Siu, 2020). Active research programs in DPRA exist worldwide including PyCATSHOO (EDF France, non-DET driver) (Chraibi, 2018), DICE (the Republic of Korea) (Lee et al., 2018), SCAIS (Spain) (Queral et al., 2018), MCDET (Germany) (Kloos and Peschke, 2006), as well as products resulting from several U.S. university and national laboratory partnerships. This review focuses on four DPRA codes, three of which utilize dynamic event trees and one that graphically resembles more traditional PRA methods.
Monte Carlo Dynamic Event Trees (MCDET), Reactor Analysis and Virtual Control Environment (RAVEN), and Analysis of Dynamic Accident Progression Trees (ADAPT) are well established DET drivers and have several case-studies (Jankovsky et al., 2018 b.). These drivers all aim to perform DPRA by coupling to reactor simulation codes such as the Reactor Excursion Leak Analysis Program (RELAP) or MELCOR. Idaho National Laboratory (INL) also recently developed the Event Modeling Risk Assessment using Linked Diagrams (EMRALD, 2021) code, with the intention of being more user friendly and complementary to the commonly used SAPHIRE code, while still retaining the advantages of Dynamic PRA assessments (Prescott et al., 2018). Coupling DET drivers, or codes like EMRALD, with established reactor physics simulation codes allows for greater fidelity and trustworthiness in results, both highly valuable qualities in the eyes of a PRA analyst. The following subsections provide a brief overview of MCDET, RAVEN, ADAPT, and EMRALD.
MCDET
Each DET driver has slightly different capabilities, leading to each driver having particular applications for which they are better suited. MCDET, a driver developed in Germany, utilizes Monte Carlo sampling of probability distribution functions. Random Monte Carlo sampling allows MCDET to accommodate aleatory (probabilistic variations) and epistemic (lack of parameter knowledge) uncertainties in its DET generation (Kloos and Peschke, 2006). However, Monte Carlo sampling can quickly become computationally expensive, and using the MCDET to perform bulk analyses on systems can be computationally prohibitive. Karanki et al. analyzes the need to balance computational resources and accuracy when considering uncertainties in a Discrete DET (DDET) framework (Karanki et al., 2017). However, MCDET more naturally and efficiently models applications that target specific uncertainty categories (Kloos and Peschke, 2006).
MCDET has been paired with the reactor dynamics code MELCOR, which is designed for examining various Level 2 PRA scenarios. Kloos and Peschke modeled a 1,300 MWe Konvoi type PWR in MELCOR and generated 50 DDETs (Discrete Dynamic Event Trees) using MCDET. The transient examined was characterized by a total loss of site power with external power being restored not earlier than 5,700 s after power loss. The 50 DDETs each provide a unique accident sequence with the end goal of producing probability distributions for all process quantities of interest (pressure in RPV, core exit temperature, etc.) throughout the sequence. All 50 DDETs were also combined to produce a mean probability distribution. This usage of MCDET showcased its ability to handle aleatory uncertainties well, but epistemic uncertainty analysis was not conducted in this case study (Kloos and Peschke, 2006).
RAVEN
The RAVEN DPRA tool was created by Idaho National Laboratory (INL) in 2012 with the intention of being highly modular and adaptable to a variety of simulation codes such as the new RELAP-7 thermo-hydraulic code. Both RAVEN and RELAP-7 are developed in the MOOSE framework (Gaston et al., 2009), which allows them to be easily coupled. Additionally, RAVEN’s flexible design allows easy integration to other simulation applications using Python or C++ languages. As the driver, RAVEN contains all the control logic and DET frameworks that are produced in the analysis, and uses the simulation code, e.g., RELAP-7, to determine the sequence of events for various accident scenarios. A system simulation is performed using RELAP-7, and upon reaching probability-based branching conditions, the code is instructed to generate new, parallel system simulations that represent some possible accident scenario progressions. Each parallel simulation is continued until the user-defined end state is reached, or until the probability of said parallel simulation is negligibly small to warrant deletion (called a ‘termination law’) (Alfonsi et al., 2013). RAVEN has also been successfully linked to the Modular Accident Analysis Program version 5 (MAAP5) to perform DET generation for nuclear power plants (Picoco et al., 2017). This code, owned and licensed by the Electric Power Research Institute (EPRI) conducts severe accident analysis of light and heavy water reactors.
INL describes the synergy between RAVEN’s modules including “Analysis of Dynamic Reactor Accident evolution” (ANDREA) to perform DET analysis. The visual overview of this DET framework within RAVEN is shown in Figure 2.
