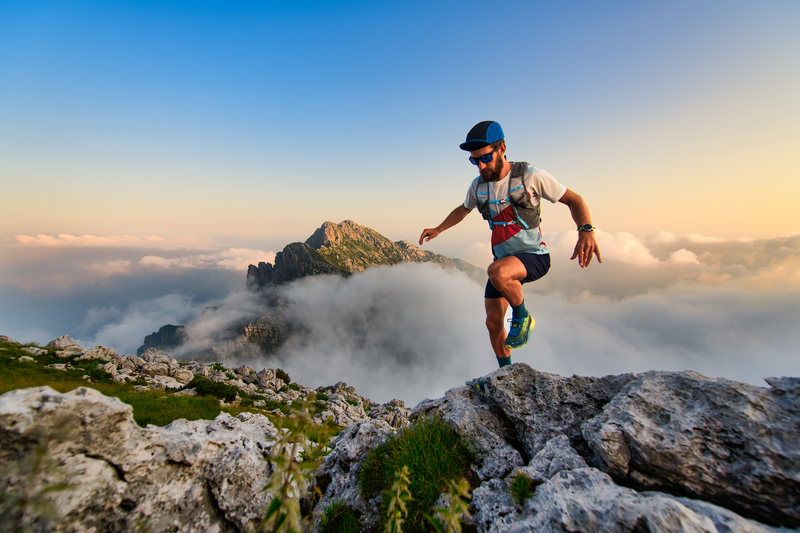
95% of researchers rate our articles as excellent or good
Learn more about the work of our research integrity team to safeguard the quality of each article we publish.
Find out more
ORIGINAL RESEARCH article
Front. Energy Res. , 03 May 2021
Sec. Sustainable Energy Systems
Volume 9 - 2021 | https://doi.org/10.3389/fenrg.2021.683166
This article is part of the Research Topic Progress Towards Achieving Cross-Regional Carbon Mitigation Targets View all 14 articles
This study empirically investigates the impact of industrial structure upgrading on global carbon dioxide (CO2) emissions by employing a balanced dataset of 73 countries over the period 1990–2019. After conducting a series of empirical tests, we used the fixed effect (FE) and random effect (RE) methods to estimate the econometric model, and divided the full sample data into two subsamples, i.e., Regional Comprehensive Economic Partnership (RCEP) countries and non-RCEP countries, for heterogeneous analysis. This study also examines the mediating role of technological innovation in the relationship between industrial structure upgrading and global CO2 emissions. The main findings indicate that: (1) both industrial structure upgrading and technological innovation show significant negative impacts on CO2 emissions in the global panel, the RCEP countries, and the non-RCEP countries; (2) industrial structure upgrading not only affects CO2 emissions directly, but also has an indirect impact on global CO2 emissions by promoting technological innovation; and (3) the environmental Kuznets curve (EKC) hypothesis is verified in this study; in other words, both economic growth and CO2 emissions exhibit a significant inverted U-shaped relationship in the global panel, the RCEP countries, and the non-RCEP countries. Finally, we highlighted some important policy implications with respect to promoting industrial structure upgrading and mitigating the greenhouse effect.
The past few decades have witnessed unparalleled economic growth due to rapid industrialization across the globe. According to the statistics from the former British Petroleum company (BP, 2020), along with this boom in economics, the primary energy consumption has increased nearly twofold worldwide, from 7,820.7 million tons of oil equivalent (Mtoe) in 1990 to 13,301.4 Mtoe in 2019. This rapid increase in energy consumption has triggered tremendous challenges related to the global environmental pressures (Cheng et al., 2019; Dong et al., 2020a; Zhao et al., 2021a), particularly the greenhouse effect. To be specific, global carbon dioxide (CO2) emissions increased from 21,331.5 million tons (Mt) in 1990 to 34,169.0 Mt in 2019 (BP, 2020). Since CO2 is the main contributor to the greenhouse effect, lowering CO2 emissions has become a critical issue that needs to be addressed all over the world. Many scholars have investigated the driving factors of CO2 emissions; among these, industrial structure upgrading has been widely accepted as an effective pathway to realize low-carbon development (Du et al., 2012; Tian et al., 2019; Zhao et al., 2020a; Ren et al., 2021). In this study, the definition of industrial structure upgrading is that the national industrial structure shifts from low value-added labor-intensive industries to high value-added technology-intensive industries; in other words, industrial structure upgrading refers to the process or trend of the transformation of the industrial structure from a low-level form to the high-level form. From the perspective of changes in the national industrial structure, industrial structure upgrading means that the national economic barycenter moves from primary industry to secondary industry, and then to tertiary industry. Since this process is usually accompanied by the development of high-tech industries, many scholars believed that it can mitigate environmental pressure caused by CO2 emissions (Cheng et al., 2018; Peng et al., 2018; Zhou et al., 2018). However, to the best of our knowledge, the existing literature on the impact of industrial structure upgrading on CO2 emissions is based mainly on Chinese cases, and very few studies on this issue are obtained from the global perspective.
Recently, the signing of the largest Free Trade Agreement (FTA) – the Regional Comprehensive Economic Partnership (RCEP) agreement – has attracted attention worldwide. The signing of the RCEP agreement provides a favorable platform to promote friendly exchanges, strengthen cooperation, and jointly promote the transformation of industrial structure and achieve carbon emission reduction targets. Its member countries cover 10 Association of Southeast Asian Nations (ASEAN) countries and include China, Japan, South Korea, Australia, and New Zealand. The member countries of the RCEP agreement account for nearly half of the world population and comprise the most diverse membership structure in the world. Under the framework of the RCEP, China and other RCEP member countries will accelerate the formation of an expanded version of the “world factory,” and the agglomeration effect of the industrial chain and the supply chain will be further amplified. Based on the above circumstances, the signing of the RCEP can significantly boost the high-end manufacturing industry chains of member countries, which plays an important role in their national industrial structure upgrading. Thus, the organization of the RCEP countries may have the most development potential. Furthermore, since several RCEP countries (e.g., Japan, South Korea, and Singapore) have relatively higher technology levels, the technology spillover effect might be stronger among the RCEP countries. These factors indicate that heterogeneity may exist between the RCEP countries and the non-RCEP countries in the nexus between industrial structure upgrading and CO2 emissions. However, very few studies have systematically examined the impact of technological innovation on the industrial structure upgrading–CO2 nexus, and heterogeneity is often overlooked in the existing literature.
