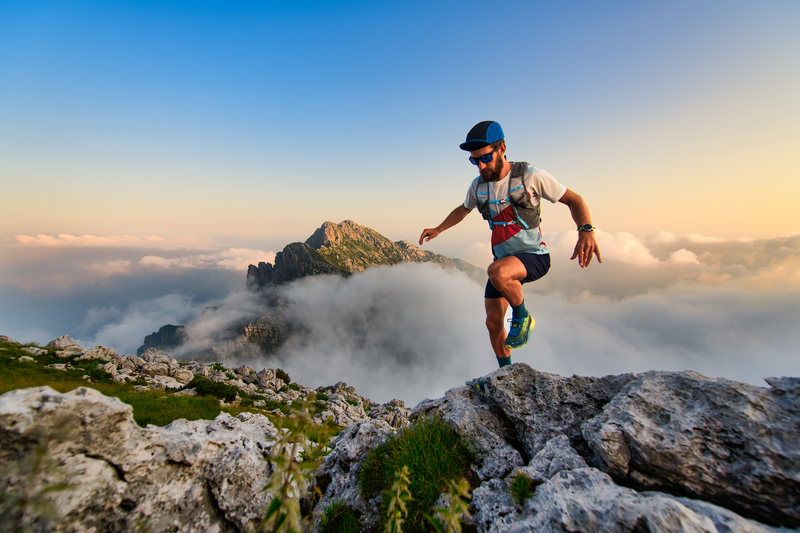
95% of researchers rate our articles as excellent or good
Learn more about the work of our research integrity team to safeguard the quality of each article we publish.
Find out more
ORIGINAL RESEARCH article
Front. Energy Res. , 14 April 2021
Sec. Smart Grids
Volume 9 - 2021 | https://doi.org/10.3389/fenrg.2021.659154
This article is part of the Research Topic New Solutions for Smart Grids with High-Penetration Distributed Energy Resources View all 19 articles
Electricity production and consumption must be balanced for the electrical grid. However, the rapidly growing intermittent power sources are now challenging the supply-demand balance, leading to large flexibility needs for grid management. The plant integrating biomass gasification and reversible solid-oxide cell stacks can be potential means of flexibility, which could flexibly switch among power generation, power storage, and power neutral modes. This paper investigates the economic feasibility of such grid-balancing plants, i.e., plant capital expenditure (CAPEX) target, via a systematic overall decomposition-based methodology for real geographical zones and flexibility-need scenarios. The plant CAPEX target (€/ref-stack) is defined as the maximum affordable investment cost for each reference stack (active cell area 5,120 cm2). The results show that, for a 5-year payback time, 5-year stack lifetime, and 40 €/MWh grid balancing price, the plant concept with 10–100 MWth gasifier has high economic potential with target reaching 17,000 €/ref-stack; however, the plant concept with 100–1,000 MWth gasifier has a limited commercialization potential with the target reaching below 1,000 €/ref-stack due to high biomass supply costs. Considering the sale of chemical product, plant CAPEX target can reach up to 22,000 and 3,000–12,000 €/ref-stack for the plants with 10–100 and 100–1,000 MWth, respectively. The plant CAPEX target is decreased by increasing the total capacities of all plants deployed since more and more capacities will be put into power neutral mode (isolated from the electrical grid) via the coordination of multiple plants. The plant CAPEX target can be further increased by higher grid up/down-regulating price and longer payback years.
Sustainable renewable energy sources are urgently required to satisfy the rapidly growing energy demand and limit greenhouse gas emissions. The penetration of renewables in the global electricity supply has reached a record of 27% in 2019 and is expected to be 49% in 2030 (IEA, 2020). This rapid growth is largely contributed by wind and solar power (IRE Agency, 2014). The high penetration of the intermittent renewables challenges the electricity market in terms of supply-demand balance, transient and frequency stability, thus the Transmission System Operators (TSOs) will require large flexibility needs for grid management. In Denmark, with a 10 GW offshore wind plant built, the grid flexibility needs will vary between a power shortage of 8 GW to a power surplus of 20 GW (Energinet, 2020).
There is a portfolio of supply- and demand-side options for advanced TSOs evolving toward high flexibility by means of, e.g., flexible power generators as a capacity reserve, demand-side management, cross-region interconnections, and crucial alternative energy storage (Koltsaklis et al., 2017). Particularly, when the penetration of renewable power becomes high enough that energy is no longer a limiting factor, while thermal power plants and nuclear power plants will still give a firm supply and not be dispatched frequently, there will be a considerable power surplus (Energinet, 2020). The excess electricity could be addressed by energy storage technologies, which store excess energy and release it when needed. A renewable energy penetration of up to 50% can be addressed in Texas by employing a storage capacity of average daily energy demand, which is 15% higher than only employing flexible power generators as reserve (Denholm and Hand, 2011).
