- Shenyang University of Technology, Shenyang, China
The large-scale penetration of renewable energy and deeply integrated multiple energy forms pose a major challenge for the accounting and allocation process of additional adjustment cost in multi-energy system and electricity market. The variable wind power and the operating status of multi-energy systems faced by the electricity market have driven researchers towards the decision of electricity spot market with complicated pricing mechanism. This paper presents a locational marginal price-based partition optimal economic dispatch model of multi-energy system with a high proportion of renewable energy. First, a model of the additional adjustment cost of multi-energy adjustable units to improve the power grid adjustment capacity is studied based on the power balance model of multi-energy conversion and storage. In the second, to improve the effectiveness of the ESM mechanism, an optimal economic dispatch model is established, with the goal of minimizing the additional adjustment cost of multi-energy adjustable units. Moreover, according to the characteristics of the model and the mechanism of locational marginal price-based economic dispatch, a solution method based on lagrangian relaxation is proposed. The incentive compatible locational marginal price is obtained. Finally, a simulation model of multi-energy systems based on IEEE 39-nodes power system and 7-nodes natural gas system is established. And the model is based on operating data of an actual grid with high proportion of renewable energy in Northeast China. The results of the examples show that the proposed method can effectively improve the efficiency and flexibility of multi-energy system. Another advantage of the proposed method is that the capabilities of the complementary multi-energy coordination can be promoted.
Introduction
The real-time transaction mechanism of multi-agent decision effort in multi-energy system (MES) facilitates the clean and efficient development of power grids, and has become a hot issue in the field of power system research (Jiang and Ai, 2017; Peng and Tao, 2018). In MES environment, a reasonable pricing mechanism (PM) can be used to implement market-oriented construction and optimization of the supply and demand balance of power commodities. Such mechanism can also be used for increasing the grid-connected capacity of renewable energy and other issues (PrudhvirajKiran and Pindoriya, 2020; Fang and Cui, 2020). At present, the traditional way of pricing mechanism mainly used in electricity spot market (ESM) is zonal marginal price (ZMP) and locational marginal price (LMP) (Jovanovic et al., 2017; Gong et al., 2018; Fang et al., 2020b). A key challenge in designing efficient ESM is the flexible price signals and reduce overall market operating costs in a complex power network. Therefore, the LMP is the pricing method adopted by most electricity spot markets in most countries or regions, which can provide the transmission line capacity constraints and the ability to inhibit branch blocking.
With the continuous expansion of the multi-energy system and electricity spot market, constructing a sophisticated LMP has become a key research topic in the future energy system. In order to study the effect mechanism of power network topology changes on LMP, (Choi and Xie. 2017) studied a LMP model based on grid topology state evaluation. And a simple analytical congestion price equation is proposed, which can improve the LMP model rationality and calculation quality. Morstyn et al. (2020) proposed a distributed energy bilateral trading strategy based on peer-to-peer (P2P) energy trading and probabilistic locational marginal pricing, which can effectively improve the grid-connected capacity of renewable energy and system flexibility. In order to increase the solution accuracy and efficiency of the LMP, (Hajiabadi and Samadi. 2019) proposed a nodal marginal price solution strategy based on unit-based LMP_S index; the test results show that the strategy can improve the incremental profit of unit. Faqiry et al. (2020) proposed a day-ahead electricity market (EM) clearing model based on distributed LMP, and a transactive day-ahead EM model under different renewable energy confidence intervals was constructed to solve the problem of line congestion and renewable energy uncertainty; the results show that the proposed method can reduce the impact of random events such as peak load and renewable energy fluctuations on the grid. Vaskovskaya et al. (2018) proposed a LMP model based on price-bonding factor that effectively decompose the LMP model and improve the rationality of PM for transmission capacity and voltage. Aiming at investigating spatiotemporal correlations among various uncertainty sources, (Fang et al. (2020a) established a distributionally-robust chance constrained multi-interval optimal power flow model, that can establish LMP with uncertainty. Similarly, (Fang et al., 2019) proposed an EM clearing model that takes into account economy and reliability for the uncertainty of wind power and load; the simulation results show that the proposed method can fully reflect the changes in system cost caused by wind power and load uncertainty, and improve the effectiveness of the PM. Zheng et al. (2020) proposed a LMP forecasting model considering congestion and network loss for short-term LMP forecasting with high accuracy and robustness. Considering the problems of energy balance, network loss, and voltage stability in the EM, (Hanif et al., 2019) proposed a new distribution locational marginal price model, which can effectively improve the optimal allocation of flexible resources in the electricity market. The above studies have made in-depth studies on various aspects of the ESM, such as clearing methods, price mechanisms, and flexible distribution.