To fill the academic gaps discussed earlier, this study first investigates the impact of industrial structure upgrading on global CO2 emissions by employing a balanced panel dataset covering 73 countries for the period 1990–2019, and then divides the full sample into two subsamples (i.e., the RCEP and non-RCEP countries) to examine the heterogeneous impact of industrial structure upgrading on CO2 emissions. This study also investigates the mediating effect of technological innovation in the industrial structure upgrading–CO2 emissions nexus. Accordingly, this study contributes to the existing literature in the following three aspects. (1) From the global perspective, after a series of tests, we empirically investigated the impact of industrial structure upgrading on CO2 emissions and then identified the specific causal relationship between industrial structure and its determinants, which provides more generalized policy implications for reducing global CO2 emissions. (2) By dividing the full sample into two subregions, i.e., RCEP and non-RCEP countries, we examined the heterogeneous impact of industrial structure upgrading on CO2 emissions. This not only effectively provides evidence for policymakers to implement specific policies for carbon reduction, but also helps the RCEP countries to develop a low-carbon economic growth pattern. (3) To identify the internal impact channels between industrial structure upgrading and CO2 emissions, we conducted the mediation effect in the industrial structure upgrading–CO2 nexus by considering technological innovation as mediating variable, which can help advance our understanding of the impact mechanism between industrial structure upgrading and CO2 emissions and facilitate the formulation of more compatible policies to reduce CO2 emissions.
The remainder of this study is organized as follows. The “Literature review and research gap” section covers the literature review. The “Econometric model, method, and data” section provides the empirical model, estimation strategy, and data. The “Empirical results” section reports the empirical results. The “Further discussion on the mediating effect between industrial structure upgrading and CO2 emissions” section further discusses the mediating effect between industrial structure upgrading and CO2 emissions. The “Conclusions and policy implications” section concludes this study and provides some implications.
In recent years, a growing body of scholars has shed light on the impact of industrial structure upgrading on CO2 emissions. For instance, based on the STIRPAT framework, Li et al. (2017) found a significant negative correlation between industrial structure upgrading and CO2 emissions in China. By employing a dataset covering 50 cities in China, Li et al. (2018) investigated the impact of industrial structure change on CO2 emissions, and proposed that industrial structure change can significantly reduce CO2 emissions. Furthermore, Tian et al. (2019) utilized the methods of input–output analysis (IOA) and structural decomposition analysis (SDA) to investigate the impact of industrial structure change on CO2 emissions in southwest China. Their empirical results show that secondary industries (e.g., construction and manufacturing) are the main contributors of CO2 emissions, and that industrial structure upgrading is conducive to carbon reduction, assertions that are supported by many other scholars who have also conducted analyses based on Chinese case (Chen et al., 2011; Yang et al., 2014; Chang, 2015; Liu et al., 2015; Zhang et al., 2020).
Additionally, some other researchers have examined the relationship between industrial structure and CO2 emissions at the provincial level. For example, Zhang and Ren (2011) found a long-run equilibrium relationship between industrial structure and CO2 emissions in Shandong Province and stated that industrial structure change could unidirectionally affect CO2 emissions. Considering potential nonlinear characteristics, Wei and Zhang (2020) examined the threshold effect of industrial structure upgrading on CO2 emissions based on the panel smooth transition regression model in Chinese 30 provinces for the period 1997–2015. The results indicate that the increase in the proportion of tertiary industry has an obvious positive impact on CO2 emissions, while the increased proportion of the secondary industry would slow down the trend of CO2 emissions. It is obvious that the current literature on the nexus between industrial structure and CO2 emissions is based mainly on the Chinese case. Thus, the earlier conclusions have limitations, and their policy implications are not generalized for most global countries. In addition, very few studies have mentioned the impact of external shocks (e.g., the signing of the RCEP) on the nexus observed, which may cause the estimation bias and make the results inaccurate.
In addition to industrial structure upgrading, some other factors have been found to contribute significantly to CO2 emissions. These factors include technological innovation, economic growth, trade openness, and population size. Specifically, many scholars have investigated the impact of technological innovation on CO2 emissions and consistently concluded that technological innovation can significantly mitigate CO2 emissions (York et al., 2003; Zhao et al., 2010; Irandoust, 2016; Yii and Geetha, 2017; Cheng et al., 2020). As for the economic growth–CO2 nexus, scholars in this field have largely accepted the well-known environmental Kuznets curve (EKC) hypothesis proposed by Grossman and Krueger (1993). The Kuznets curve clearly depicts an inverted U-shaped relationship between economic growth and CO2 emissions (Zhang et al., 2017; Balado-Naves et al., 2018; Dong et al., 2018a; Leal and Marques, 2020). With regard to the impact of trade openness on CO2 emissions, there has been some disparity among various studies. Some scholars believed that trade openness would produce more CO2 emissions due to its positive impact on the production of goods in a country, which requires massive energy consumption and leads to more CO2 emissions (Ertugrul et al., 2016; Ahmed et al., 2017). However, other researchers reached an opposing conclusion based on empirical results, which indicate that trade openness can reduce CO2 emissions (Gozgor, 2017; Ho and Iyke, 2019; Lv and Xu, 2019). These scholars argued that trade openness is conducive to expanding the technology spillover effect between global countries, which consequently improves their national energy efficiency. Moreover, many scholars have investigated the role of population in CO2 emissions, and claimed that population has a significantly positive impact on CO2 emissions (Ghazali and Ali, 2019; Wang et al., 2019; Dong et al., 2020b). Other factors are also considered as contributing to CO2 emissions. These include population aging (Menz and Welsch, 2012; Wang et al., 2017; Yu et al., 2018), natural gas consumption (Alkhathlan and Javid, 2013; Saboori and Sulaiman, 2013; Dong et al., 2018b), and urbanization (Liu and Bae, 2018; Bai et al., 2019). However, these variables are not considered in this study.
