- 1School of Electrical Engineering, Southeast University, Nanjing, China
- 2Guodian Nanjing Automation Co., Ltd., Nanjing, China
Large-scale distributed demand response is a hotspot in the development of power systems, which is of much significance in accelerating the consumption of new energy power generation and the process of clean energy substitution. However, the rigorous distributed algorithms utilized in current research studies are mostly very complicated for the large-scale demand response, requiring high quality of information systems. Considering the electrical features of power systems, a weak-consistency–oriented collaborative strategy is proposed for the practical implementation of the large-scale distributed demand response in this study. First, the basic conditions and objectives of demand response are explored from the view of system operators, and the challenges of large-scale demand response are discussed and furthermore modelled with a simplification based on the power system characteristics, including uncertainties and fluctuations. Then, a weakly consistent distributed strategy for demand response is proposed based on the Paxos distributed algorithm, where the information transmission is redesigned based on the electrical features to achieve better error tolerance. Using case studies with different information transmission error rates and other conditions, the proposed strategy is demonstrated to be an effective solution for the large-scale distributed demand response implementation, with a robust response capability under even remarkable transmission errors. By integrating the proposed strategy, the requirement for the large-scale distributed systems, especially the information systems, is highly eased, leading to the acceleration of the practical demand response implementation.
Introduction
Energy transition has become a key issue for sustainable economic and social development. The world urgently needs to transform energy production, allocation, and consumption methods today, so evolutions are emerged in both generation and consumption sides (Heffron et al., 2020). Developing and utilizing clean energy instead of the traditional fossil fuel are welcomed in the power production side, while much attention should also be paid to the intelligent interaction of power loads in the consumption side (Hoggett, 2014). Wind power, photovoltaic power generation, and other intermittent new energy sources and new controllable loads have been integrated to the grid on a large scale, leading to the new features of power system operations (Kolhe, 2012).
In spite of the electricity contribution, the high penetration of renewable generations brings some challenges. On the one hand, the uncertainties of the renewable power generation will make the power output and electricity flow in the grid fluctuate more seriously (Dvorkin et al., 2015), which would influence the safe and stable operation of the system. On the other hand, the proportion of conventional power generation is decreasing, which will significantly weaken the dynamic voltage and power regulation capability of the grid (Zheng et al., 2015). Based on these problems caused on the generation side, the consumption side is highly expected to help with the situation. Hence, demand response (DR) emerges. DR emphasizes the direct interaction between the power users and systems via the electricity market or other patterns featuring faster response action, lower cost, better environmental protection, and high potential to increase the system’s flexibility (Huang et al., 2019), which is desired by users and utilities, resulting in a fast development in recent years (Ribó-Pérez et al., 2021).
There has been a lot of research on the utilization of demand-side resources. Among the loads that can participate in demand response, the resident load has high potential. A resident-side demand response energy management model can be established to analyze and evaluate the flexibility from the demand side and to optimize the entire response system (D’hulst et al., 2015). Considering real-time electricity prices, a kind of demand-side energy management controller based on dynamic pricing for residential power usage can be used to reasonably dispatch electrical equipment to maximize user satisfaction and benefit (Chen et al., 2021). The air conditioning load as a kind of thermostatically controlled load, which would account for more than half of the total electricity demand in summer, can become the main control resource in the DR process (Mahdavi and Braslavsky, 2020), realizing an adaptive control mode according to the index of ambient temperature and human comfort (Perez et al., 2016). Considering the small capacity of scattered air conditioning loads, the practical demand response usually requires the participation of quantities of residents through aggregation technology to achieve efficient utilization (Gao et al., 2017), leading to a large-scale problem.