In recent years, the scale of renewable energy has continued to expand. Hence, the increasing multi-energy conversion and storage have been connected to the grid, resulting in the complexity of the pricing mechanism of multi-energy coordination (Teng et al., 2019a; Teng et al., 2019b; Zhang et al., 2021). Multi-energy system has advanced energy conversion technology and information transmission technology (Gao et al., 2020; Teng et al., 2019c; Yang et al., 2020). Additionally, during the operation of the traditional ESM, only the adjustment cost of conventional generation in the power system is considered, and the coordination effect of multiple energy is ignored. The lack of rationality of the cost-sharing method restricts the development of the multi-energy coordinated ESM. Therefore, multi-energy coordinated ESM clearing model with a growing importance in the ESM for PM is the best method, which can enhance the flexibility and rationality of price signals in ESM.
Based on the above analysis, multi-energy adjustable units with obvious differences of adjustment characteristics in time scale and capacity scale should be constructed. LMP model calculation efficiency should be improved. With that as motivation, a locational marginal price-based partition optimal economic dispatch model of multi-energy system is proposed in the article, which including multi-energy adjustable units, the process of lagrangian relaxation and locational price. Based on the obtained results, the contributions of this paper are as following, briefly.
(1) The characteristics of multi-energy conversion and storage are studied. Based on this, the mechanism of the additional adjustment cost of the multi-energy adjustable units considering the balance of supply and demand is studied. An additional adjustment cost allocation mechanism is established in multi-energy coordinated electricity spot market environment. It provides a theoretical basis for calculating the adjustment additional cost of electricity spot market in grid with multi-energy coordinated.
(2) An optimal economic dispatch model of multi-energy system based on the additional adjustment costs is proposed. The objective function is to minimize the operating costs and adjustment costs of multi-energy adjustable units. The constraints include the power network constraints, gas network constraints, and multi-energy adjustable equipment constraints. This model plays an important role for pricing mechanism of multi-energy systems coordinated dispatch.
(3) Lagrangian relaxation is applied as optimized algorithm to solve the problem of dispatch. Based on the results it is possible to accomplish a safe, efficient and flexible multi-energy system. Another advantage of the proposed method is that decrease the run time of the proposed problem.
This paper is structured as following. Multi-Energy System and Additional Adjustment Cost illustrates a systems model with multi-energy conversion and storage and additional adjustment cost. Partition Optimal Economic Dispatch Model of Multi-Energy System proposes a partition optimal economic dispatch model of multi-energy system. Solving Algorithm presents a solution method based on lagrangian relaxation. Case Study verifies the performance of the proposed model in simulation. Conclusion presents the conclusion.
Multi-Energy System and Additional Adjustment Cost
The Structure of Multi-Energy System
The structure of multi-energy system is shown in Figure 1, including wind power, photovoltaic, natural gas sources, multi-energy adjustable units (MEAUs) and multi-energy loads. Among them, MEAUs include conventional generation, multi-energy conversion units (such as electric boiler, electric cooling, power to hydrogen, hydrogen methanation and methane fuel cell) and multi-energy storage units (such as electricity storage, cold storage, heat storage, hydrogen storage and natural gas storage). Electric and natural gas energy from renewable energy and natural gas sources are converted and stored before being delivered to user terminals. The multi-energy system has the characteristics of high efficiency, cleanliness and flexibility, compared with the traditional independent energy supply system.
