- 1Department of Electrical, Electronic, and Information Engineering, University of Bologna, Bologna, Italy
- 2Department of Industrial Electronics, Centro ALGORITMI, University of Minho, Guimarães, Portugal
- 3Department of Electronics and Telecommunications, Rio de Janeiro State University, Rio de Janeiro, Brazil
The growing penetration of distributed renewable energy sources (RES) together with the increasing number of new electric vehicle (EV) model registrations is playing a significant role in zero-carbon energy communities’ development. However, the ever-larger share of intermittent renewable power plants, combined with the high and uncontrolled aggregate EV charging demand, requires an evolution toward new planning and management paradigms of energy districts. Thus, in this context, this paper proposes novel smart charging (SC) techniques that aim to integrate as much as possible RES generation and EV charging demand at the local level, synergically acting on power flows and avoiding detrimental effects on the electrical power system. To make this possible, a centralized charging management system (CMS) capable of individually modulating each charging power of plugged EVs is presented in this paper. The CMS aims to maximize the charging self-consumption from local RES, flattening the peak power required to the external grid. Moreover, the CMS guarantees an overall good state of charge (SOC) at departure time for all the vehicles without requiring additional energy from the grid even under low RES power availability conditions. Two methods that differ as a function of the EV power flow direction are proposed. The first SC only involves unidirectional power flow, while the second one also considers bidirectional power flow among vehicles, operating in vehicle-to-vehicle (V2V) mode. Finally, simulations, which are presented considering an actual case study, validate the SC effects on a reference scenario consisting of an industrial area having a photovoltaic (PV) plant, non-modulable electrical loads, and EV charging stations (CS). Results are collected and performance improvements by operating the different SC methods are compared and described in detail in this paper.
Introduction
Renewable energy sources (RESs), together with electric vehicles (EVs), constitute one of the main points in the green energy shift toward a less carbon-dependent society (Directorate-General for Climate Action (European Commission), 2019). On this matter, particular attention is dedicated to the growing context of positive energy districts (PEDs) to reduce carbon emissions coming from urban areas (Hinterberger et al., 2020; Tuominen, 2020; Rancilio et al., 2021). The general purpose of PED is to be a flexible part of the whole energy system organized in districts rather than single buildings, ensuring an energy exchange between the various stakeholders (Mirbagheri et al., 2018; Tuominen, 2020). A similar paradigm can resemble a net-zero-energy community (ZEC) where renewable energy matches electrical loads, thermal energy consumption, and EVs charging demand (Dogan et al., 2015; Lopes et al., 2016; Zatti et al., 2017).
However, it has been highlighted that replacing conventional vehicles with electric counterparts would sharply increase peak demand forcing a relevant rethinking of the generation, transmission, and distribution system with significant investment already at moderate levels of EV penetration (Nelder and Fitzgerald, 2016; Bovera et al., 2021). A similar concern is caused by the intermittent nature of RES, whose power generation strongly depends on the availability of the primary source (wind, solar, etc.) and cannot usually provide the control and regulation services that conventional sources can do (Luo and Ooi, 2006; Kroposki et al., 2017; Lo Franco et al., 2021a). Intermittent power injection together with localized load power peaks (aggregate EV charging) might even bring to the nonoptimal utilization of conventional plannable power sources for grid supporting operation (ancillary services). However, since conventional generation methods are fossil fuel reliant, this phenomenon is in contrast to the green policy that characterizes the PED, ZEC, and in general RES and EV development. It stands clear that, for mitigating efforts, detrimental effects, and expensive expedients, a synergic integration of EVs with RES (especially photovoltaic (PV)) is an imperative requirement for future smart power systems (Aldik and Khatib, 2019; Hasan et al., 2019; Rakhshani et al., 2019). Indeed, unmanaged grid-to-vehicle (G2V) charging technique is recognized for not being a long-term economically sustainable option, and the development of vehicle-to-anything (V2X) alternatives has been presented as a possible sustainable solution to this issue (Ferreira et al., 2014; Thompson and Perez, 2020). The umbrella term V2X is often used for indicating any unidirectional (V1X) or bidirectional (the actual meaning of V2X) charging management system (CMS) aiming to provide benefits like peak reduction, load shifting, and flattening together with RES self-consumption optimization (Thompson, 2018; Pearre and Ribberink, 2019). Some of the prevailing techniques are vehicle-to-grid (V2G), vehicle-to-building (V2B), vehicle-to-load (V2L), and vehicle-to-community (V2C) (Monteiro et al., 2016). Among them, V2G constitutes by far the most focused method because of the tremendous potential benefits guaranteed by energy and ancillary services that the interaction between EV batteries and the grid might unlock (Lopes et al., 2011; Hasan et al., 2019; Noel et al., 2021). Similar to V2X, V2G presents a unidirectional subset referred to as V1G or more often as smart charging (SC). However, the highly sophisticated real-time communication and control backbone necessary for providing grid services has not reached an adequate technological maturity, and only a small number of commercial products are available yet (Thingvad et al., 2016; Zipf and Most, 2016).
For this reason, multiple authors proposed CMSs capable of providing the above benefits at a local level without necessarily involving the active interaction with the grid and all the associated technological challenges (Bons et al., 2020; Lo Franco et al., 2020). These SC systems, mainly if employed over an aggregate EV fleet, blend particularly well with the definitions of V2B and V2C (Thompson, 2018; Noel et al., 2019). Indeed, taking advance of the capacity made available by aggregated EV fleets, it is possible to optimize the energy consumption of the buildings, mainly in the commercial and industrial context, and energy communities by acting solely at the local value (Yamagata et al., 2014; Tanguy et al., 2016). Although a centralized controller capable of communicating with all the vehicles and charging equipment is still required, its complexity results to be noticeably lower if compared to the V2G mode. Finally, V2L can be simplistically defined as any EV (or aggregates) supplying generic loads, including EVs themselves (Thompson and Perez, 2020). For this reason, it is generally accepted vehicle-to-vehicle (V2V) technique to be a particular case of V2L (Mao et al., 2018). In this case, EVs can act as an energy source for supplying other EVs, pursuing a determined charging policy at a local level. Since there is no sharp edge between all these technologies, it would not be surprising if CMS strategies might achieve specific V2B/V2C and V2V optimizations, especially if considered in contexts, like PEDs, presenting internal generation from RES.
Multiple SC methods have been presented in the literature (Wang et al., 2016; Fachrizal and Munkhammar, 2020; Fouladi et al., 2020; Lo Franco et al., 2020). The list below compares the achieved results of some main reference papers and points out the literature gap that this work tries to fill:
• Authors in (Wang et al., 2016) discussed a strategy for achieving flat power demand employing peak-shaving and valley-filling techniques considering cost minimization constraints. This reference provides a survey from the algorithmic perspective. However, a qualitative and quantitative analysis of the SC benefit based on actual case studies has not been provided. The integration of EV charging with RES considering a variegated population of EV model and the capability of bidirectional power flow among vehicles has not been investigated in this reference.
• In (Fachrizal and Munkhammar, 2020; Ramadhani et al., 2021), the authors investigate the probabilistic impact of EV SC, including the temporal and spatial variability of EV charging demand, household load, and PV system generation. The conclusions show that SC results in improved distribution system performance. Although this reference paper provides an estimation of EV power load considering quantitative data analyses, the PV-EV system integration under low irradiance availability and SC strategies involving the bidirectionality of EV power flow have not been evaluated.
• Three different SC strategies to increase PV solar exploitation are proposed in (Heinisch et al., 2021). The results show that SC strategies can increase up to 62% of the share of PV energy in the charging energy mix, compared to 24% obtained using standard G2V charging. However, this work does not consider a variegate population of EVs, to which different models with different specifications and characteristics belong (such as vehicle battery sizes, onboard charger ratings, and battery state of charge (SOC) at the beginning of the charging process). Moreover, considerations of the SC performance under low PV power conditions are not provided.