The coordination strategy is extremely important for the large-scale systems, which is also the research hotspot for demand response. Currently, there are three majorly investigated strategies, i.e., centralized control, decentralized control, and distributed control. Through the centralized control, the power consumption of loads is controlled directly using a utility or by a system operator, which features fast response speed and concise control volume (Chen and Liu, 2017). However, this control method depends largely on the information transmitted between the operator and the loads, which issues a higher requirement on the accuracy of information transmission (Tang et al., 2018). It depends on an information platform based on the coupled cyber-physical power system to achieve the coordination between the operator and the user, which requires particularly solid robustness to secure information transmission process (Qi et al., 2017). Although with desired performance, the centralized control requires a process center and a complete information system, which is not economic at all. Hence, the decentralized control combines local control strategy and centralized display, which can avoid the problem of information delay and the high cost to build a strictly robust information transmission system (Bahrami et al., 2018). Although many methods and theories have been proposed to improve the time-delay systems, it is found difficult to keep the balance between small delay and good control precision (Zhu and Fridman, 2020). As can be seen, the response performance is comparatively poor with that of decentralized control strategy, while the over-response and under-response cannot be avoided. For the sake of combining the advantages of the abovementioned control strategies, the distributed control is proposed, which is based on centralized management and distributed control (Burns, 2018). It has been widely used in the IoT industry for its interoperability, scalability, and dependability, which are the key features of many distributed systems (Iwanicki, 2018). Different algorithms and control strategies are widely studied and adopted to improve the efficiency of the distributed control (Xiao et al., 2019) and its natural features of error tolerance (Raynal, 2018). Specific algorithms have been used, including clock synchronization theories (Dalwadi and Padole, 2017) and self-stabilization algorithm (Altisen et al., 2019), to ensure the information consistency and control stability, which are very precise and complicated to realize in some real scenarios as for controllable power loads. Therefore, the distributed control strategy is prominent in large-scale collaborations at the current stage. Meanwhile, the existing demand response process has certain challenges, where the reliability of the physical information system is highlighted (Ming et al., 2018). In the reviewed demand response system, the coordinated and stable status of the information and physical system is the basis of the reliable implementation of demand response (Huang et al., 2019). However, practically speaking, based on the power system features, the power system–demand response does not need these tight conditions, such as the rigorous control algorithm or the extremely reliable information systems. First, the demand response objective value can be flexible around the target since the system state is time-varying. Second, the response individuals do not need the accurate system information all the time, while the focus of the system is the final objective and their focus is their own behaviors. These characteristics provide a potential way to simplify the large-scale distributed demand response implementation.
Based on abovementioned observations, a weak-consistency–oriented distributed control algorithm is proposed in this study to tackle the large-scale demand response problem in a practical way. At the first stage, the air conditioning model and demand response structure are presented and the severe impacts of information errors are extensively discussed. Then, by revising the transmitted information format, the consistency requirement is slacked, resulting in a weak-consistency distributed algorithm. By implementing the proposed algorithm, the demand response performance of large-scale systems is highly improved, especially with a high rate of information errors. The contributions of this study are as follows. First, a weak-consistency–oriented distributed algorithm is proposed based on the features of demand-side resources and the structure of DR in power systems. Then, a specific DR strategy is proposed and proved valid to realize the demand response under the control structure, which is of much robustness especially to solve the problem of errors in the information transmission process, improving the performance of large-scale systems.
Air Conditioning–Based Demand Response Formulation
Model of Air Conditioners for Demand Response
The air conditioning load is a kind of TCL. The modeling of the air conditioning load consists of building a thermodynamic model and the air conditioning unit electromechanical transformation model (Shen et al., 2019).
Thermodynamic Model of Buildings
The thermodynamic model of a house usually adopts an equivalent thermal parameter model. In order to simplify the calculation, only the change process of the indoor temperature is considered (Lu, 2012). In this study, the first-order equivalent thermal parameter model is used, as shown in Figure 1.
The model equation is shown as follows:
where
Assuming that the outdoor temperature
Electromechanical Transformation Model of Air Conditioners
The electromechanical transformation model of air conditioners describes the relationship between the air conditioning power and the cooling (heat) quantity, that is, the energy efficiency ratio. The energy efficiency ratio of fixed-frequency air conditioners is a constant value, while the energy efficiency ratio of inverter air conditioners is related to the working frequency.
The fixed-frequency air conditioner adopts a fixed-speed compressor to control ON and OFF states according to the temperature
The compressor start–stop state is represented by S. S is denoted by 0 to indicate that the compressor is in a stopped state, and S is denoted by 1 to indicate that the compressor is in a constant-speed operation state. The relationship between the indicator S and the indoor temperature can be expressed as
Therefore, the relationship between the indicator S and the electric power of the air conditioner,
where
The inverter air conditioner uses a frequency converter to drive the compressor and continuously controls the cooling capacity by adjusting the working frequency and changing the compressor speed (Zhou et al., 2015). As shown in Figure 3, the frequency setting is related to the difference between the actual indoor temperature and the set temperature, which can be expressed by
where
Structure of Demand Response
The research in this work is based on a hierarchical distributed control architecture based on load aggregators. As shown in Figure 4, the entire control system is divided into grid dispatch layer, load aggregator layer, and user layer. Continuous information exchange between the layers realizes overall load control (Samad et al., 2016).