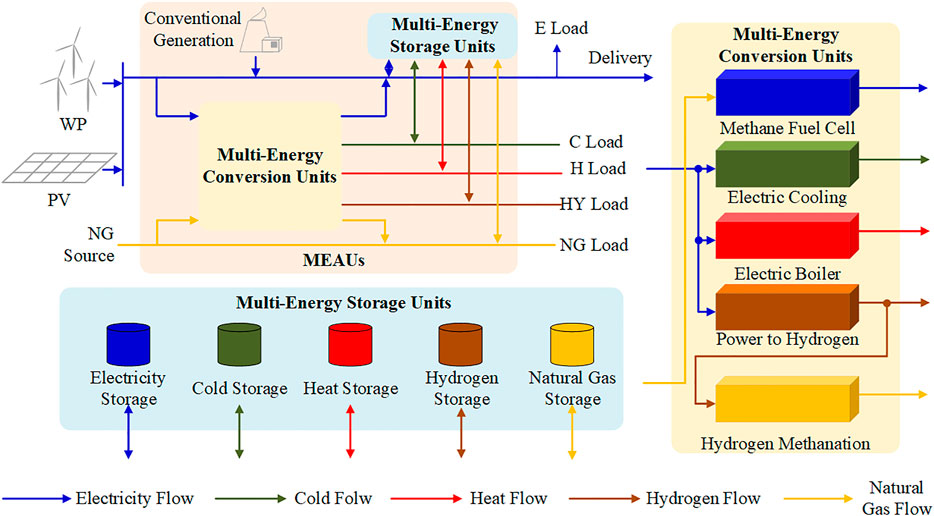
FIGURE 1. The structure of a grid with high proportion of renewable energy and multi-energy conversion and storage.
The Model of Multi-Energy Conversion Units
The model of multi-energy conversion units is:
where
The Model of Multi-Energy Storage Units
The model of multi-energy storage units is:
where
The Model of Additional Adjustment Cost
The coordinated operation of multi-energy coordinated grid with a high proportion of renewable energy needs to meet multi-energy power balance. On the one hand, it satisfies the regional multi-energy load demand and the power transmission demand; on the other hand, the multi-energy coordinated adjustment of the reserve capacity change caused by the uncertainty of renewable energy is to ensure the stable operation of the regional power grid. Therefore, the additional adjustment cost refers to the adjustment cost of MEAUs under the uncertainty of wind power and photovoltaic in multi-energy coordinated ESM. Specifically, the uncertainty of wind power and photovoltaic will lead to system adjustment requirements.
Based on the uncertainty fluctuation coefficient of wind power, the increase and decrease power of wind power are:
where
The increase and decrease power of PV are:
where
The dispatch costs of MEAUs include operating cost and additional adjustment cost.
The dispatch costs of conventional generation are:
where
The dispatch costs of multi-energy conversion units are:
where
The dispatch costs of multi-energy storage units are:
where
Partition Optimal Economic Dispatch Model of Multi-Energy System
The essence of the partition optimal economic dispatch model is to build a day-ahead dispatch model with an efficient solution for the multi-energy systems. The model can determine the output and reserve of MEAUs, and can also provide the required price signal for the electricity spot market.
Objective Function
The objective function is as follow:
where
Constraints
Power Balance Constraints
Power balance constraints refer to the multi-energy power balance, including electricity, heat, cold, hydrogen, and natural gas.
where,
Transmission Capacity Constraints
Transmission capacity constraints refer to the transmission capacity constraints in power and natural gas networks. Since active power is the main commodity traded in the ESM, the power network transmission capacity is calculated using the power transmission distribution factor. According to the laws of fluid mechanics, the calculation of natural gas network transmission capacity should be based on pipeline flow and pressure equations.
where
where
Reserve constraints
The reserve constraints refer to the upgrade reserve and downgrade reserve under certain adjustment requirements causing by the uncertainty of renewable energy.
