- 1Department of Agricultural and Resource Economics, University of California, Berkeley, Berkeley, CA, United States
- 2Department of Trade and Finance, Faculty of Economics and Management, Czech University of Life Sciences Prague, Prague, Czechia
- 3Trade and Social Studies, Faculty of Economics and Management, Institute of Marketing, Slovak University of Agriculture, Nitra, Slovakia
- 4Department of Economics and Finance, Institute of Finance and Economics, University of Tyumen, Tyumen, Russia
This paper focuses on the strategies that employ the fifth generation (5G) wireless networks in the optimal management of demand-side response in the future energy systems with the high penetration of renewable energy sources (RES). It also provides a comparison between advantages and challenges of 5G networks in demand-response renewable energy grids. Large-scale renewable energy integration always leads to a mismatch between generation and load demand in the short run due to the intermittency. It is often envisioned that 5G wireless networks that were recently launched and would most likely be fully deployed worldwide by 2035 would bring many technological and economic benefits for a plethora of the future high-renewables grids featuring electric transport and heating as well as prosumers generating renewable energy and trading it back to the grid (for example, in the vehicle-to-grid (V2G) framework) and among themselves using peer-to-peer (P2P) networks. Our paper offers a comprehensive analysis of 5G architecture with the perspectives of optimal management of demand-side response in the smart grids of the future. We show that the effective deployment of faster and more reliable wireless networks would allow faster data transfers and processing, including peer-to-peer (P2P) energy trade market, Internet of Vehicles (IoV) market, or faster smart metering, and thence open the path for the full-fledged Internet of Energy (IoE). Moreover, we show that 5G wireless networks might become in the future sustainable energy systems paving the road to even more advanced technologies and the new generations of networks. In addition, we demonstrate that for the effective management of energy demand-side response with a high share of renewables, certain forms of governments funding and incentives might be needed. These are required to strengthen the support of RES and helping to shift to the green economy.
Introduction
Any intelligent energy management system is intended to keep the power grid in a stable state by balancing the power generated from all sources with the power consumed (Avancini et al., 2019; Jannati et al., 2020). This is a very complex task in today’s globalized world with a huge demand for energy both at the industrial and at the household level. Smart grids that exist today and those that will appear in the future have to work with lots of data points in very short time spans and quickly and promptly react to any changes. Here is where the Internet of Energy (IoE), a novel concept that became popular recently and originates from and related to the Internet of Things (IoT) comes into the picture. Ioe makes it possible to send information signals back and forth through the grid with an unprecedented speed and accuracy. However, unlike IoT, the IoE focuses on the links and connection between the energy appliances and various devices represented by the sensors and smart meters (Hossein Motlagh et al., 2020; Liao et al., 2020; Mahmud et al., 2020; Pawar et al., 2020).
One would probably agree with the fact that IoE enables consumers and prosumers to independently coordinate supply and demand. Moreover, it is equipped with intelligent forecasting systems that use weather forecasts, expected traffic flows and other information to predict future energy needs (Strielkowski et al., 2019; Chu et al., 2020; Majumdar et al., 2020). Some examples of these advanced apps include measurement data management, network analysis, substation management, distributed energy resource management systems and the low voltage outage management system (Choi, 2019; Kumar et al., 2019; Horowitz et al., 2020; Momen et al., 2020).
The technological limitations in the measurement of energy consumption no longer lead to the averaged peak prices being passed on equally to all consumer. This becomes especially relevant when the renewable energy sources (RES) step in (see a study from Australia (Simshauser, 2016) or a study from the United Kingdom (Strielkowski et al., 2017). The rapidly declining costs indicate a major shift from the central network topology to a highly distributed one, where power is generated and consumed directly at the grid boundaries (Aberilla et al., 2020; Van Summeren et al., 2020). Another problem is that telecom costs may be prohibitive for the full support of smart grids (Rehmani et al., 2018). The network frequency could be used to transmit load information without the need for an additional telecommunications network but would not support economic negotiations or quantification of contributions. The smart grid requires not only the technology, but also the cooperation of the electricity suppliers, their tariff payers and, above all, the regulatory authorities in order to become very efficient and effective (Chawla et al., 2020; Yanine et al., 2020). In addition, it also requires a full-fledged cooperation of all consumers of energy. The whole situation becomes even more complex with the introduction of the renewable energy sources and the renewable energy generation as a part of the great leap towards the green economy and low-carbon economy (Peng and Poudineh, 2019; Elavarasan et al., 2020). In order to make the picture complete, one has to bring in the factor of self-generation of renewable energy by the consumers who become the prosumers of energy (both consumers and producers of energy from the renewable sources who can trade the energy back to the grid or among themselves using peer-to-peer (P2P) networks and technological solutions) (Sousa et al., 2019; Wang et al., 2020).
This paper focuses on the optimal management of the demand-side response that can be achieved through the introduction of the fifth generation (5G) wireless networks in the future renewable energy systems. Effective demand response allows energy to be directed to the areas and businesses where it most needed in order to avoid peak hours which is crucial in the case of RES that are distinguished by intermittency (dependency on the nature’s conditions and day times) (Rayati et al., 2019; Ju et al., 2020).
The main contribution of our paper is in demonstrating that 5G wireless networks might help to efficiently manage and enhance the demand-side response in the high-renewables energy systems and smart grids using a comprehensive evidence from the research literature and other sources which is meaningful and worth research efforts (see e.g., Xu et al., 2019; Sakib et al., 2020). Furthermore, the paper provides a comparison between advantages and challenges of 5G networks in demand-response renewable energy grids. In addition, the paper devotes a great deal of effort on discussing the role and the place of 5G networks in the future of the demand response and use the existing knowledge and evidence in order to outline its main advantages and disadvantages.
The layout of this paper can be presented as such: Introduction open the discussion, provides main points, lists the main contributions of this paper and offers the paper’s layout. Literature Review provides a comprehensive literature review of using data in energy technologies and managing these technologies in the most effective ways with the help of the Internet and communications solutions. Demand Side Management Strategies outlines the theoretical framework for the demand-side management strategies. Renewable Energy Sources and Demand-Side Response explains the implications for the high-renewables penetration in the smart grids and demand-side response management. Fifth Generation Wireless Networks in the Internet of Energy provides an overview of the fifth-generation wireless networks in the IoE. Advantages and Challenges of 5G Networks in Managing Demand Response comes up with the advantages and disadvantages of the fifth generation (5G) wireless networks and the Internet of Energy (IoE) in managing smart energy grids based on RES using demand-side response. Finally, Conclusion concludes with general outcomes, implications, and pathways for further research.
Literature Review
In general, one would probably agree with the fact that the optimal management of demand-side response appears to be crucial for smart grids that encompass the electrical equipment in combination with such novel elements as electric vehicles (EV) and the use of smart grid technology (Eseye et al., 2019; Wu et al., 2019; Venegas et al., 2021). New technologies will enable customers to move from event-based demand response, where the supplier demands load relief, to the 24/7-based demand response, where customers request peak load and see an incentive to control the load at all times. Customers who traditionally pay fixed tariffs for energy consumption (kWh) or peak load can adjust their consumption and request to take advantage of fluctuating prices.
Artificial intelligence (AI), in conjunction with advanced energy generation and energy storage technologies, has proven its potential in managing energy production and consumption, as well as energy supply and demand (Alreshidi, 2019; Pinto et al., 2019; Ji et al., 2020; Shi et al., 2020). This will improve the ability to understand the real conditions of the energy market, such as demand and supply, and to make very accurate predictions and simulations (Ma and Zhai, 2019; Márquez et al., 2020; Ngarambe et al., 2020). Such circumstances which can obviously have cascading effects on the entire regional network and pose a difficult task for utilities, could be avoided. With the recent commitments to decarbonization many governments declare all around the world, as well as decentralization and the use of novel energy saving technologies, artificial intelligence is being used to address the imbalance between demand and supply caused by the growing share of renewable energy sources (Gomes et al., 2019; Bañales, 2020; Miglani et al., 2020). Another challenge is decentralized electricity generation, where private users generate and consume their own electricity (therefore becoming the “prosumers” – both producers and consumers in one), but this is also a challenge due to the lack of control over the supply and demand of energy (Gissey et al., 2019; Riveros et al., 2019; Roldán-Blay et al., 2019; Croce et al., 2020). Since 2010, the use of solar energy increased by several times (with China being the current leader in both the production of solar panels and generating solar energy), and this trend is expected to continue as appliances generate electricity from sunlight, reduce costs, and increase efficiency (Bastida et al., 2019; Raina and Sinha, 2019; Rabaia et al., 2020). Utilities buy surplus energy from private consumers, who produce more electricity than they consume, and send it back to the grid (Cui et al., 2017; Burgess et al., 2020). However, the current systems are generally not designed to take this into account, but there is a fundamental problem represented by the human error caused by the incomplete information and rational inattention paradigm (Matejka and McKay, 2015; Sinclair and Barense, 2019). Humans make mistakes and bad decisions, and although some of the more avoidable, artificial intelligence and machine learning can be used to consider how this will adversely affect the energy savings achieved by investing in new energy efficiency programs (Konstantakopoulos et al., 2019; Mehmood et al., 2019). This is an important step towards overcoming one of the fundamental problems of human error. This has the potential to make major events affecting the power grid, such as a major earthquake or explosion in a nuclear power plant, more predictable and efficient (Eskandarpour and Khodaei, 2016; Lu et al., 2019; Lee et al., 2020). The smart grid could be used to divert energy from plants and installations, manage multiple incoming energy sources, and adjust energy production to increase efficiency (Munshi and Yasser, 2017; Tronchin et al., 2018). Electricity grid operators also collect data that require continuous monitoring, analysis and interpretation to ensure that electricity supply meets demand and that different contributions from different sources, such as wind and solar, are met (Yen et al., 2019; Chamandoust et al., 2020). In their turn, wind farm operators also collect information about the quantity and energy content of wind turbines exported to the grid (Pei et al., 2018; Long et al., 2020). Dedicated AI can eliminate this data analysis through the use of machine learning, artificial intelligence (AI), and deep learning. The use of AI technology has had a decisive impact on the development of smart grids and their use in energy production and contribute to the complex optimization (Bhowmik et al., 2017; Soares et al., 2018). Network operators use AI software to assess the thousands of variables involved in energy distribution and consumption. Examples of this are the wind and solar industries which were developed to support the development of smart grids to maximize energy production on cloudy and windless days (Sarker et al., 2020; Wu et al., 2020). However, characterizing this technological innovation which is, after all, the core competence of the energy sector, may not be as damaging as it seems at first sight. Germany, for example, uses a machine learning and early warning system that uses a complex algorithm to predict the amount of energy generated over the next 2 days (Ghoddusi et al., 2019; Wood, 2019). It analyses fluctuating demand and changing weather conditions in order not only to predict energy demand, but also to anticipate potential problems in the grid and to propose ways to improve weaknesses in distribution lines. In addition, similar to the German example, AI can be used to analyze data from various sources such as wind, solar, wind turbines and wind farms to make informed real-time decisions to maximize demand flexibility (Adedipe et al., 2020; Sweeney et al., 2020). Other ways in which artificial intelligence can facilitate demand-side response and demand flexibility include using game-theory algorithms to create incentives to improve aggregate participation and using blockchain and other distributed ledger technologies to protect data (Ahl et al., 2019; Tsao et al., 2020). It is possible to create incentives for consumers to participate in demand-side response management initiatives in their local markets. As energy storage and artificial intelligence improve the accessibility of energy sources, they will play an important role in energy production and consumption in the future. Furthermore, advances in machine-learning systems, such as artificial intelligence, will revolutionize the demand and supply of energy in our economy (Li et al., 2019; Jose et al., 2020). This is due to the capability of the smart grids and energy storage systems to effectively collect, analyze, and synthesize various data from a wide range of sources such as wind and solar, natural gas and coal to make decisions about the best allocation of energy resources. Given the various challenges we face, one of the keys to sustainable and reliable solutions seems to be smart energy storage, where artificial intelligence will have been the brain.