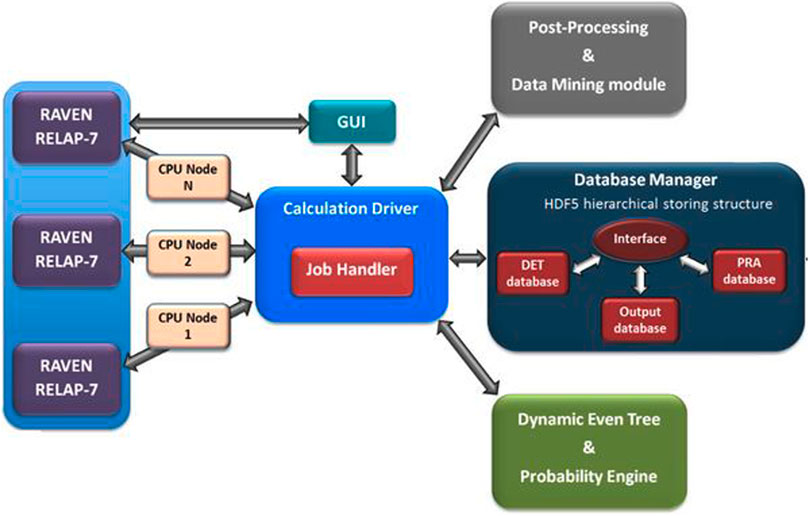
FIGURE 2. RAVEN’s framework for DPRA (Alfonsi et al., 2013).
RAVEN has been used in combination with RELAP-7 to conduct a realistic DPRA analysis of a simplified PWR model undergoing a station black out event (SBO) (Alfonsi et al., 2013). Cumulative Distribution Functions (CDFs) of diesel generator recovery time and clad failure temperature were sampled to provide branching triggers in the analysis. Two sets of DETs were produced that differ in their branching probability thresholds. One set of DETs uses Equally Spaced (on the CDF) Branching Probability (ESBP) thresholds, while the other uses probabilities that correspond to Equally Spaced Variable Values (ESVV) (Alfonsi et al., 2013). Thirty-seven DET branches with 18 complete histories were generated using ESBP and 31 DET branches with 15 complete histories were generated using ESVV (Alfonsi et al., 2013). The difference in the number of branches generated can be attributed to using the two distinct branching probability thresholds. Event sequences were considered complete when either the diesel generator power was restored (success) or the cladding temperature exceeded its design limits (failure).
The analysis illustrates various features of RAVEN that are valuable in conducting DPRA analyses, such as flexible branching condition sampling, ease of user-controlled process parameters of interest, as well as understandable and usable results. It is evident that RAVEN can be used in conjunction with an appropriate simulator to perform all three NRC defined levels of PRA analyses. New capabilities are continually being added to RAVEN, such as adaptive dynamic event tree generation and adaptive sampling (Alfonsi et al., 2013). These new capabilities aim to take advantage of the results from the analysis during an ongoing simulation, adapting the DET generation and sampling logic as the analysis unfolds.
ADAPT
ADAPT was created by Sandia National Laboratories and The Ohio State University to conduct DPRA analyses using industry standard simulation codes like MELCOR and RELAP. The.
ADAPT tool has been used extensively, and improved, by The Ohio State University, and by the developers at Sandia National Laboratories. ADAPT’s capabilities have been explored through various case studies, using example NPP analysis problems. ADAPT and RAVEN share many similarities in their functionality as DET drivers. Implemented in Python, ADAPT runs primarily on Linux based machines, and supports High Performance Computers (Jankovsky 2018 a.), which greatly assists in DET generation and management. To complete DPRA analyses, ADAPT is given control over a simulation code that calculates the results for all branched accident sequences. ADAPT then creates and stores DETs (sometimes referred to as Accident Progression Event Trees, APETs) based on all the simulations for further analysis using its various modules. As it stands, ADAPT has been linked extensively with the MELCOR analysis code to generate DETs/APETs and conduct Level 2 PRAs (Hakobyan et al., 2008). ADAPT has also been linked with the Modular Accident Analysis Program version 4 (MAAP4) to conduct a DET study (Rychkov and Kawahara, 2015). This code is also owned and licensed by EPRI.
Unique to ADAPT is the isolated method of sampling probabilistic parameters that play into the branching logic of DETs. While DET drivers like RAVEN use various known sampling methods, which includes user defined branching definitions, ADAPT relies only on user prescribed desired branching values and their associated probabilities. These probabilities can be specified directly, or as a collective from a CDF (Jankovsky 2018 a.). This provides the user additional flexibility in conducting specific analyses, allowing branching rules to be defined by the analyst, rather than being forced to choose from a predefined set of probabilistic sampling functions using a bank of parameter values.
ADAPT jobs are created by supplying the code various files that define a given experiment. These files contain all the information and input templates that ADAPT requires to control the simulator of choice as well as the logic used by ADAPT to generate DETs through branching. ADAPT has useful visualization tools as well. Figure 3 is an example ADAPT branching visualization that shows branches are completed, currently running, or in the queue.