Various energy storage technologies are available. Currently, physical storage options, including pumped-hydro storage and thermal storage, are dominating, i.e., 50% of the global flexibility needs (European Commission, 2016); however, they are generally limited by geographical and environmental restrictions, or low energy conversion efficiency (Frate et al., 2021). Electrochemical storage options, particularly lithium batteries and flow batteries, have gained large development and reached commercialization. Batteries can participate in primary frequency response and also energy management (Lee et al., 2019), expected to account for 25% of the 2030 flexibility needs in Belgium (Elia, 2019). However, large-scale, long-term energy management may be better handled by electrolysis-based power-to-hydrogen technologies, which convert excess electricity to hydrogen and its derivatives. The stored chemical energy can be converted back to electricity via fuel-to-power. This energy storage-release cycle is named as power-to-x-to-power (PXP). It has been expected that over 20% of Danish electricity will be converted to hydrogen or hydrogen-based fuels in 2030 for the down-regulation of the electrical grid (Energinet, 2020), while the fuels generated can be converted to electricity for the up-regulation of the grid, or injected to chemical and transportation sectors.
Economic flexibility of power-to-hydrogen system for down-regulation of the grid has been investigated in the literature, which mainly focuses on the systems enabled by proton exchange membrane or alkaline electrolyzers (Jorgensen and Ropenus, 2008; Paulus and Borggrefe, 2011; Kroniger and Madlener, 2014; Guinot et al., 2015; Grueger et al., 2017). The power-to-hydrogen systems can hardly be economically viable due to (1) low hydrogen production efficiency of 65% (lower heat value, LHV) (Grueger et al., 2017), (2) high investment cost 3,400 €/kW (Jorgensen and Ropenus, 2008; Guinot et al., 2015), and (3) expensive hydrogen storage (Kroniger and Madlener, 2014). Those power-to-hydrogen systems for down-regulation of the grid can further attach a hydrogen-to-power system for up-regulation of the grid via fuel cells. However, using proton exchange membrane fuel cell for Germany secondary control reserve market is also not economically potential (Grueger et al., 2017), because (1) the threshold of hydrogen price is calculated to be 1.1 €/kg, which is far from the current market price (around 5 €/kg; Apostolou, 2020); and (2) the annual equivalent full-load hours are as low as 150 h, due to the expensive bidding balance service using hydrogen.
The investment costs of PXP are mainly contributed by the fuel cell and electrolyzer stacks, which could be largely reduced by employing solid-oxide technology. The same solid-oxide stack can operate “reversibly” by switching between fuel cell mode and electrolysis mode. Thus, the solid-oxide cell can also be called reversible solid-oxide cell (RSOC) and enables a single RSOC-based plant to work for power generation (PowGen) or power storage (PowSto) at different time periods. This reversible operation of the same plant can potentially (1) enhance the utilization hours, (2) reduce the total capital expenditure (CAPEX), (3) achieve high round-trip efficiency of 55–70% (LHV) (Butera et al., 2020; Mogensen, 2020; Motylinski et al., 2021), and (4) utilize hydrocarbons as fuels directly, such as methane (Mogensen, 2020) and methanol (Butera et al., 2020) to avoid expensive hydrogen storage. Additional CO2 sources needed for the case of hydrogen derivatives can be solved by combining the biomass with RSOC systems.
Using renewable, carbon-neutral, and widely available biomass, RSOC-based plants are potential to provide grid balancing services, which is called as Waste2GridS (Waste2GridS, 2020) (W2G) plants. In the literature, only a few case studies of such a plant concept can be found, e.g., RSOC-based plants for power generation and power storage fueled by wood chips (Sigurjonsson and Clausen, 2018) or municipal solid wastes (Perna et al., 2018) with an improvement in Butera et al. (2020), where four operating modes are proposed to cope with different grid flexibility needs. These studies focus mainly on the thermodynamic perspective. The authors have recently proposed a triple-mode plant concept for grid balancing in Wang L. et al. (2020) by connecting the sectors of electrical grid, gas grid, and chemical market (Figure 1). The plant concept integrates biomass/waste gasification and RSOC technology, and can interact with or be isolated from the electrical grid as capacity reserves, thus is capable of non-stop operation all year round. The optimal conceptual plant design has been investigated by focusing on the thermodynamic performances, leading to an application-free pool of optimal plant designs.
This paper is a follow-up of our previous study (Wang L. et al., 2020). The objective is to evaluate in a systematically manner from both the technological and application viewpoints, the economic feasibility of the W2G plants in 2030, with a decomposition-based optimization method. The maximum affordable plant CAPEX, named as plant CAPEX target, is evaluated by considering (1) different conversion technology combinations, (2) different geographical zones, (3) different magnitudes of grid flexibility needs, (4) a number of plant design alternatives, (5) optimal plant sizing and cooperative operation of multiple plants, and (6) minimum cost for biomass supply chain. Sensitivity analysis of key influencing factors are also performed, including (1) grid regulating prices, (2) payback time of capital expenditure, and (3) revenue from chemical product sale.