With regard to the above, the demand response can and might be altered and steered using the AI and smart grids which bring many comparative advantages. Among the most obvious features are: 1) practicality; 2) advantages; 3) security and data protection; and 4) reliability of DR power systems (Di Santo et al., 2018; Shareef et al., 2018; Tsaousoglou et al., 2020). Another issue of the demand-side response and the smart grids is the economies of scale that might require the use of smart meters and smart plugs to control electric devices and appliances (De Wildt et al., 2019; Langendahl et al., 2019). A smart meter can control electrical appliances by evaluating the performance of appliances to optimize power consumption in case of certain limitations. The plug can also control the total power consumption of the device by adjusting the electricity price in a place where it is calculated in advance by the utility and sent to the smart meter days in advance in real time. Algorithm architecture can be designed to bridge the benefits DR can bring to the electricity grid by using the underlying information infrastructure of the smart grid. Therefore, implementing this algorithm has the potential to minimize electricity prices by optimizing electricity consumption during the hours of distribution in the grid to detect parts of the grid (Brinkel et al., 2020; Das et al., 2020).
It goes without saying that energy costs can be saved by using the smart grid as a platform for managing energy consumption and energy efficiency while taking into account uncertainty in electricity prices and weather information (Worighi et al., 2019; Rathor and Saxena, 2020). Customers are encouraged to switch from higher peak hours to lower (and therefore cheaper) peak hours, or simply reduce peak hours. Time-prices can vary intelligently, and appliances that are used in this system can cause significant energy costs-saves customers. It is a smart business for utilities to reduce customers electricity consumption when demand for electricity has peaked or when there is a balancing problem in the grid (Oprea et al., 2018). Utilities buy electricity from other utilities and heat it - and generate equipment and supply customers when they need it. Thence, utilities send signals to a central control unit that uses the flexibility of the application to reduce their customer’s energy consumption (Eid et al., 2016; Morstyn et al., 2018). As an essential element of an intelligent grid, users participating in the electricity market-oriented DR enables heterogeneous end users can acquire tailor-made utilities by coordinating interactions with utilities (Sharifi et al., 2019). Thence, demand-response programs play an important role in influencing the energy consumption of end users (Alasseri et al., 2020). Utilities offer demand-side response services to improve customer satisfaction, and the amount of electricity customers demand influences demand response policies. The demand response aims to adjust demand for electricity rather than adapt supply. Customers can adjust electricity demand to shift tasks that require large amounts of electricity, shift some of their consumption to alternative sources, or choose to pay a higher price for their electricity. Utilities can signal demand needs to their customers in a number of ways, including “electricity is cheap” or “smart metering,” where customers can be notified of an explicit price change. In this way, utilities should constantly balance the supply and demand of electricity across the network, from generation to consumption, balancing both demand and supply in a smart grid (Kakran and Chanana, 2018). One of the ways to do this is precisely through demand response, where price mechanisms, including incentives, are used to encourage consumers, particularly in industry, to change consumption patterns in times of high demand. Another approach is virtual power plants, which bring decentralized generation facilities such as industrial generation clusters (including photovoltaic generators) to the grid in case of higher demand, or “virtual power plant systems” (Guerrero et al., 2019). This brings greater flexibility to delivery, but also requires more complex communication, increases the complexity of management and control, and further complicates supply chain management. The last approach integrates command and control infrastructure with transmission, distribution and control of the infrastructure (Galbusera et al., 2020; Shrestha et al., 2020). Here, the demand response management solution approach is emerging as a critical infrastructure component linked to the transmission and distribution infrastructure and the supply chain.
Moreover, an additional discussion is needed here concerning the field where the bigger opportunity for 5G emerges, which is in the Internet of Vehicles (IoV) market (Zhou et al., 2020; Tufail et al., 2021). It goes without saying that communication improvement in charging stations or among charging stations, as well as in vehicles and between vehicles and other objects might have a favorable impact on the development of the renewable energy (Li et al., 2020; Rajper and Albrecht, 2020; Savari et al., 2020).
With regard to the above, 5G mobile networks (5G) are considered to be on the way to widespread ubiquity at the IoV market and the essential part of the “vehicles-to-everything” concept. Vehicle-to-everything (V2X) means vehicles communicating with more vehicles than vehicle to vehicle (V2V) or the vehicle to infrastructure (V2I) (Storck and Duarte-Figueiredo, 2020; Yang et al., 2021). The concept includes V2X technologies include communication between the vehicle, the infrastructure and other road users thus allowing the moving vehicles to communicate with moving parts of the traffic system around them in real time (Fallgren et al., 2018; Hakeem et al., 2020). In the connected automotive industry, solutions are based on a variety of communication technologies, including DRCS (dedicated short-distance communication), Wi-Fi, GPS and mobile communications. This type of connection is abbreviated as V2N (Vehicle to network communication)and it effectively enables a form of direct wireless communication when needed Căilean and Dimian, 2017; Luo et al., 2020). This means that communication takes place using the same cellular network technology that mobile phones use. Thus, using 5G networks is going to make automakers, governments and the aftermarket to be capable of putting connected vehicles with cellular and V2X communication on the road (Han et al., 2020). As assisted driving progresses towards autonomous vehicles, 5G communication will therefore play a key role. Autonomous vehicles will not need external connectivity with other vehicle infrastructures, such as the cloud or the Internet. Cars produce a vast amount of data, and a connected autonomous vehicle can generate up to 5 TB of data per hour (Miller, 2020). 5G mobile connectivity is becoming the key technology for the construction of autonomous vehicles since it will enable fully automated driving. Together with 5G, the V2X ecosystem will be able to improve the driving experience and open up new horizons for automotive companies. V2X technology will enable the millions of connected cars sold worldwide to communicate with the infrastructure in the cloud and among themselves. High-speed communication, continuous network connectivity and rapid response will pave the way for smart cars (Zhao et al., 2018).
One of the main advantages of 5G/V2X technology is that it does not depend on traditional mobile coverage. For the first time, we are attempting to use coherent beamforming technology to establish a communication network between the Internet of Vehicle (IOV) and the 5G network. The answer is that 5G/V2X technology allows communication between the car and the infrastructure around them, regardless of the range. Instead of connecting to the cloud, 5G V2X can communicate between nearby vehicles and nearby infrastructure (Husain et al., 2019; Abbasi et al., 2021).
The vehicles are equipped with mobile router-on-board units to connect them to the LTE network by detecting their position and dynamic parameters. Each vehicle employs a graphical user interface which makes it possible to visualize the information from the mobile router and thus test jointly developed use cases (Ndikumana et al., 2020). Its spectrum can be used independent of mobile networks for direct communication 5G and V2X side links using traditional licensed frequencies, while the second transmission mode provides in-vehicle communication (V2N), mobile broadband and voice telephony. Direct communication modes include vehicle-to-truck (V2V), vehicle-to-infrastructure (V2I) and vehicle-to-pedestrian (V2P), increasing the range and reliability of communication (Chen et al., 2017; Khan and Lee, 2020). V2X could complement the car sensors with more data from traffic signals, road construction and emergency vehicles to send clear signals to cars that can overtake them if a fire truck or ambulance wants to pass. Based on the discussion above, one can predict that with the help of 5G networks and IoV, cities could do away with traditional traffic lights altogether in the nearest future, which could accommodate millions of cars, and help the regulators and policymakers to start introducing them in all new cars much faster.
All in all, one can see how the demand response is becoming increasingly important in the smart grid and in many other areas of the network in the recent years. This is critical to the operation of many electricity suppliers, as it is a key element of the decision that the customers can make to potentially reduce their energy consumption. Recent innovations, such as 5G wireless networks and peer-to-peer prosumer energy exchange are likely to boost its development further. This is discussed in the next section that follow.
Demand Side Management Strategies
Energy efficiency differs from the response to demand because it produces a measurable reduction in consumption over the course of a year and not just a reduction in consumption for selected hours in a given year (Cooremans and Schönenberger, 2019; Hou et al., 2019). A large commercial or industrial enterprise could probably invest in improving energy efficiency and offer the savings from the project as a resource on the capacity market.
Demand-side response leads to the adjustment of power consumption for keeping the power system in a balanced state. This can be expressed using the following equations (see Eqs. 1–3). First, let us express the functions of the balanced state that can be written as follows Eq. 1:
where:
PG(f) - generation power related to the system frequency, PC(f) - consumption power related to the system frequency.
The more advanced state of the power system can then be defined using the following equation (see Eq. 2):
where:
∆PG – an increase in power generation output, ∆PC – an increase in consumption power output.
Finally, one should increase the load flexibility to system power that includes the demand-side response backed up by power generators, photovoltaic solar panels, energy storages (e.g., Tesla PowerWall household energy storage device), electric vehicles, or wind turbines (see Eq. 3):
where:
∆PDRG - generation power from onsite standby energies.
∆PDRC - adjustment power by flexible loads.
Demand side response is a characterization of certain programs that specifically reduce peak load in order to shift the use of electricity from peak hours (Azarova et al., 2020). The technology gives consumers the opportunity to be paid by the responsible system operators in the same way as a generator is remunerated. Typically, demand respondents use distributed power generation strategies or power management controls to reduce their demand for operator-provided price signals (Dominković et al., 2020). This is where IoE is required to react promptly to price signals and to mitigate the demand for electricity.
There are three layers of IoE in smart grids covering management services, namely the perceptual, network and application levels (Mrabet et al., 2020). The perceptual layer is the physical layer that has sensors for collecting and collecting information about the environment. It detects some physical parameters or identifies other intelligent objects in the environment. Then there is a network layer is responsible for connecting to other intelligent things, network devices, and servers. Its functions are also used to transmit and process sensor data. Finally, there is the application layer is responsible for providing application-specific services to the users.
In general, the cost of compliance with renewable portfolio standards averaged less than one percent of retail electricity prices (Zhou and Solomon 2020). Although these costs are relatively low in themselves it should be noted that these estimates often fail to take into account the more general social benefits of using renewable energy. Quantifying these benefits can be even more difficult than quantifying the costs. However, most experts agree that reducing pollution, conserving water, diversifying fuel sources, stabilizing electricity prices and promoting wider economic development are of considerable value (Aryanpur et al., 2019; Malav et al., 2020). Of course, there is plenty of room for discussion, and further studies and approaches would be useful. However, the relatively wide range of estimates also provides an excellent opportunity: there is a good ground for good policy and regulation to promote better implementation of renewable standards. In addition, the sharp fall in the price of renewable energy generation should help to further reduce its costs, provided that the integration cost of variable energy generation can be maintained at a relatively low level (Schmid et al., 2019; O'Ryan et al., 2020). Demand side management strategies need to address some investment costs at various implementation levels. However, the connection between investment costs and implementation time follows. Obviously, the inclusion of technology (e.g., efficient provision of household appliances, smart grid) in achieving demand-side management costs is more important than any other strategy (Iris and Lam, 2019; Khan, 2019).