Based on the literature review discussed earlier, although many scholars have examined the nexus between industrial structure upgrading and CO2 emissions, certain research gaps still exist. First, to the best of our knowledge, earlier studies on the nexus between industrial structure upgrading and CO2 emissions were based mainly on Chinese case; very few studies investigated the impact of industrial structure upgrading on CO2 emissions from a global perspective, which can provide more generalized evidence for policymakers to implement effective policies for carbon reduction. Second, the existing literature neglects the influence of the signing of the RCEP on the nexus between industrial structure upgrading and CO2 emissions. In other words, few studies explore the heterogeneity between RCEP and non-RCEP countries on this issue, which may cause bias in the estimation results. Third, very few studies have explored the impact mechanism of industrial structure upgrading on CO2 emissions. In particular, the role of technological innovation in the industrial structure upgrading–CO2 nexus remains unclear.
To explore the impact of industrial structure upgrading on CO2 emissions, this study conducts an empirical analysis by constructing an econometric model, where CO2 emissions are utilized as the dependent variable, and industrial structure upgrading is used as the core independent variable. Based on the literature review discussed earlier, technological innovation, economic growth, trade openness, and population size are introduced as control variables. Furthermore, to verify the EKC hypothesis in this study, we also introduced the quadratic term of economic growth into the model. Therefore, the multivariate framework can be written as follows:
where subscripts i and t represent the country and year, respectively. CO2 represents the CO2 emissions of each country, ISU indicates industrial structure upgrading, Tec denotes technological innovation, Pgdp refers to economic growth, Tra means trade openness, and Pop represents the population of each country.
Furthermore, to eliminate possible heteroscedasticity in the model, this study takes all the variables in Eq. (1) into logarithmic form as follows:
where β0 and εit denote the constant and random error terms, respectively. β1–β6 are the coefficients to be estimated. X stands for a vector that contains a series of control variables, i.e., technological innovation, economic growth and its square term, trade openness, and population size.
To investigate the impact of industrial structure upgrading on CO2 emissions across the globe, the estimation strategies in this study consist of four steps: (1) the Breusch–Pagan Lagrange multiplier (LM) test and the Pesaran cross-sectional dependence (CD) test are simultaneously conducted to examine CD within the panel data (see the “CD tests” section); (2) two panel unit root tests, i.e., the Pesaran cross-sectionally augmented Dickey–Fuller (CADF) and cross-sectionally augmented Im, Pesaran, and Shin (CIPS) tests, are utilized to examine the stationarity of the selected variables (see the “Panel CADF and CIPS unit root tests” section); (3) both the fixed effect (FE) and random effect (RE) estimation methods are employed to estimate the impact of industrial structure upgrading on CO2 emissions (see the “FE and RE estimates” section); and (4) to detect the causal relationship between all the variables, the Dumitrescu–Hurlin (D-H) causality test is conducted in this study (see the “D-H panel causality test” section).
Since countries around the world are more linked due to the rapid globalization of trade and economics, a shock affecting one country might cause economic fluctuations in other countries. This means CD may exist in the global panel data, which can cause biased and even inconsistent estimates of the econometric model (Dong et al., 2019, 2021; Jiang et al., 2020). Therefore, to obtain consistent estimations, this study first conducts two CD tests, that is, the Breusch–Pagan LM test developed by Breusch and Pagan (1980) and the Pesaran CD test proposed by Pesaran (2004) to examine the existence of CD in the panel data.
Before estimating the parameters in the model, it is necessary to ensure that all the selected variables in this study are stable. Considering the potential CD in the panel data, two updated panel unit root tests (i.e., the Pesaran CADF and CIPS tests) are conducted to examine the stationarity of the variables. To be specific, the Pesaran CIPS test is proposed by Pesaran (2007) and the test statistics for the Pesaran CIPS can be obtained as follows:
where and represent the cross-sectional averages of lagged levels and first differences of individual series, respectively. From the CADF, the CIPS test statistics can be calculated as follows:
where CADFi represents the t-statistic in the CADF regression defined by Eq. (3).
Considering multiple individuals are involved in the panel data, the different individual effects in the econometric model might cause bias in the process of estimation. Thus, we employed two conventional static panel data estimation approaches (i.e., the FE and RE methods) to calculate the estimated parameters in Eq. (2). Moreover, both the FE and RE estimation methods could avoid the problem of omitted variables by eliminating the impact from individual effects. Technically, the FE model is suitable for the conditions where the individual factors are correlated with some independent variables, while the RE model is appropriate for the situation where the individual factors are irrelevant with all the independent variables. Based on this, before applying the two estimation methods, it is necessary to figure out which method fits the panel data in this study. Following the specification test principle proposed by Hausman (1978), we utilized the Hausman test to identify the more effective estimates. Specifically, the null hypothesis of the Hausman test is a non-correlation between individual factors and the independent variables, which means the RE estimates are more effective. Additionally, to detect whether the signing of the RCEP affects the nexus between industrial structure upgrading and CO2 emissions, this study further estimates the econometric model based on two subsamples (i.e., the RCEP countries and the non-RCEP countries). By comparing the estimation results of the full panel sample and the two subsamples, we conducted a heterogeneous analysis of the impact of industrial structure upgrading on CO2 emissions.
The causal relationship between CO2 emissions and their determinants cannot be verified merely from a regression equation. Thus, Granger (1969) proposed a causality test to examine causalities between the selected variables in the empirical model. The results of the causality tests are particularly useful for policymakers to formulate specific policies. Since CD may exist in the panel data, the D-H panel causality test developed by Dumitrescu and Hurlin (2012) is used in this study. The test can also deal with the heterogeneity in the slope coefficients in the model.
This study employs a balanced panel dataset covering 73 countries over the period 1990–2019 to investigate the impact of industrial structure upgrading on CO2 emissions. Notably, other countries are not considered due to unavailability of data. Moreover, considering the potential heterogeneity between the RCEP countries and the non-RCEP countries, this study further divides the full panel into two subsamples, the RCEP countries (12 countries) and the non-RCEP countries (61 countries); the specific countries are highlighted in Appendix Table A1.
The data on CO2 emissions (denoted as CO2) are sourced from the BP Statistical Review of World Energy (BP, 2020). Industrial structure upgrading (denoted as ISU) is calculated by the ratio of the added value of tertiary industry to secondary industry, and the data are obtained from the World Development Indicators (WDI) published by the World Bank (2020). Technological innovation (denoted as Tec) is measured by the proportion of gross domestic product (GDP) to energy consumption, where the data on energy consumption are obtained from BP (2020), while the data on GDP are obtained from the World Bank (2020). Economic growth (denoted as Pgdp) is expressed by per capita GDP, trade openness (denoted as Tra) is measured by the ratio of total imports and exports to GDP, and population (denoted as Pop) is measured by the national population of each country. The data on economic growth, trade openness, and population were collected from the World Bank (2020). In sum, the detailed description of the variables is listed in Table 1, while the descriptive statistics (i.e., mean value, SD, maximum value, and minimum value) of the variables are illustrated in Table 2.