The remaining paper is organized as follows: In section 2, the W2G plant is introduced with the description of plant concept. Then, method for evaluating the economic feasibility of W2G plants is described in section 3 with the overall method introduced first followed by detailed mathematical formulations. In section 4, the applications of W2G plants for addressing grid flexibility needs are specified. Economic feasibility of W2G plants in applications is discussed comprehensively in section 5. Finally, conclusions are drawn in section 6.
The generic plant concept proposed in our previous work (Wang L. et al., 2020) is illustrated in Figure 2. The plant is comprised of the processes of onsite biomass pretreatment, gasification, syngas cleaning, RSOC stacks, methanation, heat exchanger network, and steam turbine network for heat recovery. The biomass is pretreated and gasified to produce syngas, which is further cleaned in the cleaning section. The clean syngas is fed to the RSOC-methanation subsystems for electricity or methane production.
Figure 2. The generic concept of W2G plant (Wang L. et al., 2020). The chemical produced in the PowSto and PowNeu modes is synthesis natural gas, due to the existing large infrastructure.
The biomass pretreatment, gasification, and syngas cleaning sections are considered to operate at full load all the time with no load shifting, so that the same amount of clean syngas can be produced continuously for the RSOC-methanation subsystems. The mode switch of the plant is enabled by the change of operating modes of the RSOC-methanation subsystems. Specifically, the RSOC subsystems involves two RSOC blocks, whose coordination as shown in Figure 2 enables the switch among the PowGen, PowSto, and PowNeu modes:
• PowGen mode: biomass-to-electricity with both RSOC blocks under the fuel cell mode, offering up-regulation service.
• PowSto mode: biomass-to-chemicals with both RSOC blocks under electrolyzer mode powered by electricity, offering down-regulation service.
• PowNeu mode: biomass-to-chemical with one RSOC block under fuel cell mode to power the other block under electrolyzer mode for chemical production. The plant is not interacted with the electrical grid.
The thermodynamic performance is affected by multiple degrees of freedom of plant designs, including (1) the combination of gasification technology (entrained flow gasifier or circulating fluidized bed gasifier), syngas cleaning technology (hot/cold), electrolysis mode (steam electrolysis or co-electrolysis), (2) the design operating points of the key components, particularly the stacks (under both fuel cell and electrolyzer modes), (3) optimal heat cascade utilization, and (4) the optimal placement of steam turbine network. Two gasifier options were considered: entrained-flow gasifier with direct heating (EFG) for large-scale applications (100–1,000 MWth), and fast internally circulating fluidized-bed (FICFB) for medium-scale applications (10–100 MWth).
These degrees of freedom of process design have been considered in the optimal conceptual plant design performed in Wang L. et al. (2020), which employed a well-established multi-period, multi-objective process optimization method. The optimization considers three objective functions: the PowGen efficiency, the PowSto efficiency, and the specific cell area needed to process 1 kW-LHV dry basis biomass. A pool of optimal Pareto designs is obtained, revealing the trade-off between the three objectives. Thus, for different plant designs, the plants with the same stack size can provide up- and down-regulation services at different capacities.
For the deployment of W2G plants, a case study must based on a specific geographical zone to consider realistic (or reasonably predicted) grid flexibility needs and biomass availability. However, it is difficult to simultaneously consider the non-linear programming for optimal conceptual plant design and the mixed-integer programming for optimal plant scheduling to cope with a specific imbalance profile, not even to mention the computation-expensive supply chain optimization. Thus, an overall decomposition-based methodology has been initially proposed by Wang L. et al. (2020) and is slightly adapted as shown in Figure 3. The method involves the following seven steps with detailed described in Wang L. et al. (2020):
• Step 1: Assessment of future grid flexibility needs of the specific geographical zones, referring to Olsen et al. (2020),
• Step 2: Assessment of future biomass availability (i.e., a geodatabase with biomass weight, characteristics and location coordinates) of the specific geographical zones, referring to Láınez et al. (2011), Pérez-Fortes et al. (2014), and Puigjaner (2011),
• Step 3: Generation of plant design pool and preselection of plant design, referring to Wang L. et al. (2020),
• Step 4: Optimal design selection, and plant sizing and scheduling to maximize the profits from grid-balancing services when addressing the grid flexibility needs identified in step 1,
• Step 5: Minimization of biomass supply chain cost given the plant sizes resulted from step 4,
• Step 6: Evaluation of the economic indicator, i.e., Plant CAPEX Target, by considering the profits (step 4) and costs (step 5),
• Step 7: Business case evaluation with actual plant CAPEX evaluation to determine the economic prerequisites for potential business cases.
Figure 3. The decomposition-based method to identify potential business cases for the triple-mode grid-balancing plants, adapted from Wang L. et al. (2020).