With regard to the above, it seems important to note that an effective demand-side response is based on the properly calibrated and interconnected smart meters and similar devices that function in a well-balanced network. Efficient protocols are required to effectively manage the failure, configuration, billing, performance, and security aspects of these devices. Similar efforts are being made for the smart city systems to provide standard mechanisms and services for the efficient management and control of device communication at the various levels of architecture (Khattak et al., 2019; Sengan et al., 2020; Strielkowski et al., 2020).
Therefore, it is apparent that the future energy balance would be based on the optimalization of power usage during the day in order to avoid the peaks that occur in the mornings and evenings. Moreover, it would also be centered around the energy efficiency that would allow to save vast amounts of energy using smart meters and devices. These devices would be equipped with the AI-based algorithms that would help to achieve the perfect balance of energy saving and consumption. In addition, solution such as smartphone apps tailored for energy users might become a breakthrough in helping them to monitor and control their energy intake. Some apps would also help the users who would produce their own electricity to find potential buyers or to sell it back to the grid.
When it comes to industry, it is easy to see how the effective and digitalized demand-side response might be of a great help there. Smart meters and sensors attached to various industrial machines might become an effective way how to collect and analyze vast amounts of data (Big Data actually when large industrial enterprise come to mind) for improving the efficiency and minimizing energy losses, while AI solutions would become a powerful tool for processing these data in real time (Rausser et al., 2018). All of that would lead to the creation of the balanced and intelligent smart grid of the future that would change the ways the energy is produced, distributed, and consumed today.
Renewable Energy Sources and Demand-Side Response
As it was shown above, the demand response includes all intentional changes in the power usage patterns of the consumers of electricity that envisages altering the patters the power is consumer including the timing throughout the day or the total demand. This can be achieved using novel approaches to handling the energy meters in households and industries in response to certain conditions within the power grid (e.g., peak power congestion or high prices), including the above-mentioned demand-side response. Dynamic demand also means advancing or delaying the operating cycles of the device in order to alter the consumption of energy. This is achieved through controlling the power in the grid and balancing the power discrepancies by subsequently turning on and off intermittent loads in appropriate time intervals. All of this depends on the consumption patterns of energy users that have its own regularities tied to the daily routine.
Let us show this using the data obtained by the Customer-Led Network Revolution project (CLNR) in the selected households Northern England (CLNR, 2013). The data were obtained during a time span of 4 years from 2012 until 2015 using the smart meter readings from 155 households with and without solar PV (see Figures 1, 2).
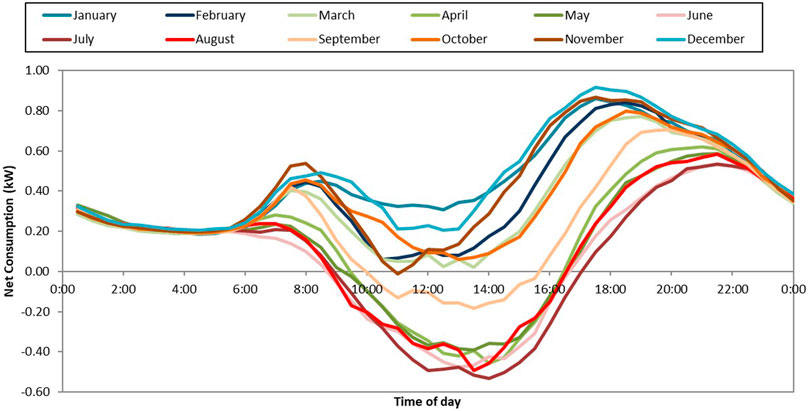
FIGURE 1. Load profile of a typical household (half-hourly net consumption averaged across all customers in the group averaged across all days in each month, kW). Source: Own results based on CLNR (2013).
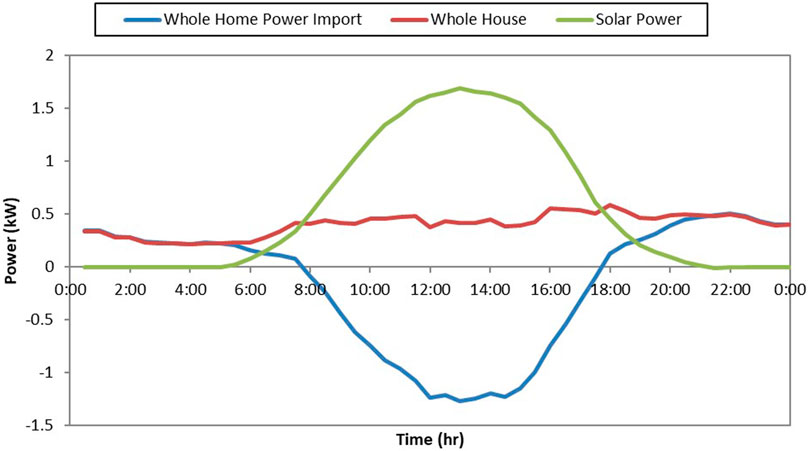
FIGURE 2. Peak solar power generation and import on a typical day (half-hourly measures for a PV household, kW). Source: Own results based on CLNR (2013).
From the Figures 1, 2 it becomes quite clear that the peaks in electricity consumption happen in the morning and in the evening and there is a drop in the electricity consumption during the day. This is, however, is an issue because (as Figure 2 demonstrates), this is the time when most of the solar energy is produced through the photovoltaic solar batteries. This is where the demand-side response is required in order to meet the requirements of the renewable energy generation, transmission and consumption.
In general, there is a variety of theoretical models that play with the demand response impacts on various infrastructures that include renewable energy sources such as solar, wind, and hydro. Typically, the demand response strategy with the load modelling is applied for improving operational flexibility (Huang et al., 2019). This can be achieved by using deterministic stochastic target functions that should include factors such as price, demand, as well as the amounts of generated power. Another important factor that needs to be considered is a storage-based demand response that can manipulate with the energy storage devices in order to supply the required power during the peaks so that the consumers have a constant supply of electricity without having to cut their demand or compromise their comfort. Another bonus of all that is that the total energy bill is reduced while the penetration of renewable energy sources is increased. Within this framework, network operators have the ability to choose their location and production management for renewable energy in their regions so they can decide regionally when a cutback or storage will occur to minimize supply and demand gaps. A mindset to maximize renewable energy production and avoid downsizing made sense when variable renewable energy was extremely expensive and fixing solutions even more expensive. However, as the recent and projected savings in the renewable energy cost, grid management and storage systems are changing the optimal solution package, starting with the superstructure of, for example, solar panels and wind turbines. Due to their low cost of ownership, new renewable capacity often displaces more expensive generators on the grid and lowers wholesale clearing prices. The combination of inconsistent supply of renewable energy sources and hourly electricity demand, coupled with inflexible heat generation, can significantly reduce RES revenues and undermine their potential to increase market share. All around the world, renewable energy revenues are falling due to increased adoption of renewables, grid congestion, and the inflexibility of other generators. In addition, one has to take into account the factor of the geographical distribution and climate zones where, for example, wind and solar power generation is more optimal than in the other locations. Information technology would enable us to exchange precise information signals and conduct precise coordination and flows of energy from its production to its consumption.
Fifth Generation Wireless Networks in the Internet of Energy
With all the discussion above, it becomes apparent that fifth generation (5G) wireless networks might become a solution to the issues such as the intermittency of the renewable energy generation and the effective control over energy efficiency and saving (Dragičević et al., 2019; Nižetić et al., 2019; Zahed et al., 2020). Even now, at their infancy, it is expected that 5G networks are going to contribute trillions to the global economy by creating the conditions for new advances in productivity and innovation (Khan et al., 2019; Forge and Vu, 2020). 5G networks will be able to exploit the full potential of Internet of Energy (IoE) by introducing the technology that employs such ubiquitous devices as smartphones, tablets, or smart electric vehicles. This means that large amounts of sensor data from devices such as drones and tractors could be transferred to cloud computing resources.
In addition to reducing costs, coupling 5G networks with renewable energy will also reduce the telecommunications industry’s carbon footprint by eliminating its dependence on fossil fuels. The energy market is developing a warmer and more productive relationship with the Internet of Things, and the addition of 5G will improve usability and utility of the Internet of Things by making our homes and. networks smarter and more energy efficient. Tying renewable energy to the telecommunications sector is something that industry experts exploit and grow. All energy consuming appliances have a unique power demand and as our technology becomes smarter and easier to connect, it is becoming increasingly difficult to. locate areas of industrial waste. Business-level insights into energy use are a good thing, as they help to distribute demand peaks and identify opportunities for investment in more efficient infrastructure. The combination of digitised power grid, 5G and IoT at the same time will help customers monitor their own energy consumption and save energy. Utilities and. consumers will have real-time access to critical energy information from mobile devices. For this reason, 5G will bring significant changes to society, as it relies on an interconnected wireless network.
Here, also one interesting question emerges: how can 5G improve renewable energy trade market? In addition to the peer-to-peer (P2P) energy trading markets that would involve not only energy companies but also prosumers, 5G networks would also help to reduce the costs, coupling 5G networks with renewable energy that would also reduce the telecommunications industry’s carbon footprint by eliminating its dependence on fossil fuels (Hsu et al., 2020; Israr et al., 2020). As the energy market is developing a more productive relationship with the IoT, the addition of 5G will improve usability and utility of the IoT by making households and networks smarter and more energy efficient. Tying renewable energy to the telecommunications sector is something that industry experts would welcome. All energy consuming appliances have a unique power demand and as our technology becomes smarter and easier to connect, it is becoming increasingly difficult to locate areas of industrial waste. Business-level insights into energy use are a good thing, as they help to distribute demand peaks and identify opportunities for investment in more efficient infrastructure. The combination of digitised power grid, 5G and IoT at the same time will help customers monitor their own energy consumption and save energy (Wu et al., 2021). Utilities and consumers will have real-time access to critical energy information from mobile devices. For this reason, 5G will bring significant changes to society, as it relies on an interconnected wireless network. This transformation will enable utilities to establish themselves as leaders in efficient and clean energy production. By improving energy distribution through smart grids, utilities can manage voltage fluctuations, which will help reduce power losses. Homeowners would be able to use their smartphones to access smart meters to monitor their daily energy use. And the electricity grids would enable data communication at higher voltage levels between customer buildings and smart meters using faster 5G networks.
Supporters of super-fast networks promise that faster data speeds will transform the way people interact with the Internet, foster self-driving car initiatives, and improve everything from health care to tourism. Such speed and connectivity increases will facilitate data fusion and improve situational awareness, improve command and control capabilities, while providing a significant operational advantage in future battlefields. 5G networks will not be able to meet the enormous demands of the growth of machine-to-machine communication. With population growth and increasing mobile penetration, capacity is one of the most prominent benefits touted. The number of devices connected to the Internet is increasing exponentially, leading to an increase in bandwidth and energy consumption. As more and more data are generated online and consumed, existing spectrum bands will struggle to cope, and congested areas will often be of poor quality. Figure 3 that follows, provides a timeline of wireless networks from 1G to 5G that took 40 years in total.
