- College of Economics and Management, Northwest A&F University, Yangling, China
The impacts of widespread carbon emission trends possessed tremendous pressure for global food security, sustainable development, and ecosystems. Several temporal and spatial patterns of green technology have been adopted to reduce carbon emissions in different regions of China. In China, agriculture industries may have colossal importance for reducing carbon emissions. On the basis of the data from 1998 to 2018, the study uses the heterogeneous stochastic frontier model to quantify the carbon emission reduction potential of agricultural green technology progress in eastern, central, and western regions of China by using the heterogeneous stochastic frontier model. We also analyze the coefficient of variation and its spatial and temporal evolution pattern of carbon intensity decline potential index and explore the potential factors related to the agriculture green technology progress of China. The finding of the study revealed that the carbon emission rate in the agriculture industry of China is very high, whereas adopting green technology is slower because of economic and policy-related factors—the carbon emission of green technological progress. In terms of spatial variations, the changes in various regions were consistent with the overall fluctuating rate compared with the state of another country, but an increasing trend has been traced within the “east-central-west” regions. The overall regional differences are gradually trending, but differences between regions mainly cause them. The increase in the structure of the agricultural agriculture industry, the level of labor, and the increase in administrative environmental regulations will weaken the obstacles to the carbon emission reduction potential of green technological progress. The increase in urbanization, the level of the agricultural economy, and economic and environmental regulations will increase the carbon emission reduction potential of green technological progress. It is necessary to actively promote exchanges and cooperation in green agricultural technology and advanced management concepts, accelerate the optimization and upgrading of the industrial structure, and achieve the goal of peaking carbon emissions through regional coordinated development. Regionally, the overall external environment and the level of green technology progress in the western region need to be improved in all respects. The central and eastern regions need to focus on combining different policy tools to transform them from hindrance to promotion.
Introduction
In recent years, the widespread trends of greenhouse gas (GHG) emissions have led to some of the massive challenges such as global warming, climate change, and frequent natural disasters (Prastiyo et al., 2020). Moreover, the continuous trends of carbon emissions trigger uneven seasonal changes, monsoon, and soil and water pollutions (West and Marland, 2002; Zhao et al., 2018). The international bodies are also formulating various agreements, tactics, and symposiums to minimize the emission rate at a superior level. As a result, several nations have gradually realized the importance of a carbon emission reduction strategy and possessed a high potential for carbon reduction (Chen et al., 2020). Seemingly, by introducing the “13th Five-Year Plan,” the Chinese government adopted “persist in green development and focus on improving the ecological environment” and “three wastes green development method” by fostering the ecosystem in a structured and planned way. At the same time, China has a wrong impression as it holds top positions in its carbon emissions to support its staggering economic and production sectors (Dong et al., 2018; L. Zhang et al., 2019a). The Chinese government has currently taken some remarkable initiatives to uphold its stand to minimize its carbon footprint and reduce emissions to an optimum level. As China signed in the “Paris Agreement” 2016, it promised that CO2 emissions per unit of GDP in 2030 would be reduced by 60%–65% compared with that in 2005 (Amjath-Babu et al., 2019). At the general debate of the 75th UN General Assembly in September 2020, the ambitious goal of achieving carbon neutrality by 2060 was also put forward.
Agriculture is the foundation and an essential pillar of the stunning economic growth of China, whereas CO2 emissions from agricultural activities contribute to 50% of CH4, 70% of N2O, and 28.5% of CO2 (X. Zhang et al., 2019b). As a fundamental measure for reducing consumption and emission reduction, green-oriented technological progress is a key choice for fostering green agriculture development to actively respond toward carbon reduction schemes (Cordoba and Jansen, 2014; Rebolledo-Leiva et al., 2017; Yan et al., 2019). Improving resource utilization efficiency through green technology progress is an important means of agricultural low-carbon development. However, the research, development, and dissemination of green technologies usually do not proceed at the same speed in different regions (Du et al., 2019). Therefore, the impact of green technological progress may depend on the specific social or economic environment (Bonds and Downey, 2012). The study intends to assess human interactions, explore the potential carbon emission reduction of agricultural green technological progress, grasp the spatial relationship and evolution of the potential regional carbon emission reduction tactics, and address the urgency of sustainable development in different regions and group development stages. The differences in adaptability will guide each region to formulate targeted policies on the basis of its carbon emission reduction potential characteristics. This has important theoretical and practical significance for adhering to green development and fulfilling the established carbon emission reduction targets of China, thereby realizing the vision of “green water and green mountains are golden and silver mountains”.
Agricultural carbon emission reduction potential has been addressed frequently within national and international symposiums and academic discussions in recent years. The discussion mainly comprises the spatial mechanism of agricultural emission reduction potential, approaches, and policy formulation, and the relationship between emission reduction potential and technology has grasped focal points. Regarding the spatial relationship of agricultural emission reduction potential, central and western regions of China have shown greater adaption capabilities than the eastern region (Tang et al., 2016). However, the provincial spatial distribution has a certain degree of relevance and concentration (Wu et al., 2015). Regarding emission reduction approaches and policy formulation, Li et al. (2014) provided a brief assessment of the potential development of renewable energy, such as wind, water, and small hydropower in rural China. They concluded that the government should increase the investment to avail solar, small hydropower, and geothermal energy. Delin et al. (2013) employed the GTAP-E model to evaluate the significance of the reduction potential of agricultural GHG emissions and anticipated to improve carbon emission reduction through the taxation tactics of agricultural GHG emissions in China. Tang et al. (2019) conducted a comprehensive assessment for exploring the effectiveness of agricultural carbon emission reduction in 31 provinces (municipalities and autonomous regions) in China. They completed the reconstruction of the target and path optimization for quantifying emission reduction within “rural areas” on the basis of the relationship between emission reduction potential and technology. According to the relationship between carbon emission reduction potential and technology, Cole et al. (1997) have made a rough estimate of the global reduction potential of agricultural GHG emissions and studied the willingness to adopt emission reduction technologies from the perspectives of farmers. Seemingly, the study of Guo et al. (2018) clarified that the main source of agrarian GHG emissions is chemical fertilizers (77%–95%), and through the use of advanced farming techniques, GHG emissions can be reduced by about 13%–35%. Interestingly, the heterogeneity of the agricultural sector of China led to several technological gaps and formulated management errors, which are the main burden to facilitate effective emission reduction potential within the agricultural sector (Fei and Lin, 2017; Jiao et al., 2020).
At the same time, green technological progress refers to technological advancements that can promote energy conservation and emission reduction and are extended to the environment theories that favor low input productivity (Van Long and Stähler, 2018; S. Wang et al., 2018a; Woodhouse, 2009). The radical progress of innovative green technology has grown into an important means of reducing global CO2 emissions (Gras and Hernández, 2016; Khan et al., 2020; Nikzad and Sedigh, 2017). At present, the research on the relationship between green technological progress and carbon emissions mainly focuses on the relationship between green technology, green technology innovation, green technology vertical spillover, and environmentally biased technology progress and carbon emissions. According to Bai et al. (2020), green technology positively affected the carbon dioxide emissions of the entire country, especially the east and west parts. However, its leading role in promoting environmental protection has not yet been explored satisfactorily, and the scale effect is still prevailing. Du et al. (2019) explored that green technological innovation has no significant contribution to reducing CO2 emissions for economies with income levels below the threshold, whereas its mitigation effect is obvious for economies with income levels above the threshold. Jiao et al. (2020) found that the vertical green technology of China has a greater carbon emission reduction effect than green technology itself. Song et al. (2020) studied environmentally biased technological progress emission reductions. They found that the direct impact of technological advances in reducing emissions on CO2 emissions was significantly negative, whereas the direct impact of technological advances in reducing emissions was significantly greater than reducing energy-saving deviation technological progress. Whereas, the inductive technological progress not only can reduce the economic cost of carbon dioxide emission reduction but also can produce a “learning effect” (Gerlagh, 2007).