Conventional generation constraints
Conventional generation constraints include upper and lower limits of output, ramping and start-up.
where
Multi-Energy Conversion Unit Constraints
Multi-energy conversion unit constraints refer to the power upper and lower limits of electric boiler, electric cooling, power to hydrogen, hydrogen methanation and methane fuel cell.
where
Multi-Energy Storage Unit Constraints
Multi-energy storage unit constraints refer to the power and capacity limits of electricity storage, cold storage, heat storage, hydrogen storage and natural gas storage.
where
Solving Algorithm
Lagrangian relaxation algorithm solution method is proposed, taking into account the characteristics of optimal economic dispatch model of multi-energy system. The approach carries out the optimization of the dispatch model by using multiple iterations to reduce the dual gap between the objective function and the original function. At the same time, the incentive-compatible LMP is calculated. The principle is to calculate the partial derivative of the optimal objective cost function to different nodes, and use the lagrangian multiplier in the solution process to calculate the price.
Lagrangian relaxation problem rewritten by the original objective function is:
The relaxation problem can be decomposed into 4 subproblems:
where
where
The lagrangian dual problem is:
the surrogate subgradient method is adopted to update the lagrange multiplier for solving the dual problem. The solving process is shown in Figure 2.
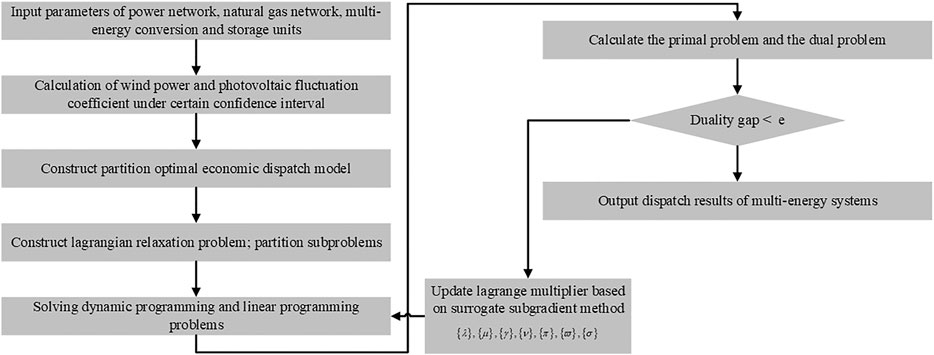
FIGURE 2. The solving process of the locational marginal price-based partition optimal economic dispatch model of multi-energy system.
In the process of solving the model, according to the definition of LMP, the node
where
The process of solving algorithm is summarised in Algorithm 1.
Case Study
Experiment Setup
In this section of paper, a simulation system coupling IEEE 39-nodes power system and 7-nodes natural gas system is established, which is shown in Figure 3. Operating data adopt an actual power grid in Northeast China. In the power network, node 38 is wind power with a capacity of 300 MW; node 35 is wind power with a capacity of 300 MW; node 37 is photovoltaic with a capacity of 300 MW; and node 31 is methane fuel cell with a capacity of 200 MW. Wind power and photovoltaic provide 42% of the total generation capacity of the test system. Typical power curves of wind power, photovoltaic and electrical loads are shown in Figure 4. The parameters of the multi-energy conversion and storage unit are shown in Table 1. The dispatching cycle is 24 h. The time interval is 1 h. The model is verified with the PC, and the host is 6148 CPU, 2.40 GHz and 128 GB RAM memory.
Two scenarios are given in this section: 1) only considering the regulation capability of conventional generations; 2) considering the regulation capability of multi-energy systems.