From the Figure 1 above, one can see that 5G technology was developed to deal with limitations that previous generations of networks could not sustain. While 4G and LTE support peak speeds of up to 100 gigabits per second (G/LTE), but are not immune to signal interference, 5G is the fifth generation of mobile networks that promises higher speeds and lower latency (Mannweiler et al., 2020). In order for it to meet bandwidth needs and network-connected industrial equipment such as mobile phones, tablets, computers and other devices. Currently, 5G is still quite difficult to define and there is still a long way to go before it is fully implemented, but one thing is certain: it will have an impact on energy market of the future (Kim et al., 2019). And when it comes to the 5G in the global race, there will be a lot of pressure on countries to adopt the new connectivity technology. Over the next few years, 5G will have a much greater impact and disrupt the current mobile ecosystem. Wireless technology stands for improving the consumer experience of mobile technology by offering faster speeds and more reliable connectivity. It is the key to accelerating machine-machine interaction, faster response time and data exchange, and improving automation capacities. Its cloud-based technology enables connected devices to stream anywhere in the world, from the cloud to mobile phones, tablets, computers and other devices. This technology is crucial to realise its potential, as it is the platform that will serve as the basis for tomorrow’s economy, and governments play an important role. The new 5G standard promises a transformational technology that is crucial to enabling the next industrial revolution. In addition, it will contribute trillions to the global economy and create the conditions for new advances in productivity and innovation. All in all, it seems that 5G will form the basis for future economic development and national competitiveness, including certain military applications. It is interesting to note that the United States has failed to seize this opportunity to meet the challenges of 5G technology and has lost a crucial competitive advantage. Today, China seems poised to become the first country in the 5G to spur innovation, but the United States has not yet done so. The next generation of mobile communications will inspire the emergence of new industries, and existing companies will need to develop products and services around the next generation of mobile communications. Technological inventions inspired by 5G will lead to smarter, more efficient, and cheaper products, services and technologies for existing companies and will revolutionize the energy market and smart grids. The evolution from a pure network to a robust ecosystem will be the result of the convergence of new 5G technologies that build on current cloud technologies - processed analytics and analytics technologies such as those used in cloud computing, cloud storage, mobile data storage, and data analysis, while developing some unique data insights. Because technology affects almost every aspect of life, all countries around the world have to keep pace with technological developments to improve the lives of their citizens as they evolve in the global economy. This next-generation technology offers the opportunity to share the spectrum with a larger number of wireless users and support energy applications that require greater capacity. 5G technology could prove fundamental to smart cities, and the high throughput of 5G could handle data loads transmitted at speeds of up to 100 gigabits per second (Gbps) or more which could have a significant benefit for the future energy market with a high share of renewables and IoE-like solutions. All of this is not about the current non-5G communication system in smart grid resulting in the waste of electricity. It is about 5G networks making the communication faster and more efficient. According to industry experts, 5G networks will be 90% more energy efficient (and twice as fast as 4G LTE today) than previous network technologies of the current generation (Flynn, 2021).
Figure 4 that follows demonstrates the design of the demand-side response and 5G wireless networks in the future renewable energy systems. It demonstrates how the 5G wireless networks will become the centre of the coordination between the generation of renewable energy and its transmission and consumption. Moreover, it also reflects upon the effective management of demand-side response through the signals sent across the network from the energy generators to the energy consumers (as well as prosumers).
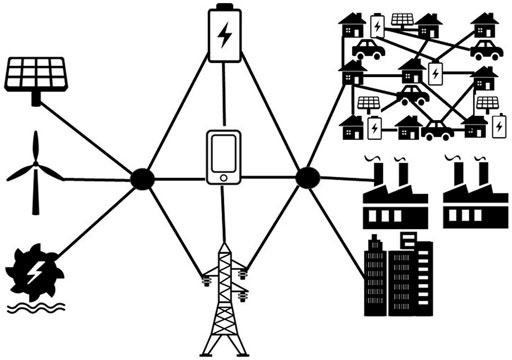
FIGURE 4. The design of the demand-side response and 5G wireless networks in the future renewable energy systems. Source: Own results.
The successful introduction of smart meters that would constitute the backbone of the IoE and intelligent demand response will depend on the choice of communication technologies, however likely the use of 5G wireless networks and other communication technologies may be. Unfortunately, there is currently no consensus on which communication technology meets the needs. There are many different types of communication technologies available for smart grids, and sometimes more than one technology is used for the same use. The choice of the most suitable technology is strongly influenced by the availability of network capacity, network bandwidth and other factors such as the size of a network and the number of users. In the energy and utilities sectors, 5G is a technology particularly suited to the deployment of smart meters, smart grid systems and smart grids due to its advanced capabilities. Green Power investigates the development of IoT technologies in the electricity and energy market, with a particular focus on the introduction of wireless 5G networks. Wireless communication technology can be fully satisfied by wired communication technologies such as mobile phones, tablets, computers and mobile phones. However, most utilities typically have power and grid monitoring systems based on wired communication technologies (e.g., smart meters, smart thermostats, etc.), while wireless communication is limited to less mission-critical applications. The use of a previously static top-down network has become an increasingly important part of a smart grid’s lifecycle, and a comprehensive communication solution is needed to support it. Specific fixed-line communications solutions are typically based on a single-channel high-speed and latency network with limited bandwidth. Wireless solutions are necessary due to the distribution of the network and its size and are therefore imperative for use in smart grid applications such as smart meters, smart thermostats and smart lighting. Today, communication environments can be built wirelessly, but integrating protection and communication within them may not be feasible. 5G wireless communication networks could be used to cover a small geographical area, such as a substation or building surrounded by infrastructure, and for example cover a large area of the network, such as the substations and buildings surrounding the infrastructure. Its low latency and high reliability could also provide the opportunity to create redundancy in fixed communications. Smart grids combine traditional networks with communication and information technologies that are characterised by efficiency, cleanliness, security and privacy. Smart grid solutions are evolving to cope with distributed energy resources with interactive load management, which requires advanced communication technologies for real-time control and protection applications. As the demand for energy continues to increase due to the increasing use of renewable energy sources by customers, the electricity system of the future will face new challenges when it comes to balancing the electricity flows in the grid. Part of this intermittent generation is intermittent, and networks require integrated monitoring and control to manage different energy flows and plan complementary standby capacities. Here, 5G is becoming a key element to enable networks to better adapt to dynamic renewable energies and decentralised generation. The main challenge is to balance the dynamic conditions between supply and demand and to enable the grid to redirect electricity in the event of local outages. The basic problem, particularly in decentralised generation systems, is that communication and network infrastructure are a key factor in monitoring a network. The management and control of the future network requires the ability to collect information from a wide range of sensor devices and actuators. This allows wirelessly connected devices to remotely detect, monitor and adjust power consumption and power consumption. 5G will be a catalyst enabling the network to deliver the throughput and ultra-low latency required for high-speed communication between smart devices and smart grid systems.
Advantages and Challenges of 5G Networks in Managing Demand Response
Overall, 5G networks will likely play an important role in managing demand response in the future energy networks. Because device information is consumed by a cloud-based platform, the integration and sharing of information can be simplified using software applications running on the cloud platform. The cloud platform is becoming a data lake that various applications can use. An intelligent energy management system keeps the grid stable by balancing the power generated from all sources with the power consumed. An IoE enables consumers and prosumers to independently coordinate supply and demand and is equipped with intelligent forecasting systems that use weather forecasts, expected traffic flows and other information to predict future energy needs.
Let us attempt to summarize the advantages and disadvantages of the implementation of 5G networks to the smart grids with a high share of renewables using the demand-side response. This summary is based on a vast body of literature analyzed earlier in this paper (Literature Review) and the line of reasoning presented in the sections that followed. Table 1 that follows outlines the advantages and challenges of 5G networks used for enhancing the demand-response renewable energy grids.
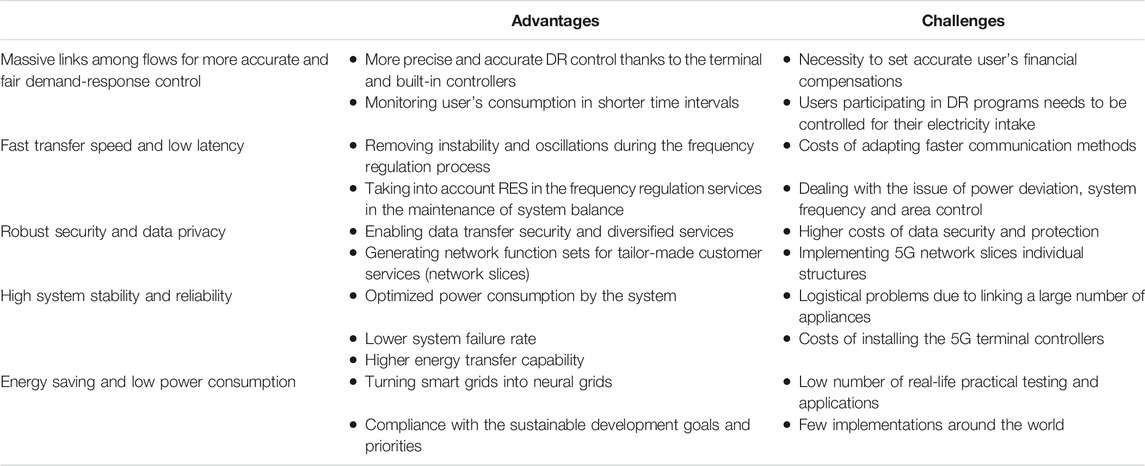
TABLE 1. Advantages and challenges of 5G networks in demand-response renewable energy grids. Source: Own results.
From Table 1, one can see that the advantages of the 5G networks in demand-response renewable energy grids feature such aspects as the massive links among flows for more accurate and fair demand-response control, fast transfer speed and low latency, robust security and data privacy, high system stability and reliability, as well as the energy saving and low power consumption. The disadvantages are not numerous and mostly concentrate on financial and logistical problems, as well as limited experience with using 5G networks and associated techniques.
Moreover, with regard to the 5G wireless networks in the energy sector, it is apparent that a highly collaborative infrastructure featuring several layers of access and security might be the safest and the most optimal for all involved players. In addition, various vulnerabilities of digital and technical systems that include all types of devices, smart meters and sensors, as well as a wide range of control systems should also be tackled and taken care of. This is due to the fact that IoE is not immune to attacks and a good system should be developed with security in mind. Security architecture and policies, the potentially beneficial security strategy, the ubiquity of security, the security devices, wireless security, the risk of personal data with the IoE and system redundancy, and ensuring security are all important for the proper functioning of IoE.
The increased number of connected devices and the amount of data they generate increase the security requirement for that data. Ioe networks and mobile cellular networks in terms of requirements such as the need for a long battery life, the support of a large number of devices, the scalability of the network, low equipment and deployment costs and extended coverage. Future opportunities and challenges for IoT and IoE have been addressed in particular by the security and privacy risks associated with using the smartphone on these networks and possible countermeasures.
Smart energy grids offer sustainable solutions that cover the entire energy value chain from energy generation through to its transformation to the final consumers. In addition, they employ the advances in the modern information and communications technologies (ICT) and the Internet of Energy (IoE) which offer artificial intelligence (AI)-based solutions for matching energy production and energy consumption in the future sustainable energy systems. However, the transition to these smart grids might require certain forms of governments funding and incentives that would support the willingness to shift to the renewable energy sources and to participate in their generation and trading (i.e., by becoming effective prosumers on the market of power and energy). In addition, policymakers and stakeholders should promote the effective management of demand-side response through energy saving and energy efficiency. This can be achieved through some monetary and non-monetary incentives, effective monitoring and control of energy consumption using smart meters and trackers, as well as through the public relations campaigns aimed at raising environmental awareness and enhancing sustainable education.