The estimation results of the CD tests for the global panel and the two subsamples are all reported in Table 3. As the table shows, the statistics of the Breusch–Pagan LM tests and the Pesaran CD tests are all larger than the critical values at the 1% significance level, which confirms strong CD for the full panel and the groups of the RCEP and non-RCEP countries. Therefore, when conducting the following empirical analysis, the CD in the three panels (i.e., the full panel and two subpanels) should be fully considered.
Following the discussions earlier, the CD is verified in both the full panel and the two subpanels (the RCEP and non-RCEP countries). Thus, the panel CADF and CIPS unit root tests are valid and more suitable in this study to examine the stationarity of the selected variables. The results of the two tests for the full panel and the two subsamples of the RCEP and non-RCEP countries are listed in Table 4. From the table, the variables of the full panel sample are not all stable at the level; however, after taking the first-order difference, all the series data are stable due to the significant statistics of the panel unit root tests. Similar results are obtained for the subsamples of the RCEP countries and non-RCEP countries. Therefore, the selected variables in this study are integrated of order [i.e., I (1)], for both the full sample data and the two subsamples. This ensures stable estimation of the empirical model and avoids the problem of spurious regression. To sum up, the econometric model we built in this study is valid and reliable for the full panel sample and the two subsamples, respectively, due to the same order of integration of the selected variables in the three samples.
Based on the empirical analysis earlier, this study then investigates the nexus between industrial structure upgrading and CO2 emissions by estimating Eq. (2) for the global panel. Furthermore, technological innovation may have a significant impact on industrial structure upgrading–CO2 emissions. To verify this, we first eliminated technological innovation in Eq. (2) and then reestimated the model by introducing it into an empirical framework. The results of the FE and RE estimates are displayed in Table 5. Specifically, the first and second columns of the table report the estimation results for the empirical model without technological innovation based on the FE and RE methods, respectively; the third and fourth columns of the table present the results of the FE and RE estimates for the model with technological innovation, respectively. Also, the estimation results of the Hausman tests are reported in the table. From the table, the FE estimates are more effective due to the significant statistics of the Hausman tests for the model both before and after technological innovation is introduced. Therefore, the FE estimates are considered the benchmark estimation results for the global panel. Notably, the results of the RE estimates are basically consistent with the FE estimates, which indicates that the estimation results of Eq. (2) are robust and stable.
As for the impact of industrial structure upgrading on global CO2 emissions, it is obvious that industrial structure upgrading can significantly reduce CO2 emissions. This is rational because, with rapid industrialization around the world, industrial structure upgrading appears as a shift from secondary to tertiary industries. To the best of our knowledge, the secondary industry usually has the characteristics of high pollution and high emissions, while the tertiary industry shows the advantages of high added value and low pollution (Zhao et al., 2021b). The secondary industries consist mainly of industry and construction, which are the main contributors of CO2 emissions in the world. However, the tertiary industries are dominated mainly by the service sectors (e.g., computer services and software, information transmission, and financial), which are more efficient and emit low carbon. Accordingly, the promotion of transformation from secondary industry to tertiary industry can play an important role in carbon emission reduction; in other words, industrial structure upgrading is conducive to carbon reduction. Thus, it is necessary for policymakers to promote the shift of industrial structure from secondary to tertiary industries, which will reduce CO2 emissions and achieve green economic development. Furthermore, it is noteworthy that when technological innovation is introduced into the empirical model, the estimated coefficient of industrial structure upgrading (i.e., ISU) shrinks a lot. This indicates that the impact of industrial structure upgrading on CO2 emissions might be attributed partly to technological innovation. At the same time, accelerating technological innovation can significantly reduce CO2 emissions. In other words, industrial structure upgrading may not only affect CO2 emissions directly, but also influence CO2 emissions through technological innovation.
As for the control variables, the estimated coefficients of technological innovation (i.e., Tec), economic growth (i.e., Pgdp), trade openness (i.e., Tra), and population (i.e., Pop) are all significant, and their signs basically coincide with the actual conditions. Specifically, both technological innovation and trade openness can significantly reduce global CO2 emissions due to their negative estimated coefficients. This is rational because both of them can improve production efficiency and optimize the production procedure, which are conducive to reducing energy use while maintaining the current production level. Since energy use is the main source of CO2 emissions, the decline in energy utilization will certainly slow the growth of CO2 emissions. As for economic growth, this study confirms the EKC hypothesis according to the positive coefficient of economic growth and the negative of its quadratic term, which indicates that an inverted U-shaped relationship exists between economic growth and CO2 emissions. Specifically, during the initial stage, rapid economic growth is anchored by massive energy consumption, which is accompanied by large amounts of CO2 emissions. However, as national economic growth rises to a certain level, the environmental regulation tends to be more intensified, considering the consequences of the greenhouse effect. Furthermore, public awareness of energy conservation and emission reduction tends to be greater when their income level improves. These factors result in a downward trend of CO2 emissions after economic growth crosses a certain value (i.e., a turning point). The results also indicate that population has a significantly positive impact on CO2 emissions. This is because rapid population growth causes more demand for energy consumption, particularly for some developing countries whose energy efficiency is relatively low. This certainly would cause more CO2 emissions and would not mitigate the greenhouse effect.
After estimating the causal nexus between industrial structure upgrading on CO2 emissions based on the global panel, this study further reestimates the benchmark model [i.e., Eq. (2)] based on the two subsamples (i.e., the RCEP and non-RCEP countries), respectively. The estimation results are listed in Table 6. Similarly, the FE and RE estimates are simultaneously employed for the models before and after technological innovation is introduced into the empirical framework. And from the results of the Hausman tests, the FE estimates are still preferred as the benchmark estimations for both the RCEP and non-RCEP countries.