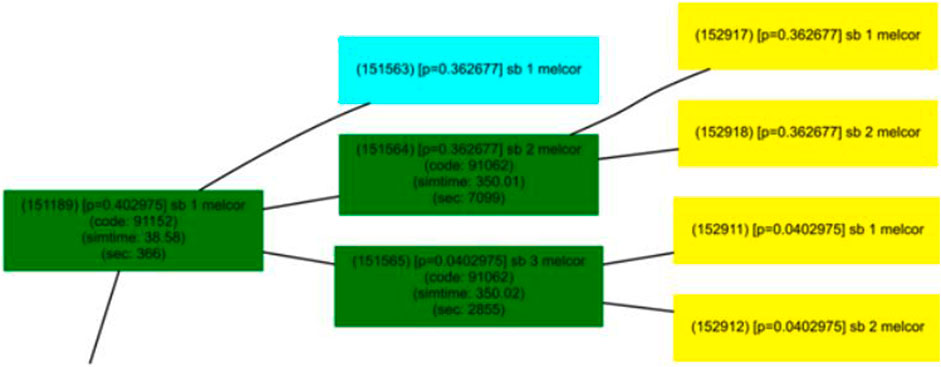
FIGURE 3. ADAPT Branch Visualization (Jankovsky 2018 a.).
Similar in nature to the other DET drivers, ADAPT has the proven ability to be coupled to a variety of simulators and used for DPRA analyses. One such example is the linkage demonstrated with the SAS4A/SASSYS-1 Sodium Cooled Fast Reactor simulation code, developed by Argonne National Laboratory (Fanning, 2012; 2017). For ADAPT to work properly as a DET driver, any code to which it is linked must have some basic capabilities that allow ADAPT adequate control to create branches and generate DETs. The simulator must take input in the form of an editable text file, have some ability to restart simulations already performed, and must have some user-controlled termination capability (Metzroth et al., 2009).
The SAS4A/SASSYS-1 code was modified and linked to ADAPT to demonstrate its DET generation and analysis capabilities. A relatively small DET was generated from various Transient Overpower (TOP) and Uncontrolled Transient Overpower (UTOP) initiating events (Jankovsky et al., 2018 b.). TOP/UTOP events are an important design basis event for SFRs as the operator and control system actions following these events can have major safety implications on the plant, sometimes leading to a reactivity insertion that is much larger than intended. The analysis resulted in 2,052 unique event sequences. These event sequences were then used to examine important physical parameters such as SFR reactivity coefficients. This analysis also involved the application of various Dynamic Important Measures (DYIs), which allow analysts to examine relationships between otherwise unconnected plant parameters (Jankovsky 2018 b.). For example, using DYIs, statements like, “overriding the RPS primary pump trip and thermal primary pump trips may lead to higher cladding survival” (Jankovsky et al., 2018 b.) can be made with confidence. Because ADAPT (or other DET drivers) leverages established, well validated simulation tools, conclusions reached through the DPRA analysis are less dependent on human judgement and execution.
EMRALD
The EMRALD (Event Modeling Risk Assessment using Linked Diagrams), also developed at INL, aims to retain the familiarity of the industry-standard SAPHIRE tool (seamless user interface, well understood PRA analysis process), while extending the capabilities of the code to include DPRA analyses (Prescott et al., 2018). This includes the ability to link EMRALD to reactor physics codes and provide high fidelity temporal information regarding the active system analysis. The plug and play nature of SAPHIRE is something not really present in the DET drivers described thus far, but is a desirable trait in software tools intended for wide adoption in the nuclear industry. EMRALD aims to retain this characteristic. The EMRALD code has been used in many instances as part of the Light Water Reactor Sustainability (LWRS) project, whose focus is to improve the existing nuclear reactor fleet through the development of technologies that focus on the economics, safety, and reliability of NPPs (Office of Nuclear Energy, n.d). It should be noted that EMRALD’s approach emphasizes synergy with existing logic tree methodology rather than generating DETs, for possibly a more straightforward adoption by risk practitioners.
As part of the LWRS project, an integrated external hazards analysis was conducted, specifically pertaining to seismic and flooding phenomena surrounding existing generic PWR sites. In this external hazards analysis, hundreds of seismic accident sequences were modeled using SAPHIRE (Parisi et al., 2017). Of the accident sequences modeled in SAPHIRE, four sequences of high importance were selected to be re-modeled using the DPRA methodology present in EMRALD. The four sequences had a high frequency of occurrence and included activation of various safety systems (Parisi et al., 2017). When conducting analysis of the EMRALD sequences, EMRALD was linked to two physics simulators, the NEUTRINO water behavior modeling tool, and the commonly used RELAP5-3D systems code. Within these analyses the final CDF resulting from seismic and flooding initiating events was calculated with both SAPHIRE and EMRALD, and the two are compared in Table 26 of the LWRS Program report (Parisi et al., 2017). More detailed failure information can be obtained with EMRALD as well. Component failures from the model, as well as the likelihood of a pipe break, were found for all pipe locations, and are included in Table 25 and 24 of the LWRS Program report, respectively (Parisi et al., 2017).