When it comes to the issue how 5G can work on legacy communication system of smart grid, the result of the integrated use of digital technologies in the electricity grid is less redundancy in the transmission and distribution lines and higher utilization of the generators, resulting in lower electricity prices. 5G technology has the capability to increase the value-added flow of intelligent networks, including value-added innovative services and market mechanisms for a value-added flow of information. The most important applications of 5G smart grid operation can be the predictive maintenance of various components of the power grid such as electrical insulation, transmission lines, power grids, LNG gas storage, proactive recovery in emergency situations, natural disaster monitoring and real-time video surveillance. In the conclusions section that follows, we also provide an overview of the major challenges of 5G networks in demand-response renewable energy grids.
Conclusion
Overall, it becomes apparent that the introduction of the fully operational and functional 5G wireless networks is very likely to increase the economic wellbeing and to help the transition to the carbon-free energy systems helping the deployment of the renewable energy worldwide. It also becomes clear that 5G wireless standard would provide a solution for the growing data requirements including some novel important technologies such as the augmented reality, connected electric vehicles (the IoVc concept), distributed energy resources, peer-to-peer (P2P) energy networks, as well as the Internet of Energy (IoE). By offering all of the above, 5G wireless networks would also become an important element of the demand-side response mitigation and management.
Our results provide new insights about the implementation of IoE practices. In particular, we look into the novel and exciting features of the smart grids represented by the automatic electricity network capable of sending data and information from and to energy generating companies to users. In order for the future smart grids to function properly, they should be capable of assessing and analyzing large quantities of data in short time which is crucial for optimizing energy solutions including energy efficiency and optimization.
Speaking about the suggestions on how to deal with challenges of 5G networks in demand-response renewable energy grids, such major challenges as infrastructure issues, security issues, reliability, and economic challenges should be considered by the relevant stakeholders and policy-makers. When it comes to the infrastructure issues, the deployment of 5G networks still remains slow 2 years after its introduction, Moreover, many infrastructure components tend to age quickly and need to be replaced promptly. As far as the security issues are concerned, due to speed of 5G networks, they are particularly vulnerable for the attack surface of the core networks. Consumer literacy, strict regulation and privacy should be therefore reinforced. Furthermore, power outages, system malfunctions and cyber-attacks can become major threats for the reliability of the 5G networks in demand-response renewable energy grids. Finally, the economic challenges are represented by the lagging investment into the 5G infrastructure which was worsened by the COVID-19 pandemic and the overall financial downturn in the world. Optimal tariff plans need to be introduced to lure the new customers and users and to provide them with optimal and reliable connection enabling quick and efficient two-way data exchange that would suit the demands of the modern smart grids, including smart metering, vehicle charging, and the overall communication improvement in V2G/V2V/EV to charging station and among charging stations with a considerable impact on the development of the renewable energy.
In addition, one should also keep in mind the current limitations of different microgrid configurations in relation to certain metrics of communication performance for the successful functioning of microgrids with wireless and wired technologies. Some communities and ethnic groups all around the world might be more dependent on mobile services and online access that the others. When 5G technology is not deployed, gaps in the availability of clean energy for these communities could have devastating consequences for the IoE. Available and affordable 5G networks will be better able to support this emerging technology and address public interest concerns. One area that could be optimized for 5G usage is the IoE which offers huge benefits to the communities on the wrong side of the digital divide.
Author Contributions
All authors listed have made a substantial, direct, and intellectual contribution to the work and approved it for publication.
Conflict of Interest
The authors declare that the research was conducted in the absence of any commercial or financial relationships that could be construed as a potential conflict of interest.
Publisher’s Note
All claims expressed in this article are solely those of the authors and do not necessarily represent those of their affiliated organizations, or those of the publisher, the editors and the reviewers. Any product that may be evaluated in this article, or claim that may be made by its manufacturer, is not guaranteed or endorsed by the publisher.
References
Abbasi, M., Shahraki, A., Barzegar, H. R., and Pahl, C. (2021). Synchronization Techniques in "Device to Device- and Vehicle to Vehicle-Enabled" Cellular Networks: A Survey. Comput. Electr. Eng. 90, 106955. doi:10.1016/j.compeleceng.2020.106955
Abdel Hakeem, S. A., Hady, A. A., and Kim, H. (2020). 5G-V2X: Standardization, Architecture, Use Cases, Network-Slicing, and Edge-Computing. Wireless Netw. 26 (8), 6015–6041. doi:10.1007/s11276-020-02419-8
Aberilla, J. M., Gallego-Schmid, A., Stamford, L., and Azapagic, A. (2020). Design and Environmental Sustainability Assessment of Small-Scale Off-Grid Energy Systems for Remote Rural Communities. Appl. Energ. 258, 114004. doi:10.1016/j.apenergy.2019.114004
Adedipe, T., Shafiee, M., and Zio, E. (2020). Bayesian Network Modelling for the Wind Energy Industry: An Overview. Reliability Eng. Syst. Saf., 202. 107053. doi:10.1016/j.ress.2020.107053
Ahl, A., Yarime, M., Tanaka, K., and Sagawa, D. (2019). Review of Blockchain-Based Distributed Energy: Implications for Institutional Development. Renew. Sust. Energ. Rev. 107, 200–211. doi:10.1016/j.rser.2019.03.002
Alasseri, R., Rao, T. J., and Sreekanth, K. J. (2020). Institution of Incentive-Based Demand Response Programs and Prospective Policy Assessments for a Subsidized Electricity Market. Renew. Sust. Energ. Rev. 117, 109490. doi:10.1016/j.rser.2019.109490
Alreshidi, E. (2019). Smart Sustainable Agriculture (SSA) Solution Underpinned by Internet of Things (IoT) and Artificial Intelligence (AI). Ijacsa 10, 03106. doi:10.14569/IJACSA.2019.0100513
Aryanpur, V., Atabaki, M. S., Marzband, M., Siano, P., and Ghayoumi, K. (2019). An Overview of Energy Planning in Iran and Transition Pathways towards Sustainable Electricity Supply Sector. Renew. Sust. Energ. Rev. 112, 58–74. doi:10.1016/j.rser.2019.05.047
Avancini, D. B., Rodrigues, J. J. P. C., Martins, S. G. B., Rabêlo, R. A. L., Al-Muhtadi, J., and Solic, P. (2019). Energy Meters Evolution in Smart Grids: A Review. J. Clean. Prod. 217, 702–715. doi:10.1016/j.jclepro.2019.01.229
Azarova, V., Cohen, J. J., Kollmann, A., and Reichl, J. (2020). Reducing Household Electricity Consumption during Evening Peak Demand Times: Evidence from a Field experiment. Energy Policy 144, 111657. doi:10.1016/j.enpol.2020.111657
Bañales, S. (2020). The Enabling Impact of Digital Technologies on Distributed Energy Resources Integration. J. Renew. Sust. Energ. 12 (4), 045301. doi:10.1063/5.0009282
Bastida, L., Cohen, J. J., Kollmann, A., Moya, A., and Reichl, J. (2019). Exploring the Role of ICT on Household Behavioural Energy Efficiency to Mitigate Global Warming. Renew. Sust. Energ. Rev. 103, 455–462. doi:10.1016/j.rser.2019.01.004
Bhowmik, C., Bhowmik, S., Ray, A., and Pandey, K. M. (2017). Optimal green Energy Planning for Sustainable Development: A Review. Renew. Sust. Energ. Rev. 71, 796–813. doi:10.1016/j.rser.2016.12.105
Brinkel, N. B. G., Schram, W. L., AlSkaif, T. A., Lampropoulos, I., and Van Sark, W. G. J. H. M. (2020). Should We Reinforce the Grid? Cost and Emission Optimization of Electric Vehicle Charging under Different Transformer Limits. Appl. Energ. 276, 115285. doi:10.1016/j.apenergy.2020.115285
Burgess, R., Greenstone, M., Ryan, N., and Sudarshan, A. (2020). The Consequences of Treating Electricity as a Right. J. Econ. Perspect. 34 (1), 145–169. doi:10.1257/jep.34.1.145
Cailean, A.-M., and Dimian, M. (2017). Current Challenges for Visible Light Communications Usage in Vehicle Applications: A Survey. IEEE Commun. Surv. Tutorials 19 (4), 2681–2703. doi:10.1109/COMST.2017.2706940
Castagneto Gissey, G., Subkhankulova, D., Dodds, P. E., and Barrett, M. (2019). Value of Energy Storage Aggregation to the Electricity System. Energy Policy 128, 685–696. doi:10.1016/j.enpol.2019.01.037
Chamandoust, H., Derakhshan, G., Hakimi, S. M., and Bahramara, S. (2020). Tri-objective Scheduling of Residential Smart Electrical Distribution Grids with Optimal Joint of Responsive Loads with Renewable Energy Sources. J. Energ. Storage 27, 101112. doi:10.1016/j.est.2019.101112
Chand Malav, L., Yadav, K. K., Gupta, N., Kumar, S., Sharma, G. K., Krishnan, S., et al. (2020). A Review on Municipal Solid Waste as a Renewable Source for Waste-To-Energy Project in India: Current Practices, Challenges, and Future Opportunities. J. Clean. Prod. 277, 123227. doi:10.1016/j.jclepro.2020.123227
Chawla, Y., Kowalska-Pyzalska, A., and Silveira, P. D. (2020). Marketing and Communications Channels for Diffusion of Electricity Smart Meters in Portugal. Telematics Inform. 50, 101385. doi:10.1016/j.tele.2020.1013810.1016/j.tele.2020.101385
Chen, S., Hu, J., Shi, Y., Peng, Y., Fang, J., Zhao, R., et al. (2017). Vehicle-to-everything (V2X) Services Supported by LTE-Based Systems and 5G. IEEE Comm. Stand. Mag. 1 (2), 70–76. doi:10.1109/MCOMSTD.2017.1700015
Choi, J. S. (2019). A Hierarchical Distributed Energy Management Agent Framework for Smart Homes, Grids, and Cities. IEEE Commun. Mag. 57 (7), 113–119. doi:10.1109/MCOM.2019.1900073
Chu, K.-F., Lam, A. Y. S., and Li, V. O. K. (2020). Deep Multi-Scale Convolutional LSTM Network for Travel Demand and Origin-Destination Predictions. IEEE Trans. Intell. Transport. Syst. 21 (8), 3219–3232. doi:10.1109/TITS.2019.2924971
CLNR (2013). Insight Report: Domestic Solar PV Customers. Available at: http://www.networkrevolution.co.uk/wp-content/uploads/2015/01/CLNR-L090-Insight-Report-Domestic-Solar-PV.pdf (Accessed May 12, 2021).