• SC methods for self-consumption optimization have been performed in (Lo Franco et al., 2020) employing CMSs capable of modulating each EV charging power individually to industrial districts. This work quantitatively analyzes EV power demand based on a statistical approach and using actual data. However, although self-consumption improvement and peak load reduction are achieved, the proposed CMS does not provide enough energy to the vehicle when low PV availability occurs, leading to a lower EVs’ SOC at the end of the charging process (nonoptimal exploitation of PV sources). Also, bidirectional EV power flows capability has not been considered.
• A preliminary solution was provided in (Lo Franco et al., 2021b), where the proposed CMS differently allocates the available PV energy among charging vehicles as a function of the irradiance availability. Although the results show improved performance in terms of EVs’ SOC at the end of charging, even this paper does not consider the possibility of power-sharing among vehicles given by the bidirectional V2V mode.
Finally, to the best of the authors’ knowledge, no work provides a CMS capable of modulating each EV charging power to optimally exploit the intermittent PV source under low irradiance condition guaranteeing an appreciable trade-off between self-consumption improvement, peak load reduction, and overall good SOC level at the departure time, and in addition, it is also capable of involving power-sharing among vehicle at the local level (bidirectional V2V charging mode). To overcome the aforementioned limitations, two novel charging strategies are proposed in this paper to improve the EV charging process by differently and smartly allocating the available RES power to each EV without involving external grid absorptions. Both new proposed SC strategies ensure an overall good state of charge at departure time for all the vehicles by distributing the generated PV power as a function of the EV SOC. It means that SC prioritizes charging EVs which present a lower SOC value. On the other hand, in the case of abundant RES availability, the two novels SC strategies ensure optimal exploitation of PV power by allocating the latter as a function of the vehicle’s maximum absorbable power. The main difference between the two optimized charging management systems (OCMSs) lies in the EV power flows direction. The first SC only involves unidirectional power flow; on the other hand, the second one also considers bidirectional power flow among vehicles. Operating in V2V mode, the latter might reduce the charge level of a portion of plugged vehicles in favor of other plugged EVs. The two OCMSs are implemented and tested on an actual case study. Simulations are carried out considering an industrial area having a PV plant, non-modulable electrical loads, and EV charging stations (CS). Results are collected and compared based on uninterrupted power data measurement. Finally, power flows’ analysis shows, with an appreciable statistical relevance, the improved performance experienced by operating the novel OCMSs.
The paper is arranged as follows. In EV Aggregate Charging Demand Evaluation for a Working Place Parking Lot Scenario Section, the EV aggregate charging demand in the scenario is evaluated. In SC Method for Self-Consumption Optimization Section, SC methods are introduced and detailed. Assessment of the results is performed in Results Discussion Section. Finally, the conclusion ends the article.
EV Aggregate Charging Demand Evaluation for a Working Place Parking Lot Scenario
The CMS proposed in this paper aims to control the charging power flows of an aggregated EV population inside the energy district. For the sake of clarity, Figure 1 shows the structure of a generic PED in which the CMS operates. From the electrical point of view, the reference system consists of the following:
• Electrical loads such as buildings, household consumers, offices, and industrial areas which adsorb a non-modulable power (
• Internal power sources, usually renewable (RES), such as PV plants that generate an intermittent power (
• A point of common coupling (PCC) electrically connecting the internal loads and distributed RESs to the external grid. Through the PCC, the grid shares the power
• A population of aggregate EVs connected to the electrical system through the CS. The aggregate EV fleet absorbs the power
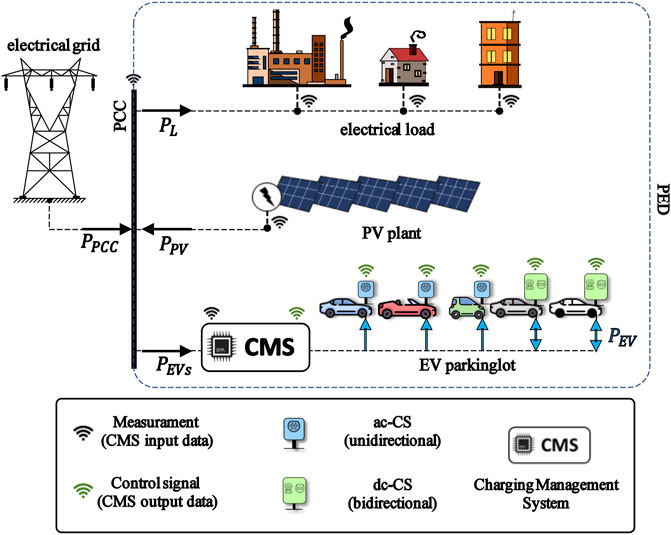
FIGURE 1. Positive energy district (PED) electrical reference system. PED’s power flow convention and sharing among grid (
Following the above convention, the power flow involving the PED can be described through the power balance in Eq. 1.
From the above equation, it can be noted that even if the internal load and generated power (
For the implementation of the SC system, both production and load power measurements are necessary. In this paper, the daily load and generation profiles are extracted from yearly acquisitions. The same procedure is done for the PED power-sharing with the grid (PCC measurements). On the other hand, EVs’ daily power flow is more challenging to be extrapolated due to its gathering complexity, data lacking, and the statistically weak number of EVs. Current measurement data are not sufficient to extrapolate meaningful EV charging profiles. For these reasons, a predictive method capable of estimating the daily aggregate charging power flows is used and described in EV Charging Power Flow Calculation Method Section. Then, in Reference Scenario and Standard G2V EV Charging Effects on Power Demand section, the standard G2V charging effects on the power flow of an actual reference scenario are quantitatively evaluated.
EV Charging Power Flow Calculation Method
The aggregate EV power is obtained by collecting the contribution of each i-th vehicle
The charging power
• The maximum power made available by the CS, named
• The maximum power
• The maximum storable energy, which depends on the
• The
• The parking duration (
To implement the power flows forecasting, the method described in (Lo Franco et al., 2020) has been utilized for carrying out the statistical analysis of vehicles’ data and drivers’ behavior. Considerations about the CS power rating are obtained from data analysis reported in (European Alternative Fuel Observatory - EAFO, 2021). With reference to the Italian scenario, most of the public charging points (about 90%) have a power rating below 22 kW (standard charge), and the latter turns out to be the most widespread value. Thus, in this paper, a homogeneous population of CS, with
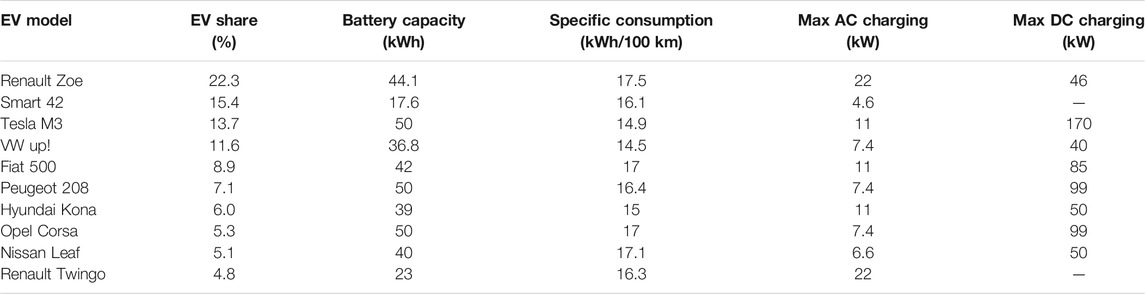
TABLE 1. Data and parameters of the EVs reference population (top 10 best sellers registered in Italy in 2020).