On the one hand, the load aggregator layer needs to receive power control instructions from the grid dispatch center, as well as integrate and analyze load types, operating status, adjustable capacity, whether or not it can participate in DR, and other information of the scattered flexible load within its jurisdiction, in order to evaluate the response potential and reaction time which will be fed back to the dispatch center. On the other hand, the load aggregator layer allocates the power adjustment tasks issued by the power grid to users in the area and receives information feedback after participating in the response process. In such a multilevel control structure, the information transmitted can be divided into information control signals, power reduction signals, control decision signals, price signals, and so on.
Impacts of Information Errors
It can be seen that if there are errors in the information transmission between different layers, the results of regional demand response will be highly influenced. For example, there are 2500 air conditioner users in a certain area who can participate in demand response. The ratio of fixed-frequency air conditioners to inverter air conditioners is 1:1, and their rated maximum operating power is 1500 and 2100 W, respectively. The total response task issued by the load aggregator is 500 kW. When there are errors in information transmission between users, the overall response effect will be considerably affected. The result is shown in Supplementary Table S1 and Figure 5.
This study defined an index of response rate (%) in order to describe the effect of demand response process. If the response rate is more than 90%, the demand response process can be anticipated as a successful one. It can be calculated by the following function, where
It can be seen from Supplementary Table S1 and Figure 5 that when the information transmission process is correct, the response result met the response task well; when the information error rate is increasing, the number of users who can normally participate in the response is obviously reduced and the response rate index drops to 27%. Meanwhile, as shown in Figure 6, operation results under 1% information error rate fluctuated in a large range and the average response volume of 50 rounds of operation has quite a large gap to the demand.
The purpose of this study is to optimize the response rate index, combined with the distributed theory and Paxos algorithm, proposing a weakly consistent distributed strategy for demand response, which can effectively avoid the corresponding consequences caused by the problem of information error in the process of demand response, by which the demand response process could achieve a better effect.
A Weak-Consistency–Oriented Distributed Algorithm
Paxos Algorithm
In a distributed computing environment, solving the problems of data synchronization between nodes and distributed resource competition and autonomous recovery of single-node failures is the basic guarantee for the correctness and reliability of the system (Shi and Li, 2012). Paxos algorithm is a consensus algorithm based on message passing and high fault tolerance (Lamport, 2006; Rao et al., 2011). In the algorithm, different nodes are represented by three roles: Proposer, Accepter, and Learner (Marzullo et al., 2011). Among them, the Proposer submits a proposal to the Accepter and the proposal contains a resolution; the Accepter approves the proposal; and the Learner obtains and knows the resolution contained in the approved proposal. A node plays multiple roles. The consistency problem of the distributed theory can be simply described as follows: in a distributed system, each node as a participant can propose a plan and the consensus algorithm ensures that only one of these proposals can be selected, while other nodes should also be aware of the proposal which is selected. As a result, all participants will reach only one agreement.
According to the abovementioned Paxos algorithm, the process of air conditioning load participating in demand response can be illustrated. It is assumed that the load aggregator issues power adjustment instructions to users within a certain range, and five users A, B, C, D, and E can participate in the demand response. To simplify the analysis, the order of participation priority of these five users is arranged in an alphabetical order. The response process is shown in Figure 7. The load aggregator releases a power shortage task to each user as 100, and the load capacities that users A, B, C, D, and E can respond to are 20, 30, 30, 40, and 50, respectively. The specific process is as shown in Figure 7.
The rectangle box in Figure 7 represents the information sent by a certain user after participating in the demand response and the round box represents the information received by a certain user. The number format is “response amount; remaining demand amount.” Before user E responds, the remaining demand received is 0, and hence, there is no need for user E to participate in this demand response.
Weak-Consistency Control Strategy
The weak-consistency control strategy is carried out on the basis of ensuring that the information sent or received by each user is correct. However, in the actual response process, there is a certain bias in information transmission between users. This study believes that demand response objective value can be flexible around the target since the system state is time-varying and the inconsistent state of data in the process will not affect the overall availability and the response performance of the system. There is no need for a strong data consistency, so a weak-consistency distributed strategy and a new kind of information format for demand response are proposed as follows.