As for the RCEP countries, industrial structure upgrading still has a significantly negative impact on CO2 emissions, which is consistent with the results for the global panel. However, compared with the estimated results in for the full sample, the absolute value of the coefficient of industrial structure upgrading is larger, which indicates that the impact of industrial structure upgrading on CO2 emissions is greater for the RCEP countries. This might be because, among the RCEP countries, some developed countries (e.g., Australia, Japan, South Korea, and Singapore) are at or near the stage of post-industrial society. This means most of these countries have successfully shifted their national structure of manufacturing industry from capital-intensive industries to technology-intensive industries; thus, these countries have relatively higher technology levels. At the same time, people’s lifestyles in these countries are more modernized. Additionally, the signing of the RCEP will greatly strengthen the links among member countries, and the technology spillover among these countries will be stronger than in other countries. Based on this, the marginal effect of industrial structure upgrading on carbon reduction in the RCEP countries is larger than that of countries in other regions. Furthermore, similar to the global panel, it is obvious that the coefficient of industrial structure upgrading shrinks after technological innovation is introduced into the model. This implies that the impact of industrial structure upgrading on CO2 emissions is affected by technological innovation. The significance and signs of the coefficients of other control variables are consistent with those of the global panel, which is in line with the actual conditions of the RCEP countries.
With respect to the non-RCEP countries, the significance and absolute values of the coefficients of the independent variables are almost the same as the global panel. This implies that, in both RCEP and non-RCEP countries, the upgrading of industrial structure is conducive to the realization of carbon emission reduction targets. Furthermore, the evident difference of the coefficients between the RCEP countries and other global countries highlights the significance of the signing of the RCEP on the nexus between industrial structure upgrading and CO2 emissions. To conclude, the impact of industrial structure upgrading on CO2 emissions is heterogeneous between the RCEP countries and the non-RCEP countries. Thus, policymakers should adjust measures to local conditions when implementing policies for carbon reduction.
The results of the D-H panel causality tests for the global panel and the two subpanels are listed in Table 7. In addition, to clearly display the causal relationships between the selected variables, we also depicted the causality flows in Figure 1. As the figure shows, bidirectional causality runs between any two of the variables for the global panel and non-RCEP countries, which indicates that the correlations between global CO2 emissions and their determinants are getting much closer due to the tightening link of economic activities and environmental quality. However, as for the RCEP countries, the causality is unidirectional, running from technological innovation to CO2 emissions and from technological innovation to economic growth. The varying causal relationships among the different samples again verify the existence of heterogeneity between the RCEP and non-RCEP countries. This implies that policymakers should implement specific policies to reduce CO2 emissions.
To effectively investigate the impact of industrial structure upgrading on CO2 emissions, it is necessary to explore the specific impact mechanism behind the observed relationship. Furthermore, based on the discussions on the estimation results in the “Results of the industrial structure upgrading–CO2 nexus” section, the coefficient of industrial structure upgrading changes a lot after technological innovation is introduced into the empirical model, which indicates that technological innovation might be a mediating factor between industrial structure upgrading and CO2 emissions. To verify this, this study further conducts a mediating effect analysis on the nexus between industrial structure upgrading and CO2 emissions. Following the work by Zhao et al. (2020b), we constructed the mediating effect model as follows:
where X indicates a vector of control variables, including economic growth and its square term, trade openness, and population. εit represents the random disturbance term in three equations [i.e., Eqs. (5)–(7)]. The logic of the mediating effect model lies in that the direct effect of industrial structure upgrading on CO2 emissions is reflected from the parameter δ1 in Eq. (7), and the indirect effect through technological innovation is valid if the parameters ϕ1 in Eq. (6) and δ2 in Eq. (7) are simultaneously significant. Also, to detect whether the impact mechanism varies with different panel data, we conducted a mediating effect analysis based on the global panel and the two subsamples (i.e., the RCEP and non-RCEP countries), respectively.
The estimation results of the mediating effect model are reported in Table 8. In this table, Models (1)–(3) correspond to Eqs. (5)–(7), respectively. As the table shows, the coefficients of industrial structure upgrading in Eqs. (5) and (6) and technological innovation in Eq. (7) are all significant, no matter which sample data are employed. Thus, industrial structure upgrading not only affects CO2 emissions directly, but can also indirectly affect CO2 emissions through technological innovation. Specifically, industrial structure upgrading can significantly promote the development of technological innovation, while technological innovation has a significantly negative impact on CO2 emissions. This is rational because industrial structure upgrading means the booming of service sectors, such as information transmission and financial; these industries, compared with secondary industries, are technology and knowledge intensive, and their current boom is accelerating the process of national technological innovation. Similar to the discussions in the “Results of the industrial structure upgrading–CO2 nexus” section, technological innovation can significantly reduce CO2 emissions. Therefore, the indirect impact of industrial structure upgrading on CO2 emissions is negative.
In addition, it is worth noting that the specific impact mechanism between industrial structure upgrading and CO2 emissions of RCEP countries is slightly different from that of global countries and non-RCEP countries. Specifically, for RCEP countries, the coefficient of industrial structure in Eq. (6) is larger than that of the global panel and the non-RCEP countries. This verifies the existence of a technology spillover effect among the RCEP countries, which again indicates that the impact of industrial structure upgrading on CO2 emissions is heterogeneous between the RCEP countries and non-RCEP countries; the specific impact mechanism between industrial structure upgrading and CO2 emissions is presented in Figure 2.
By employing a balanced panel dataset of 73 countries over the period 1990–2019, this study empirically investigates the impact of industrial structure upgrading on CO2 emissions across the globe. After conducting a series of empirical tests, this study uses the conventional panel data estimation methods, i.e., the FE and the RE methods. Moreover, we also explored the impact mechanism between industrial upgrading and CO2 emissions by employing the mediating effect model. And to detect heterogeneity between the RCEP and non-RCEP countries, we also conducted a series of comparative analyses on industrial structure upgrading–CO2 emissions nexus. Several interesting findings are highlighted as follows.
First, upgrading industrial structure can significantly reduce global CO2 emissions, which provides new evidence for policymakers to promote this process to reduce carbon emissions. Furthermore, the marginal impact of upgrading industrial structure on CO2 reduction is larger for the RCEP countries, which indicates that heterogeneity exists in the nexus between industrial structure upgrading and CO2 emissions.