Cooremans, C., and Schönenberger, A. (2019). Energy Management: A Key Driver of Energy-Efficiency Investment? J. Clean. Prod. 230, 264–275. doi:10.1016/j.jclepro.2019.04.333
Crespo Márquez, A., Crespo Del Castillo, A., and Gómez Fernández, J. F. (2020). Integrating Artificial Intelligent Techniques and Continuous Time Simulation Modelling. Practical Predictive Analytics for Energy Efficiency and Failure detectionPractical Predictive Analytics for Energy Efficiency and Failure Detection. Comput. Industry 115, 103164. doi:10.1016/j.compind.2019.103164
Croce, D., Giuliano, F., Bonomolo, M., Leone, G., Musca, R., Tinnirello, I., et al. (2020). A Decentralized Load Control Architecture for Smart Energy Consumption in Small Islands. Sust. Cities Soc. 53, 101902. doi:10.1016/j.scs.2019.101902
Cui, S., Wang, Y. W., and Liu, N. (2017). Distributed Game‐based Pricing Strategy for Energy Sharing in Microgrid with PV Prosumers. IET Renew. Power Generation 12 (3), 380–388. doi:10.1049/iet-rpg.2017.0570
Das, H. S., Rahman, M. M., Li, S., and Tan, C. W. (2020). Electric Vehicles Standards, Charging Infrastructure, and Impact on Grid Integration: A Technological Review. Renew. Sust. Energ. Rev. 120, 109618. doi:10.1016/j.rser.2019.109618
De Wildt, T. E., Chappin, E. J. L., Van de Kaa, G., Herder, P. M., and Van de Poel, I. R. (2019). Conflicting Values in the Smart Electricity Grid a Comprehensive Overview. Renew. Sust. Energ. Rev. 111, 184–196. doi:10.1016/j.rser.2019.05.005
Di Santo, K. G., Di Santo, S. G., Monaro, R. M., and Saidel, M. A. (2018). Active Demand Side Management for Households in Smart Grids Using Optimization and Artificial Intelligence. Measurement 115, 152–161. doi:10.1016/j.measurement.2017.10.010
Dominković, D. F., Junker, R. G., Lindberg, K. B., and Madsen, H. (2020). Implementing Flexibility into Energy Planning Models: Soft-Linking of a High-Level Energy Planning Model and a Short-Term Operational Model. Appl. Energ. 260, 114292. doi:10.1016/j.apenergy.2019.114292
Eid, C., Koliou, E., Valles, M., Reneses, J., and Hakvoort, R. (2016). Time-based Pricing and Electricity Demand Response: Existing Barriers and Next Steps. Utilities Policy 40, 15–25. doi:10.1016/j.jup.2016.04.001
Eseye, A. T., Lehtonen, M., Tukia, T., Uimonen, S., and Millar, R. J. (2019). Optimal Energy Trading for Renewable Energy Integrated Building Microgrids Containing Electric Vehicles and Energy Storage Batteries. IEEE Access 7, 106092–106101. doi:10.1109/ACCESS.2019.2932461
Eskandarpour, R., and Khodaei, A. (2017). Machine Learning Based Power Grid Outage Prediction in Response to Extreme Events. IEEE Trans. Power Syst. 32 (4), 3315–3316. doi:10.1109/TPWRS.2016.2631895
Fallgren, M., Vilalta, R., Dillinger, M., Alonso-Zarate, J., Boban, M., Abbas, T., et al. (2018). Fifth-generation Technologies for the Connected Car: Capable Systems for Vehicle-To-Anything Communications. IEEE Veh. Technol. Mag. 13 (3), 28–38. doi:10.1109/MVT.2018.2848400
Flynn, S. (2021). How Renewable Energy Could Power 5G for Sustainable Networking. Available at: https://www.altenergymag.com/story/2021/02/how-renewable-energy-could-power-5g-for-sustainable-networking/34627 (Accessed August 10, 2021).
Forge, S., and Vu, K. (2020). Forming a 5G Strategy for Developing Countries: A Note for Policy Makers. Telecommunications Policy 44 (7), 101975. doi:10.1016/j.telpol.2020.101975
Galbusera, L., Trucco, P., and Giannopoulos, G. (2020). Modeling Interdependencies in Multi-Sectoral Critical Infrastructure Systems: Evolving the DMCI Approach. Reliability Eng. Syst. Saf. 203, 107072. doi:10.1016/j.ress.2020.107072
Ghoddusi, H., Creamer, G. G., and Rafizadeh, N. (2019). Machine Learning in Energy Economics and Finance: A Review. Energ. Econ. 81, 709–727. doi:10.1016/j.eneco.2019.05.006
Gonzalez Venegas, F., Petit, M., and Perez, Y. (2021). Active Integration of Electric Vehicles into Distribution Grids: Barriers and Frameworks for Flexibility Services. Renew. Sust. Energ. Rev. 145, 111060. doi:10.1016/j.rser.2021.111060
Guerrero, J. I., Personal, E., García, A., Parejo, A., Pérez, F., and León, C. (2019). Distributed Charging Prioritization Methodology Based on Evolutionary Computation and Virtual Power Plants to Integrate Electric Vehicle Fleets on Smart Grids. Energies 12 (12), 2402. doi:10.3390/en12122402
Han, B., Peng, S., Wu, C., Wang, X., and Wang, B. (2020). Lora-based Physical Layer Key Generation for Secure V2v/v2i Communications. Sensors 20 (3), 682. doi:10.3390/s20030682
Horowitz, K. A. W., Jain, A., Ding, F., Mather, B., and Palmintier, B. (2020). A Techno-Economic Comparison of Traditional Upgrades, Volt-Var Controls, and Coordinated Distributed Energy Resource Management Systems for Integration of Distributed Photovoltaic Resources. Int. J. Electr. Power Energ. Syst. 123, 106222. doi:10.1016/j.ijepes.2020.106222
Hossein Motlagh, N., Mohammadrezaei, M., Hunt, J., and Zakeri, B. (2020). Internet of Things (IoT) and the Energy Sector. Energies 13 (2), 494. doi:10.3390/en13020494
Hou, Y., Iqbal, W., Muhammad Shaikh, G., Iqbal, N., Ahmad Solangi, Y., and Fatima, A. (2019). Measuring Energy Efficiency and Environmental Performance: a Case of South Asia. Processes 7 (6), 325. doi:10.3390/pr7060325
Hsu, C.-L., Chen, W.-X., and Le, T.-V. (2020). An Autonomous Log Storage Management Protocol with Blockchain Mechanism and Access Control for the Internet of Things. Sensors 20 (22), 6471. doi:10.3390/s20226471
Huang, W., Zhang, N., Kang, C., Li, M., and Huo, M. (2019). From Demand Response to Integrated Demand Response: Review and prospect of Research and Application. Prot. Control. Mod. Power Syst. 4 (1), 12. doi:10.1186/s41601-019-0126-4
Husain, S. S., Kunz, A., Prasad, A., Pateromichelakis, E., and Samdanis, K. (2019). Ultra-high Reliable 5G V2X Communications. IEEE Comm. Stand. Mag. 3 (2), 46–52. doi:10.1109/MCOMSTD.2019.1900008
Iris, Ç., and Lam, J. S. L. (2019). A Review of Energy Efficiency in Ports: Operational Strategies, Technologies and Energy Management Systems. Renew. Sust. Energ. Rev. 112, 170–182. doi:10.1016/j.rser.2019.04.069
Israr, A., Yang, Q., Li, W., and Zomaya, A. Y. (2021). Renewable Energy Powered Sustainable 5G Network Infrastructure: Opportunities, Challenges and Perspectives. J. Netw. Comp. Appl. 175, 102910. doi:10.1016/j.jnca.2020.102910
Jannati, M., Foroutan, E., Mousavi, S. M. S., and Grijalva, S. (2020). An Intelligent Energy Management System to Use Parking Lots as Energy Storage Systems in Smoothing Short-Term Power Fluctuations of Renewable Resources. J. Energ. Storage 32, 101905. doi:10.1016/j.est.2020.101905
Ji, H., Alfarraj, O., and Tolba, A. (2020). Artificial Intelligence-Empowered Edge of Vehicles: Architecture, Enabling Technologies, and Applications. IEEE Access 8, 61020–61034. doi:10.1109/ACCESS.2020.2983609
Jose, R., Panigrahi, S. K., Patil, R. A., Fernando, Y., and Ramakrishna, S. (2020). Artificial Intelligence-Driven Circular Economy as a Key Enabler for Sustainable Energy Management. Mater. Circ. Econ. 2 (1), 1–7. doi:10.1007/s42824-020-00009-9
Ju, L., Tan, Q., Lin, H., Mei, S., Li, N., Lu, Y., et al. (2020). A Two-Stage Optimal Coordinated Scheduling Strategy for Micro Energy Grid Integrating Intermittent Renewable Energy Sources Considering Multi-Energy Flexible Conversion. Energy 196, 117078. doi:10.1016/j.energy.2020.117078
Kakran, S., and Chanana, S. (2018). Smart Operations of Smart Grids Integrated with Distributed Generation: A Review. Renew. Sust. Energ. Rev. 81, 524–535. doi:10.1016/j.rser.2017.07.045
Khan, I. (2019). Energy-saving Behaviour as a Demand-Side Management Strategy in the Developing World: the Case of Bangladesh. Int. J. Energ. Environ Eng 10 (4), 493–510. doi:10.1007/s40095-019-0302-3
Khan, R., Kumar, P., Jayakody, D. N. K., and Liyanage, M. (2020). A Survey on Security and Privacy of 5G Technologies: Potential Solutions, Recent Advancements, and Future Directions. IEEE Commun. Surv. Tutorials 22 (1), 196–248. doi:10.1109/COMST.2019.2933899
Khan, U. A., and Lee, S. S. (2020). Distance-Based Resource Allocation for Vehicle-To-Pedestrian Safety Communication. Electronics 9 (10), 1640. doi:10.3390/electronics9101640
Khattak, H. A., Farman, H., Jan, B., and Din, I. U. (2019). Toward Integrating Vehicular Clouds with IoT for Smart City Services. IEEE Netw. 33 (2), 65–71. doi:10.1109/MNET.2019.1800236
Kim, Y. M., Jung, D., Chang, Y., and Choi, D. H. (2019). Intelligent Micro Energy Grid in 5G Era: Platforms, Business Cases, Testbeds, and Next Generation Applications. Electronics 8 (4), 468. doi:10.3390/electronics8040468
Konstantakopoulos, I. C., Barkan, A. R., He, S., Veeravalli, T., Liu, H., and Spanos, C. (2019). A Deep Learning and Gamification Approach to Improving Human-Building Interaction and Energy Efficiency in Smart Infrastructure. Appl. Energ. 237, 810–821. doi:10.1016/j.apenergy.2018.12.065
Kumar, A., Meena, N. K., Singh, A. R., Deng, Y., He, X., Bansal, R. C., et al. (2019). Strategic Integration of Battery Energy Storage Systems with the Provision of Distributed Ancillary Services in Active Distribution Systems. Appl. Energ. 253, 113503. doi:10.1016/j.apenergy.2019.113503
Langendahl, P.-A., Roby, H., Potter, S., and Cook, M. (2019). Smoothing Peaks and Troughs: Intermediary Practices to Promote Demand Side Response in Smart Grids. Energ. Res. Soc. Sci. 58, 101277. doi:10.1016/j.erss.2019.101277