First, the responding users could be considered as the nodes in the Paxos distributed algorithm. Then, in the process, the responding user, the Proposer in Paxos, transmits two sets of information to the system pool instead of one, including the load response information of the current user and of the previous one, which is received before the response takes place. The next responding user, the Accepter, could check the two sets of information received and correct errors in the information transmission process, in order to confirm whether the proposal has passed and decide whether to proceed with the next response action. The response process after adopting this strategy is shown in Figure 8. If the information is transmitted correctly, the response is normal.
If information errors exist in the process, the errors could be corrected in some occasions. It is assumed that there is no error in the information transmission between the first two users who participated in the response to ensure that the subsequent response process can proceed normally; otherwise, there will be surplus errors in the subsequent process and the demand response cannot be performed normally.
As shown in Figure 9, there are four possible kinds of information errors in the response process:
1) A Learner, user A for example, has errors in the information received after other’s response, which can be corrected based on the two sets of data received before and after.
2) An Accepter receives incorrect information after the former participant responded. It can only be judged and rectified by previous response information, the current response user’s adjustable capacity, and other conditions. There can be three possible situations:
a) The error occurs in the response information of the previous participant, which can be rectified by the information previously received. In Figure 9, “10; 90” in red can be corrected to “20; 80.”
b) The error occurs in the response information of the current participant, but it can be judged but cannot be corrected only, depending on the previous information and the capacity index. Hence, in order to avoid errors in the subsequent response process, the next participant, user C in Figure 9 for example, should give up participation and send the same response signal as B to the system pool.
c) The error occurs in the response information of the current participant, which cannot even be detected because it coincides with the capacity and other information. It may finally cause some over- or under-response problems, which is 10 in the implementation, and its proportion in the whole demand would be smaller if more users participate in the process. So, this kind of error could be ignored.
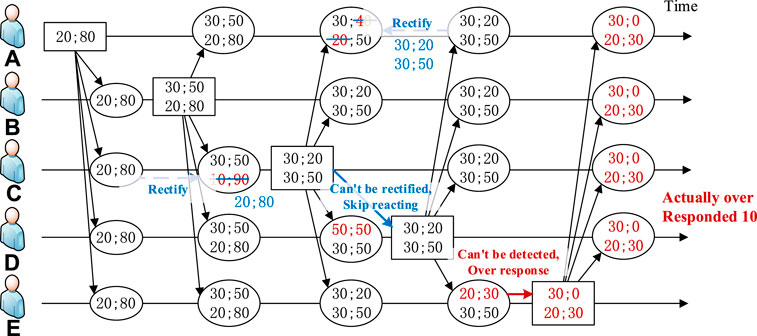
FIGURE 9. Implementation using the weak-consistency strategy when the information is incorrectly transmitted.
Method Flow
After analyzing different possible information errors, a control strategy which can effectively solve the DR problem can be proposed, which is shown in Figure 10.
1) Each user sends two sets of load information to the system pool when participating in demand response: the information of the current responding user and of the previous user.
2) It should be ensured that there is no error in the information transmission between the first two users, so that the process can continue correctly
3) Starting from the second user, it is necessary to verify each time whether the information received is correct. If correct, the information is updated according to the received information and passed on to other users. If not, when the error takes place in the previous response information, it should be corrected based on the historical information and the process could be continued. However, when the error takes place in the current response information, the user should skip the current response period without conducting a response action and the process could start directly from the next user to continue responding.
4) After the current user information is updated, the demand remaining needs to be judged to determine whether the response process is over.
5) For the information received by all the learners, it can be verified based on the information received before and after and the wrong information should be corrected as much as possible without affecting the final response result.
Case Studies
This section uses multiple cases to explore the probability distribution of the response degree when the response strategy proposed in this study is fully adopted, or not. It is assumed that the demand response process takes place on summer days of 34°C outside and the rated maximum operating power of the fixed-frequency air conditioners and the inverter air conditioners is, respectively, 1500 and 2100 W. The simulation is based on the MATLAB 2020 platform, and the computer is configured as Intel(R) Core (TM) i5-8400 2.80 GHz with 8 Gb memory.