Second, this study verifies the EKC hypothesis; in other words, an inverted U-shaped relationship exists between economic growth and global CO2 emissions. Thus, there exists a threshold value (i.e., a turning point) between economic growth and CO2 emissions, and CO2 emissions increase initially and then decline as the economic growth crosses the threshold value.
Third, technological innovation is verified as a significant mediating variable between industrial structure upgrading and CO2 emissions. To be specific, upgrading industrial structure not only affects CO2 emissions directly, but can also have an indirect impact on CO2 emissions through technological innovation. Furthermore, the different estimation results between the RCEP countries and non-RCEP countries again verify the existence of heterogeneity in the relationship between industrial structure upgrading and CO2 emissions.
Based on the findings earlier, several policy implications are provided as follows.
First, since upgrading industrial structure contributes significantly to carbon mitigation, it is necessary for policymakers to implement effective and specific policies to encourage the development of the modern service industry and limit the expansion of secondary industries, especially those with high energy consumption. For example, the government can provide targeted subsidies to promote the development of service industries and set a series of environmental regulations for high-emission secondary industries to promote energy conservation and carbon reduction. The government also should work to eliminate traditional high-polluting sunset industries, formulate relevant promotion measures for emerging sunrise industries, provide sufficient scientific R&D funds, and lay a solid foundation for the subsequent sustainable and green transformation of industrial structure. Furthermore, as for the RCEP countries, it is helpful to expand the technology spillover effect among the relevant countries by promoting technology trade among these countries.
Second, although economic growth is anchored by massive energy consumption and carbon emissions, CO2 emissions would eventually show a downward trend due to technology development fueled by economic growth. This emphasizes the importance of the green economy to a certain extent. Thus, governments of various countries should strive to promote environmental protection while maintaining sustained economic growth. Furthermore, new industrialization paths should be developed by changing the patterns of the industry. Specifically, the percentage of high-tech industries, such as information transmission and financial, should be improved to accelerate economic development and upgrade the national industrial structure.
Third, technological innovation should be promoted due to its role in the mediation effect mechanism between industrial structure upgrading and CO2 emissions. Some low-carbon and high-efficiency technologies should be improved through development, demonstration, and promotion. For example, governments should encourage enterprises to establish scientific management systems to improve information levels and operational efficiencies. Moreover, R&D technologies for clean, renewable energy and exploration technologies for natural gas, coal bed methane, and shale gas should also be promoted to rationalize the energy consumption structure.
However, this study provides only preliminary empirical evidence on the industrial structure upgrading–CO2 nexus, and there exist some limitations. One such limitation is the empirical method. In this study, we employed the static regression model (e.g., FE and RE estimation), and in future research, we tend to consider the dynamic linkage between industrial structure upgrading and CO2 emissions. Another limitation is that it would be interesting to focus on the industrial structure rationalization, but the upgrading of the industrial structure shows the importance of the tertiary industry. However, the secondary industry is also indispensable. Therefore, how to realize the rational allocation of industrial structure is also crucial.
The original contributions presented in the study are included in the article/supplementary material, further inquiries can be directed to the corresponding author/s.
JD contributed to datacuration, methodology, and software. YD contributed to conceptualization and wrote the original draft. QJ contributed to conceptualization and wrote, reviewed, edited, and supervised. JZ contributed to conceptualization, methodology, validation, investigation, wrote, reviewed, and edited. All authors contributed to the article and approved the submitted version.
This article is supported by the National Social Science Foundation of China (Grant No. 18VDL017) and the Innovation Methods Special Foundation of the Chinese Ministry of Science and Technology (Grant No. 2018IMO40100). Certainly, all remaining errors are our own.
The authors declare that the research was conducted in the absence of any commercial or financial relationships that could be construed as a potential conflict of interest.
Ahmed, K., Rehman, M. U., and Ozturk, I. (2017). What drives carbon dioxide emissions in the long-run? Evidence from selected South Asian Countries. Renew. Sust. Energ. Rev. 70, 1142–1153. doi: 10.1016/j.rser.2016.12.018
Alkhathlan, K., and Javid, M. (2013). Energy consumption, carbon emissions and economic growth in Saudi Arabia: an aggregate and disaggregate analysis. Energ. Policy 62, 1525–1532. doi: 10.1016/j.enpol.2013.07.068
Bai, Y., Deng, X., Gibson, J., Zhao, Z., and Xu, H. (2019). How does urbanization affect residential CO2 emissions? An analysis on urban agglomerations of China. J. Clean. Prod. 209, 876–885. doi: 10.1016/j.jclepro.2018.10.248
Balado-Naves, R., Baños-Pino, J. F., and Mayor, M. (2018). Do countries influence neighbouring pollution? A spatial analysis of the EKC for CO2 emissions. Energ. Policy 123, 266–279. doi: 10.1016/j.enpol.2018.08.059
Breusch, T. S., and Pagan, A. R. (1980). The lagrange multiplier test and its applications to model specification in econometrics. Rev. Econ. Stud. 47, 239–253. doi: 10.2307/2297111