This work focuses on the step 4–6, i.e., red-colored task in Figure 3 to calculate the plant CAPEX target, so that potential case studies can be listed. The step 4 performs (1) optimal design selection from design pool, (2) optimal sizing and scheduling (i.e., mode switch) of the plants deployed, and (3) optimal capacity and scheduling of the auxiliaries, particularly the oxygen tank. Particularly, the deployment of multiple plants are considered to increase the profits from the grid balancing services. Thus, it concludes the grid-balancing profits, and the corresponding design, size, and operation of each plant. Then, for each plant with its size obtained, the costs of biomass supply chain are minimized in step 6 to enhance the economic feasibility and determine the plant location. Then, the maximum affordable plant investment cost, i.e., the Plant CAPEX Target (€/ref-stack) defined as the maximum affordable total plant investment costs divided by the equivalent number of reference stacks (ref-stack, each with 5,120 cm2 active cell area) of all plants deployed in a case study, can be calculated for a payback time of l years:
The plant CAPEX target is an economic index of the W2G plant, including the investment costs of components employed in the RSOC blocks and biomass gasification. Those case studies with high plant CAPEX target are considered to be economically potential, and each case study provides exact information on geographical zones (grid flexibility needs and biomass availability), the plants deployed (design, location, size, and operation), and biomass supply chain.
The mathematical formulations for step 4, optimal plant design selection and optimal plant sizing and scheduling, are presented in this section. To reduce the computation efforts, the hourly imbalance profiles with 8,760 points are processed to typical days.
The profit of the W2G plants deployed for l payback years (Equation 2) is associated with (1) the revenue from grid-balancing services , €, (2) additional revenue (positive) or cost (negative) of oxygen trade with the chemical market , €, and (3) the cost of oxygen storage tank Rtank, €:
where αd is the repetition time of the typical day d in an entire year, i is the hour in a day, and r is the interest rate (0.05).
The revenue from grid-balancing services can be calculated by the balancing energy Eu, d, i, n (MWh) and the regulating price (€/MWh) for both PowGen and PowSto modes:
where n represents PowGen, PowSto, or PowNeu mode, u is the index of plant design in the design pool.
The additional oxygen revenue is calculated based on the trade with market, outflow , inflow , kg/h, and the oxygen price θoxy, €/kg:
The cost of oxygen storage tank Rtankis evaluated by the tank capacity m (kg) and the tank price θtank (€/kg):
The grid balancing power provided by the plants deployed at each time step (d, i) is calculated based on the mode and capacity of each plant, and only mode switch but no load shifting is considered:
where u,n (MW) is the capacity of the plant using design u in mode n (PowGen or PowSto), the binary variable Yu represents whether the design u is selected or not, ru is the sizing factor scaling from the reference size of the design to the size of the actual plant deployed, and the binary variable Zu, d, i, n represents the status of the PowGen and PowSto modes. The coordination among multiple plants is considered by employing multiple plants U:
The grid balancing power provided by the plants in the PowGen and PowSto modes should be lower than the up-regulation and down-regulation needs (MW):
The status of each plant is unique in a time step (d, i):
The oxygen storage level (kg) in the gas tank is limited by the tank capacity m (kg):
The storage level of the tank in time (d, i) is related to the the level in previous hour (d, i−1) and the oxygen generated/consumed by plants (, , kg/h), and the trade with the chemical market (, , kg/h):
The oxygen production and generation in time step (d, i) are calculated based on the design value , kg/h, and the sizing factor:
The storage levels in the first and the last hour in each typical day are set to be equal for continuous operation of the storage tanks:
Existing wind and solar extreme zones (with a high penetration of variable renewable energy sources) in Europe (e.g., Denmark and Italy) are identified to provide typical grid flexibility needs. The zones in-and-around the electricity market zones DK-DK1 (northern Denmark) and IT-SUD (southern Italy), as shown in Figure 4A, are selected for deploying the overall methodology (section 3) to determine the economic feasibility of the W2G plants. The W2G plants are expected to provide up-regulation under the PowGen mode (biomass-to-electricity), down-regulation under the PowSto mode (biomass-to-chemical), or reserve capacity under the PowNeu mode (biomass-to-chemical). All evaluations are for the timeline 2030.
Figure 4. The grid flexibility needs: (A) Geographic locations and (B) theoretical and scaled flexibility needs.
The theoretical flexibility needs in 2030 of the two zones have been predicted in Olsen et al. (2020) based on historical data and available forecast data by using the multi-timescale data-driven method. The hourly profiles (365 days, 8,760 values) are clustered into a set of typical days using the k-means method developed in Fazlollahi et al. (2014) to reduce the number of data points and hence the computational load. The theoretical flexibility needs of DK-DK1, IT-SUD in 2030 represented by typical days are shown in Figure 4B, which vary within -6–2 GW and -7–3 GW.