Response Capability Analysis
When ratio of fixed-frequency and inverter air conditioners is 1:1 and the information error rate is 0, different numbers of users could meet the demand of different power adjustment tasks. Under the same responding conditions, more participants or smaller demand volume would lead to higher success rate of demand response. That is to say, the total response volume is restricted by the whole capacity of the cluster, and if the task volume is within the capacity, the response would be close to success. The maximum response load that the air conditioning load cluster can afford is positively proportional to the number of users. The actual response volume when the information error rate is 0 can be approximately considered as the maximum response capacity of the whole cluster, which is shown in Table 1 and Figure 11.
With the development of technology in air conditioners, additional inverter air conditioners are used, replacing fixed-frequency ones, because of their convenience, low cost, and easy maintenance. In Table 1 and Figure 11, the comparison between different ratios of fixed-frequency and inverter air conditioners participating in demand response is shown, which illustrates that the ratio of air conditioner types could influence response capacity. Under different numbers of users and information error rates, the response capacity also changes by the change in type ratio, which shows a peak when the ratio is about 1:2. The next three groups of results are listed in Table 2 to analyze the reason behind this.
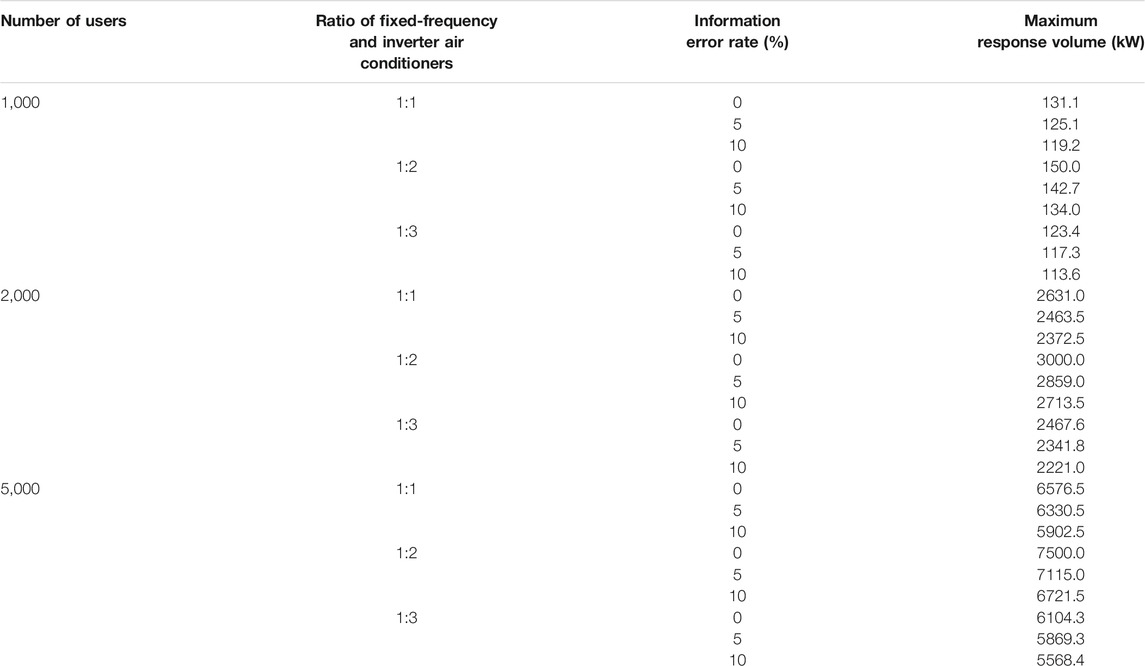
TABLE 2. Maximum response volume of different numbers of users with different type ratios and different information error rates.
The two types of air conditioners taking part in the simulation are of different rated power outputs. As shown in Table 2, no matter what the information error rate is, the response result of 1:2 ratio is the best correspondingly. The rated maximum operating power of the fixed-frequency air conditioner and the inverter air conditioner, is respectively, 1500 and 2100 W, while the former one works with a fixed-speed compressor and the ON–OFF strategy and the working power of the latter one is related to ΔT, the difference between the actual indoor temperature and the temperature set by the user. That is to say, the actual power of fixed-frequency air conditioners which can take part in the response is constantly 1500W, having the ability to provide more response capacity with less number of air conditioners. Also, the actual power of inverter air conditioners changes between 755W and 2100 W according to ΔT, which is randomly emerged in the process to simulate the real working situation. It is of better flexibility to provide power when the inverter ones are comparatively more. However, when the ratio comes to 1:3, the decrease in the number of fixed-frequency ones will lead to a drop in the overall response capacity.