Figure 4 is a schematic conceptually illustrating this hazards analysis coupling using EMRALD, with a primary advantage of retaining traditional PRA (SAPHIRE) while gaining insights from detailed seismic and flooding analysis along with dynamic PRA methods.
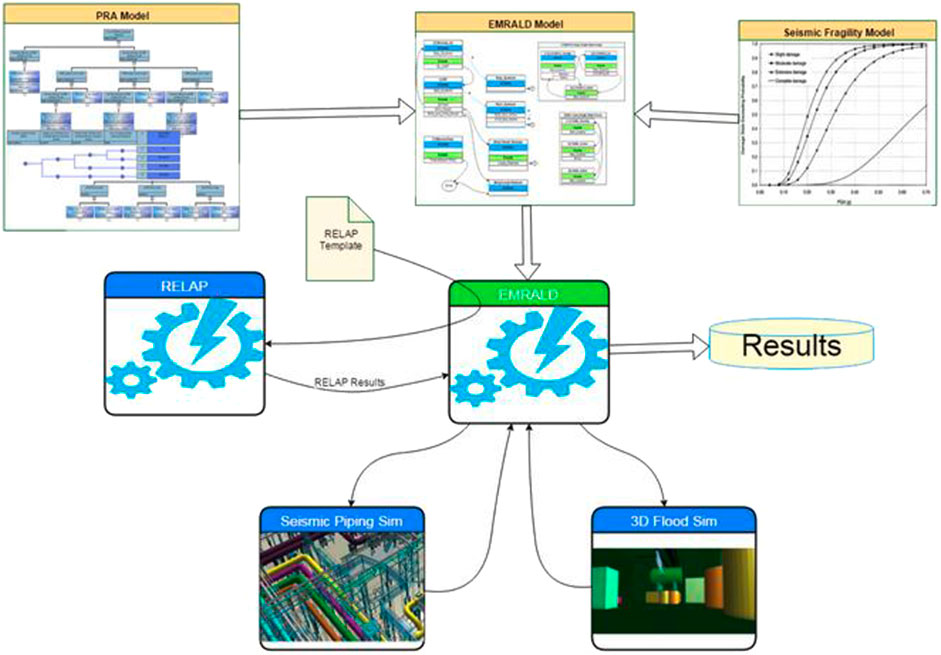
FIGURE 4. Integrated hazards analysis coupling in EMRALD (EMRALD, 2021).
The four DPRA tools summarized here reflect the variety of approaches that can be taken when conducting DPRA analyses, using dynamic event trees or otherwise (EMRALD). While DPRA approaches have yet to be broadly adopted by industry, the Nuclear Regulatory Commission (NRC) recently showed a renewed interest. NRC’s Office of Nuclear Regulatory Research, Division of Risk Analysis, recently presented the NRC’s “Dynamic PRA Study” at the 2021 Regulatory Information Conference (RIC) (Gonzalez, 2021). Additionally, in 2018 Oklo Inc. utilized DPRA as a pilot to the NRC’s Draft Regulatory Guide (DG)-1,353, “Guidance for a Technology-Inclusive Risk -Informed and Performance-Based Approach to Inform the Content of Applications for Licenses, Certifications, or Approvals for Non-Light Water Reactors.”
(ML19038A473) (Oklo, 2018). The future of DPRA in the U.S. nuclear industry has yet to be resolved, however, the availability of tools such as those discussed provide a promising path forward.
Needs Assessment for DPRA
An especially important attribute common to all four tools is the ability to tightly link reactor physics-based simulation codes to the DPRA analysis. Leveraging these reactor physics tools and using established codes to capture the detailed sequence of events over time for any given fault tree, provides the analyst results with a high degree of confidence. Still, these DPRA tools and methodologies have yet to be widely adopted by industry. Additional development is likely needed before the tools and approaches reach the desired level of technological maturity to be voluntarily adopted by the nuclear industry.