Real grid flexibility needs to be coped by the W2G plants will be smaller or even much smaller than the theoretical flexibility needs, due to multiple flexibility means. In 2030, cross-country interconnections are expected to play an important role in coping with the flexibility needs, while conventional technologies will not be completely replaced by new technologies, as agreed by several TSOs (European Commission, 2016; Elia, 2019; Energinet, 2020). Unfortunately, Terna (the Italian TSO) and Energinet (the Danish TSO) have not provided their official prediction for the real flexibility needs for 2030.
The grid flexibility needs addressed by the W2G plants are thus scaled from the theoretical flexibility needs, with the sizing factors taken from the Adequacy and Flexibility Study of Belgian TSO (Elia, 2019), which predicts the 2030 flexibility needs of Belgium. It is reported that in 2030, 34% of the up-regulation and 32% of the down-regulation can be handled by cross-country interconnections. Considering also batteries and classical thermal power plants, only 14% of the up-regulation and 30% of the down-regulation may be handled by the W2G plants and other competing technologies. Thus, two scenarios are defined to represent the flexibility needs for the W2G technology:
• S1: excluding interconnections: 66% of theoretical up-regulation needs and 68% of theoretical down-regulation needs,
• S2: excluding interconnections, batteries, and classic plants: 14% of theoretical up-regulation needs and 30% of theoretical down-regulation needs.
The theoretical flexibility needs and the two scenarios defined have been given in Figure 4B. The scenarios S1 and S2 will result in the deployment of W2G plants at different scales. Considering the economy of scale, a capacity factor x is introduced to set a basis for the comparison of the deployment under the two scenarios. It represents the contribution of the W2G plants installed to address the target flexibility needs:
with the symbols introduced for Equation (6).
Available biomass in-and-around the specific zones are assessed considering the resources of the zones. In IT-SUD, the main available biomass comes from agriculture residues, forestry, and municipal solid waste (organic, paper, and wood). The amount of the available biomass in 2030 is predicted by a geodatabase built based on the future Europe directives in The European Parliament and The Council Of The European Union (2018), with energy (LHV) evaluated as 19 TWhth in 2030. In DK-DK1, agricultural residues, forestry, and municipal solid waste organic fractions are available, which are able to supply 22 TWhth in 2030 referring to work in S2Biom project (S2Biom, 2016). The detailed distributions of each type of biomass are fed to the optimization of biomass supply chain.
The 27 plant designs considered in step 4–6 are preselected from the Pareto-front obtained in our previous study (Wang L. et al., 2020). These designs consider the trade-off between specific cell area m2/kW-LHV biomass, PowGen efficiency and PowSto efficiency, as shown in Figure 5.
Figure 5. The pool of plant design with FICFB and EFG option: (A) PowGen efficiency vs. specific cell area (B) PowSto efficiency vs. specific cell area.
The characteristics of each design used for optimal deployment (step 4 in Figure 3) include:
• Specific PowGen capacity: Net electricity generation, kWe/ref-stack,
• Specific PowSto capacity: Total electricity consumption, kWe/ref-stack,
• Oxygen consumed/generated in each mode, (kg/h)/ref-stack,
• Synthesis natural gas (SNG) produced in each mode, (kg/h)/ref-stack,
• Biomass energy need, in MWth/ref-stack.
The key economic parameters specified in the optimal plant deployment are given in Table 1.
The economic feasibility of the W2G plants is evaluated with the plant CAPEX target (€/ref-stack), with an overview given first and a subsequent investigation of the effects of the influential factors.
The plant CAPEX targets (Equation 1) with respect to the capacity factor x (Equation 15) are shown in Figure 6 for a payback time of 5 years, a stack lifetime of 5 years, different flexibility-need scenarios and plant technologies. The longest acceptable duration of the W2G plants is set as 5 years, which approaches the stack lifetime of cutting-edge technologies (SOLIDpower, 2017). Overall, the FICFB-based W2G plants with typical gasifier size of 10–100 MWth biomass feed with the installed capacity factor x below 8% show high economic-potential, with the plant CAPEX target reaching over 16,000 €/ref-stack. While the EFG based W2G plant with typical gasifier size of 100–1,000 MWth biomass feed can hardly be economically feasible due to the low and even negative plant CAPEX target. The FICFB-based W2G plant achieves a plant CAPEX target of 2,000–17,000 €/ref-stack, which is significantly higher than that of the EFG based plant (i.e., -5,000 to 1,000 €/ref-stack). This is mainly due to the profit from grid-balancing services and the costs of biomass supply, which will be further analyzed below.
Figure 6. Plant CAPEX target of the case studies with different plant technologies, geographical zones, and flexibility-need scenarios. Note the profit from methane sale is not considered in this figure. The reference stack is defined as a stack with a total active cell area of 5,120 cm2.
The plant CAPEX target decreases with the increase in the capacity factor x, i.e., an increase in the plant capacities for a given imbalance to be handled. Particularly, when the capacity factor of the FICFB-based W2G plant is around 3%, the plant CAPEX target can be up to 17,000 €/ref-stack for the case study SUD-S1. Increasing the capacity factor to 10%, the plant CAPEX target is sharply decreased to 5,000 €/ref-stack.