The existing research on green technological approaches mainly involves the industrial field, and there are limited researches that have been traced to quantify the progress of green agricultural technology. Green technological progress is essentially a concrete manifestation of technological progress, supporting environmental ecosystems by employing several technological innovations (Mariyono et al., 2010; Q. Wang et al., 2019a). The advancement of green technology requires clarification of green connotations and adherence to green goals in the whole process of the invention, innovation, and diffusion of green technologies to ensure the realization of green technological progress (Wang et al., 2016). In a broad sense, green technological progress compares the unrepresented economic units on the production frontier in different periods by considering undesired output. However, the economic growth factor caused by non-production factors is called TFP, or “Solo Residual Value,” which cannot be evaluated on the basis of assessing the factors. Green technological progress calculates the economic benefits of a single short-term technological progress, the adoption and innovative use of ordinary green technologies, and the embodiment of the accumulation of technological innovation (Asheim, 2000; Geels, 2005). Interestingly, the researches within a similar design used ordinary panel regression, Kuznets curve, and other methods to evaluate the carbon dioxide emissions of technological progress, but those possessed relatively vague results for an overall evaluation.
Moreover, several studies utilized index decomposition technology such as IPAT, STIRPAT, Kaya, and LMDI to decompose carbon dioxide emissions influencing factors (Braungardt et al., 2016; Wang et al., 2012; Weina et al., 2016) and used mathematical optimization methods to build system models (MARKAL-MACRO model, GTAP, CGE, Monte Carlo, LEAP, IESOCEM, and IPAC) and to carry out empirical studies (Tone and Tsutsui, 2010; Y.; Wang et al., 2019b). These studies may characterize the evolution of carbon emission reduction in a certain industry technology or estimate the carbon emission reduction potential of the entire ecosystem but cannot effectively evaluate green agricultural technologies of various provinces. The progress of carbon emission reduction potential cannot effectively give corresponding opinions on inter-provincial differences, and there are certain shortcomings. Therefore, it is necessary to improve the carbon emission reduction potential of agricultural green technological progress.
Furthermore, the progress of carbon emission reduction potential cannot effectively give corresponding opinions on inter-provincial differences (Vetőné Mózner, 2013; Cui et al., 2021), and there are certain shortcomings too. Therefore, it is necessary to improve agricultural green technological progress of carbon emission reduction potential (Smith et al., 2020). Frontier is a one-step model that incorporates influencing factors into the stochastic frontier. It can consider time effects and individual heterogeneity and reduce random errors in calculation. The heterogeneous stochastic frontier model can incorporate influencing factors and effectively evaluate the progress of the agricultural green technology carbon reduction effect. Therefore, establishing a potential carbon emission reduction model that includes the progress of green agricultural technology is the key issue of this paper.
The assessment of carbon emission reduction potentials of agricultural green technological progress indicates that indicators need more structured and well-balanced tactics. Therefore, in-depth assessment and observations should be employed to provide a profound measurement of agricultural green technological progress of carbon emission reduction potential. Within the study context, we have carefully crafted and intended to explore the three specific research questions: 1) Can the progress of green agricultural technology effectively reduce CO2 emissions? 2) If so, under the influence of other factors, is the impact of agricultural green technological progress on CO2 emissions consistent? and 3) How does the assessment quantify the heterogeneity of different provinces and regions? to explore and verify the changing trend of carbon emission reduction potential of the green technology progress of China since 1998–2018, to assess whether the elapsed time has improved, and to study the spatial difference of carbon emission reduction potential to grasp the spatial change dynamics of carbon emission reduction potential after green technology transfer and diffusion. The investigation of these problems will help put forward clear and specific suggestions for decision-makers, which is the motive of this study.
The possible contributions of this paper may include three aspects. First, the carbon emission reduction potential of the agricultural green technology progress index was evaluated, which made up for the shortage of research literature in this field. Second, taking the large-scale region as the research object, this paper measures the spatial and temporal evolution pattern and characteristics of the carbon emission reduction potential index of green technology progress. It broadens the field of vision that the existing research ignores the importance and non-stationarity of spatial location. Last, the influencing factors of carbon emission reduction by green technology are discussed, and the internal motivation of carbon emission reduction by agricultural green technology progress is revealed quantitatively so that policy-makers in different regions can adopt the carbon emission reduction strategy by green technology progress according to local conditions, which is of great significance for further promoting the agricultural green development policy of China.
For feeding the prime objectives and the research question, this article expanded in the following aspects: First, we have utilized EBM-GML to measure the level of agricultural green technological progress, carbon footprint model to measure the carbon emission level of the agriculture industry, and the heterogeneous stochastic frontier model to measure the green plant industry in China from 1998 to 2018. On the basis of the technological advancement in carbon emission reduction efficiency, we have constructed a carbon emission reduction potential index for eastern, central, and western regions of China. Then, with the help of the “Theil index and coefficient of variation,” we analyze its temporal and spatial evolution pattern, whereas economic development has possessed a certain practical guiding significance. Last, we discussed the influencing factors of the carbon emission reduction potential of green technology. The structure of this article is as follows: Methodology and Data introduces the research methods and data sources. The Temporal and Spatial Dynamic Distribution of the Reduction Potential is the temporal and spatial dynamic distribution of the reduction potential of agricultural green technological progress of the carbon emission model. Findings is the influencing factors of the reduction potential of agricultural green technological progress of the carbon emission model. The last section is the conclusion and recommendations.
Methodology and Data
Models
The Heterogeneous Stochastic Frontier Model
Stochastic frontier analysis was first proposed by Aigner (1977) and Meeusen and Broeck (1977) and then improved by Battese and Coelli (1995), and W. Wang et al. (2018b) improved its heterogeneity. The model formula is as follows:
where
where
Seemingly, the study constructed the optimal boundary for the decline of agricultural carbon emission reductions due to green technology advancement, but this optimal boundary is often not reached in reality due to random shocks. The feature has consistent with the assumption and construction process of the stochastic frontier model. Therefore, the article also used the stochastic frontier model estimation method as a subsequent estimation process.
The internal structure of agricultural development has greatly influenced agricultural economic development, labor level, urbanization level, administrative environmental regulations, and economic and environmental regulations that vary among various provinces. To reflect this heterogeneity constraint, referring to previous research results of Wang and Yu (2018), Eq. 4 has improved as follows:
where Xit=(1,Te,Di,Dt)′, β is the corresponding coefficient vector, and Di and Dt are dummy variables reflecting individual effects and time effects, respectively.
The mixed interference term in Eq. 4, Ɛit, consisted of two parts, vit represents the random interference term, and assuming that it obeys a normal distribution and was independent of each other, vit:i.i.d. N (0,
The heterogeneity constructed is also reflected in Eqs 5 and 6, where b0 and b1 represent constant terms. Both the actual decline value and the nominal optimal decline value of carbon emission intensity have been crafted on the basis of Eqs 2 and 3, which included the random interference items (v). However, random interference items are caused by some external factors that cannot be effectively controlled. Therefore, this paper eliminated the random interference term (v) and obtained the actual optimal decline boundary (X′itβ) of agricultural carbon emission intensity. The actual decrease value of the current agricultural carbon emission intensity after removing the random interference is X′itβ-uit. The actual decreased value of the agricultural carbon emission intensity was further compared with the actual optimal decrease value, and the potential for the decrease of the agricultural carbon emission intensity could be obtained (
The actual decline value of agricultural carbon emission intensity could not exceed the actual optimal decline value, so the potential decline value of agricultural carbon emission intensity (
Epsilon-Based Measure Global Malquist–Luenberger
Some scholars have recently tried to quantify green technology development (Kou et al., 2016; Cao and Wang, 2017). With some references (Tone and Tsutsui (2010); Song and Wang (2016)), the study constructs a hybrid distance function EBM model containing radial and non-radial features. The expression is as follows:
where X, Y, and Si- are input, output, weight coefficient, and input relaxation vector, respectively;
Then, with the study of Oh (2010), we use the GML index decomposition method (global Malquist–Luenberger) is introduced to obtain the green technological progress index. Its formula is as follows:
Data Sources
The article utilized the empirical data of small- and medium-sized agriculture industry, extracted from 31 provinces (municipalities, autonomous regions, excluding Hong Kong, Macao, and Taiwan) in China from 1998 to 2018. We have selected small- and medium-sized agriculture industry to capture the main proportion of the sectors. The data include several resources input, including employees and labor, chemical fertilizers, pesticides, agricultural film, fuel, the total power of agricultural machinery, and agriculture area. The output includes the expected output of the total output value and undesired output carbon emissions of the agriculture industry. The data used are from the “China Rural Statistical Yearbook,” “China Agricultural Statistical Yearbook,” and “China Fishery Statistical Yearbook.” In addition, the total power data of agriculture machinery is obtained by subtracting the total power of forestry, animal husbandry, and fishing machinery from the total power of farming, forestry, animal husbandry, and fishery machinery in the current year. The data of agriculture industry employees are calculated by multiplying the number of people employed in agriculture, forestry, animal husbandry, and fishery that year by the ratio of the total output value of the agriculture industry and the total output value of agriculture, forestry, animal husbandry, and fishery within those years. The descriptive statistical analysis results of specific indicators are shown in Table 1:
The Temporal and Spatial Dynamic Distribution of the Reduction Potential
The article measures the carbon emission reduction potential in agricultural green technological progress in 31 provinces in China under the internal structure of agriculture, agricultural economic development, labor level, urbanization level, administrative environmental regulations, and economic and environmental regulations. Its temporal and spatial dynamic distribution is as follows.