As shown in Table 1, the charging power strongly differs as a function of the vehicle charging rating in the considered CS and EV population. Regardless of whether AC or DC charging is considered, the power that
While
The aggregate EV charging demand profile depends on the charging duration of each
Parking times of each
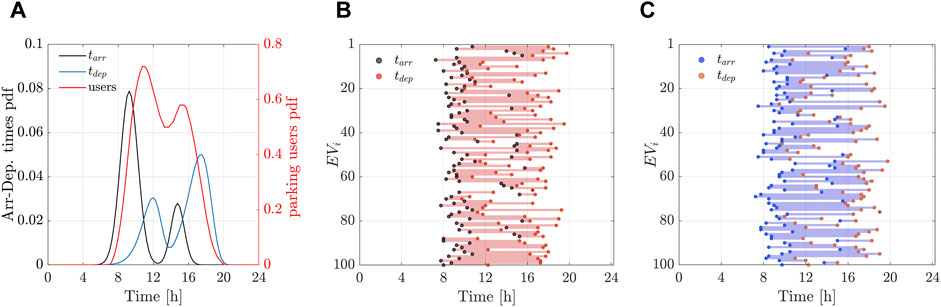
FIGURE 2. (A) Probability distribution function (pdf) of arrival (black line) and departure (blue line) times together with parking lot user’s distribution (red line) during the 24 h. (B,C) Two consecutive days’ set of arrival and departure times.
For all the vehicles, the same value of
Based on the statistical analysis carried out in (Lo Franco et al., 2020), a Weibull function having
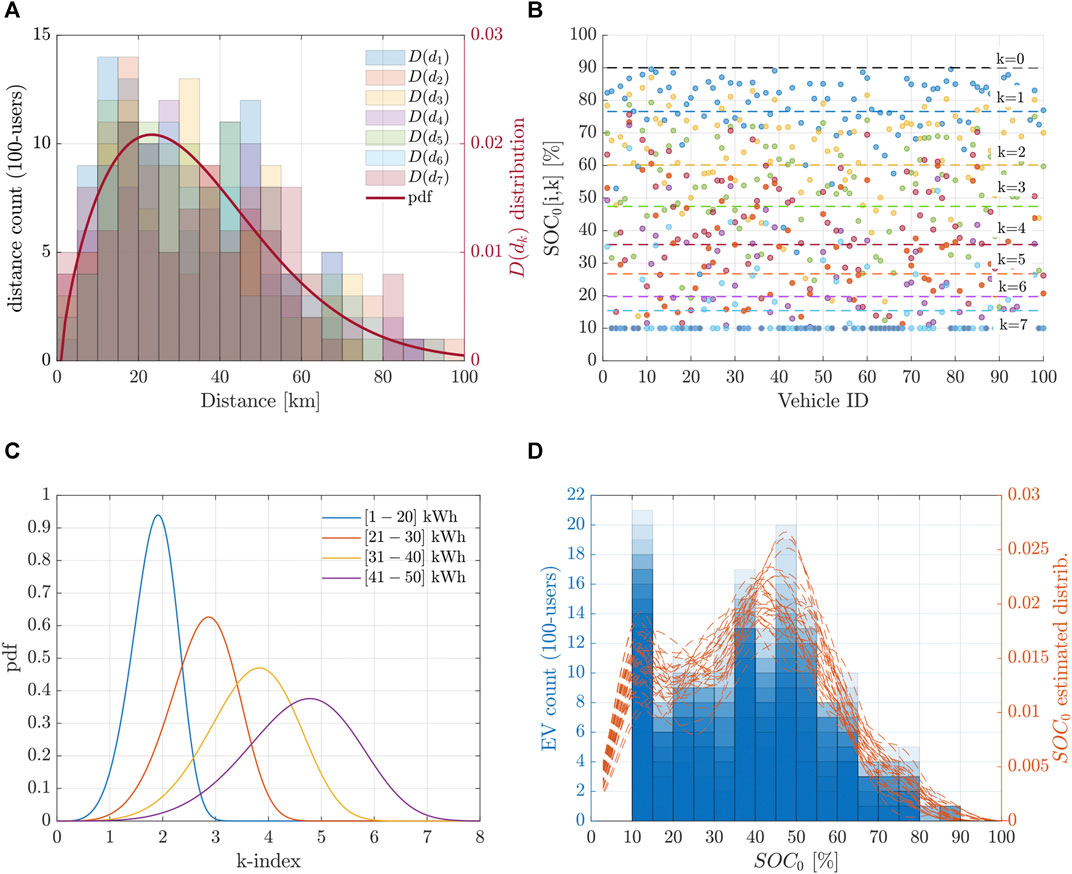
FIGURE 3. (A) distance
To calculate the
The energy consumption during the days after the last full charging (
Finally, information about each EV’s parking time and arrival SOC and data in Table 1 are used as input in a dynamic model developed in MATLAB/Simulink (MathWorks) environments. The model calculates the charging power profile of each
Reference Scenario and Standard G2V EV Charging Effects on Power Demand
Figure 4A shows the reference scenario employed in the simulation. It represents an industrial building (metalworking company) with 50% EV penetration represented by a parking lot of 100 stands having 22 kW charging ports each. The internal generation is provided by a 500 kWp PV plant. Aggregated EV load is calculated, and its impact on the daily plant power profile is evaluated. The metalworking company load
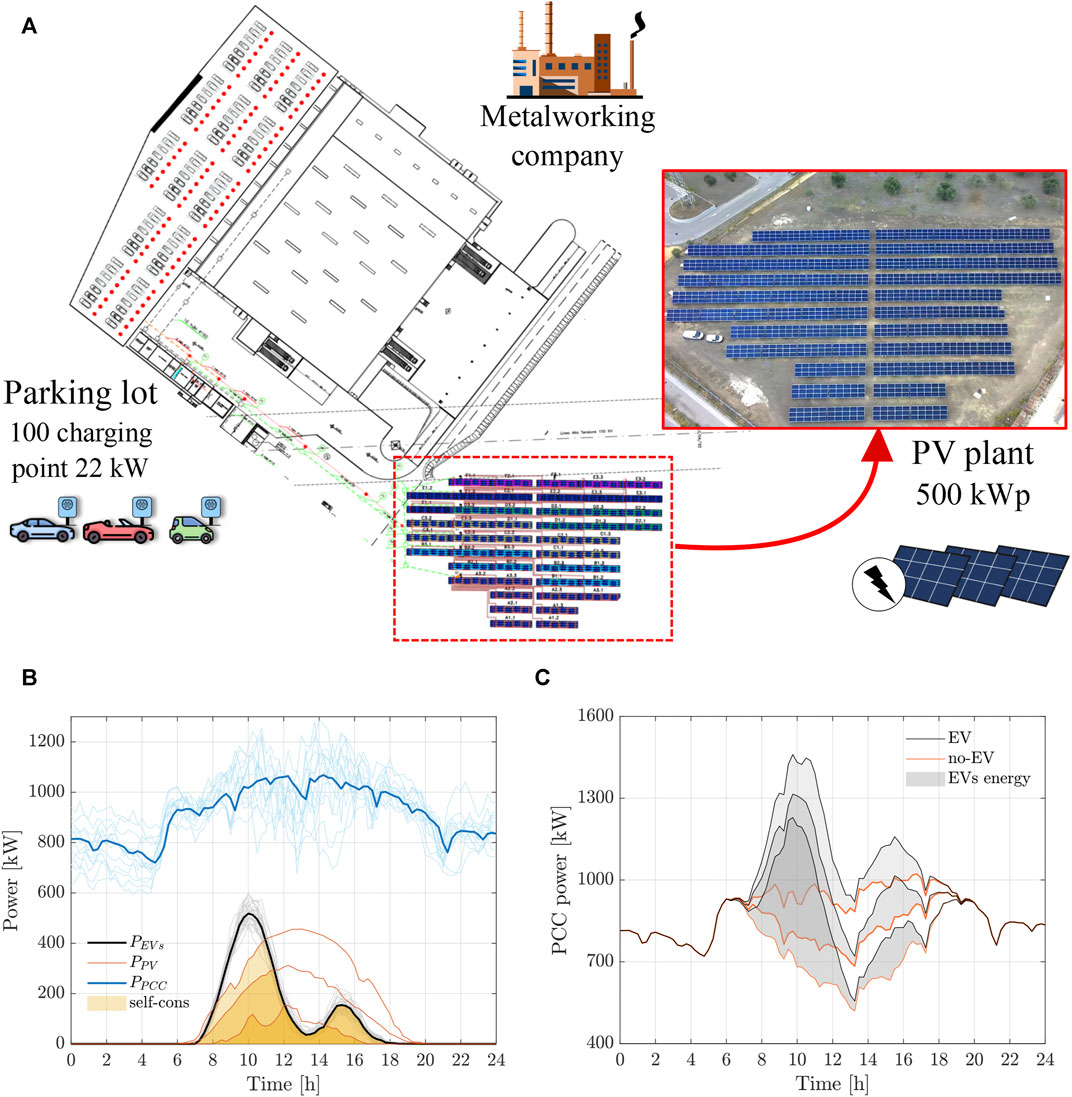
FIGURE 4. (A) Industrial building reference scenario. (B)
To quantitatively characterize the effects of aggregate EV charging on power flow and evaluate EV profile overlapping with the PV generation, some figures of merit are defined. The ratio between the orange area of Figure 4B and the total EV charging energy (gray area in Figure 4C)) is the charging self-consumption coefficient
To evaluate the effect of EVs charging on PCC power flow, the normalized peak power
The annual analysis carried out for evaluating
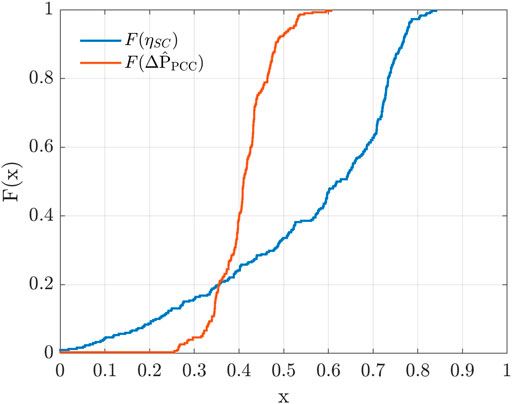
FIGURE 5. Cumulative distribution assessment of the self-consumption
To improve the EV aggregate charging impact on power flows (mitigate
SC Method for Self-Consumption Optimization
To improve performances, increase charging self-consumption, and mitigate the detrimental effects due to grid power peaks, a CMS has been proposed in (Lo Franco et al., 2020). This CMS permits the management of the charging power of each EV leading to SC mode operations. It aims to optimize
Considering a 1-day simulation, the charging self-consumption is defined by
The proposed management system operates with a 1 min sampling. In each time step, it is possible to assume constant