A common complication that DPRA analysts face is an overabundance of information produced during an analysis. Because DPRA tools were created to be more accurate, and more closely model realistic situations, the amount of information and event trees in any given simulation can become unmanageable, or very difficult to meaningfully interpret. While the analyst does have some control over this because they define the problem, any realistic DPRA analysis of a complex nuclear plant is going to be equally complex, involving big data. Taking ADAPT as an example, DETs produced throughout the analysis have the possibility of containing hundreds of thousands of branches (Jankovsky et al., 2018 b.). ADAPT is not unique in this attribute. In the EMRALD analysis conducted as part of the LWRS project, the EMRALD simulation effort required three workstations and approximately two million individual physics simulations (Prescott et al., 2018). This kind of information overload is a main drawback of current DPRA tools. Creators of these tools recognize this drawback. In ADAPT there exist modules whose sole purpose is to simplify DETs to produce more useful results (Jankovsky et al., 2018 b.). Also, in most DET drivers there exist termination laws that prune branches of the DETs being generated if those branches are determined to be too unrealistic, as defined by the analyst. Sifting through massive amounts of DETs is time consuming and solutions like in-tool simplification modules are extremely valuable if any useful analyses are to be conducted with these tools.
Another drawback, that may not be necessarily unique to the DPRA aspect of the nuclear industry, is the need for more computing power. A DET drivers software package initiates simulations of thousands of variations of a reactor system using a sophisticated physics code. The physics code requires a significant amount of computing power to perform just one detailed simulation; the amount of simulations required to capture any conceivable event sequence following some initiating event with enough fidelity to then calculate probability of occurrences, core damage frequencies, etc. may be unavailable, particularly in the nuclear industry. Within ADAPT there exists, by necessity, the ability to store branches in a “queue waiting for computing resources” (Jankovsky et al., 2018 b.). This issue is user dependent, and while research institutions (national labs or universities) often have sufficient computing resources, private industry must consider the computing resources required before adopting DPRA approaches.
Future Prospects
As Generation III and IV reactor designs come to fruition (Giorgio et at., 2013), there is a clear role for DPRA. They offer advantages that are in some ways uniquely suited for generation III + reactor designs. Passive safety systems are being incorporated into most new reactor designs in some form, and from a traditional PRA perspective, passive safety systems can be modeled, so long as the situations remain relatively simple. According to N. Siu, more complex situations involving passive safety systems, such as those with, “significant departure from design T-H conditions” would be more amenable to a dynamic PRA analysis (Siu, 2020). In contrast to traditional PRA that heavily relies on past operational data, DPRA methodologies can be applied when operational data is lacking, such as with new reactor types and passive safety systems. Assuming there are well validated computational tools available and relevant to the new reactor designs, capturing the dynamics present in these systems is much easier using a DPRA framework that leverages simulation codes during the analysis.
The human element of nuclear reactor design/safety is another feature that could be captured by DPRAs. In reactor accidents there exists the concept of Human Failure Events (HFEs). These are vital events that must be accounted for in the design of safety systems. Siu points out that the three most well-known reactor accidents, Chernobyl, Fukushima, and Three Mile Island, all suffered from “Errors of Commission,” which are HFEs (Siu, 2020). Regardless of why these events happen, having a probabilistic safety analysis framework that can account for these is highly valuable. Existing DPRA researchers are already incorporating this capability into their tools. One human behavior modeling tool is the Accident Dynamic Simulator Information, Decision, and Actions in a Crew (ADS-IDAC) DET driver. Specifically, the IDAC complex cognitive model addresses human interactions during emergency situations or accident mitigation situations (LaChance et al., 2012). Developed by the University of Maryland, IDAC is a rule-based methodology that attempts to probabilistically account for actions taken by an operating crew. IDAC is used within the ADS DET in an analogous fashion to a reactor system simulation code, as an attached module that increases the modeling capabilities of the DETs (LaChance et al., 2012).
Incorporating human behavior prediction capabilities into a DPRA analysis also paves the way for the accounting of adversary actions. Advanced reactor designs are continually leveraging digital information systems and digital control systems to streamline reactor design and control. These systems introduce unique security challenges that are difficult to quantify in a PRA context. The existence of modules like IDAC, that are designed to model operator behavior, make possible similar modules that allow the analyst to consider actions that could be taken by a remote adversary. In fact, there are existing tools, such as the Critical Infrastructure Cyberspace Analysis Tool (CICAT) that attempt to evaluate and model various cyber-attack scenarios (Wynn et al., 2020). If linked with a DET driver, tools like CICAT could expand the applicability of DPRAs to safety analyses for advanced reactor designs with digital infrastructure. As reactor designs evolve, so do their potential vulnerabilities, and so too must the safety analysis tools used to establish a strong safety case.