Chronological Characteristics of the Reduction Potential of Carbon Emission in Agricultural Green Technological Progress
It can be seen from Figure 1 that the reduction potential of carbon emission in the agricultural green technological progress of China showed a fluctuating downward trend from 1998 to 2018. The average carbon emission reduction potential was found at 0.115 in 1998, 0.066 in 2015, and, finally, 0.036 in 2018. It has reflected the remarkable effect of green technological progress on promoting carbon emission reduction of the agriculture industry of China year by year. From different periods, 1) the carbon emission reduction potential in the eastern region increased synchronously between the years 1998–1999 with an increase of 2.19%. In contrast, the carbon emission reduction potential of central and western regions declined rapidly. It could happen as the policy of these regions is largely. Different from the eastern regions, where the main goal is to increase grain production and improve efficiency, so less attention is paid to the environmental protection of agricultural resources and their capital investment. Simultaneously, the eastern region was affected by the severe flood in 1998, which was also a major reason for the differences. 2) From 1999 to 2007, the carbon emission reduction potential in eastern, central, and western regions showed a fluctuating upward trend, rising from 0.064 to 0.077, increasing 1.30%. Carbon emission reduction has increased significantly, affected by factors such as expanding the market scale and capital deepening exposure. Affected by the urgent need to enhance the market competitiveness of agricultural products after entry of China into World Trade Organization, high-yield, high-quality, high-efficient, and ecological and safe agriculture has become the core to support the green development agriculture. The government has issued the implementation opinions on comprehensively promoting the action plan of pollution-free food. Furthermore, necessary measures should be taken to restrict the use of chemical fertilizers, pesticides, and animal quarantine, which have clearly defined the specific objectives and implementation paths for the development of green agriculture (Li et al., 2020). These measures have significantly promoted the reduction potential of carbon emission in agricultural green technological progress. 3) From 2008 to 2018, the reduction potential of carbon emission in the three major regions of China declined slowly. Moreover, the average carbon emission reduction potential decreased from 0.065 to 0.037, with 2.86%. Affected by factors such as the expansion of the market scale and the exposure of capital deepening, the reduction potential of carbon emission has decreased significantly. However, in quantifying those situations, Chinese authorities realize some key reports and regulatory measures such as “Measures for the Administration of Green Food Labeling” and “Measures for the Administration of Quality and Safety Inspection of Agricultural Products.” It has curbed the decrease of the reduction potential of carbon emission in agricultural green technological progress.
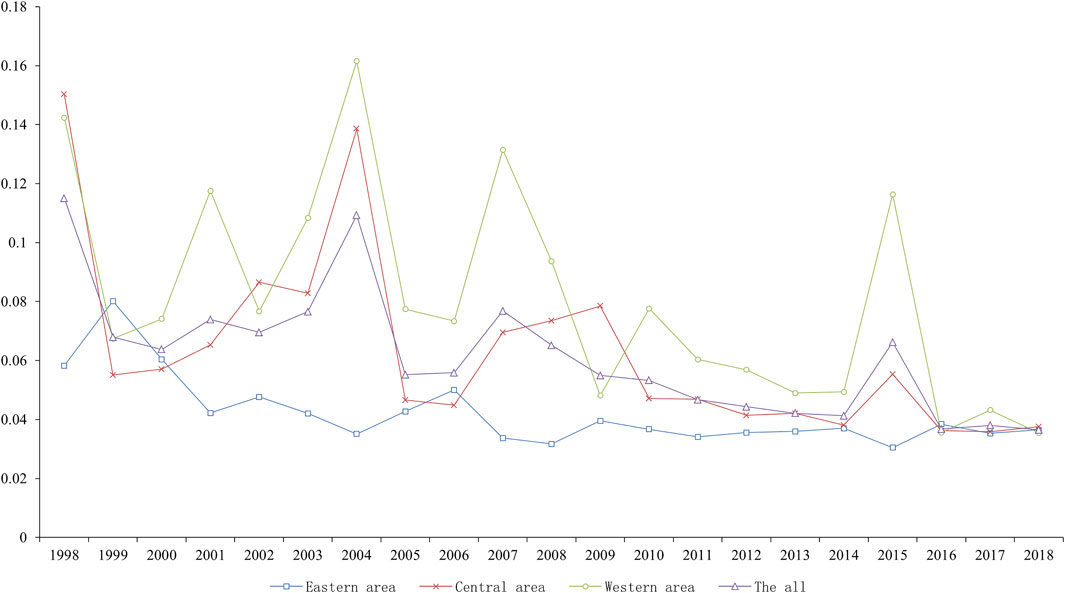
FIGURE 1. The trend carbon by region of emission reduction potential change in agricultural green technological progress (1998–2018).
Figure 2 shows the temporal evolution characteristics of carbon emission reduction potential of agricultural green technological progress in eastern, central, and western regions of China. The regional difference in carbon emission reduction potential of agricultural green technological progress in China from 1998 to 2018 has fluctuated consistently and showed a downward trend. Specifically, the variation coefficient in the eastern, central, and western regions decreased from 1.836 to 0.089 from 1998 to 2018. The fluctuation of eastern region is relatively small, whereas the fluctuation range in the western region is the largest. Nevertheless, the difference in carbon emission reduction potential among the three regions gradually narrows. Theil index decreased from 1.711 to 0.328, with a fluctuation range of 138.33% (Figure 3). Through the utilization of the “Theil index,” it is found that the evolution of inter-regional differences is highly consistent with the evolution of overall differences, and the inter-regional differences are far greater than the intra-regional differences. Their average contribution rate to the overall differences is as high as 56.19%. The intra-regional differences in the western region show an inverted U shape, whereas the central and eastern regions show a downward trend. The contribution rate of intra-regional differences to overall differences is only 43.81%, and the internal differences in the central region are the largest, followed by the western region and the smallest in the eastern region. Overall, the differences in the regional carbon emission reduction potential zones of China are shrinking. However, the regional differences in the eastern, central, and western regions are also shrinking. In particular, the contours and trends of the “Theil index” curve and coefficient of variation curve are inconsistent, which indicates that the imbalance of carbon emission reduction efficiency in the agricultural green technological progress of China is becoming more prominent.