Discussion on Demand Response Implementation
In this section, several cases are set in Table 3 in order to discuss the difference in the performance of demand response with or without the strategy proposed and the performance under different information error rates of the strategy proposed in order to test and prove the robustness of it. The information error rate is set from 0 to 0.5%. The results are listed in Tables 4, 5 for comparison.
As shown in Tables 4, 5, and Figure 12, Figure 13, if the information error rate increases, the number of users who cannot take part in the response will increase correspondingly. With the strategy, when the response demand is comparatively small, the information error will not affect the final response result and the system response requirements can still be reached. Although there are data inconsistencies caused by information transmission errors in the response process, this intermediate state does not affect the availability and final consistency of the overall system. It illustrates the fault tolerance characteristic of the distributed system. Through the comparison among the corresponding rows of Table 4 and Table 5, a significant difference occurred in the response situation between adopting the demand response strategy proposed in this study or not, which could convincingly illustrate that the strategy is of high validity and good fault tolerance feature.
In order to test the robustness of the system with the strategy proposed, we set different information error rates to the response process to observe the consistency of the response result with the method of repeated trials.
As shown in Figure 14, where information error rate is set to 5 and 10% under Case 1, if we perform 50 rounds of response process, it will illustrate that when adopting the strategy, the response rate can mostly reach the requirement of 90% under information error rates even up to 10%. The response rate of 50 rounds is listed in Supplementary Table S2. However, without the strategy, when the security and correctness of information transmission are ignored in the demand response process, the response rate will be extremely poor, which drops to 39% in average when the information error rate is only 0.1%. In addition, when performing 50 rounds of response process, the response amount and response rate vary greatly, but there is no obvious regulation, as shown in Figure 15 and Supplementary Table S3. The phenomenon, on the one hand, can illustrate the significant influence of information transmission error on the response result and, on the other hand, prove the necessity to adopt a strategy to prevent or avoid the problem. The strategy proposed can effectively improve fault tolerance ability and stabilize the result when the errors exist.
Conclusion
This study considers the possible errors in information transmission when large-scale loads participate in demand response. Based on the information format modification and the Paxos distributed algorithm, a weakly consistent demand response strategy considering information collation and correction is proposed, targeting the practical DR implementation with robustness. Through the analysis of specific demand response cases of residential air conditioning loads under different conditions, the proposed strategy is demonstrated to be effective in information self-inspection and furthermore the robust demand response implementation. Specifically, the proposed approach is especially applicable to the large-scale systems, while the error tolerance range is increased to 10%. The proposed distributed algorithm provides a reliable and robust scheme for the implementation of large-scale demand response, which is a strong foundation of the future smart-grid development.
Data Availability Statement
The original contributions presented in the study are included in the article/Supplementary Material; further inquiries can be directed to the corresponding author.
Author Contributions
YL: Conceptualization, Writing–original draft, Funding acquisition. TG: Software, Validation. XS: Project administration. ZY: Formal Analysis. YZ: Writing–review and editing. SG: Conception, Methodology. XH: Supervision.
Funding
This work was supported in part by the National Nature Science Foundation of China under Grant 51907024.
Conflict of Interest
Author XS is employed by Guodian Nanjing Automation Co., Ltd.
The remaining authors declare that the research was conducted in the absence of any commercial or financial relationships that could be construed as a potential conflict of interest.
Publisher’s Note
All claims expressed in this article are solely those of the authors and do not necessarily represent those of their affiliated organizations, or those of the publisher, the editors and the reviewers. Any product that may be evaluated in this article, or claim that may be made by its manufacturer, is not guaranteed or endorsed by the publisher.