Simulation results
The results for the prices are showed in Figure 5 and Figure 6 respectively. Computation time are 27.38 s and 31.56 s respectively. In scenario 1, since only considered the conventional generation, the system frequently adjusts prices to provide the reserve adjustment demand. In other word, the adjustment ability to deal with uncertainty is insufficient. It can be seen from the Figure 5 that there is a large differentiation between peak electricity prices and valley electricity prices. The electricity prices fluctuate greatly, which easily leads to line congestion in power system. In particular, the maximum price of node 26 is 115.38 $/MW, and the minimum price is 38.46 $/MW over the whole dispatching cycle. The price differentiation can easily cause a decline in power transmission capacity, and the system will appear abandon curtailment of renewable energy. In contrast, the fluctuation of electricity prices is relatively small in scenario 2. Due to the system has multi-energy coordination capabilities, it can effectively deal with the uncertainty of renewable energy. Moreover, the overall price can better reflect the actual supply and demand relationship in ESM. In particular, the maximum price of node 26 is 85.23 $/MW, and the minimum price is 56.92 $/MW over the whole dispatching cycle. The transmission capacity of external power is more stable. The results of price show that the coordination ability of multi-energy system is much higher than a single conventional generation. Furthermore, the reasonable additional adjustment cost sharing mechanism makes the adjustment willingness of multi-energy adjustable units stronger. The overall system cost also lower than only considering the adjustment process of conventional generation.
Table 2 is the results of cost of conventional generations to test whether the low cost occurs in the process of optimal dispatch. For each conventional generation, the scheduling cost of scenario 2 is lower than that of scenario 1. In particular, scheduling costs of G1 and G5 with peak shaving capabilities, have obvious differences in two scenarios. It shows that the balance adjustment of supply and demand caused by the uncertainty of renewable energy is mainly undertaken by conventional generation with peak shaving capabilities, and they have to be paid higher costs. However, in scenario 2, the multi-energy adjustable units provide the system with adjustment capabilities. The system can strive to with the minimum cost for the multi-energy balance.
Figure 7 is the power of basic dispatch and reserve of G1 in two scenarios. And Figure 8 is the power of basic dispatching and reserve of G5 in two scenarios. In general, the basic dispatching and reserve of the conventional generation in scenario 2 are less than the results in scenario 1. And the reserve changes caused by wind power and photovoltaic will be undertaken by the multi-energy adjustable units during the peak load period in scenario 2. It shows that scenario 2 has more diversified adjustment means, and the multi-energy adjustable units can operate intelligently according to the price signal, which is the main reason for the decrease in system operating costs. The conventional generation still needs to make standby adjustments to the uncertainty of renewable energy during the valley load period in scenario 1, but it is not necessary in scenario 2. The adjustment power can be 0 during the valley load period in scenario 2. The main reason for the above situation is that a reasonable price signal is calculated in scenario 2, which can direct the multi-energy storage unit to peak shaving, and there is a willingness to leave a certain margin for adjustment.
Figure 9 is the comparison of total cost changes under different confidence levels of fluctuation coefficient of renewable energy in two scenarios. It can be seen that the overall operating cost of scenario 2 with multi-energy adjustable units is higher than scenario 1 when the confidence level of the fluctuation coefficient of renewable energy is in (0.1, 0.6). Since the improvement of the confidence level, the demand for adjustment of renewable energy fluctuations will increase. Scenario 1 has a single adjustment method and cannot reflect the actual supply and demand relationship. Therefore, the rising trend of the cost curve is obvious. On the contrary, the overall system cost has also increased in scenario 2. The rate of increase gradually decreases due to the stronger adjustment ability of the multi-energy adjustable units. The operating cost of scenario 2 is less than the cost of scenario 1 under a certain confidence interval, which indicates that the uncertainty of renewable energy has enormous influence on the operating cost of the system.
Figure 10 is the comparison of wind power in two scenarios. It presents the actual output of wind power in the system under two scenarios. Scenario 1 does not get the support of multi-energy systems. Therefore, the proportion of wind power is relatively small, and wind curtailment often occurs. On the contrary, the proportion of wind power generation is significantly increased due to the consideration of multi-energy regulation capabilities in scenario 2. The partition optimal economic dispatch model of multi-energy system can bring more adjustment means to the system operation. The potential of wind power will be obtained. Moreover, total operating cost of the system can be reduced.
Conclusion
In this paper, a locational marginal price-based partition optimal economic dispatch model of multi-energy system with a high proportion of renewable energy is proposed. And a solution method based on lagrangian relaxation is established for clearing and dynamic price. The results demonstrate that the proposed optimal economic dispatch model can improve the rationality of the pricing mechanism, the regulation ability of the multi-energy coordination, and the flexibility of multi-energy system.