Chang, N. (2015). Changing industrial structure to reduce carbon dioxide emissions: a Chinese application. J. Clean. Prod. 103, 40–48. doi: 10.1016/j.jclepro.2014.03.003
Chen, S., Jefferson, G. H., and Zhang, J. (2011). Structural change, productivity growth and industrial transformation in China. China Econ. Rev. 22, 133–150. doi: 10.1016/j.chieco.2010.10.003
Cheng, C., Ren, X., Dong, K., Dong, X., and Wang, Z. (2020). How does technological innovation mitigate CO2 emissions in OECD countries? Heterogeneous analysis using panel quantile regression. J. Environ. Manag. 280:111818. doi: 10.1016/j.jenvman.2020.111818
Cheng, C., Ren, X., Wang, Z., and Yan, C. (2019). Heterogeneous impacts of renewable energy and environmental patents on CO2 emission-evidence from the BRIICS. Sci. Total Environ. 668, 1328–1338. doi: 10.1016/j.scitotenv.2019.02.063
Cheng, Z., Li, L., and Liu, J. (2018). Industrial structure, technical progress and carbon intensity in China’s provinces. Renew. Sust. Energ. Rev. 81, 2935–2946. doi: 10.1016/j.rser.2017.06.103
Dong, K., Dong, X., and Dong, C. (2019). Determinants of the global and regional CO2 emissions: what causes what and where? Appl. Econ. 51, 5031–5044. doi: 10.1080/00036846.2019.1606410
Dong, K., Dong, X., and Jiang, Q. (2020a). How renewable energy consumption lower global CO2 emissions? Evidence from countries with different income levels. World Econ. 43, 1665–1698. doi: 10.1111/twec.12898
Dong, K., Dong, X., and Ren, X. (2020b). Can expanding natural gas infrastructure mitigate CO2 emissions? Analysis of heterogeneous and mediation effects for China. Energ. Econ. 90:104830. doi: 10.1016/j.eneco.2020.104830
Dong, K., Sun, R., Jiang, H., and Zeng, X. (2018a). CO2 emissions, economic growth, and the environmental Kuznets curve in China: what roles can nuclear energy and renewable energy play? J. Clean Prod. 196, 51–63. doi: 10.1016/j.jclepro.2018.05.271
Dong, K., Sun, R., Li, H., and Liao, H. (2018b). Does natural gas consumption mitigate CO2 emissions: testing the environmental Kuznets curve hypothesis for 14 Asia-Pacific countries. Renew. Sust. Energ. Rev. 94, 419–429. doi: 10.1016/j.rser.2018.06.026
Dong, K., Dong, X., Jiang, Q., and Zhao, J. (2021). Valuing the greenhouse effect of political risks: the global case. Appl. Econ. 53, 1–15. doi: 10.1080/00036846.2021.1883543
Du, L., Wei, C., and Cai, S. (2012). Economic development and carbon dioxide emissions in China: provincial panel data analysis. China Econ. Rev. 23, 371–384. doi: 10.1016/j.chieco.2012.02.004
Dumitrescu, E. I., and Hurlin, C. (2012). Testing for Granger non-causality in heterogeneous panels. Econ. Model. 29, 1450–1460. doi: 10.1016/j.econmod.2012.02.014
Ertugrul, H. M., Cetin, M., Seker, F., and Dogan, E. (2016). The impact of trade openness on global carbon dioxide emissions: evidence from the top ten emitters among developing countries. Ecol. Indic. 67, 543–555. doi: 10.1016/j.ecolind.2016.03.027
Ghazali, A., and Ali, G. (2019). Investigation of key contributors of CO2 emissions in extended STIRPAT model for newly industrialized countries: a dynamic common correlated estimator (DCCE) approach. Energ. Rep. 5, 242–252. doi: 10.1016/j.egyr.2019.02.006
Gozgor, G. (2017). Does trade matter for carbon emissions in OECD countries? Evidence from a new trade openness measure. Environ. Sci. Pollut. R. 24, 27813–27821. doi: 10.1007/s11356-017-0361-z
Granger, C. W. J. (1969). Investigating causal relations by econometric models and cross-spectral methods. Econometrica 37, 424–438. doi: 10.2307/1912791
Grossman, G. M., and Krueger, A. B. (1993). “Environmental impacts of a North American free trade agreement,” in The US-Mexico free Trade Agreement, ed. P. Barber (Cambridge, MA: MIT Press), doi: 10.3386/w3914
Hausman, J. A. (1978). Specification tests in econometrics. Econometrica 46, 1251–1271. doi: 10.2307/1913827
Ho, S., and Iyke, B. (2019). Trade openness and carbon emissions: evidence from central and eastern European countries. Rev. Econ. 70, 41–67. doi: 10.1515/roe-2018-0001
Irandoust, M. (2016). The renewable energy-growth nexus with carbon emissions and technological innovation: evidence from the Nordic countries. Ecol. Indic. 69, 118–125. doi: 10.1016/j.ecolind.2016.03.051
Jiang, H., Dong, X., Jiang, Q., and Dong, K. (2020). What drives China’s natural gas consumption? Analysis of national and regional estimates. Energ. Econ. 87:104744. doi: 10.1016/j.eneco.2020.104744
Leal, P. H., and Marques, A. C. (2020). Rediscovering the EKC hypothesis for the 20 highest CO2 emitters among OECD countries by level of globalization. Int. Econ. 164, 36–47. doi: 10.1016/j.inteco.2020.07.001
Li, L., Lei, Y., Wu, S., He, C., Chen, J., and Yan, D. (2018). Impacts of city size change and industrial structure change on CO2 emissions in Chinese cities. J. Clean Prod. 195, 831–838. doi: 10.1016/j.jclepro.2018.05.208
Li, W., Wang, W., Wang, Y., and Qin, Y. (2017). Industrial structure, technological progress and CO2 emissions in China: analysis based on the STIRPAT framework. Nat. Hazards 88, 1545–1564. doi: 10.1007/s11069-017-2932-1
Liu, N., Ma, Z., and Kang, J. (2015). Changes in carbon intensity in China’s industrial sector: decomposition and attribution analysis. Energ. Policy 87, 28–38. doi: 10.1016/j.enpol.2015.08.035
Liu, X., and Bae, J. (2018). Urbanization and industrialization impact of CO2 emissions in China. J. Clean. Prod. 172, 178–186. doi: 10.1016/j.jclepro.2017.10.156
Lv, Z., and Xu, T. (2019). Trade openness, urbanization and CO2 emissions: dynamic panel data analysis of middle-income countries. J. Int. Trade. Econ. Dev. 28, 317–330. doi: 10.1080/09638199.2018.1534878
Menz, T., and Welsch, H. (2012). Population aging and carbon emissions in OECD countries: accounting for life-cycle and cohort effects. Energ. Econ. 34, 842–849. doi: 10.1016/j.eneco.2011.07.016
Peng, B., Tu, Y., Elahi, E., and Wei, G. (2018). Extended Producer Responsibility and corporate performance: effects of environmental regulation and environmental strategy. J. Environ. Manag. 218, 181–189. doi: 10.1016/j.jenvman.2018.04.068