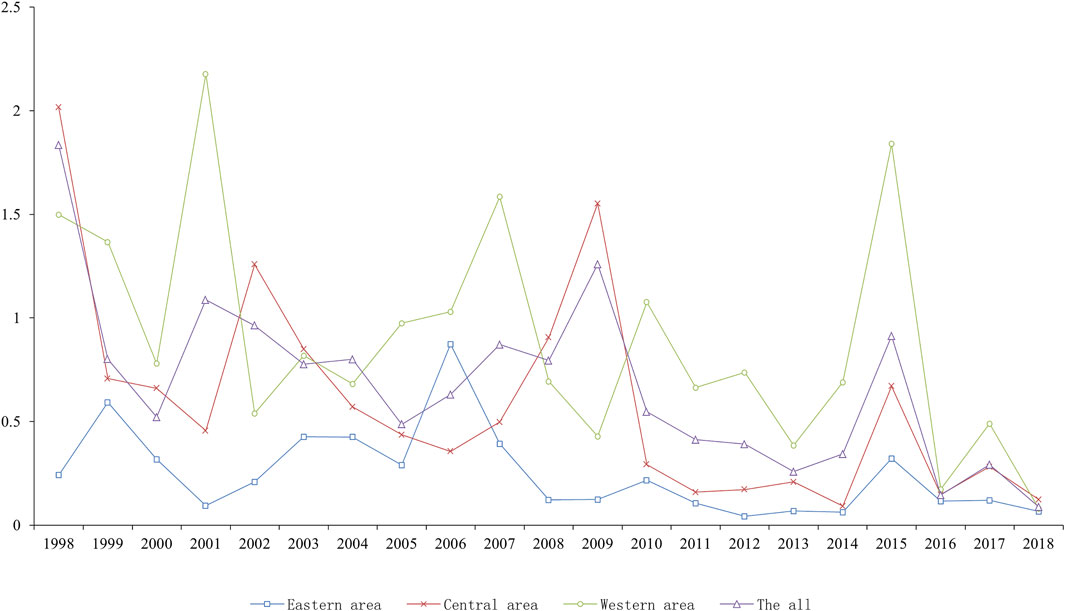
FIGURE 2. Coefficient of variation of carbon emission reduction potential of agricultural green technological progress from 1998 to 2018.
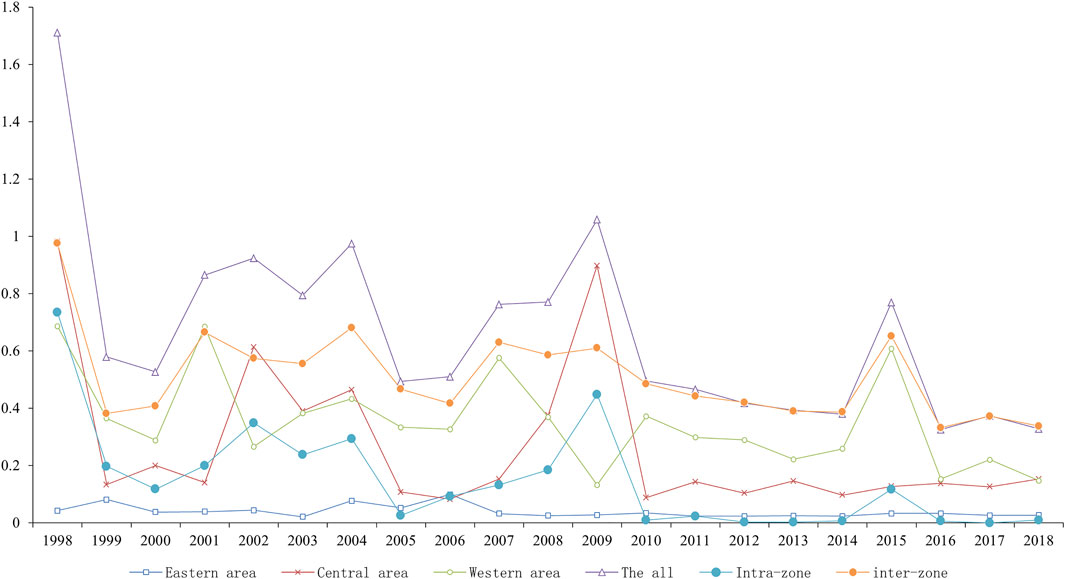
FIGURE 3. Agricultural Green technological progress Carbon Emission Reduction Potential Theil Index (1998–2018).
From the perspective of inter-provincial carbon emission reduction potential, the five provinces with the most tremendous carbon emission reduction potential in agricultural green technological progress from 1998 to 2018 are Shanxi, Guizhou, Ningxia, Qinghai, and Yunnan, which are mainly located in the western region. The five provinces with the lowest carbon emission reduction potential are Tianjin, Beijing, Shanghai, Liaoning, and Jiangsu, all located in the eastern region.
The Spatial Characteristics of the Reduction Potential of Carbon Emission of Green Technological Progress
To intuitively reflect the spatial distribution characteristics and evolution trend of carbon emission reduction potential of green technological progress, this paper divides 31 provinces (municipalities and autonomous regions) into four types according to carbon emission reduction potential: higher carbon emission reduction potential area, high carbon emission reduction potential area, lower carbon emission reduction potential area, and low carbon emission reduction potential area (Figure 4). 1) From 1998 to 2005, the reduction potential of carbon emission mainly shifted to a high level. The carbon emission reduction potential in the eastern region shifted from medium to high carbon emission reduction potential and medium to low carbon emission reduction potential areas. The higher carbon emission reduction potential area has decreased by 54.55%, and the lower carbon emission reduction potential area increased by 72.72%. The high carbon emission reduction potential area in central China decreased from 10% to 0%. The higher carbon emission reduction potential area increased by 20%, whereas the lower carbon emission reduction potential area increased by 20%.
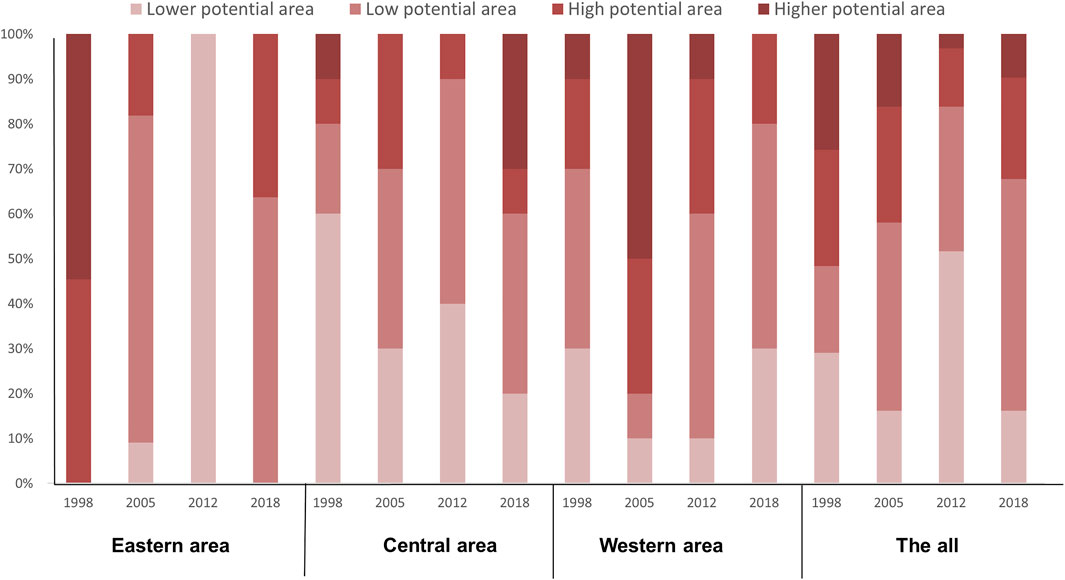
FIGURE 4. Changes in the types of carbon emission reduction potential under agricultural green progress.
The western region has shifted from medium and low carbon emission reduction potential to high carbon emission reduction potential areas. Moreover, the medium and high potential carbon emission reduction areas have increased by 10% and 40%, respectively. Except for the eastern region, the reduction potential of the carbon emissions from central and western regions has greatly increased. 2) From 2005 to 2012, the reduction potential of carbon emission in the western regions decreased significantly except eastern regions. The eastern region mainly changed from higher and lower carbon emission reduction potential area to low carbon emission reduction potential area, and the low carbon emission reduction potential area increased substantially. The central region has mainly shifted from a higher carbon emission reduction potential area to a medium and lower carbon emission reduction potential area, and the reduction potential of carbon emission has dropped significantly accounting for 40% and 50%, respectively. The western region mainly changed from a high carbon emission reduction potential area to a low carbon emission reduction potential area, accounting for 50%, indicating that the overall carbon emission reduction potential decreased significantly. 3) From 2012 to 2018, the reduction potential of carbon emission declined slightly. Among them, the reduction potential of carbon emission in the eastern region increased slightly. The central region is transforming into a high potential region. The western region mainly turns to low and low potential carbon emission reduction areas, with the proportion of low carbon emission reduction potential areas reaching 50% and low potential carbon emission reduction areas reaching 30%.