Data Availability Statement
The raw data supporting the conclusions of this article will be made available by the authors, without undue reservation.
Author Contributions
HJ was responsible for the specific work of this paper. YT and TZ guided the work of this article. ZW and BD carried out some of the calculation work.
Funding
The authors acknowledge the funding of the National Key Research and Development Plan (2017YFB0902100).
Conflict of Interest
The authors declare that the research was conducted in the absence of any commercial or financial relationships that could be construed as a potential conflict of interest.
References
Choi, D.-H., and Xie, L. (2017). Impact of Power System Network Topology Errors on Real-Time Locational Marginal price. J. Mod. Power Syst. Clean. Energ. 5, 797–809. doi:10.1007/s40565-017-0280-5
Fang, X., and Cui, M. (2020). Analytical Model of Day-Ahead and Real-Time price Correlation in Strategic Wind Power Offering. J. Mod. Power Syst. Clean Energ. 8, 1024–1027. doi:10.35833/MPCE.2019.000598
Fang, X., Hodge, B.-M., Du, E. S., Kang, C. Q., and Li, F. (2019). Introducing Uncertainty Components in Locational Marginal Prices for Pricing Wind Power and Load Uncertainties. IEEE Trans. Power Syst. 34, 2013–2024. doi:10.1109/TPWRS.2018.2881131
Fang, X., Sedzro, K. S., Yuan, H. Y., Ye, H. X., and Hodge, B.-M. (2020a). Deliverable Flexible Ramping Products Considering Spatiotemporal Correlation of Wind Generation and Demand Uncertainties. IEEE Trans. Power Syst. 35, 2561–2574. doi:10.1109/TPWRS.2019.2958531
Fang, X. X., Yang, Z. F., Yu, J., Zhou, P., Zhao, W. J., and Lai, X. W. (2020b). Theoretical Analysis and Extension of Locational Marginal price. Proc. CSEE 40, 379–390. doi:10.13334/j.0258-8013.pcsee.190023
Faqiry, M., Edmonds, L., Wu, H., and Pahwa, A. (2020). Distribution Locational Marginal price-based Transactive Day-Ahead Market with Variable Renewable Generation. Appl. Energ. 259, 114103. doi:10.1016/j.apenergy.2019.114103
Gao, H. H., Qin, X., Barroso, R. J. D., Hussain, W., Xu, Y., and Yin, Y. (2020). Collaborative Learning-Based Industrial Iot Api Recommendation for Software-Defined Devices: the Implicit Knowledge Discovery Perspective. IEEE Trans. Emerg. Top. Comput. Intell. 99, 1–11. doi:10.1109/TETCI.2020.3023155
Gong, G. J., Wang, H. J., Zhang, T., Chen, Z. M., Wei, P. F., Su, C., et al. (2018). Research on Electricity Market about Spot Trading Based on Blockchain. Proc. CSEE 38, 6955–6966+7129. doi:10.13334/j.0258-8013.pcsee.172645
Hajiabadi, M. E., and Samadi, M. (2019). Locational Marginal price Share: a New Structural Market Power index. J. Mod. Power Syst. Clean. Energ. 7, 1709–1720. doi:10.1007/s40565-019-0532-7
Hanif, S., Zhang, K., Hackl, C. M., Barati, M., Gooi, H. B., and Hamacher, T. (2019). Decomposition and Equilibrium Achieving Distribution Locational Marginal Prices Using Trust-Region Method. IEEE Trans. Smart Grid 10, 3269–3281. doi:10.1109/TSG.2018.2822766
Jiang, Z., and Ai, Q. (2017). Agent-based Simulation for Symmetric Electricity Market Considering price-based Demand Response. J. Mod. Power Syst. Clean. Energ. 5, 810–819. doi:10.1007/s40565-017-0270-7
Jovanovic, N., Garcia-Gonzalez, J., Barquin, J., and Cerisola, S. (2017). Electricity Market Short-Term Risk Management via Risk-Adjusted Probability Measures. IET Generation Transm. Distribution 11, 2599–2607. doi:10.1049/iet-gtd.2016.1731