Supplementary Material
The Supplementary Material for this article can be found online at: https://www.frontiersin.org/articles/10.3389/fenrg.2021.718859/full#supplementary-material
References
Altisen, K., Devismes, S., Dubois, S., and Petit, F. (2019). Introduction to Distributed Self-Stabilizing Algorithms. Synth. Lectures Distributed Comput. Theor. 8 (1), 1–165. doi:10.2200/s00908ed1v01y201903dct015
Bahrami, S., Amini, M. H., Shafie-Khah, M., and Catalao, J. P. S. (2018). A Decentralized Renewable Generation Management and Demand Response in Power Distribution Networks. IEEE Trans. Sustain. Energ. 9 (4), 1783–1797. doi:10.1109/tste.2018.2815502
Burns, B. (2018). Designing Distributed Systems: Patterns and Paradigms for Scalable, Reliable Services. Boston, MA: O'Reilly Media, Inc. doi:10.1109/aspdac.2018.8297270
Chen, L., Yang, Y., and Xu, Q. (2021). Retail Dynamic Pricing Strategy Design Considering the Fluctuations in Day-Ahead Market Using Integrated Demand Response. Int. J. Electr. Power Energ. Syst. 130, 106983. doi:10.1016/j.ijepes.2021.106983
Chen, S., and Liu, C.-C. (2017). From Demand Response to Transactive Energy: State of the Art. J. Mod. Power Syst. Clean. Energ. 5 (1), 10–19. doi:10.1007/s40565-016-0256-x
D'hulst, R., Labeeuw, W., Beusen, B., Claessens, S., Deconinck, G., and Vanthournout, K. (2015). Demand Response Flexibility and Flexibility Potential of Residential Smart Appliances: Experiences from Large Pilot Test in Belgium. Appl. Energ. 155, 79–90. doi:10.1016/j.apenergy.2015.05.101
Dalwadi, N. N., and Padole, C. M. (2017). Comparative Study of Clock Synchronization Algorithms in Distributed Systems. Adv. Comput. Sci. Technol. 10 (6), 1941–1952.
Dvorkin, Y., Lubin, M., Backhaus, S., and Chertkov, M. (2015). Uncertainty Sets for Wind Power Generation. IEEE Trans. Power Syst. 31 (4), 3326–3327. doi:10.1109/TPWRS.2015.2476664
Gao, Y., Sun, Y., Wang, X., Chen, F., Ehsan, A., Li, H., et al. (2017). Multi-objective Optimized Aggregation of Demand Side Resources Based on a Self-Organizing Map Clustering Algorithm Considering a Multi-Scenario Technique. Energies 10 (12), 2144. doi:10.3390/en10122144
Heffron, R., Körner, M.-F., Wagner, J., Weibelzahl, M., and Fridgen, G. (2020). Industrial Demand-Side Flexibility: A Key Element of a Just Energy Transition and Industrial Development. Appl. Energ. 269, 115026. doi:10.1016/j.apenergy.2020.115026
Hoggett, R. (2014). Technology Scale and Supply Chains in a Secure, Affordable and Low Carbon Energy Transition. Appl. Energ. 123, 296–306. doi:10.1016/j.apenergy.2013.12.006
Huang, W., Zhang, N., Kang, C., Li, M., and Huo, M. (2019). From Demand Response to Integrated Demand Response: Review and prospect of Research and Application. Prot. Control. Mod. Power Syst. 4 (1), 1–13. doi:10.1186/s41601-019-0126-4
Iwanicki, K. (2018). “A Distributed Systems Perspective on Industrial IoT,” in 2018 IEEE 38th International Conference on Distributed Computing Systems, Vienna, Austria, July 2–6, 2018 (Vienna, Austria: ICDCS), 1164–1170.
Kolhe, M. (2012). Smart Grid: Charting a New Energy Future: Research, Development and Demonstration. Electricity J. 25 (2), 88–93. doi:10.1016/j.tej.2012.01.018
Lu, N. (2012). An Evaluation of the HVAC Load Potential for Providing Load Balancing Service. IEEE Trans. Smart Grid 3 (3), 1263–1270. doi:10.1109/TSG.2012.2183649
Mahdavi, N., and Braslavsky, J. H. (2020). Modelling and Control of Ensembles of Variable-Speed Air Conditioning Loads for Demand Response. IEEE Trans. Smart Grid 11 (5), 4249–4260. doi:10.1109/tsg.2020.2991835
Marzullo, K., Meling, H., and Mei, A. (2011). “Brief Announcement: When You Don’t Trust Clients: Byzantine Proposer Fast Paxos,” in International Symposium on Distributed Computing, Macau, China, January 1, 2012, 143–144.