Considering the PV power availability and the EV power demand at a generic instant t, the main target of the proposed SC methods is to manage the aggregate EV charging to maximize
where the problem’s constraints are reported in Eq. 12. The constraint equation is given by Eq. 3 and depends on the vehicle’s model, the number of plugged vehicles, and the SOC level evolution during the charging process (please refer to EV Aggregate Charging Demand Evaluation for a Working Place Parking Lot Scenario Section of the paper for more details). Moreover, the constraint ensures the unidirectionality of power flows.
The solution can be obtained considering Figure 6A that shows the contour plot of
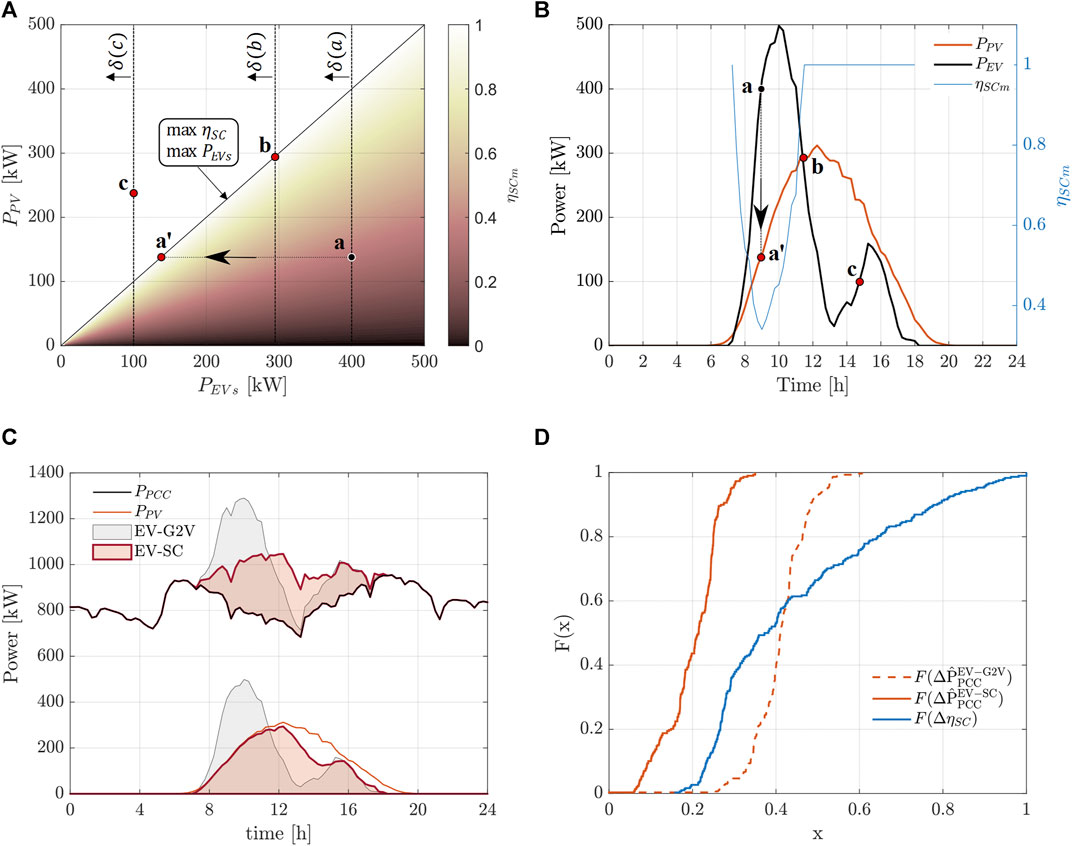
FIGURE 6. (A) The 1 min charging self-consumption (
Finally, it is possible to note that the points that fulfill Eq. 11 are represented by the diagonal black line (
Through Eq. 13, the CMS modulates each vehicle charging to ensure that the aggregate charging power overlaps the PV available power (
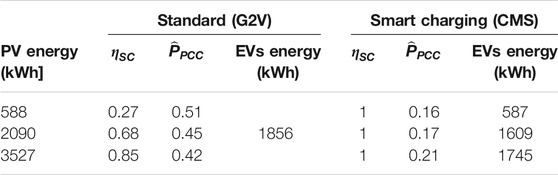
TABLE 2. Quantitative comparison between standard G2V charging and SC performance under low (588 kWh/d), medium (2090 kWh/d), and high (3,527 kWh/d) irradiance PV scenarios.
Although the results show an improvement in charging self-consumption and a reduction in PCC peak power, the above-described SC method presents two relevant weaknesses:
• Weakness 1. The CMS based on Eq. 13 provides less total energy to the vehicles compared to standard G2V charging. Indeed,
• Weakness 2. Under the condition where the power required by the whole EV fleet is totally covered by PV generation, the charging may still underutilize the available PV energy. Since the CMS based on Eq. 13 equally allocates
A suboptimal solution could be to charge the vehicles from the grid during low irradiance availability, nevertheless leading to a self-consumption reduction of the whole district. Two novel charging strategies are investigated to verify which improvements could be achieved by handling differently the EVs charging without involving the external grid (no detrimental effect on
• Solution 1. Instead of evenly share
• Solution 2. To overcome weakness 2, which occurs when the PV power is more or equal to the aggregate EV power request, the OCMS allocates each
Although they both overcome the above weaknesses, the two proposed OCMS differ for the power flow direction. The first only consider the PV to EV flow and is indicated as unidirectional OCMSs (OCMS) (1d-OCMS). On the other hand, the second one is defined as bidirectional OCMS (2d-OCMS) because it also considers the power flow among EVs (V2V). The main difference is that the 2d-OCMS might reduce the charge level of a portion of plugged vehicles (discharging operation) in favor of other plugged EVs. Finally, Table 3 summarizes the optimization objective and constraints between the CMS and the proposed novel 1d-OCMS and 2d-OCMS.