From the perspective of the spatial distribution of carbon emission reduction potential (Figure 5): 1) From 1998 to 2005, the spatial distribution of provinces of different grades experienced a distribution change from concentration to dispersion. In 1998, the lower potential carbon emission reduction areas were mainly distributed in the lower potential carbon emission reduction areas in the south, the lower potential carbon emission reduction areas in the north, and the upper potential areas in the northwest. In 2005, the spatial clustering degree of provinces of different grades was greatly reduced, and only the western region became high potential carbon emission reduction areas, whereas other regions were scattered. However, the balanced development trend in central and western regions is obvious, and the differentiation and evolution pattern of the regional potential of China “East-Middle-West” is gradually weakening. 2) From 2005 to 2012, the potential low carbon emission reduction areas expanded greatly, from dispersion to concentration, mainly concentrated in the eastern coast and North China and distributed in a chain from north to south.
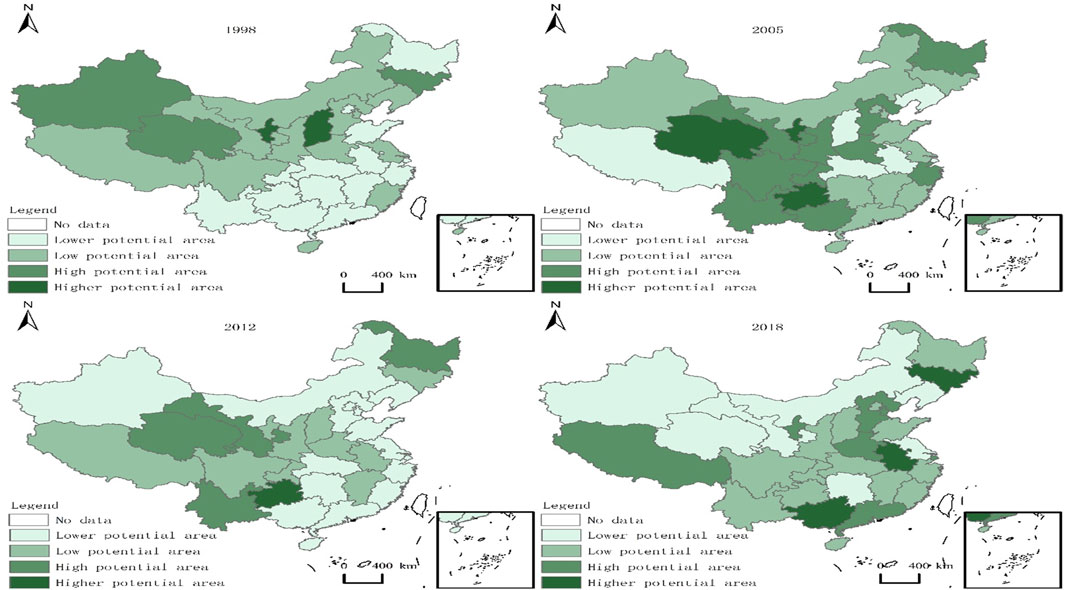
FIGURE 5. The spatial distribution of the reduction potential of carbon emission of the agricultural green technological progress of China.
The high carbon emission reduction potential area and potential higher carbon emission reduction areas decreased. However, the higher carbon emission reduction potential area has mainly concentrated in the central and western regions, and only Guizhou belongs to the high carbon emission reduction potential area. 3) From 2012 to 2018, the high potential carbon emission reduction areas increased, but the spatial distribution of different grades of provinces was relatively scattered. Compared with the previous period, it increased in the high potential carbon emission reduction areas. The possible explanation is that the reduction potential of carbon emission increased significantly during this period due to the expansion of the market scale and the exposure of the drawbacks of capital deepening.
Findings
Model Results
On the basis of the heterogeneous stochastic frontier model constructed by the existing literature (Such as Wanke et al., 2020; Zhang et al., 2020; Lin and Du, 2015), the study measures the carbon emission reduction potential of green technology advancement under various constraints. The results are shown in Table 2. Model 1) refers to Eq. 6 and does not impose any constraints. Model 2) shows that the internal structure of agriculture, the level of agricultural economic development, the level of labor, the level of urbanization, administrative environmental regulations, and economic and environmental regulations have a constant influence on the carbon emission reduction potential of green technological progress, that is, the variance is constant. Model 3) shows that the internal structure of agriculture, the level of agricultural economic development, the level of labor, the level of urbanization, administrative environmental regulations, and economic and environmental regulations have a constant influence on the carbon emission reduction potential of green technological progress. That is, the expected value of potential is constant. Model 4) indicates that the internal structure of agriculture, the level of agricultural economic development, the level of labor, the level of urbanization, administrative environmental regulations, and economic and environmental regulations have no impact on the carbon emission reduction potential of green technological progress. That is, the expected inefficiency is 0. Model 5) is a model that does not consider the impact of factors such as internal agricultural structure, agricultural economic development level, labor level, urbanization level, administrative-environmental regulation, and economic and environmental regulation on the carbon emission reduction potential green technological progress. At the same time, this article also conducts a regional model study on the basis of the three regions: east, central, and west. Among the five general model sets, the test results of LR1 and LR2 show that model 1) is better than the other four models, where LR1 mean does not have the null hypothesis of heterogeneous carbon emission reduction effect and inefficiency, whereas LR2 mean has heterogeneous carbon emission reduction effect inefficiency.
From the estimation results of model (1), it can be seen that the impact of agricultural green technological progress on carbon emission reduction potential is significantly positive. With the continuous improvement of the agricultural green technology level, the current agricultural emission intensity of each province is reduced compared with the previous period, and agricultural green technological progress contributes to carbon emission reduction. Besides, internal agricultural structure, agricultural economic development level, labor force level, urbanization level, administrative environmental regulation, and economic and environmental regulation will also affect the reduction potential of carbon emission of green technological progress. The increase of agricultural agriculture structure will reduce the hindrance of carbon emission reduction potential of green technological progress but increase its uncertainty. This finding is consistent with the research results of Ning et al. (2017) and Zhang and Wei (2014). The increase in urbanization will increase the hindrance of carbon emission reduction potential of green technological progress but reduce its uncertainty. The possible explanation is that the acceleration of urbanization forced agricultural production to transform into modern agriculture, which accelerated the pace of green technological progress and increased energy consumption, and failed to achieve carbon emission reduction. The increase of the labor force will reduce the hindrance and uncertainty of the carbon emission reduction potential of green technological progress and significantly enhance the carbon emission reduction potential (Song et al., 2015). Generally speaking, the increase of the labor force will accelerate the development of labor-intensive resources and form an extrusion effect on green technology progress. The increase of the labor force significantly enhances the reduction potential of carbon emission of green technological progress. The possible explanation is that the labor force will reduce the use of agricultural energy means of production such as machinery and increase renewable energy agricultural means of production such as biomass energy to reduce carbon emissions. Improving the agricultural economic level will significantly increase the hindrance of carbon emission reduction potential of green technological progress and reduce its uncertainty. The reason may be that the improvement of agricultural economic development level also promotes total energy consumption and contributes to the innovation, progress, dissemination, and application of green technology. However, it cannot meet the needs of economic development level, so it is not conducive to carbon emission reduction. The increase of administrative environmental regulations will reduce the uncertainty of carbon emission reduction potential of green technological progress and then increase carbon emissions, consistent with the research result of Yan et al. (2016) and Cheng et al. (2019). The economic intervention of the government may offset the positive impact of green technological progress on the low carbonization of the industrial structure. The increase of economic and environmental regulation will also increase the uncertainty of the carbon emission reduction potential of green technological progress. The influence of economic and environmental regulation on the progress of green technology changes with the change of green regulation scale could happen. Green regulation helps to promote the progress of pollution treatment technology in low level, but when the level of green regulation reaches a certain level, further regulation is not conducive to maintaining green technology at a higher level.