Ming, N., Qian, C., Manli, L., Qi, W., and Yi, T. (2018). A Frequency Control Model for Cyber Physical Power System Considering Demand Response Strategy. Energ. Proced. 145, 38–43. doi:10.1016/j.egypro.2018.04.008
Perez, K. X., Baldea, M., and Edgar, T. F. (2016). Integrated HVAC Management and Optimal Scheduling of Smart Appliances for Community Peak Load Reduction. Energy and Buildings 123, 34–40. doi:10.1016/j.enbuild.2016.04.003
Qi, L., Zhang, X., Dou, W., and Ni, Q. (2017). A Distributed Locality-Sensitive Hashing-Based Approach for Cloud Service Recommendation from Multi-Source Data. IEEE J. Select. Areas Commun. 35 (11), 2616–2624. doi:10.1109/jsac.2017.2760458
Rao, J., Shekita, E. J., and Tata, S. (2011). Using Paxos to Build a Scalable, Consistent, and Highly Available Datastore. arXiv preprint arXiv, 1103.2408.
Raynal, M. (2018). Fault-Tolerant Message-Passing Distributed Systems. Cham, Switzerland: Gewerbestrasse, 459.
Ribó-Pérez, D., Larrosa-López, L., Pecondón-Tricas, D., and Alcázar-Ortega, M. (2021). A Critical Review of Demand Response Products as Resource for Ancillary Services: International Experience and Policy Recommendations. Energies 14, 846. doi:10.3390/en14040846
Samad, T., Koch, E., and Stluka, P. (2016). Automated Demand Response for Smart Buildings and Microgrids: The State of the Practice and Research Challenges. Proc. IEEE 104 (4), 726–744. doi:10.1109/JPROC.2016.2520639
Shen, C., Bao, Y. Q., Ji, T. Z., Zhang, J. L., and Wang, Z. (2019). “A Robust Control Strategy for Air Conditioner Group to Participate in Power System Frequency Regulation,” in 2019 IEEE Innovative Smart Grid Technologies-Asia. (Chengdu, China: ISGT Asia), 678–682.
Shi, W. C., and Li, J. P. (2012). “Research on Consistency of Distributed System Based on Paxos Algorithm,” in 2012 International Conference on Wavelet Active Media Technology and Information Processing, Chengdu, China, December 17, 2012 (Chengdu, China: ICWAMTIP), 257–259.
Tang, R., Wang, S., and Yan, C. (2018). A Direct Load Control Strategy of Centralized Air-Conditioning Systems for Building Fast Demand Response to Urgent Requests of Smart Grids. Automation in Construction 87, 74–83. doi:10.1016/j.autcon.2017.12.012
Xiao, F., Zhang, Z., and Abawajy, J. (2019). Workflow Scheduling in Distributed Systems under Fuzzy Environment. Ifs 37 (4), 5323–5333. doi:10.3233/jifs-190483
Zheng, W., Wu, W., Zhang, B., Sun, H., and Liu, Y. (2015). A Fully Distributed Reactive Power Optimization and Control Method for Active Distribution Networks. IEEE Trans. Smart Grid 7 (2), 1021–1033. doi:10.1109/TSG.2015.2396493
Zhou, R., Zhang, Z., Liu, X., Ju, P., Qin, C., and Chen, Q. (2015). “November). Simulation and Modeling of Blended Constant-Frequency and Variable-Frequency Air-Conditioner,” in 2015 5th International Conference on Electric Utility Deregulation and Restructuring and Power Technologies, Changsha, China, November 26–29, 2015 (Chengdu, China: DRPT), 269–273.
Keywords: demand side management, distributed control, large-scale collaboration, slacked control, weak consistency
Citation: Liu Y, Gao T, Sun X, Yang Z, Zhang Y, Gao S and Huang X (2021) A Weak-Consistency–Oriented Collaborative Strategy for Large-Scale Distributed Demand Response. Front. Energy Res. 9:718859. doi: 10.3389/fenrg.2021.718859
Received: 01 June 2021; Accepted: 27 July 2021;
Published: 26 August 2021.
Edited by:
Dongdong Zhang, Guangxi University, ChinaCopyright © 2021 Liu, Gao, Sun, Yang, Zhang, Gao and Huang. This is an open-access article distributed under the terms of the Creative Commons Attribution License (CC BY). The use, distribution or reproduction in other forums is permitted, provided the original author(s) and the copyright owner(s) are credited and that the original publication in this journal is cited, in accordance with accepted academic practice. No use, distribution or reproduction is permitted which does not comply with these terms.
*Correspondence: Yu Liu, eXVsaXVAc2V1LmVkdS5jbg==