Summary
Dynamic PRA can provide insight for nuclear risk analysis by explicitly simulating the evolution of physical systems with time. DPRA maintains a similar methodology to traditional PRA, with fewer constraints that allows for assessment to consider scenarios such as equipment degradation, human performance, adversarial actions, or numerous mitigating actions that may vary over time. In particular, there is motivation to leverage DPRA when evaluating new or advanced reactor designs, or when there is limited operational data available. DPRA Tools highlights four existing DPRA tools that are currently available to perform these comprehensive, time-dependent risk assessments. While these, and other, DPRA tools are accessible, the enormity of data produced when conducting a thorough DPRA evaluation can still be a hindrance. As the availability of computational resources advances, it is likely that DPRA may be more attainable across the nuclear sectors with less access to high performance computing. Still, a strategy to uniformly balance between formulating a comprehensive risk profile and constraining the branching scenarios would be valuable to practitioners. While the promise of DPRA is appealing, it is also important to note the value of DPRA is contingent on having access to well validated reactor simulation that requires accurate data, physics models, and informed users.
Author Contributions
All authors listed have made a substantial, direct, and intellectual contribution to the work and approved it for publication.
Funding
Funding provided through Oregon State University and the U.S. Nuclear Regulatory Commission’s Research and Development Grant, Award Number 31310021M0005.
Conflict of Interest
The authors declare that the research was conducted in the absence of any commercial or financial relationships that could be construed as a potential conflict of interest.
Publisher’s Note
All claims expressed in this article are solely those of the authors and do not necessarily represent those of their affiliated organizations, or those of the publisher, the editors and the reviewers. Any product that may be evaluated in this article, or claim that may be made by its manufacturer, is not guaranteed or endorsed by the publisher.
References
Aldemir, T. (2013). A Survey of Dynamic Methodologies for Probabilistic Safety Assessment of Nuclear Power Plants. Ann. Nucl. Energ. 52, 113–124. doi:10.1016/j.anucene.2012.08.001
Alfonsi, A., Rabiti, C., Mandelli, D., Cogliati, J., Kinoshita, R., and Naviglio, A. (2013). Dynamic Event Tree Analysis Through RAVEN. Idaho Falls, ID: Idaho National Laboratory. INL/CON-13-29344.
Chraibi, H. (2018). Getting Started with PyCATCHOO. Available at: http://pycatshoo.org/Getting%20started%20with%20PyCATSHOO-PYCV1228.pdf.
Electric Power Research Institute (EPRI) (2012). The EPRIA HRA Calculator. Available at: https://primepra.com/wp/wp-content/uploads/HRA%20User%20Group%20Brochure.pdf.
EMRALD (2021). Idaho National Laboratory. Available at: https://emrald.inl.gov/SitePages/Application.aspx.
Fanning, T., Brunett, A., and Sumner, T. (2017). The SAS4A/SASSYS-1 Safety Analysis Code System. Argonne, IL: Argonne National Laboratory. ANL/NE.
Fanning, T. (2012). The SAS4A/SASSYS-1 Safety Analysis Code System. Argonne, IL: Argonne National Laboratory. ANL/NE-12/4.
Gaston, D., Hansen, G., Kadioglu, S., Knoll, D. A., Newman, C., Park, H., et al. (2009). Parallel Multiphysics Algorithms and Software for Computational Nuclear Engineering. J. Phys. Conf. Ser. 180 (1), 012012. doi:10.1088/1742-6596/180/1/012012
Giorgio, L., Mauro, M., and Nicola, T. (2013). Generation IV Nuclear Reactors: Current Status and Future Prospects. Energy Policy 61, 1503–1520. doi:10.1016/j.enpol.2013.06.101
Gonzalez, M. Dynamic PRA Study (2021). Regulatory Information Conference, U.S. NRC. Available at: https://ric.nrc.gov/docs/abstracts/gonzalezm-m5-hv.pdf.
Hakobyan, A., Denning, R., Aldemir, T., Dunagan, S., and Kunsman, D. (2008). A Methodology for Generating Dynamic Accident Progression Event Trees for Level 2 PRA. SAND2008-4746. New Mexico: Sandia National Laboratories Albuquerque.
Jankovsky, Z. (2018). Development of Computational and Data Processing Tools for ADAPT to Assist Dynamic Probabilistic Risk Assessment. [Electronic Dissertation]. Columbus, OH: Ohio State University. OhioLINK Electronic Theses and Dissertations Center.
Jankovsky, Z., Haskin, T., and Denman, M. (2018). How to ADAPT. Albuquerque, NM: Sandia National Laboratory. SAND2018-6660. [Online]. Available at: https://www.sandia.gov/adapt/_assets/documents/document.pdf.