For the plants with the same gasification technology, there is no big difference in plant CAPEX target among different magnitudes of grid flexibility needs (S1 and S2) and different zones (DK-DK1 or IT-SUD). For the FICFB-based W2G plant, the variation of plant CAPEX targets among different cases with similar sizes of plants deployed is less than 1,000 €/ref-stack.
Referring to the definitions of plant CAPEX target (Equations 1 and 2), the major influential factors leading to such observations are (1) the total size of all plants deployed, represented by the total number of reference stacks employed, (2) the revenue from grid balancing services, (3) the costs of onsite oxygen storage, (4) the cost/revenue from oxygen trade with the chemical market, and (5) the costs of biomass supply. The effects of these factors will be further discussed below.
The W2G plants switch between PowGen and PowSto modes to interact with the grid by providing or consuming electricity. Since the W2G plant is set to operate full load under all modes, when the grid flexibility needs are lower than the PowGen or PowSto capacities of a plant, the plant will be put under PowNeu mode. The optimal operating strategy of the W2G plants for SUD-S2 is taken as an example as shown in Figure 7. The maximum flexibility needs are 450 MWe for up-regulation and 2,200 MWe for down regulation.
Figure 7. Dispatch of the plant deployed to cope with the flexibility needs in SUD-S2: (A) single FICFB-based plant, (B) seven EFG-based plants.
For FICFB-based W2G plant (Figure 7A), the power capacity of the plant is limited to 10–100 MWth biomass feed. When employing a single plant, the optimal plant capacity is with a gasifier capacity of 100 MWth providing 60 MWe for the PowGen mode and 160 MWe for the PowSto mode. Such power capacities are far below the grid flexibility needs, with the capacity factor of only 3%. Thus, the plant is rarely put into PowNeu mode due to the constraint of grid flexibility needs (Equation 8).
For EFG-based W2G plant (Figure 7B) with a capacity of 100–1,000 MWth biomass feed, seven plants can provide 760 MWe for the PowGen mode and 2,270 MWe for the PowSto mode, reaching a capacity factor of up to 110%. Over 90% grid flexibility needs are covered by the cooperative operation of plants, as shown in Figure 7B; however, this coordination also increases the operating hours of PowNeu mode.
The utilization hours of the PowNeu mode increase with the increasing capacity factor x, i.e., the increased capacity of all plants deployed. This remains the same for different geographical zones (DK1 and SUD) and magnitudes of flexibility needs (S1, S2) as shown in Figure 8. For the FICFB-based plant, the annual utilization hour of the PowNeu mode of scenario S1 is lower than that of scenario S2. For the scenario S1, the annual utilization hours of the PowNeu mode are within 300–1,900 h with capacity factors in 3–25%; Under the scenario S2 with less flexibility needs, the capacity factors are increased to 8–60%, resulting in an annual PowNeu operation of 600–3,600 h. For the EFG-based plant, the annual PowNeu utilization hours reach over 2,500 h and even up to 6,500 h, because larger EFG-based plants allow for coping with larger flexibility needs and thus higher profit, with the capacity factor achieving 27–200%.
Figure 8. Average annual utilization hours of the PowNeu mode with the case of FICFB-based plants highlighted.
The profits of the W2G plants defined in Equation (2) are affected by (1) the revenue from providing grid flexibility, (2) the cost/revenue from oxygen trade, and (3) the cost of oxygen storage tank. The maximum profits under different geographical zones and magnitudes of flexibility needs are illustrated in Figure 9. Increasing the total capacities of all plants deployed with an increase in the number of plant, the maximum profit obtained by 1 MWhth biomass will be decreased. This is mainly due to the high utilization of PowNeu mode under high capacity factor. High annual PowNeu utilization hours indicate low utilization of the PowSto and PowGen modes. This results in a reduced profit, due to that (1) there is no extra grid balancing gain under the PowNeu mode and (2) the PowNeu mode reaches a lower efficiency than the PowSto and PowGen modes.
The profit of the FICFB-based plants is from 40 €/MWhth (capacity factor 25% for DK1 and 20% for SUD) to 130 €/MWhth (capacity factor 4% for DK1, 3% for SUD) of scenario S1, and from 30 €/MWhth (capacity factor of 60% for DK1, and 45% for SUD) to 130 €/MWhth (capacity factor 10% for DK1 and 8% for SUD) from scenario S2. The revenue from addressing the flexibility needs contributes over 75% of the profits, with the remaining part contributed by oxygen sale. Increasing the capacity factor, the contribution of oxygen sale is increased from 6 to 25% due to the utilization of PowNeu mode. For the FICFB-based plant, the plants have oxygen generation on the PowNeu mode. For the EFG-based plant, the profits are lower, 6–21 €/MWhth due to high utilization hours of PowNeu mode as shown in Figure 8, and higher oxygen consumption of the PowNeu mode.