In the sub-regional model, the significance of each factor on the reduction potential of carbon emission of agricultural green technological progress is lower than that of the general model, but it is consistent with the whole. The influencing factors in the eastern region are not significant at all. The possible explanation is that the agricultural green technological progress in the eastern region is at a high level, whereas the internal agricultural structure, agricultural economic development level, labor force level, urbanization level, administrative-environmental regulation, and economic and environmental regulation are also at a high level, which is basically in a saturated state. Furthermore, fostering the improvement of technology has no obvious effect on carbon emission reduction. The level of the labor force in western China is inconsistent with the general model results 1). The increase in the labor force will significantly increase the carbon emission reduction potential of green technological progress in western China. The possible reason is that the low quality of the labor force in western China burdens agricultural green technological progress and is not conducive to agricultural carbon emission reduction. The economic and environmental regulation in western China is inconsistent with the general model results 1), and the increase of the labor force will significantly reduce the reduction potential of carbon emission of green technological progress in western China. The possible explanation is that the scale of green control in the western region is early, and green control helps promote the technological progress of pollution treatment. Nationally, when the level of green regulation reaches a certain level, further regulation is not conducive to maintaining a high level of green technology as far there is any inconsistency between them.
Conclusions and Recommendations
This paper used the heterogeneous stochastic frontier method to measure the reduction potential of the carbon emission index of agricultural green technological progress in 31 provinces (municipalities and autonomous regions) in China. Moreover, temporal and spatial evolution pattern was also analyzed with the help of “Theil index” and “coefficient of variation.” Last, the crucial influencing factors of green technological progress of the carbon emission reduction potential were firmly analyzed. The key findings are as follows:
1) From a perspective of the time-space differentiation of carbon emission reduction potential of the agricultural green technological progress of China, the agricultural green technological progress of the overall carbon emission reduction potential shows a downward trend in the time change. However, because of the influence of economic and policy factors, the reduction potential of carbon emission of green technological progress appears an inflection point in some years. It makes the change curve of carbon emission reduction potential of green technological progress slightly fluctuating. In terms of spatial changes, the regional changes are consistent with the fluctuation state of carbon emission reduction potential of the overall green technological progress of China, which is roughly arranged according to the “east-central-west” gradient from low to high. However, the overall regional differences show an expanding trend, which is mainly caused by regional differences.
2) Among the influencing factors of carbon emission reduction in the agricultural green technological progress of China, the increase of agricultural agriculture structure, labor force level, and administrative environmental regulation would weaken the hindrance of carbon emission potential in green technological progress. The increase in urbanization, agricultural, economic, and environmental regulation will hinder the carbon emission reduction potential of green technological progress.
By analyzing the distribution pattern of carbon emission reduction potential of green technological progress, this paper enriches the research content of carbon emission reduction of agricultural green technological progress. In practice, it warns against the new differences caused by the reduction potential of carbon emission of green technological progress. To avoid the problems caused by the further expansion of regional differences in carbon emission reduction potential of green technological progress, to improve the carbon emission reduction efficiency of the agricultural green technological progress of China, and to achieve a green economy that requires both economic growth and environmental improvement, it is necessary to set different carbon emissions reduction efficiency and some specific measures to evaluate the improvement targets within different provinces.
The carbon emission reduction potential of green technological progress actively promotes the exchange and cooperation in agricultural green technology research and development and advanced management concepts, ensures the effective promotion and diffusion of advanced technologies and management methods, and thus realizes the general improvement of agricultural carbon emission efficiency. For areas with low carbon emission reduction potential, the optimization and upgrading of the internal structure of agriculture should be accelerated, the cooperation between regions and provinces in emission reduction should be strengthened, a regional emission reduction responsibility-sharing mechanism and emission reduction compensation system, as soon as possible, should be established, and the goal of peaking carbon emissions through regionally coordinated emission reduction should be achieved. It is necessary to focus on the environmental quality of green technological progress and carbon emission reduction at the national level, mainly by clarifying the position of the government and the market in promoting green technological progress. To narrow regional differences, we should aim at the inherent needs of green agriculture development in various regions.
Although the development strategy has been implemented in the western region for many years, some progress has been made in the external environment such as internal agricultural structure, agricultural economic development level, labor force level, urbanization rate, administrative environmental regulation, and economic and environmental regulation. However, because of the poor foundation in the early stage, the overall situation is still not ideal, and the progress level of green technology is found relatively low. Therefore, the overall external environment and the level of green technological progress in the western region need to be improved in a specific manner that can foster the adoption of technological advancement. Moreover, it can maintain the strong momentum of improving the reduction potential of carbon emission of green technological progress, reduce the excessive dependence on energy elements, and alleviate the mismatch of elements, which is the top priority of future work. The central and eastern regions need to adjust their administrative environmental, and economic and environmental regulatory policies. They change from the original restraining environment to promoting the environment as an essential starting point to boost the carbon emission reduction potential of green technological progress. In particular, those regions should take necessary initiatives aiming to eliminate insufficient or obstructive policies. Furthermore, the government should pay more attention to matching and combining different policy tools and changing them from obstruction to promotion.
In addition, the carbon emissions of agricultural economic activities in the context of the COVID-19 pandemic will also have a significant impact. Under COVID-19, there is an urgent need to restore the affected livelihood activities of farmers, which requires the role of technology. The article concludes that technological progress in different regions is inconsistent with carbon emission reduction potential. Government should avoid weakening of environmental policies to reduce policy uncertainty for agri-businesses, to achieve co-benefits and to reduce political economy barriers. The supports should be extended to help farms manage liquidity problems across sectors, including renewable energy and other low-carbon technology sectors. Therefore, in terms of labor, we need to increase the opportunities for farmers to obtain training in green agricultural technology. The government should coordinate access to mechanized services and promote the use of shared machinery. China will further promote the rate of urbanization and increase the efficiency of agricultural production. Increase economic government financial subsidies to promote the progress of green technology.
Data Availability Statement
The raw data supporting the conclusion of this article will be made available by the authors, without undue reservation.
Ethics Statement
Ethical review and approval was not required for the study on human participants in accordance with the local legislation and institutional requirements. The patients/participants provided their written informed consent to participate in this study.
Author Contributions
DY and AS conceived and designed research. LQ and ZM approved the questionnaire and the final project. DY conducted experiments and collected and analyzed data. AS structured and convert the data for analysis. AS and DY wrote the manuscript. LQ and ZM modified the article and provide funding support. AS redesign the manuscript according to the requirements of the journal and provide a critical review for this study. All authors read and approved the manuscript.
Funding
The study was funded by the following: (i) National Natural Science Foundation of China (No. 72173097). (ii) National Soft Science Project of State Forestry and Grassland Administration (No. 2019131039). (iii) Key project of six industrial research institutes of Northwest Agricultural and Forestry University (No. Z221021601). (iv) Key special funds of Ministry of agriculture and Ministry of Finance (No. CARS-07-F-1). (v) Major Research Project of County Economy in Shaanxi Province (No. 2019XY012). (vi) Scientific Research and Innovation Projects of Northwest Agricultural and Forestry University (No. JGYJSCXXM202001).
Conflict of Interest
The authors declare that the research was conducted in the absence of any commercial or financial relationships that could be construed as a potential conflict of interest.
Publisher’s Note
All claims expressed in this article are solely those of the authors and do not necessarily represent those of their affiliated organizations or those of the publisher, the editors, and the reviewers. Any product that may be evaluated in this article, or claim that may be made by its manufacturer, is not guaranteed or endorsed by the publisher.
Acknowledgments
We acknowledge the profound observations and supports of the reviewers.