Karanki, D. R., Rahman, S., Dang, V. N., and Zerkak, O. (2017). Epistemic and Aleatory Uncertainties in Integrated Deterministic and Probabilistic Safety Assessment: Tradeoff Between Accuracy and Accident Simulations. Reliability Eng. Syst. Saf. 162, 91–102. doi:10.1016/j.ress.2017.01.015
Kloos, M., and Peschke, J. (2006). MCDET: A Probabilistic Dynamics Method Combining Monte Carlo Simulation with the Discrete Dynamic Event Tree Approach. Nucl. Sci. Eng. 153 (2), 137–156. doi:10.13182/NSE06-A2601
LaChance, J., Cardoni, J., Li, Y., Mosleh, A., Aird, D., Helton, D., et al. (2012). Discrete Dynamic Probabilistic Risk Assessment Model Development and Application. Albuquerque, NM: Sandia. Available at: http://pbadupws.nrc.gov/docs/ML1230/ML12305A351.pdfMartin
Lee, S. w., Baek, S. j., Heo, G., young, K., wan, T., and Kim, J. h. (2018). “Development of DICE (Dynamic Integrated Consequence Evaluation) for Procedure Coverability Studies: Conceptual Design Phase,” in Proceedings of the KNS 2018 Fall Meeting (Korea: Republic of: KNS).
Locatelli, G., Mancini, M., and Todeschini, N. (2013). Generation IV Nuclear Reactors: Current Status and Future Prospects. Energy Policy. 61, 1503–1520. doi:10.1016/j.enpol.2013.06.101
Martin, N., Denman, M. R., and Wheeler, T. A. (2016). Pruning of Discrete Dynamic Event Trees Using Density Peaks and Dynamic Time Warping. Trans. Am. Nucl. Soc. 115, 783–786.
Metzroth, K., Winningham, R., Catalyurek, U., Denning, R., and Aldemir, T. (2009). Linking of the RELAP5-3D Thermal Hydraulic Code with the ADAPT PRA Tool. Trans. Am. Nucl. Soc. 100, 448.
Miller, A., Hess, S., and Smith, C. (2020). R&D Roadmap to Enhance Industry Legacy ProbabilisticRisk Assessment Methods and Tools. Idaho Falls, ID: Idaho National Laboratory INL/EXT-20-59202. [Online]. Available at: https://lwrs.inl.gov/RiskInformed%20Safety%20Margin%20Characterization/PRA_Legacy_Tools_and%20Methods.pdf
Murphy, J. A. (1984). Probabilistic Risk Assessment (PRA) Reference Document. United States: N: 1984 Final report. p., Web. NUREG-1050.
Nuclear Energy Institute (NEI) (2006). Risk-Informed Technical Specifications Initiative 4b, Risk- Managed Technical Specifications (RMTS) Guidelines – Industry Guidance Document NEI Report 06-09. Washington, D.C: Revision 0.
Office of Nuclear Energy (n.d). Light Water Reactor Sustainability (LWRS) Program. Energy.Gov. Available at: https://www.energy.gov/ne/nuclear-reactor-technologies/light-water-reactor-sustainability-lwrs-program.
Oklo (2018). DG-1353 Pilot. Report Oklo-2018-RlO-P, Rev. 0 https://www.nrc.gov/docs/ML1903/ML19038A473.pdf.
Osborn, D., Cohn, B., Jordan Parks, M., Ryan, K., Ross, K., Faucett, C., et al. (2019). Modeling for Existing Nuclear Power Plant Security Regime. SAND2019- 12015Available at: https://lwrs.inl.gov/Physical%20Security/Modeling_for_Existing_NPP_Security_Regime.pdf.
Parisi, C., Prescott, S., Ma, Z., Spears, B., Szilard, R., Coleman, J., et al. (2017). Risk-Informed External Hazards Analysis for Seismic and Flooding Phenomena for a Generic PWR. United States: Web.
Picoco, C., Aldemir, T., Rychkov, V., Alfonsi, A., Mandelli, D., and Rabiti, C. (2017). “Coupling of RAVEN and MAAP5 for the Dynamic Event Tree Analysis of Nuclear Power Plants,” in proceedings of European Safety and Reliability Conference - ESREL Portoroz, Slovenia. doi:10.1201/9781315210469-356
Prescott, S., Smith, C., and Vang, L. (2018). EMRALD, Dynamic PRA for the Traditional Modeler. Idaho Falls, USA: Idaho National Laboratory Probabilistic Safety Assessment and Management PSAM.
Queral, C., Gómez-Magán, J., París, C., Rivas-Lewicky, J., Sánchez-Perea, M., Gil, J., et al. (2018). Dynamic Event Trees Without Success Criteria for Full Spectrum LOCA Sequences Applying the Integrated Safety Assessment (ISA) Methodology. Reliability Eng. Syst. Saf. 171, 152–168. doi:10.1016/j.ress.2017.11.004
Rasmussen, N. C. (1975). Reactor Safety Study. An Assessment of Accident Risks in U. S. Commercial Nuclear Power Plants. Executive Summary. WASH-1400 (NUREG-75/014). Rockville, MD, USA: Federal Government of the United States, U.S. Nuclear Regulatory Commission.