The costs of biomass supply chain, considering biomass collection from geographical zones, pre-treatment, and storage, should be minimized. The superstructure-based method presented in Puigjaner (2011), Láınez et al. (2011), and Pérez-Fortes et al. (2014) is adopted. Biomass is required all the time as long as the W2G plants are under operation, based on the plant concept in Figure 2. For the FICFB-based plant, the costs of biomass supply chain account for 10–50% of the profits as shown in Figure 10, supplying 1–6 TWhth biomass a year. For the larger EFG-based plant, the annual biomass needs are sharply increased to 5–37 TWh/year, costing at least 75% of the profit and even five times more than the profit. Such big biomass supply costs make the EFG-based plants hardly economically-feasible. When the biomass collection is over 10 TWh/year, biomass production costs, i.e., dry and storage, will be significantly increased, since biomass with a lower LHV and high moisture content has to be chosen. Moreover, when biomass need cannot be satisfied by the available waste in the zones considered, i.e., 19 TWhth/year in DK-DK1 and 22 TWh/year in IT-SUD, additional biomass has to be collected from the neighboring zones with potentially high transportation costs.
Figure 10. The proportion of biomass supply chain cost on profit with the FICFB-based plant highlighted.
This section presents a sensitivity analysis with respect to grid regulating price, payback time, and the revenue from SNG sale. Those parameters are critical for determining the economic feasibility.
The plant CAPEX target is evaluated with a up/down-regulating price of 20–80 €/MWh (Skytte, 1999) and a payback time of 1–4 years as shown in Figure 11 for the case SUD-S1 with a capacity factor of 9%.
Figure 11. Plant CAPEX target with different payback years and regulating prices for the cases of SUD-S1.
The plant CAPEX target decreases if reducing the up/down-regulating prices or the payback time. It may reach 8,600 €/ref-stack with 5 payback years if the regulating price is up to 80 €/MWh. While, if the regulating price is down to 20 €/MWh, the plant CAPEX target should be lower than 700 €/ref-stack to have the chance of being profitable for a 1-year payback time. The plant CAPEX target evaluated with a payback time of 1–4 years is affected by the oxygen tank costs incurred in the first year and the sum of the revenue from providing grid balancing service occurring before reaching the set payback year. With a grid balancing price of 20 €/MWh, the plant CAPEX targets are reduced from 3,300 €/ref-stack for a payback time of 5 years to 1,400 €/ref-stack for a payback time of 2 years. However, even under the most potential situation (80 €/MWh, five payback years), the cases of employing three plants is less economically-competitive than those with one single plant.
The revenue from the sale of SNG generated during the PowSto and PowNeu modes will further enhance the economic feasibility of the case studies, as shown in Figure 12. The FICFB-based plant achieves a higher plant CAPEX target than the EFG option under the same scenario. The plant CAPEX target is in 8,000–22,000 €/ref-stack for the FICFB-based plant but is limited to 12,000 €/ref-stack for the EFG-based plant, which can even be down to 3,000 €/ref-stack. The plant CAPEX target also decreases as the increase of plant capacities deployed. There is still no big difference in the plant CAPEX target among different zones and different flexibility-need scenarios when using the same gasifier type and plant number.
Figure 12. Plant CAPEX target recalculated by considering the revenue from SNG sale to market, considering the price of SNG being 0.6 €/kg (IEA, 2019).
The economic feasibility of a novel grid-balancing plants integrating biomass gasification and reversible solid-oxide cell stacks is evaluated by the plant CAPEX target, defined as the maximum affordable plant investment cost (€/ref-stack, a reference stack with 5,120 cm2 active cell area). A decomposition-based methodology is implemented to calculate the economic indicator considering different grid flexibility needs, biomass availability, and plant designs. The profit from providing grid-balancing services is maximized by cooperating multiple plants, which vary in plant design, size, and operation. Further, with the minimization of biomass supply chain, the plant CAPEX target is carried out for a set of cases in different geographical zones (DK1, SUD) and flexibility-need scenarios. The key conclusions are:
• The plants with the size of 10–100 MWth are potential to realize high economic feasibility with the plant CAPEX target reaching up to 17,000 €/ref-stack (5-year payback time, 5-year stack lifetime, and 40 €/MWh regulating price). Counting the chemical-sale profit, plant CAPEX target can even reach 22,000 €/ref-stack.
• The plants with the size of 100–1,000 MWth are much less economically feasible due to the high biomass supply chain costs, which take at least 70% of and even more than the profit gained from energy balancing of the grid. The plant CAPEX target of the plants of such sizes is 3,000–12,000 €/ref-stack with the gain from the sale of synthesis natural gas.
• For the plant enabled by the same type of gasification technology, there is no big difference in plant CAPEX target in different zones and flexibility-need scenarios. The plant CAPEX target can be further increased by higher grid up/down regulating price and longer payback years.