Unidirectional OCMS, 1d-OCMS
The unidirectional OCMS, 1d-OCMS, allocates the reference charging power of each
The exponents
Note that h varies because of the daily irradiation
Since
Weighting factors
Bidirectional OCMS, 2d-OCMS (V2V Operation)
The bidirectional OCMS, 2d-OCMS, allocates the reference charging power of each
Replacing in Eq. 17 the weighting factor
If the PV availability is sufficient to guarantee the maximum charging power for all plugged vehicles (
The difference with respect to the 1d-OCMS is that the
During the discharging phase, EVs which have higher SOC share a fraction of their energy with vehicles having lower SOC levels. The discharging power remains proportional to
Unlike the unidirectional power flow, which only considers the direction from an energy source X to the EV (X2V), the bidirectional power flow, which contemplates EV’s battery discharging toward a generic load (V2X), is feasible only if the charging infrastructure allows it. At the current stage, it is not possible to invert the power flows with AC-CS since the charging parameters (voltages and currents) are mainly managed by the vehicle onboard converter. Furthermore, the communication protocols between the AC-CS and the EV (PWM/impedance modulation in control pilot pin of the Type 2 connector (IEC 62196-1 TC 23/SC 23H, 2014)), despite allowing charging power modulation, does not permit power flow inversion. For these reasons, the V2V (in general V2X) mode may currently be feasible only via DC-CSs (off-board charging), which, as Table 1 shows, bypasses the onboard converter size limitation providing higher-rated powers. However, in order to compare the performance of the different proposed CMSs, this paper assumes the same charging rating for each EV, both considering AC or DC charging.
Results and Discussion
This section shows and discusses the obtained results considering applying the two novel OCMSs on the reference scenario described in Reference Scenario and Standard G2V EV Charging Effects on Power Demand section. Simulations are carried out using MATLAB/Simulink. A comparison between 1d-OCMS, 2d-OCMS, and the standard CMS is carried out in terms of EV fleet-level charging performance improvement. Since both OCMSs aim to mitigate the above weaknesses which happen under specific conditions, both techniques are simulated considering low irradiance conditions (low h ratio value) and high irradiance scenario (h ratio around 1 or more) referring to weaknesses 1 and 2, respectively. Together with them, standard CMS described in Eq. 13 is reported as well.
To better understand how the standard and the proposed standard CMS and the two proposed OCMS operate, simulations were run considering a winter-day (medium-low irradiance scenario). The effects on power flows of the different SC algorithms are displayed in Figure 8 and Figure 9. From multivariate analysis on 1d-OCMS performance, the best results were achieved calling off the
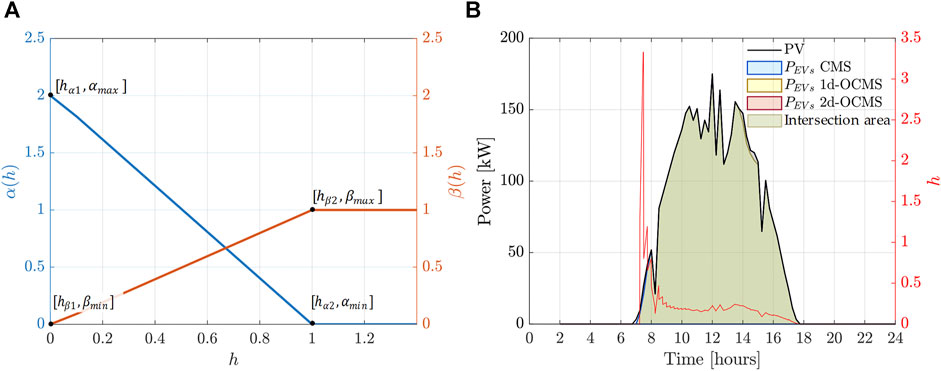
FIGURE 8. (A) 1d-OCMS setting of the weighting factors
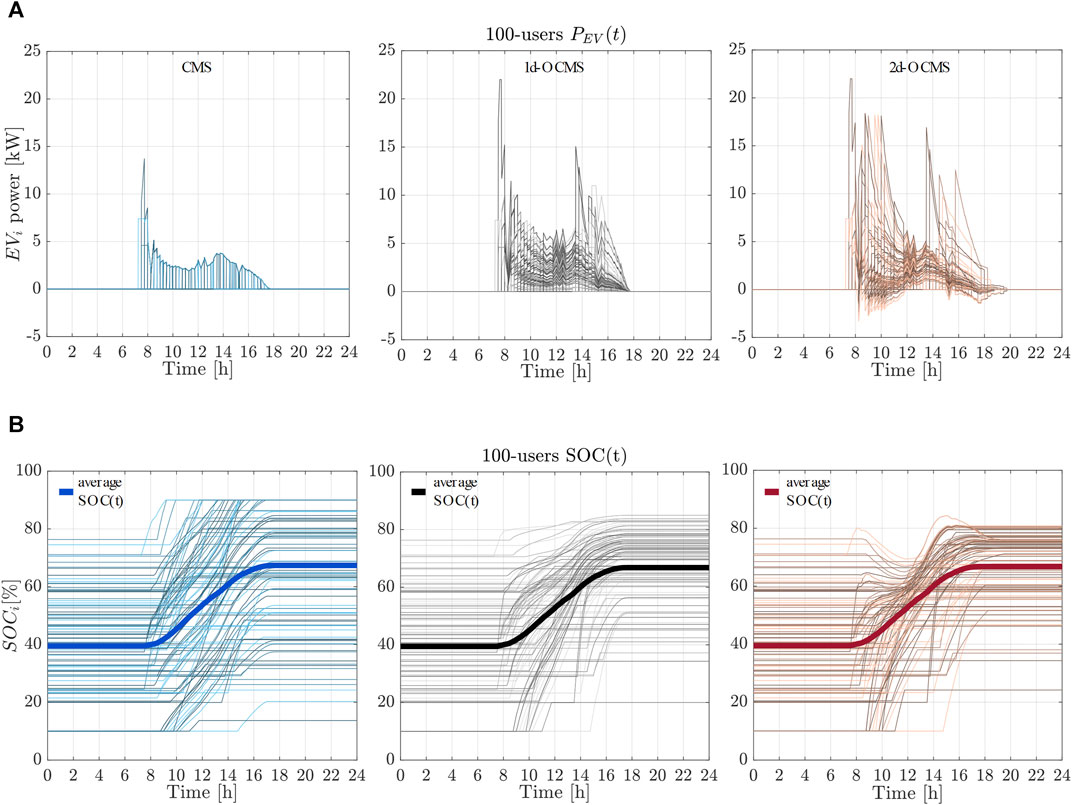