Lee, S., Park, M., Lee, H.-S., and Ham, Y. (2020). Impact of Demand-Side Response on Community Resilience: Focusing on a Power Grid after Seismic Hazards. J. Manage. Eng. 36 (6), 04020071. doi:10.1061/(ASCE)ME.1943-5479.0000844
Li, D., Zouma, A., Liao, J.-T., and Yang, H.-T. (2020). An Energy Management Strategy with Renewable Energy and Energy Storage System for a Large Electric Vehicle Charging Station. eTransportation 6, 100076. doi:10.1016/j.etran.2020.100076
Li, F. G. N., Bataille, C., Pye, S., and O'Sullivan, A. (2019). Prospects for Energy Economy Modelling with Big Data: Hype, Eliminating Blind Spots, or Revolutionising the State of the Art? Appl. Energ. 239, 991–1002. doi:10.1016/j.apenergy.2019.02.002
Liao, B., Ali, Y., Nazir, S., He, L., and Khan, H. U. (2020). Security Analysis of IoT Devices by Using mobile Computing: a Systematic Literature Review. IEEE Access 8, 120331–120350. doi:10.1109/ACCESS.2020.3006358
Long, H., Li, P., and Gu, W. (2020). A Data-Driven Evolutionary Algorithm for Wind Farm Layout Optimization. Energy 208, 118310. doi:10.1016/j.energy.2020.118310
Lu, J., Guo, J., Jian, Z., and Xu, X. (2019). Research and Application of Efficient Risk Analysis Method for Electric Power Grid Multiple Faults under Widespread Wildfire Disasters. Int. Trans. Electr. Energ Syst. 29 (9), e12055. doi:10.1002/2050-7038.12055
Luo, W., Chen, W., Feng, Y., and Yang, Y. (2020). A Novel Automobile Antenna for Vehicles Communication of IoT Systems in 5G Network. J. Wireless Com Netw. 2020, 218. doi:10.1186/s13638-020-01838-72020
Ma, Y.-J., and Zhai, M.-Y. (2019). Day-Ahead Prediction of Microgrid Electricity Demand Using a Hybrid Artificial Intelligence Model. Processes 7 (6), 320. doi:10.3390/pr7060320
Madurai Elavarasan, R., Afridhis, S., Vijayaraghavan, R. R., Subramaniam, U., and Nurunnabi, M. (2020). SWOT Analysis: A Framework for Comprehensive Evaluation of Drivers and Barriers for Renewable Energy Development in Significant Countries. Energ. Rep. 6, 1838–1864. doi:10.1016/j.egyr.2020.07.007
Mahmud, K., Khan, B., Ravishankar, J., Ahmadi, A., and Siano, P. (2020). An Internet of Energy Framework with Distributed Energy Resources, Prosumers and Small-Scale Virtual Power Plants: An Overview. Renew. Sust. Energ. Rev. 127, 109840. doi:10.1016/j.rser.2020.109840
Majumdar, S., Subhani, M. M., Roullier, B., Anjum, A., and Zhu, R. (2021). Congestion Prediction for Smart Sustainable Cities Using IoT and Machine Learning Approaches. Sust. Cities Soc. 64, 102500. doi:10.1016/j.scs.2020.102500
Mannweiler, C., Sartori, C., Wegmann, B., Flinck, H., Maeder, A., Goerge, J., et al. (2020). “Evolution of Mobile Communication Networks,” in Towards Cognitive Autonomous Networks: Network Management Automation for 5G and beyond. Editors S. Mwanje, and C. Mannweiler. 1st Edn. (NJ, USA: John Wiley & Sons), 29–92. doi:10.1002/9781119586449.ch2
Matějka, F., and McKay, A. (2015). Rational Inattention to Discrete Choices: A New Foundation for the Multinomial Logit Model. Am. Econ. Rev. 105 (1), 272–298. doi:10.1257/aer.20130047
Mehmood, M. U., Chun, D., Zeeshan, H., Han, H., Jeon, G., and Chen, K. (2019). A Review of the Applications of Artificial Intelligence and Big Data to Buildings for Energy-Efficiency and a Comfortable Indoor Living Environment. Energy and Buildings 202, 109383. doi:10.1016/j.enbuild.2019.109383
Miglani, A., Kumar, N., Chamola, V., and Zeadally, S. (2020). Blockchain for Internet of Energy Management: Review, Solutions, and Challenges. Comp. Commun. 151, 395–418. doi:10.1016/j.comcom.2020.01.014
Miller, R. (2020). Rolling Zettabytes: Quantifying the Data Impact of Connected Cars. Available at: https://datacenterfrontier.com/rolling-zettabytes-quantifying-the-data-impact-of-connected-cars/(Accessed August 10, 2021).
Momen, H., Abessi, A., and Jadid, S. (2020). Using EVs as Distributed Energy Resources for Critical Load Restoration in Resilient Power Distribution Systems. IET Generation, Transm. Distribution 14 (18), 3750–3761. doi:10.1049/iet-gtd.2019.1561
Morstyn, T., Farrell, N., Darby, S. J., and McCulloch, M. D. (2018). Using Peer-To-Peer Energy-Trading Platforms to Incentivize Prosumers to Form Federated Power Plants. Nat. Energ. 3 (2), 94–101. doi:10.1038/s41560-017-0075-y
Mrabet, H., Belguith, S., Alhomoud, A., and Jemai, A. (2020). A Survey of IoT Security Based on a Layered Architecture of Sensing and Data Analysis. Sensors 20 (13), 3625. doi:10.3390/s20133625
Munshi, A. A., and Mohamed, Y. A.-R. I. (2017). Big Data Framework for Analytics in Smart Grids. Electric Power Syst. Res. 151, 369–380. doi:10.1016/j.epsr.2017.06.006
Ndikumana, A., Tran, N. H., Kim, D. H., Kim, K. T., and Hong, C. S. (2021). Deep Learning Based Caching for Self-Driving Cars in Multi-Access Edge Computing. IEEE Trans. Intell. Transport. Syst. 22 (5), 2862–2877. doi:10.1109/TITS.2020.2976572
Ngarambe, J., Yun, G. Y., and Santamouris, M. (2020). The Use of Artificial Intelligence (AI) Methods in the Prediction of thermal comfort in Buildings: Energy Implications of AI-Based thermal comfort Controls. Energy and Buildings 211, 109807. doi:10.1016/j.enbuild.2020.109807
Nižetić, S., Djilali, N., Papadopoulos, A., and Rodrigues, J. J. P. C. (2019). Smart Technologies for Promotion of Energy Efficiency, Utilization of Sustainable Resources and Waste Management. J. Clean. Prod. 231, 565–591. doi:10.1016/j.jclepro.2019.04.397
Oprea, S. V., Bâra, A., and Ifrim, G. (2018). Flattening the Electricity Consumption Peak and Reducing the Electricity Payment for Residential Consumers in the Context of Smart Grid by Means of Shifting Optimization Algorithm. Comput. Ind. Eng. 122, 125–139. doi:10.1016/j.cie.2018.05.053
O’Ryan, R., Nasirov, S., and Álvarez-Espinosa, A. (2020). Renewable Energy Expansion in the Chilean Power Market: A Dynamic General Equilibrium Modeling Approach to Determine CO2 Emission Baselines. J. Clean. Prod. 247, 119645. doi:10.1016/j.jclepro.2019.119645
Pawar, P., TarunKumar, M., and Vittal K., P. (2020). An IoT Based Intelligent Smart Energy Management System with Accurate Forecasting and Load Strategy for Renewable Generation. Measurement 152, 107187. doi:10.1016/j.measurement.2019.107187
Pei, Y., Qian, Z., Jing, B., Kang, D., and Zhang, L. (2018). Data-driven Method for Wind Turbine Yaw Angle Sensor Zero-point Shifting Fault Detection. Energies 11 (3), 553. doi:10.3390/en11030553
Peng, D., and Poudineh, R. (2019). Electricity Market Design under Increasing Renewable Energy Penetration: Misalignments Observed in the European Union. Utilities Policy 61, 100970. doi:10.1016/j.jup.2019.100970
Pinto, T., Morais, H., and Corchado, J. M. (2019). Adaptive Entropy-Based Learning with Dynamic Artificial Neural Network. Neurocomputing 338, 432–440. doi:10.1016/j.neucom.2018.09.092
Rabaia, M. K. H., Abdelkareem, M. A., Sayed, E. T., Elsaid, K., Chae, K.-J., Wilberforce, T., et al. (2021). Environmental Impacts of Solar Energy Systems: A Review. Sci. Total Environ. 754, 141989. doi:10.1016/j.scitotenv.2020.141989
Raina, G., and Sinha, S. (2019). Outlook on the Indian Scenario of Solar Energy Strategies: Policies and Challenges. Energ. Strategy Rev. 24, 331–341. doi:10.1016/j.esr.2019.04.005
Rajper, S. Z., and Albrecht, J. (2020). Prospects of Electric Vehicles in the Developing Countries: a Literature Review. Sustainability 12 (5), 1906. doi:10.3390/su12051906
Rathor, S. K., and Saxena, D. (2020). Energy Management System for Smart Grid: An Overview and Key Issues. Int. J. Energ. Res 44 (6), 4067–4109. doi:10.1002/er.4883
Rausser, G., Strielkowski, W., and Štreimikienė, D. (2018). Smart Meters and Household Electricity Consumption: A Case Study in Ireland. Energ. Environ. 29 (1), 131–146. doi:10.1177/0958305X17741385
Rayati, M., Amirzadeh Goghari, S., Nasiri Gheidari, Z., and Ranjbar, A. (2019). An Optimal and Decentralized Transactive Energy System for Electrical Grids with High Penetration of Renewable Energy Sources. Int. J. Electr. Power Energ. Syst. 113, 850–860. doi:10.1016/j.ijepes.2019.06.017
Rehmani, M. H., Reisslein, M., Rachedi, A., Erol-Kantarci, M., and Radenkovic, M. (2018). Integrating Renewable Energy Resources into the Smart Grid: Recent Developments in Information and Communication Technologies. IEEE Trans. Ind. Inf. 14 (7), 2814–2825. doi:10.1109/TII.2018.2819169
Roldán-Blay, C., Miranda, V., Carvalho, L., and Roldán-Porta, C. (2019). Optimal Generation Scheduling with Dynamic Profiles for the Sustainable Development of Electricity Grids. Sustainability 11 (24), 7111. doi:10.3390/su11247111
S, S. R., Dragičević, T., Siano, P., and Prabaharan, S. R. S. (2019). Future Generation 5G Wireless Networks for Smart Grid: A Comprehensive Review. Energies 12 (11), 2140. doi:10.3390/en12112140
Sakib, N., Hossain, E., and Ahamed, S. I. (2020). A Qualitative Study on the united states Internet of Energy: A Step towards Computational Sustainability. IEEE Access 8, 69003–69037. doi:10.1109/ACCESS.2020.2986317
Sarker, E., Seyedmahmoudian, M., Jamei, E., Horan, B., and Stojcevski, A. (2020). Optimal Management of home Loads with Renewable Energy Integration and Demand Response Strategy. Energy 210, 118602. doi:10.1016/j.energy.2020.118602
Savari, G. F., Krishnasamy, V., Sathik, J., Ali, Z. M., and Abdel Aleem, S. H. E. (2020). Internet of Things Based Real-Time Electric Vehicle Load Forecasting and Charging Station Recommendation. ISA Trans. 97, 431–447. doi:10.1016/j.isatra.2019.08.011