Rychkov, V., and Kawahara, K. (2015). ADAPT-MAAP4 Coupling for a Dynamic Event Tree Study, PSA 2015 International Topical Meeting on Probabilistic Safety Assessment and Analysis, Sun Valley. USA: ID.
Siu, N. (2020). Dynamic PRA for Nuclear Power Plants: Not if But When? Nuclear Regulatory Commission. Rockland, MD: Technical Opinion Paper. [Online]. Available at: https://www.nrc.gov/docs/ML1906/ML19066A390.pdf.
United States Nuclear Regulatory Commission (1990). Severe Accident Risks: An Assessment for Five U.S. Nuclear Power Plants. Washington, DC. NUREG-1150. [Online]. Available at: https://www.nrc.gov/reading-rm/doc-collections/nuregs/staff/sr1150/v1/index.html.
United States Nuclear Regulatory Commission (2016). Probabilistic Risk Assessment and Regulatory Decision Making: Some Frequently Asked Questions. Rockland, MD: NRC.gov. Available at: https://www.nrc.gov/docs/ML1624/ML16245A032.pdf.
United States Nuclear Regulatory Commission (2020a). History of the NRC's Risk-Informed Regulatory Programs. Rockland, MD: nrc.gov. Available at: https://www.nrc.gov/about-nrc/regulatory/risk-informed/history.html.
United States Nuclear Regulatory Commission (2020b). Probabilistic Risk Assessment (PRA). Rockland, MD: NRC.gov. Available at: https://www.nrc.gov/about-nrc/regulatory/risk-informed/pra.html.
Wynn, J. E., Whitmore, J., Coconato, W. F., and McCracken, S. (2020). Critical Infrastructure Cyberspace Analysis Tool (CICAT) Capability Description. Bedford, MA: The MITRE Corporation. Available at: https://www.mitre.org/publications/technical-papers/critical-infrastructure-cyberspace-analysis-tool-cicat-capability
Yadav, V., Agarwal, V., Gribok, A. V., and Smith, C. L. (2017). Dynamic PRA with Component Aging and Degradation Modeled Utilizing Plant Risk Monitoring Data. United States: N. p. Web. Available at: https://inldigitallibrary.inl.gov/sites/sti/sti/Sort_1827.pdf.
Nomenclature
ADAPT Analysis of Dynamic Accident Progression Trees
ADS-IDAC Accident Dynamic Simulator-Information Decisions and Action in a Crew
AEC Atomic Energy Commission
CDF Cumulative Distribution Function Core Damage Frequency
CDF Cumulative Distribution Function Core Damage Frequency
CICAT Critical Infrastructure Cyberspace Analysis Tool
DET Dynamic Event Tree
DPRA Dynamic Probabilistic Risk Assessment
EMRALD Event Modeling Risk Assessment using Linked Diagrams
EPRI Electric Power Research Institute
ESBP Equally Spaced Branching Probability
ESVV Equally Spaced Variable Values
ET Event Tree
FT Fault Tree
GUI Graphical User Interface
HFE Human Fact
IE Initiating Event
LWRS Light Water Reactor Sustainability
MAAP4/5 Modular Accident Analysis Program version 4/5
MCDET Monte Carlo Dynamic Event Trees
MELCOR Methods for Estimation of Leakages and Consequences of Releases
RAVEN Risk Analysis and Virtual Environment
RELAP Reactor Excursion and Leak Analysis Program
SAPHIRE Systems Analysis Program for Hands-on Integrated Reliability Evaluations
SBO Station Black Out
TE Top Event
Keywords: probabilistic risk assessment, dynamic PRA, nuclear energy, generation III+, dynamic models
Citation: Wiltbank NE and Palmer CJ (2021) Dynamic PRA Prospects for the Nuclear Industry. Front. Energy Res. 9:750453. doi: 10.3389/fenrg.2021.750453
Received: 30 July 2021; Accepted: 27 October 2021;
Published: 11 November 2021.
Edited by:
Muhammad Zubair, University of Sharjah, United Arab EmiratesReviewed by:
Khalil Ur Rahman, Pakistan Nuclear Regulatory Authority, PakistanClaudia Picoco, Electricité de France, France
Copyright © 2021 Wiltbank and Palmer. This is an open-access article distributed under the terms of the Creative Commons Attribution License (CC BY). The use, distribution or reproduction in other forums is permitted, provided the original author(s) and the copyright owner(s) are credited and that the original publication in this journal is cited, in accordance with accepted academic practice. No use, distribution or reproduction is permitted which does not comply with these terms.
*Correspondence: Nathan E. Wiltbank, wiltbann@oregonstate.edu