FIGURE 9. EV population (100 users) charging power profile (A) and SOC evolution (B) as a function of the time, in case of standard CMS (left frames, blue tint), 1d-OCMS (center frames, gray tint), and 2d-OCMS (right frames, orange tint) on a 24 h period. Each line in (A) depicts the power value
Figure 8B shows the PV power (black line) used as an example in the simulation. Since the daily PV generation amount is 1,050 kWh (half of the annual-based daily average), this case study can be classified as a medium-low irradiance scenario. The colored area plots depict the
Figure 9A shows each
To provide an appreciable statistical significance of the results, the simulation runs considering 31 different days of January (1 month) are carried out. In this condition, the solar irradiation is generally low providing a scenario where the standard CMS’s weakness 1 occurs more frequently. The distribution of
Figure 10 shows the
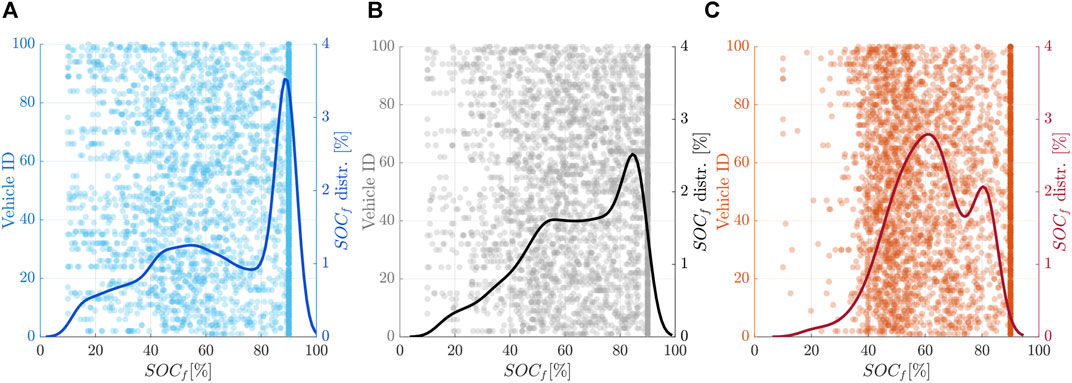
FIGURE 10. 31-day
The results demonstrate that the two OCMS (1d-OCMS and 2d-OCMS) provide better performance decreasing the number of EVs having a low
Finally, Figure 11 provides an overview of the 31-day simulation results. Figure 11A shows the monthly-based average
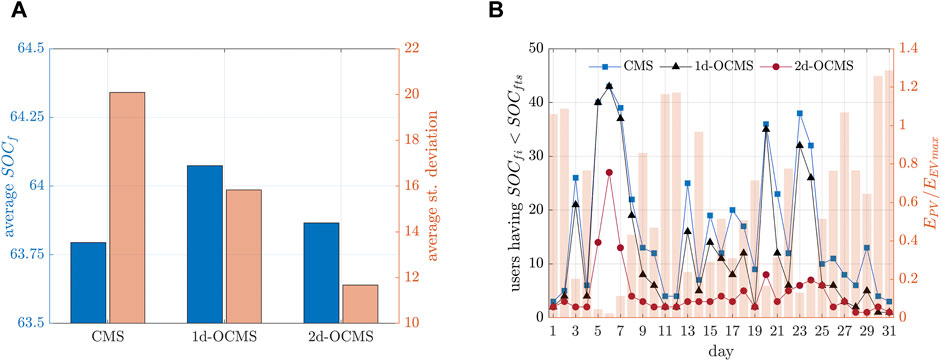
FIGURE 11. (A) Monthly-based average
In other words, if
In order to compare the 1d-OCMS and 2d-OCMS performance with respect to the standard CMS under medium-high PV irradiance conditions, simulations are run considering the 31 days of May where weakness 2 might occur more frequently. Figure 12A shows the daily power flows referring to a scenario in which the PV plant’s daily energy generation is about 1900 kWh (
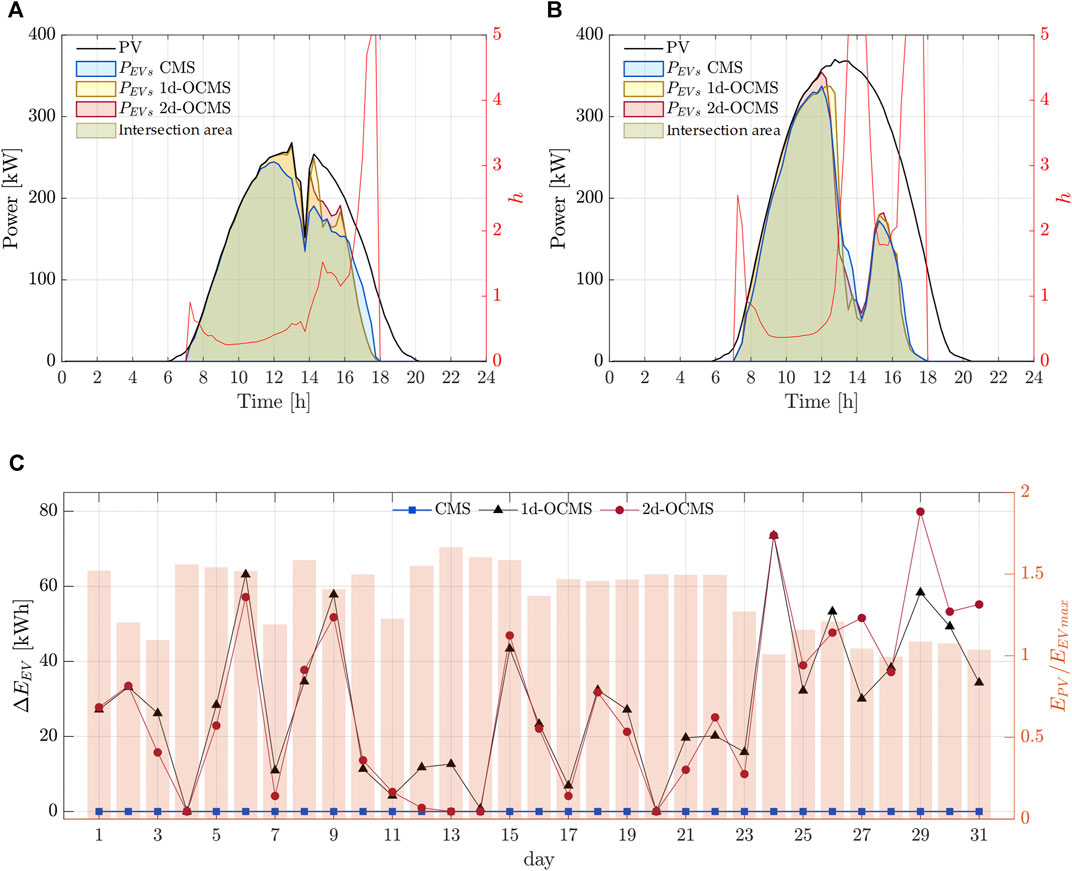
FIGURE 12. Daily evolution of h-factor (red line), PV (black line) power, and EVs charging power in case of standard CMS (light blue area), 1d-OCMS (yellow area), and 2d-OCMS (red area) in case of
To quantitatively calculate the daily energy gap, defined as
Conclusion
In the context of energy districts presenting a growing penetration of RES and EVs, this paper proposed two novel EV charging management systems able to operate in SC mode, suitable for working place parking lots or similar scenarios. Proposed SC positively integrates EV charging with intermittent energy sources by dynamically managing the aggregate EV power demand acting on each vehicle charging power. The CMS pursues local level EV charging maximization from renewable internal power sources, minimizing consumption from the external grid.
Simulations considering an actual case study validated the effects of the proposal on a reference scenario consisting of an industrial area having a PV plant, non-modulable electrical loads, and EV CS. Based on 24/7/365 data analysis, results demonstrated that SC increases charging self-consumption by 45% on average compared to the standard (G2V) charging. While a 20% reduction in peak power load (measured on PCC, grid side) is experienced as well. However, two main weaknesses emerged. Firstly, EVs’ SOC at the instant of departure is particularly low compared to standard G2V counterpart under low availabilities of the primary internal power source. Secondly, PV energy appears to be underutilized due to the equal allocation of PV power among vehicles.