References
Amjath-Babu, T. S., Aggarwal, P. K., and Vermeulen, S. (2019). Climate Action for Food Security in South Asia? Analyzing the Role of Agriculture in Nationally Determined Contributions to the Paris Agreement. Clim. Pol. 19, 283–298. doi:10.1080/14693062.2018.1501329
Asheim, G. B. (2000). Green National Accounting: Why and How. Envir. Dev. Econ. 5, 25–48. doi:10.1017/s1355770x00000036
Bai, C., Feng, C., Yan, H., Yi, X., Chen, Z., and Wei, W. (2020). Will Income Inequality Influence the Abatement Effect of Renewable Energy Technological Innovation on Carbon Dioxide Emissions. J. Environ. Manage. 264, 110482. doi:10.1016/j.jenvman.2020.110482
Bonds, E., and Downey, L. (2012). Green" Technology and Ecologically Unequal Exchange: The Environmental and Social Consequences of Ecological Modernization in the World-System. JWSR 2 (18), 167–186. doi:10.5195/jwsr.2012.482
Braungardt, S., Elsland, R., and Eichhammer, W. (2016). The Environmental Impact of Eco-Innovations: the Case of EU Residential Electricity Use. Environ. Econ. Pol. Stud. 18, 213–228. doi:10.1007/s10018-015-0129-y
Cao, B., and Wang, S. (2017). Opening up, International Trade, and green Technology Progress. J. Clean. Prod. 142, 1002–1012. doi:10.1016/j.jclepro.2016.08.145
Chen, X., Shuai, C., Wu, Y., and Zhang, Y. (2020). Analysis on the Carbon Emission Peaks of China's Industrial, Building, Transport, and Agricultural Sectors. Sci. Total Environ. 709, 135768. doi:10.1016/j.scitotenv.2019.135768
Cheng, J., Yi, J., Dai, S., and Xiong, Y. (2019). Can Low-Carbon City Construction Facilitate green Growth? Evidence from China's Pilot Low-Carbon City Initiative. J. Clean. Prod. 231, 1158–1170. doi:10.1016/j.jclepro.2019.05.327
Cole, C. V., Duxbury, J., Freney, J., Heinemeyer, O., Minami, K., Mosier, A., et al. (1997). Global Estimates of Potential Mitigation of Greenhouse Gas Emissions by Agriculture. Nutrient Cycling in Agroecosystems 49, 221–228. doi:10.1023/A:1009731711346
Cordoba, D., and Jansen, K. (2014). The Return of the State: Neocollectivism, Agrarian Politics and Images of Technological Progress in the MAS Era in Bolivia. J. Agrarian Change 14, 480–500. doi:10.1111/joac.12036
Cui, Y., Khan, S. U., Deng, Y., Zhao, M., and Hou, M. (2021). Environmental Improvement Value of Agricultural Carbon Reduction and its Spatiotemporal Dynamic Evolution: Evidence from China. Sci. Total Environ. 754, 142170. doi:10.1016/j.scitotenv.2020.142170
Delin, H., Songfeng, C., and Zhen, W. (2013). Reduction Potential and Control Policy of Agricultural Nitrous Oxide Greenhouse Gas Emissions in China. Environ. Econ. 4 (1), 15–29.
Dong, F., Yu, B., Hadachin, T., Dai, Y., Wang, Y., Zhang, S., et al. (2018). Drivers of Carbon Emission Intensity Change in China. Resour. Conservation Recycling 129, 187–201. doi:10.1016/j.resconrec.2017.10.035
Du, K., Li, P., and Yan, Z. (2019). Do green Technology Innovations Contribute to Carbon Dioxide Emission Reduction? Empirical Evidence from Patent Data. Technol. Forecast. Soc. Change 146, 297–303. doi:10.1016/j.techfore.2019.06.010
Fei, R., and Lin, B. (2017). Technology gap and CO 2 Emission Reduction Potential by Technical Efficiency Measures: A Meta-Frontier Modeling for the Chinese Agricultural Sector. Ecol. Indicators 73, 653–661. doi:10.1016/j.ecolind.2016.10.021
Geels, F. W. (2005). Technological Transitions and System Innovations: A Co-evolutionary and Socio-Technical Analysis. Cheltenham, United Kingdom: Edward Elgar Publishing.
Gerlagh, R. (2007). Measuring the Value of Induced Technological Change. Energy Policy 35, 5287–5297. doi:10.1016/j.enpol.2006.01.034
Gras, C., and Hernández, V. (2016). Hegemony, Technological Innovation and Corporate Identities: 50 Years of Agricultural Revolutions in Argentina. J. Agrarian Change 16, 675–683. doi:10.1111/joac.12162
Guo, C., Wang, X., Li, Y., He, X., Zhang, W., Wang, J., et al. (2018). Carbon Footprint Analyses and Potential Carbon Emission Reduction in China's Major Peach Orchards. Sustainability 10, 2908. doi:10.3390/su10082908
Jiao, J., Chen, C., and Bai, Y. (2020). Is green Technology Vertical Spillovers More Significant in Mitigating Carbon Intensity? Evidence from Chinese Industries. J. Clean. Prod. 257, 120354. doi:10.1016/j.jclepro.2020.120354
Khan, A., Muhammad, F., Chenggang, Y., Hussain, J., Bano, S., and Khan, M. A. (2020). The Impression of Technological Innovations and Natural Resources in Energy-Growth-Environment Nexus: A New Look into BRICS Economies. Sci. Total Environ. 727, 138265. doi:10.1016/j.scitotenv.2020.138265
Kou, M., Chen, K., Wang, S., and Shao, Y. (2016). Measuring Efficiencies of Multi-Period and Multi-Division Systems Associated with DEA: An Application to OECD Countries' National Innovation Systems. Expert Syst. Appl. 46, 494–510. doi:10.1016/j.eswa.2015.10.032
Li, C., He, L., Cao, Y., Xiao, G., Zhang, W., Liu, X., et al. (2014). Carbon Emission Reduction Potential of Rural Energy in China. Renew. Sust. Energ. Rev. 29, 254–262. doi:10.1016/j.rser.2013.08.073
Li, Y., Li, Z., Chang, S. X., Cui, S., Jagadamma, S., Zhang, Q., et al. (2020). Residue Retention Promotes Soil Carbon Accumulation in Minimum Tillage Systems: Implications for Conservation Agriculture. Sci. Total Environ. 740, 140147. doi:10.1016/j.scitotenv.2020.140147
Lin, B., and Du, K. (2015). Modeling the Dynamics of Carbon Emission Performance in China: A Parametric Malmquist index Approach. Energ. Econ. 49, 550–557. doi:10.1016/j.eneco.2015.03.028
Mariyono, J., Kompas, T., and Grafton, R. Q. (2010). Shifting from Green Revolution to Environmentally Sound Policies: Technological Change in Indonesian rice Agriculture. J. Asia Pac. Economy 15, 128–147. doi:10.1080/13547861003700109
Nikzad, R., and Sedigh, G. (2017). Greenhouse Gas Emissions and green Technologies in Canada. Environ. Dev. 24, 99–108. doi:10.1016/j.envdev.2017.01.001
Ning, L. I., He, W., Qiu, T., and Chen, L. (2017). Farmland Property Rights Structure, Efficiency of Factors of Production, and Agricultural Performance. Manage. World 3, 44–62.