Pesaran, M. H. (2004). General diagnostic tests for cross section dependence in panels. Cambridge Working Papers in Economics. Paper No. 0435. doi: 10.17863/CAM.5113
Pesaran, M. H. (2007). A simple panel unit root test in the presence of cross-section dependence. J. Appl. Econ. 22, 265–312. doi: 10.1002/jae.951
Ren, X., Cheng, C., Wang, Z., and Yan, C. (2021). Spillover and dynamic effects of energy transition and economic growth on carbon dioxide emissions for the European Union: a dynamic spatial panel model. Sustain. Dev. 29, 228–242. doi: 10.1002/sd.2144
Saboori, B., and Sulaiman, J. (2013). Environmental degradation, economic growth and energy consumption: evidence of the environmental Kuznets curve in Malaysia. Energ. Policy 60, 892–905. doi: 10.1016/j.enpol.2013.05.099
Tian, X., Bai, F., Jia, J., Liu, Y., and Shi, F. (2019). Realizing low-carbon development in a developing and industrializing region: impacts of industrial structure change on CO2 emissions in southwest China. J. Environ. Manag. 233, 728–738. doi: 10.1016/j.jenvman.2018.11.078
Wang, S., Wang, J., Li, S., Fang, C., and Feng, K. (2019). Socioeconomic driving forces and scenario simulation of CO2 emissions for a fast-developing region in China. J. Clean. Prod. 216, 217–229. doi: 10.1016/j.jclepro.2019.01.143
Wang, Y., Kang, Y., Wang, J., and Xu, L. (2017). Panel estimation for the impacts of population-related factors on CO2 emissions: a regional analysis in China. Ecol. Indic. 78, 322–330. doi: 10.1016/j.ecolind.2017.03.032
Wei, W., and Zhang, M. (2020). The non-linear impact of industrial structure on CO2 emissions in China. Appl. Econ. Lett. 27, 576–579. doi: 10.1080/13504851.2019.1644419
World Bank (2020). World Development Indicators. Available online at: https://databank.worldbank.org/source/world-development-indicators/preview/on
Yang, Y., Cai, W., and Wang, C. (2014). Industrial CO2 intensity, indigenous innovation and R&D spillovers in China’s provinces. Appl. Energy 131, 117–127. doi: 10.1016/j.apenergy.2014.06.033
Yii, K. J., and Geetha, C. (2017). The nexus between technology innovation and CO2 emissions in Malaysia: evidence from granger causality test. Energy Procedia 105, 3118–3124. doi: 10.1016/j.egypro.2017.03.654
York, R., Rosa, E. A., and Dietz, T. (2003). STIRPAT, IPAT and ImPACT: analytic tools for unpacking the driving forces of environmental impacts. Ecol. Econ. 46, 351–365. doi: 10.1016/S0921-8009(03)00188-5
Yu, Y., Deng, Y. R., and Chen, F. F. (2018). Impact of population aging and industrial structure on CO2 emissions and emissions trend prediction in China. Atmos. Pollut. Res. 9, 446–454. doi: 10.1016/j.apr.2017.11.008
Zhang, B., Wang, B., and Wang, Z. (2017). Role of renewable energy and non-renewable energy consumption on EKC: evidence from Pakistan. J. Clean Prod. 156, 855–864. doi: 10.1016/j.jclepro.2017.03.203
Zhang, F., Deng, X., Phillips, F., Fang, C., and Wang, C. (2020). Impacts of industrial structure and technical progress on carbon emission intensity: evidence from 281 cities in China. Technol. Forecast. Soc. Change 154:119949. doi: 10.1016/j.techfore.2020.119949
Zhang, X., and Ren, J. (2011). The relationship between carbon dioxide emissions and industrial structure adjustment for Shandong Province. Energy Proc. 5, 1121–1125. doi: 10.1016/j.egypro.2011.03.197
Zhao, J., Dong, C., Dong, X., and Jiang, Q. (2020a). Coordinated development of industrial structure and energy structure in China: its measurement and impact on CO2 emissions. Clim. Res. 81, 29–42. doi: 10.3354/cr01607
Zhao, J., Jiang, Q., Dong, X., and Dong, K. (2020b). Would environmental regulation improve the greenhouse gas benefits of natural gas use? A Chinese case study. Energ. Econ. 87:104712. doi: 10.1016/j.eneco.2020.104712
Zhao, J., Jiang, Q., Dong, X., and Dong, K. (2021a). Assessing energy poverty and its effect on CO2 emissions: the case of China. Energ. Econ. 97:105191. doi: 10.1016/j.eneco.2021.105191
Zhao, J., Shahbaz, M., Dong, X., and Dong, K. (2021b). How does financial risk affect global CO2 emissions? The role of technological innovation. Technol. Forecast. Soc. Change 168:120751. doi: 10.1016/j.techfore.2021.120751
Zhao, M., Tan, L., Zhang, W. G., Ji, M. H., Liu, Y., and Yu, L. Z. (2010). Decomposing the influencing factors of industrial carbon emissions in Shanghai using the LMDI method. Energy 35, 2505–2510. doi: 10.1016/j.energy.2010.02.049
Zhou, C., Wang, S., and Feng, K. (2018). Examining the socioeconomic determinants of CO2 emissions in China: a historical and prospective analysis. Resour. Conserv. Recy. 130, 1–11. doi: 10.1016/j.resconrec.2017.11.007
Keywords: industrial structure upgrading, greenhouse effect, RCEP and non-RCEP countries, mediating effect model, global analysis
Citation: Dong J, Dou Y, Jiang Q and Zhao J (2021) How Does Industrial Structure Upgrading Affect the Global Greenhouse Effect? Evidence From RCEP and Non-RCEP Countries. Front. Energy Res. 9:683166. doi: 10.3389/fenrg.2021.683166
Received: 20 March 2021; Accepted: 07 April 2021;
Published: 03 May 2021.
Edited by:
Xiaohang Ren, Central South University, ChinaReviewed by:
Jiang Hongdian, Beijing Institute of Technology, ChinaCopyright © 2021 Dong, Dou, Jiang and Zhao. This is an open-access article distributed under the terms of the Creative Commons Attribution License (CC BY). The use, distribution or reproduction in other forums is permitted, provided the original author(s) and the copyright owner(s) are credited and that the original publication in this journal is cited, in accordance with accepted academic practice. No use, distribution or reproduction is permitted which does not comply with these terms.
*Correspondence: Jun Zhao, emhhb2p1bjQ3NjhAMTYzLmNvbQ==
Disclaimer: All claims expressed in this article are solely those of the authors and do not necessarily represent those of their affiliated organizations, or those of the publisher, the editors and the reviewers. Any product that may be evaluated in this article or claim that may be made by its manufacturer is not guaranteed or endorsed by the publisher.
Research integrity at Frontiers
Learn more about the work of our research integrity team to safeguard the quality of each article we publish.