Two novel charging strategies aiming to overcome these two weaknesses are proposed. Both OCMSs ensure an overall good SOC level at departure time for all the vehicles and maximize the PV power utilization, avoiding the bottleneck phenomenon of the maximum charger rating. The two OCMS differs as a function of the power flow direction. The 1d-OCMS only considers the PV to EV flows direction (standard SC). On the other hand, the 2d-OCMS enables bidirectional power flow among EVs (V2V). Simulations carried out in conditions where these weaknesses are more highlighted showed improvements of 36.5% (1d-OCMS) and 68.9% (2d-OCMS) in the number of users presenting a departure SOC suitable for covering a 1-day trip under medium-low irradiance scenarios, considering that medium-high irradiance scenarios 1d- and 2d-OCMS present similar performances. Both techniques managed to provide up to 80 kW h/d more than the standard CMS (+30 kWh on average). Most performing results in terms of SOC distribution are achieved by operating the 2d-OCMS. Thanks to the bidirectional V2V mode, an average decrease of 40% in SOC standard deviation at the departure instant has been achieved. If suitable, 2d-OCMS should be preferred because of its ability to obtain a homogeneous charging process and overall better performance in all investigated scenarios. However, in case conventional unidirectional charging operations are required, 1d-OCMS should be preferred because of the shown improvements in comparison to the standard CMS.
Data Availability Statement
The original contributions presented in the study are included in the article/Supplementary Material; further inquiries can be directed to the corresponding author.
Author Contributions
All authors listed have made a substantial, direct, and intellectual contribution to the work and approved it for publication.
Funding
This work was supported by the project “Energy Networks Integration for Urban Systems (ENERGYNIUS)” funded by POR-FESR Region Emilia Romagna (Italy) 2014-2020.
Conflict of Interest
The authors declare that the research was conducted in the absence of any commercial or financial relationships that could be construed as a potential conflict of interest.
Publisher’s Note
All claims expressed in this article are solely those of the authors and do not necessarily represent those of their affiliated organizations or those of the publisher, the editors, and the reviewers. Any product that may be evaluated in this article or claim that may be made by its manufacturer is not guaranteed or endorsed by the publisher.
References
Aldik, A., and Khatib, T. (2019). EV Aggregators and Energy Storage Units Scheduling into Ancillary Services Markets: The Concept and Recommended Practice. Wevj 11, 8. doi:10.3390/wevj11010008
Bernards, R., van Westering, W., Morren, J., and Slootweg, H. (2020). Analysis of Energy Transition Impact on the Low-Voltage Network Using Stochastic Load and Generation Models. Energies 13, 6097. doi:10.3390/en13226097
Bons, P. C., Buatois, A., Ligthart, G., Geerts, F., Piersma, N., and van den Hoed, R. (2020). Impact of Smart Charging for Consumers in a Real World Pilot. Wevj 11, 21. doi:10.3390/wevj11010021
Bovera, F., Gabba, M., and Zatti, M. (2021). An Optimization Model for the Provision of Flexibility and Dispatching Resources by Multi-Vector Smart Energy Districts. E3s Web Conf. 238, 05007. doi:10.1051/e3sconf/202123805007
Branco, N. C., and Affonso, C. M. (2020). Probabilistic Approach to Integrate Photovoltaic Generation into Pevs Charging Stations Considering Technical, Economic and Environmental Aspects. Energies 13, 5086. doi:10.3390/en13195086
Directorate-General for Climate Action (European Commission) (2019). Going Climate-Neutral by 2050 - A Strategic Long-Term Vision for a Prosperous, Modern, Competitive and Climate-Neutral EU Economy. Luxembourg: Publications Office of the European Union. doi:10.2834/02074
Dogan, A., Kuzlu, M., Pipattanasomporn, M., Rahman, S., and Yalcinoz, T. (2015). “Impact of EV Charging Strategies on Peak Demand Reduction and Load Factor Improvement,” in 2015 9th International Conference on Electrical and Electronics Engineering (ELECO), Bursa, Turkey, November 26–28, 2015 (IEEE), 374–378. doi:10.1109/ELECO.2015.7394559
European Alternative Fuel Observatory - EAFO (2021). Available at: https://www.eafo.eu/ (Accessed March 6, 2021).
Ev database (2021). EV Database Compare Electric Vehicles. Available at: https://ev-database.org/ (Accessed March 16, 2021).
Fachrizal, R., and Munkhammar, J. (2020). Improved Photovoltaic Self-Consumption in Residential Buildings with Distributed and Centralized Smart Charging of Electric Vehicles. Energies 13, 1153. doi:10.3390/en13051153
Ferreira, J. C., Monteiro, V., and Afonso, J. L. (2014). Vehicle-to-anything Application (V2Anything App) for Electric Vehicles. IEEE Trans. Ind. Inf. 10, 1927–1937. doi:10.1109/TII.2013.2291321
Fouladi, E., Baghaee, H. R., Bagheri, M., and Gharehpetian, G. B. (2020). Power Management of Microgrids Including PHEVs Based on Maximum Employment of Renewable Energy Resources. IEEE Trans. Ind. Applicat. 56, 5299–5307. doi:10.1109/TIA.2020.3010713
Hasan, E., Sharma, S., and Brenna, M. (2019). “Virtual Energy Storage System Using Aggregated Electric Vehicles for Ancillary Services in Distribution Grid,” in 2019 AEIT International Conference of Electrical and Electronic Technologies for Automotive, AEIT AUTOMOTIVE 2019, Turin, Italy, July 2–4, 2019 (Institute of Electrical and Electronics Engineers Inc.). doi:10.23919/EETA.2019.8804570
Heinisch, V., Göransson, L., Erlandsson, R., Hodel, H., Johnsson, F., and Odenberger, M. (2021). Smart Electric Vehicle Charging Strategies for Sectoral Coupling in a City Energy System. Appl. Energ. 288, 116640. doi:10.1016/j.apenergy.2021.116640
Hinterberger, R., Gollner, C., Noll, M., Meyer, S., and Schwarz, H.-G. (2020). White Paper on PED Reference Framework White Paper on Reference Framework for Positive Energy Districts and Neighbourhoods. Vienna, Austria. Available at: https://jpi-urbaneurope.eu/ped/ (Accessed April 2, 2021).
IEC 62196-1 TC 23/SC 23H (2014). IEC 62196-1 Plugs, Socket-Outlets, Vehicle Connectors and Vehicle Inlets - Conductive Charging of Electric Vehicles - Part 1: General Requirements. IEC. Available at: https://webstore.iec.ch/publication/6582 (Accessed March 10, 2021).