Morstyn, T., Teytelboym, A., Hepburn, C., and McCulloch, M. D. (2020). Integrating P2P Energy Trading with Probabilistic Distribution Locational Marginal Pricing. IEEE Trans. Smart Grid 11, 3095–3106. doi:10.1109/TSG.2019.2963238
Peng, X., and Tao, X. (2018). Cooperative Game of Electricity Retailers in China's Spot Electricity Market. Energy 145, 152–170. doi:10.1016/j.energy.2017.12.122
PrudhvirajKiran, D. P. B. S., and Pindoriya, N. M. (2020). Stochastic Energy Management of Microgrid with Nodal Pricing. J. Mod. Power Syst. Clean Energ. 8, 102–110. doi:10.35833/MPCE.2018.000519
Teng, Y., Sun, P., Luo, H. H., and Chen, Z. (2019a). Autonomous Optimization Operation Model for Multi-Source Microgrid Considering Electrothermal Hybrid Energy Storage. Proc. CSEE 39, 5316–5324+5578. doi:10.13334/j.0258-8013.pcsee.181922
Teng, Y., Wang, Z. D., Jin, H. Y., Zhou, G. P., and Chen, Z. (2019b). A Model and Coordinated Optimization for the Multi-Energy Storage System of Electricity Heat Hydrogen to Regulation Enhancement of Power Grid. Proc. CSEE 39, 7209–7217+7494. doi:10.13334/j.0258-8013.pcsee.182564
Teng, Y., Wang, Z., Li, D. Y., Ma, Q., Hui, Q., and Li, S. B. (2019c). Multi-energy Storage System Model Based on Electricity Heat and Hydrogen Coordinated Optimization for Power Grid Flexibility. CSEE J. Power Energ. Syst. 5, 266–274. doi:10.17775/CSEEJPES.2019.00190
Vaskovskaya, T., Thakurta, P. G., and Bialek, J. (2018). Contribution of Transmission and Voltage Constraints to the Formation of Locational Marginal Prices. Int. J. Electr. Power Energ. Syst. 101, 491–499. doi:10.1016/j.ijepes.2018.04.004
Yang, X. X., Zhou, S. J., and Cao, M. (2020). An Approach to Alleviate the Sparsity Problem of Hybrid Collaborative Filtering Based Recommendations: the Product-Attribute Perspective from User Reviews. Mobile Netw. Appl. 25, 376–390. doi:10.1007/s11036-019-01246-2
Zhang, N., Sun, Q. Y., Wang, J. W., Yang, L. X., Sun, Q., Wang, J., et al. (2021). Distributed Adaptive Dual Control via Consensus Algorithm in the Energy Internet. IEEE Trans. Ind. Inf. 17, 4848–4860. doi:10.1109/TII.2020.3031437
Keywords: multi-energy systems (MES), renewable energy, locational marginal price, economic dispatch, lagrangian relaxation algorithm
Citation: Jin H, Teng Y, Zhang T, Wang Z and Deng B (2021) A locational Marginal Price-Based Partition Optimal Economic Dispatch Model of Multi-Energy Systems. Front. Energy Res. 9:694983. doi: 10.3389/fenrg.2021.694983
Received: 14 April 2021; Accepted: 24 May 2021;
Published: 15 June 2021.
Edited by:
Ying Li, Zhejiang University, ChinaReviewed by:
Mingyang Lu, The University of Manchester, United KingdomXinhao Che, Dalian University of Technology, China
Jingwei Hu, Northeastern University, China
Kun Ma, Tsinghua University, China
Copyright © 2021 Jin, Teng, Zhang, Wang and Deng. This is an open-access article distributed under the terms of the Creative Commons Attribution License (CC BY). The use, distribution or reproduction in other forums is permitted, provided the original author(s) and the copyright owner(s) are credited and that the original publication in this journal is cited, in accordance with accepted academic practice. No use, distribution or reproduction is permitted which does not comply with these terms.
*Correspondence: Yun Teng, dGVuZ3l1bkBzdXQuZWR1LmNu