Oh, D.-h. (2010). A Global Malmquist-Luenberger Productivity index. J. Prod. Anal. 34, 183–197. doi:10.1007/s11123-010-0178-y
Prastiyo, S. E., Irham, M., Hardyastuti, S., and Jamhari, A. H. (2020). How Agriculture, Manufacture, and Urbanization Induced Carbon Emission? the Case of Indonesia. Environ. Sci. Pollut. Res. 27, 42092–42103. doi:10.1007/s11356-020-10148-w
Rebolledo-Leiva, R., Angulo-Meza, L., Iriarte, A., and González-Araya, M. C. (2017). Joint Carbon Footprint Assessment and Data Envelopment Analysis for the Reduction of Greenhouse Gas Emissions in Agriculture Production. Sci. Total Environ. 593-594, 36–46. doi:10.1016/j.scitotenv.2017.03.147
Smith, P., Soussana, J. F., Angers, D., Schipper, L., Chenu, C., Rasse, D. P., et al. (2020). How to Measure, Report and Verify Soil Carbon Change to Realize the Potential of Soil Carbon Sequestration for Atmospheric Greenhouse Gas Removal. Glob. Change Biol. 26, 219–241. doi:10.1111/gcb.14815
Song, M., Cen, L., Zheng, Z., Fisher, R., Liang, X., Wang, Y., et al. (2015). Volume on Improving Natural Resource Management and Human Health to Ensure Sustainable Societal Development Based upon Insights Gained from Working within ‘Big Data Environments. J. Clean. Prod. 94, 1–4. doi:10.1016/j.jclepro.2015.02.010
Song, M., and Wang, S. (2016). Can Employment Structure Promote Environment-Biased Technical Progress. Technol. Forecast. Soc. Change 112, 285–292. doi:10.1016/j.techfore.2016.02.016
Song, M., Xie, Q., Wang, S., and Zhou, L. (2021). Intensity of Environmental Regulation and Environmentally Biased Technology in the Employment Market. Omega 100, 102201. doi:10.1016/j.omega.2020.102201
Tang, K., Gong, C., and Wang, D. (2016). Reduction Potential, Shadow Prices, and Pollution Costs of Agricultural Pollutants in China. Sci. Total Environ. 541, 42–50. doi:10.1016/j.scitotenv.2015.09.013
Tang, K., He, C., Ma, C., and Wang, D. (2019). Does Carbon Farming Provide a Cost‐effective Option to Mitigate GHG Emissions? Evidence from China. Aust. J. Agric. Resour. Econ. 63, 575–592. doi:10.1111/1467-8489.12306
Tone, K., and Tsutsui, M. (2010). An Epsilon-Based Measure of Efficiency in DEA - A Third Pole of Technical Efficiency. Eur. J. Oper. Res. 207, 1554–1563. doi:10.1016/j.ejor.2010.07.014
Van Long, N., and Stähler, F. (2018). General Equilibrium Effects of Green Technological Progress. Environ. Resource Econ. 69, 159–166. doi:10.1007/s10640-016-0069-4
Vetőné Mózner, Z. (2013). A Consumption-Based Approach to Carbon Emission Accounting - Sectoral Differences and Environmental Benefits. J. Clean. Prod. 42, 83–95. doi:10.1016/j.jclepro.2012.10.014
Wang, Q., Qu, J., Wang, B., Wang, P., and Yang, T. (2019a). Green Technology Innovation Development in China I 1990-2015. Sci. Total Environ. 696, 134008. doi:10.1016/j.scitotenv.2019.134008
Wang, S., Chen, M., and Song, M. (2018a). Energy Constraints, green Technological Progress and Business Profit Ratios: Evidence from Big Data of Chinese Enterprises. Int. J. Prod. Res. 56, 2963–2974. doi:10.1080/00207543.2018.1454613
Wang, S., Fang, C., and Wang, Y. (2016). Spatiotemporal Variations of Energy-Related CO 2 Emissions in China and its Influencing Factors: An Empirical Analysis Based on Provincial Panel Data. Renew. Sust. Energ. Rev. 55, 505–515. doi:10.1016/j.rser.2015.10.140
Wang, W. X., and Yu, B. (2018). Measurement of the Reduction Potential of Industrial Air Pollutants Intensity Based on Environmental Learning Curves. Econ. Probl., 68–76. doi:10.16011/j.cnki.jjwt.2018.12.011
Wang, W., Yu, B., Yao, X., Niu, T., and Zhang, C. (2018b). Can Technological Learning Significantly Reduce Industrial Air Pollutants Intensity in China?-Based on a Multi-Factor Environmental Learning Curve. J. Clean. Prod. 185, 137–147. doi:10.1016/j.jclepro.2018.03.028
Wang, Y., Zhao, T., Wang, J., Guo, F., Kan, X., and Yuan, R. (2019b). Spatial Analysis on Carbon Emission Abatement Capacity at Provincial Level in China from 1997 to 2014: An Empirical Study Based on SDM Model. Atmos. Pollut. Res. 10, 97–104. doi:10.1016/j.apr.2018.06.003
Wang, Z., Yang, Z., Zhang, Y., and Yin, J. (2012). Energy Technology patents-CO2 Emissions Nexus: An Empirical Analysis from China. Energy Policy 42, 248–260. doi:10.1016/j.enpol.2011.11.082
Wanke, P., Chen, Z., Zheng, X., and Antunes, J. (2020). Sustainability Efficiency and Carbon Inequality of the Chinese Transportation System: A Robust Bayesian Stochastic Frontier Analysis. J. Environ. Manage. 260, 110163. doi:10.1016/j.jenvman.2020.110163
Weina, D., Gilli, M., Mazzanti, M., and Nicolli, F. (2016). Green Inventions and Greenhouse Gas Emission Dynamics: a Close Examination of Provincial Italian Data. Environ. Econ. Pol. Stud. 18, 247–263. doi:10.1007/S10018-015-0126-1
West, T. O., and Marland, G. (2002). A Synthesis of Carbon Sequestration, Carbon Emissions, and Net Carbon Flux in Agriculture: Comparing Tillage Practices in the United States. Agric. Ecosyst. Environ. 91, 217–232. doi:10.1016/S0167-8809(01)00233-X
Woodhouse, P. (2009). Technology, Environment and the Productivity Problem in African Agriculture: Comment on theWorld Development Report 2008. J. Agrarian Change 9, 263–276. doi:10.1111/j.1471-0366.2009.00205.x
Wu, X. R., Zhang, J. B., Cheng, L. L., and Tian, Y. (2015). Potential of Agricultural Carbon Reduction under Climate Change and its Spatial Correlation Characteristics in China: Based on the Spatial Durbin Model. China Popul. Resour. Environ. 25, 53–61.
Yan, G. Q., He, Y. C., and Zhang, X. H. (2019). Green Technology Progress, Agricultural Economic Growth and Pollution Space Spillover Effect: Evidence Og Agricultural Water Utilization Progress in China. Resour. Environ. Yangtze Basin 28, 2921–2935.
Yan, Z., Deng, X., and Chen, B. (2016). The Impact of Green Technology Progress on the Low Carbon Transformation of China’s Industrial Structure. Comp. Econ. Soc. Syst. 4, 25–39.
Zhang, H., Fan, L. W., and Zhou, P. (2020). Handling Heterogeneity in Frontier Modeling of City-Level Energy Efficiency: The Case of China. Appl. Energ. 279, 115846. doi:10.1016/j.apenergy.2020.115846
Zhang, H., and Wei, X. P. (2014). Green Paradox or Forced Emission-Reduction: Dual Effect of Environmental Regulation on Carbon Emissions. China Popul. Resour. Environ. 24, 21–29.
Zhang, L., Pang, J., Chen, X., and Lu, Z. (2019a). Carbon Emissions, Energy Consumption and Economic Growth: Evidence from the Agricultural Sector of China's Main Grain-Producing Areas. Sci. Total Environ. 665, 1017–1025. doi:10.1016/j.scitotenv.2019.02.162
Zhang, X., Meng, F., Li, H., Wang, L., Wu, S., Xiao, G., et al. (2019b). Optimized Fertigation Maintains High Yield and Mitigates N2O and NO Emissions in an Intensified Wheat-maize Cropping System. Agric. Water Manage. 211, 26–36. doi:10.1016/j.agwat.2018.09.045
Keywords: green technological progress, carbon emission reduction potential, temporal and spatial evolution, influence factor, carbon reduction
Citation: Yue D, Sarkar A, Yu C, Qian L and Minjuan Z (2021) The Evolution of Factors Influencing Green Technological Progress in Terms of Carbon Reduction: A Spatial-Temporal Tactic Within Agriculture Industries of China. Front. Energy Res. 9:661719. doi: 10.3389/fenrg.2021.661719
Received: 01 February 2021; Accepted: 02 November 2021;
Published: 25 November 2021.
Edited by:
Joni Jupesta, Research Institute of Innovative Technology for the Earth, JapanReviewed by:
Ehsan Rasoulinezhad, University of Tehran, IranMike Tsionas, Lancaster University, United Kingdom
Copyright © 2021 Yue, Sarkar, Yu, Qian and Minjuan. This is an open-access article distributed under the terms of the Creative Commons Attribution License (CC BY). The use, distribution or reproduction in other forums is permitted, provided the original author(s) and the copyright owner(s) are credited and that the original publication in this journal is cited, in accordance with accepted academic practice. No use, distribution or reproduction is permitted which does not comply with these terms.
*Correspondence: Lu Qian, bHVxaWFuQG53YWZ1LmVkdS5jbg==; Zhao Minjuan, bWluanVhbi56aGFvQG53YWZ1LmVkdS5jbg==
†These authors have contributed equally to this work