Kroposki, B., Johnson, B., Zhang, Y., Gevorgian, V., Denholm, P., Hodge, B.-M., et al. (2017). Achieving a 100% Renewable Grid: Operating Electric Power Systems with Extremely High Levels of Variable Renewable Energy. IEEE Power Energ. Mag. 15, 61–73. doi:10.1109/MPE.2016.2637122
Lo Franco, F., Ricco, M., Mandrioli, R., and Grandi, G. (2020). Electric Vehicle Aggregate Power Flow Prediction and Smart Charging System for Distributed Renewable Energy Self-Consumption Optimization. Energies 13, 5003. doi:10.3390/en13195003
Lo Franco, F., Morandi, A., Raboni, P., and Grandi, G. (2021a). Efficiency Comparison of DC and AC Coupling Solutions for Large-Scale PV+BESS Power Plants. Energies 14, 4823. doi:10.3390/en14164823
Franco, F. L., Ricco, M., Mandrioli, R., Paternost, R. F. P., and Grandi, G. (2021b). “State of Charge Optimization-Based Smart Charging of Aggregate Electric Vehicles from Distributed Renewable Energy Sources,” in 2021 IEEE 15th International Conference on Compatibility, Power Electronics and Power Engineering, Florence, Italy, July 14–16, 2021 (CPE-POWERENG, IEEE), 1–6. doi:10.1109/CPE-POWERENG50821.2021.9501214
Lopes, J. A. P., Soares, F. J., and Almeida, P. M. R. (2011). Integration of Electric Vehicles in the Electric Power System. Proc. IEEE 99, 168–183. doi:10.1109/JPROC.2010.2066250
Lopes, R. A., Martins, J., Aelenei, D., and Lima, C. P. (2016). A Cooperative Net Zero Energy Community to Improve Load Matching. Renew. Energ. 93, 1–13. doi:10.1016/j.renene.2016.02.044
Luo, C., and Ooi, B.-T. (2006). Frequency Deviation of Thermal Power Plants Due to Wind Farms. IEEE Trans. Energ. Convers. 21, 708–716. doi:10.1109/TEC.2006.874210
Mao, T., Zhang, X., and Zhou, B. (2018). Modeling and Solving Method for Supporting 'Vehicle-To-Anything' EV Charging Mode. Appl. Sci. 8, 1048. doi:10.3390/APP8071048
Mirbagheri, S. M., Bovera, F., Falabretti, D., Moncecchi, M., Delfanti, M., Fiori, M., and Merlo, M. (2018). “Monte Carlo Procedure to Evaluate the E-Mobility Impact on the Electric Distribution Grid,” in 2018 International Conference of Electrical and Electronic Technologies for Automotive, AUTOMOTIVE 2018, Milan, Italy, July 9–11, 2018 (Institute of Electrical and Electronics Engineers Inc.). doi:10.23919/EETA.2018.8493169
Monteiro, V., Pinto, J. G., and Afonso, J. L. (2016). Operation Modes for the Electric Vehicle in Smart Grids and Smart Homes: Present and Proposed Modes. IEEE Trans. Veh. Technol. 65, 1007–1020. doi:10.1109/TVT.2015.2481005
Nelder, C., and Fitzgerald, G. (2016). Electric Vehicles as Distributed Energy Resources. Available at: https://www.researchgate.net/publication/324417842_Electric_Vehicles_as_Distributed_Energy_Resources (Accessed March 8, 2021).
Noel, L., Zarazua de Rubens, G., Kester, J., and Sovacool, B. K. (2019). Vehicle-to-Grid. Cham: Springer International Publishing. doi:10.1007/978-3-030-04864-8
Noel, L., Zarazua de Rubens, G., Kester, J., and Sovacool, B. K. (2021). Leveraging User-Based Innovation in Vehicle-To-X and Vehicle-To-Grid Adoption: A Nordic Case Study. J. Clean. Prod. 287, 125591. doi:10.1016/j.jclepro.2020.125591
Pearre, N. S., and Ribberink, H. (2019). Review of Research on V2X Technologies, Strategies, and Operations. Renew. Sustain. Energ. Rev. 105, 61–70. doi:10.1016/j.rser.2019.01.047
Rakhshani, E., Rouzbehi, K., J. Sánchez, A. A., Tobar, A. C., and Pouresmaeil, E. (2019). Integration of Large Scale PV-Based Generation into Power Systems: A Survey. Energies 12, 1425. doi:10.3390/en12081425
Ramadhani, U. H., Fachrizal, R., Shepero, M., Munkhammar, J., and Widén, J. (2021). Probabilistic Load Flow Analysis of Electric Vehicle Smart Charging in Unbalanced LV Distribution Systems with Residential Photovoltaic Generation. Sustain. Cities Soc. 72, 103043. doi:10.1016/j.scs.2021.103043
Rancilio, G., Bovera, F., and Delfanti, M. (2021). “A Techno-Economic Evaluation of the Impact of Electric Vehicles Diffusion on Italian Customer Billing Tariffs. E3s Web Conf. 238, 07003. doi:10.1051/e3sconf/202123807003
Tanguy, K., Dubois, M. R., Lopez, K. L., and Gagné, C. (2016). Optimization Model and Economic Assessment of Collaborative Charging Using Vehicle-To-Building. Sustain. Cities Soc. 26, 496–506. doi:10.1016/j.scs.2016.03.012
Thingvad, A., Martinenas, S., Andersen, P. B., Marinelli, M., Olesen, O. J., and Christensen, B. E. (2016). “Economic Comparison of Electric Vehicles Performing Unidirectional and Bidirectional Frequency Control in Denmark with Practical Validation,” in 2016 51st International Universities Power Engineering Conference (UPEC), Coimbra, Portugal, September 6–9, 2016 (IEEE), 1–6. doi:10.1109/UPEC.2016.8113988
Thompson, A. W., and Perez, Y. (2020). Vehicle-to-Everything (V2X) Energy Services, Value Streams, and Regulatory Policy Implications. Energy Policy 137, 111136. doi:10.1016/J.ENPOL.2019.111136
Thompson, A. W. (2018). Economic Implications of Lithium Ion Battery Degradation for Vehicle-To-Grid (V2X) Services. J. Power Sourc. 396, 691–709. doi:10.1016/j.jpowsour.2018.06.053
Tuominen, P. (2020). Yes to Positive Energy Districts. Available at: https://www.vttresearch.com/en/news-and-ideas/yes-positive-energy-districts-how-make-it-happen (Accessed March 21, 2021).
Ul-Haq, A., Cecati, C., and El-Saadany, E. (2018). Probabilistic Modeling of Electric Vehicle Charging Pattern in a Residential Distribution Network. Electric Power Syst. Res. 157, 126–133. doi:10.1016/j.epsr.2017.12.005
UNRAE (2020). Top 10 Per Alimentazione - Dicembre 2019. UNRAE, 1–2. Available at: http://www.unrae.it/dati-statistici/immatricolazioni/4831/top-10-per-alimentazione-dicembre-2019 (Accessed March 16, 2021).
Wang, Q., Liu, X., Du, J., and Kong, F. (2016). Smart Charging for Electric Vehicles: A Survey from the Algorithmic Perspective. IEEE Commun. Surv. Tutorials 18, 1500–1517. doi:10.1109/COMST.2016.2518628
Yamagata, Y., Seya, H., and Kuroda, S. (2014). Energy Resilient Smart Community: Sharing Green Electricity Using V2C Technology. Energ. Proced. 61, 84–87. doi:10.1016/j.egypro.2014.11.912
Zatti, M., Martelli, E., and Amaldi, E. (2017). “A Three-Stage Stochastic Optimization Model for the Design of Smart Energy Districts under Uncertainty,” in 27th European Symposium on Computer Aided Process Engineering. Computer Aided Chemical Engineering (Amsterdam: Elsevier B.V.), 2389–2394. doi:10.1016/B978-0-444-63965-3.50400-1
Keywords: electric vehicles, smart charging, vehicle-to-vehicle (V2V), renewable energy source (RES), state of charge (SOC), photovoltaic (PV), parking lot charging station, charging management system (CMS)
Citation: Lo Franco F, Mandrioli R, Ricco M, Monteiro V, Monteiro LFC, Afonso JL and Grandi G (2021) Electric Vehicles Charging Management System for Optimal Exploitation of Photovoltaic Energy Sources Considering Vehicle-to-Vehicle Mode. Front. Energy Res. 9:716389. doi: 10.3389/fenrg.2021.716389
Received: 28 May 2021; Accepted: 27 September 2021;
Published: 08 November 2021.
Edited by:
Ningyi Dai, University of Macau, ChinaReviewed by:
Hongcai Zhang, University of Macau, ChinaShabana Urooj, Princess Nourah bint Abdulrahman University, Saudi Arabia
Copyright © 2021 Lo Franco, Mandrioli, Ricco, Monteiro, Monteiro, Afonso and Grandi. This is an open-access article distributed under the terms of the Creative Commons Attribution License (CC BY). The use, distribution or reproduction in other forums is permitted, provided the original author(s) and the copyright owner(s) are credited and that the original publication in this journal is cited, in accordance with accepted academic practice. No use, distribution or reproduction is permitted which does not comply with these terms.
*Correspondence: Mattia Ricco, mattia.ricco@unibo.it