Schmid, D., Korkmaz, P., Blesl, M., Fahl, U., and Friedrich, R. (2019). Analyzing Transformation Pathways to a Sustainable European Energy System-Internalization of Health Damage Costs Caused by Air Pollution. Energ. Strategy Rev. 26, 100417. doi:10.1016/j.esr.2019.100417
Sengan, S., V., S., Nair, S. K., V., I., J., M., and Ravi, L. (2020). Enhancing Cyber-Physical Systems with Hybrid Smart City Cyber Security Architecture for Secure Public Data-Smart Network. Future Generation Comp. Syst. 112, 724–737. doi:10.1016/j.future.2020.06.028
Shareef, H., Ahmed, M. S., Mohamed, A., and Al Hassan, E. (2018). Review on home Energy Management System Considering Demand Responses, Smart Technologies, and Intelligent Controllers. IEEE Access 6, 24498–24509. doi:10.1109/ACCESS.2018.2831917
Sharifi, R., Anvari-Moghaddam, A., Hamid Fathi, S., Guerrero, J. M., and Vahidinasab, V. (2019). An Optimal Market-Oriented Demand Response Model for price-responsive Residential Consumers. Energy Efficiency 12 (3), 803–815. doi:10.1007/s12053-018-9713-x
Shi, Q., Dong, B., He, T., Sun, Z., Zhu, J., Zhang, Z., et al. (2020). Progress in Wearable Electronics/photonics-Moving toward the Era of Artificial Intelligence and Internet of Things. InfoMat 2 (6), 1131–1162. doi:10.1002/inf2.12122
Shrestha, M., Johansen, C., Noll, J., and Roverso, D. (2020). A Methodology for Security Classification Applied to Smart Grid Infrastructures. Int. J. Crit. Infrastructure Prot. 28, 100342. doi:10.1016/j.ijcip.2020.100342
Simshauser, P. (2016). Distribution Network Prices and Solar PV: Resolving Rate Instability and Wealth Transfers through Demand Tariffs. Energ. Econ. 54, 108–122. doi:10.1016/j.eneco.2015.11.011
Sinclair, A. H., and Barense, M. D. (2019). Prediction Error and Memory Reactivation: How Incomplete Reminders Drive Reconsolidation. Trends Neurosciences 42 (10), 727–739. doi:10.1016/j.tins.2019.08.007
Soares, J., Pinto, T., Lezama, F., and Morais, H. (2018). Survey on Complex Optimization and Simulation for the New Power Systems Paradigm. Complexity 2018, 1–32. doi:10.1155/2018/2340628
Sousa, T., Soares, T., Pinson, P., Moret, F., Baroche, T., and Sorin, E. (2019). Peer-to-peer and Community-Based Markets: A Comprehensive Review. Renew. Sust. Energ. Rev. 104, 367–378. doi:10.1016/j.rser.2019.01.036
Storck, C. R., and Duarte-Figueiredo, F. (2020). A Survey of 5G Technology Evolution, Standards, and Infrastructure Associated with Vehicle-To-Everything Communications by Internet of Vehicles. IEEE Access 8, 117593–117614. doi:10.1109/ACCESS.2020.3004779
Strielkowski, W., Streimikiene, D., Fomina, A., and Semenova, E. (2019). Internet of Energy (IoE) and High-Renewables Electricity System Market Design. Energies 12 (24), 4790. doi:10.3390/en12244790
Strielkowski, W., Štreimikienė, D., and Bilan, Y. (2017). Network Charging and Residential Tariffs: A Case of Household Photovoltaics in the United Kingdom. Renew. Sust. Energ. Rev. 77, 461–473. doi:10.1016/j.rser.2017.04.029
Strielkowski, W., Veinbender, T., Tvaronavičienė, M., and Lace, N. (2020). Economic Efficiency and Energy Security of Smart Cities. Econ. Research-Ekonomska Istraživanja 33 (1), 788–803. doi:10.1080/1331677X.2020.1734854
Sweeney, C., Bessa, R. J., Browell, J., and Pinson, P. (2020). The Future of Forecasting for Renewable Energy. Wires Energ. Environ. 9 (2), e365. doi:10.1002/wene.365
Tronchin, L., Manfren, M., and Nastasi, B. (2018). Energy Efficiency, Demand Side Management and Energy Storage Technologies - A Critical Analysis of Possible Paths of Integration in the Built Environment. Renew. Sust. Energ. Rev. 95, 341–353. doi:10.1016/j.rser.2018.06.060
Tsao, Y.-C., Thanh, V.-V., and Wu, Q. (2021). Sustainable Microgrid Design Considering Blockchain Technology for Real-Time price-based Demand Response Programs. Int. J. Electr. Power Energ. Syst. 125, 106418. doi:10.1016/j.ijepes.2020.106418
Tsaousoglou, G., Steriotis, K., Efthymiopoulos, N., Makris, P., and Varvarigos, E. (2020). Truthful, Practical and Privacy-Aware Demand Response in the Smart Grid via a Distributed and Optimal Mechanism. IEEE Trans. Smart Grid 11 (4), 3119–3130. doi:10.1109/TSG.2020.2965221
Tufail, A., Namoun, A., Sen, A. A. A., Kim, K.-H., Alrehaili, A., and Ali, A. (2021). Moisture Computing-Based Internet of Vehicles (IoV) Architecture for Smart Cities. Sensors 21 (11), 3785. doi:10.3390/s21113785
Van Summeren, L. F. M., Wieczorek, A. J., Bombaerts, G. J. T., and Verbong, G. P. J. (2020). Community Energy Meets Smart Grids: Reviewing Goals, Structure, and Roles in Virtual Power Plants in Ireland, Belgium and the Netherlands. Energ. Res. Soc. Sci. 63, 101415. doi:10.1016/j.erss.2019.101415
Vilaça Gomes, P., Saraiva, J. T., Carvalho, L., Dias, B., and Oliveira, L. W. (2019). Impact of Decision-Making Models in Transmission Expansion Planning Considering Large Shares of Renewable Energy Sources. Electric Power Syst. Res. 174, 105852. doi:10.1016/j.epsr.2019.04.030
Wan Yen, S., Morris, S., Ezra, M. A. G., and Jun Huat, T. (2019). Effect of Smart Meter Data Collection Frequency in an Early Detection of Shorter-Duration Voltage Anomalies in Smart Grids. Int. J. Electr. Power Energ. Syst. 109, 1–8. doi:10.1016/j.ijepes.2019.01.039
Wang, Z., Yu, X., Mu, Y., and Jia, H. (2020). A Distributed Peer-To-Peer Energy Transaction Method for Diversified Prosumers in Urban Community Microgrid System. Appl. Energ. 260, 114327. doi:10.1016/j.apenergy.2019.114327
Wood, D. A. (2019). German Solar Power Generation Data Mining and Prediction with Transparent Open Box Learning Network Integrating Weather, Environmental and Market Variables. Energ. Convers. Manag. 196, 354–369. doi:10.1016/j.enconman.2019.05.114
Worighi, I., Maach, A., Hafid, A., Hegazy, O., and Van Mierlo, J. (2019). Integrating Renewable Energy in Smart Grid System: Architecture, Virtualization and Analysis. Sustainable Energ. Grids Networks 18, 100226. doi:10.1016/j.segan.2019.100226
Wu, Y., Ravey, A., Chrenko, D., and Miraoui, A. (2019). Demand Side Energy Management of EV Charging Stations by Approximate Dynamic Programming. Energ. Convers. Manag. 196, 878–890. doi:10.1016/j.enconman.2019.06.058
Wu, Y., Wu, Y., Guerrero, J. M., and Vasquez, J. C. (2021). A Comprehensive Overview of Framework for Developing Sustainable Energy Internet: From Things-Based Energy Network to Services-Based Management System. Renew. Sust. Energ. Rev. 150, 111409. doi:10.1016/j.rser.2021.111409
Wu, Y., Wu, Y., Guerrero, J., Vasquez, J., Palacios-García, E., and Guan, Y. (2020). IoT-enabled Microgrid for Intelligent Energy-Aware Buildings: A Novel Hierarchical Self-Consumption Scheme with Renewables. Electronics 9 (4), 550. doi:10.3390/electronics9040550
Xu, Y., Ahokangas, P., Louis, J.-N., and Pongrácz, E. (2019). Electricity Market Empowered by Artificial Intelligence: A Platform Approach. Energies 12 (21), 4128. doi:10.3390/en12214128
Yang, K., Huang, Y., Qin, Y., Hu, C., and Tang, X. (2021). Potential and Challenges to Improve Vehicle Energy Efficiency via V2X: Literature Review. Ijvp 7 (3-4), 244–265. doi:10.1504/IJVP.2021.116058
Yanine, F., Sánchez-Squella, A., Barrueto, A., Parejo, A., Cordova, F., and Rother, H. (2020). Grid-Tied Distributed Generation Systems to Sustain the Smart Grid Transformation: Tariff Analysis and Generation Sharing. Energies 13 (5), 1187. doi:10.3390/en13051187
Zahed, M. I. A., Ahmad, I., Habibi, D., Phung, Q. V., and Mowla, M. M. (2020). Proactive Content Caching Using Surplus Renewable Energy: A Win-Win Solution for Both Network Service and Energy Providers. Future Generation Comp. Syst. 105, 210–221. doi:10.1016/j.future.2019.11.041
Zapata Riveros, J., Kubli, M., and Ulli-Beer, S. (2019). Prosumer Communities as Strategic Allies for Electric Utilities: Exploring Future Decentralization Trends in Switzerland. Energ. Res. Soc. Sci. 57, 101219. doi:10.1016/j.erss.2019.101219
Zhao, L., Li, X., Gu, B., Zhou, Z., Mumtaz, S., Frascolla, V., et al. (2018). Vehicular Communications: Standardization and Open Issues. IEEE Comm. Stand. Mag. 2 (4), 74–80. doi:10.1109/MCOMSTD.2018.1800027
Zhou, H., Xu, W., Chen, J., and Wang, W. (2020). Evolutionary V2X Technologies toward the Internet of Vehicles: Challenges and Opportunities. Proc. IEEE 108 (2), 308–323. doi:10.1109/JPROC.2019.2961937
Keywords: renewable energy sources, 5G networks, internet of energy (IoE), demand-side response (DSR), smart grids (SG), energy resource management (ERM), energy eficiency
Citation: Strielkowski W, Dvořák M, Rovný P, Tarkhanova E and Baburina N (2021) 5G Wireless Networks in the Future Renewable Energy Systems. Front. Energy Res. 9:714803. doi: 10.3389/fenrg.2021.714803
Received: 25 May 2021; Accepted: 28 September 2021;
Published: 12 October 2021.
Edited by:
Yunhe Hou, The University of Hong Kong, Hong Kong, SAR ChinaReviewed by:
Subhasis Thakur, National University of Ireland Galway, IrelandYixuan Chen, The University of Hong Kong, Hong Kong, SAR China
Copyright © 2021 Strielkowski, Dvořák, Rovný, Tarkhanova and Baburina. This is an open-access article distributed under the terms of the Creative Commons Attribution License (CC BY). The use, distribution or reproduction in other forums is permitted, provided the original author(s) and the copyright owner(s) are credited and that the original publication in this journal is cited, in accordance with accepted academic practice. No use, distribution or reproduction is permitted which does not comply with these terms.
*Correspondence: Wadim Strielkowski, c3RyaWVsa293c2tpQGJlcmtlbGV5LmVkdQ==