• The economic feasibility is affected by their contribution to address the targeted grid flexibility needs. The higher the contribution, the more plant capacities deployed will be coordinated to operate under the PowNeu mode. The increased annual utilization hours of the PowNeu mode, which does not gain from energy-balancing services, cause a decrease in the plant CAPEX target.
The plant CAPEX should be calculated in future work based on the process flow diagram and the component sizes of each plant deployed. Comparison of the plant CAPEX and plant CAPEX target can further reveal the economic feasibility of W2G plants.
The original contributions presented in the study are included in the article/supplementary material, further inquiries can be directed to the corresponding author/s.
YZ: data curation, formal analysis, and writing - original draft. NW and LD: supervision, validation, and writing - review, editing. CL: data curation and writing - review, editing. JV and YY: funding acquisition, resources, and writing - review, editing. FM: resources, software, and writing - review, editing. TL: Writing - review, editing. LW: conceptualization, methodology, supervision, and writing - review, editing. All authors contributed to the article and approved the submitted version.
The authors declare that the research was conducted in the absence of any commercial or financial relationships that could be construed as a potential conflict of interest.
LW and JV have received funding from the European Union's Horizon 2020 under grant agreement No. 826161 (Waste2GridS), 826234 (Waste2Watts), 815284 (BLAZE), and 735692 (CH2P), and the support from the Fuel Cells and Hydrogen Joint Undertaking, Hydrogen Europe and Hydrogen Europe research. LD has received funding from the National Nature Science Foundation of China (Grant No. 52076078) and the National Science and Technology Major Project (Grant No. 2017-I-0002-0002). YZ has received funding from the Fundamental Research Funds for the Central Universities (2019QN029). YY has received funding from the National Nature Science Foundation of China (Grant No. 51821004).
Apostolou, D. (2020). Optimisation of a hydrogen production – storage – re-powering system participating in electricity and transportation markets. a case study for denmark. Appl. Energy 265:114800. doi: 10.1016/j.apenergy.2020.114800
Bellotti, D., Rivarolo, M., Magistri, L., and Massardo, A. (2017). Feasibility study of methanol production plant from hydrogen and captured carbon dioxide. J. CO2 Util. 21, 132–138. doi: 10.1016/j.jcou.2017.07.001
Butera, G., Jensen, S. H., Gadsbøll, R. Ø., Ahrenfeldt, J., and Clausen, L. R. (2020). Flexible biomass conversion to methanol integrating solid oxide cells and TwoStage gasifier. Fuel 271:117654. doi: 10.1016/j.fuel.2020.117654
Denholm, P., and Hand, M. (2011). Grid flexibility and storage required to achieve very high penetration of variable renewable electricity. Energy Policy 39, 1817–1830. doi: 10.1016/j.enpol.2011.01.019
Energinet (2020). System Perspectives for the 70% Target and Large-Scale Offshore Wind. Technical report, Energinet.
European Commission (2016). The Role and Need of Flexibility in 2030: Focus on Energy Storage. Technical report, European Commission.
Fazlollahi, S., Bungener, S. L., Mandel, P., Becker, G., and Maréchal, F. (2014). Multi-objectives, multi-period optimization of district energy systems: I. selection of typical operating periods. Comput. Chem. Eng. 65, 54–66. doi: 10.1016/j.compchemeng.2014.03.005
Frate, G. F., Ferrari, L., and Desideri, U. (2021). Energy storage for grid-scale applications: technology review and economic feasibility analysis. Renew. Energy 163, 1754–1772. doi: 10.1016/j.renene.2020.10.070
Grueger, F., Mhrke, F., Robinius, M., and Stolten, D. (2017). Early power to gas applications: reducing wind farm forecast errors and providing secondary control reserve. Appl. Energy 192, 551–562. doi: 10.1016/j.apenergy.2016.06.131
Guinot, B., Montignac, F., Champel, B., and Vannucci, D. (2015). Profitability of an electrolysis based hydrogen production plant providing grid balancing services. Int. J. Hydrogen Energy 40, 8778–8787. doi: 10.1016/j.ijhydene.2015.05.033
IRE Agency (2014). Remap 2030 - A Renewable Energy Roadmap. Agency I R E. REmap 2030 - A renewable energy roadmap[J]. International Renewable Energy Agency.
Jorgensen, C., and Ropenus, S. (2008). Production price of hydrogen from grid connected electrolysis in a power market with high wind penetration. Int. J. Hydrogen Energy 33, 5335–5344. doi: 10.1016/j.ijhydene.2008.06.037
Koltsaklis, N. E., Dagoumas, A. S., and Panapakidis, I. P. (2017). Impact of the penetration of renewables on flexibility needs. Energy Policy 109, 360–369. doi: 10.1016/j.enpol.2017.07.026
Kroniger, D., and Madlener, R. (2014). Hydrogen storage for wind parks: a real options evaluation for an optimal investment in more flexibility. Appl. Energy 136, 931–946. doi: 10.1016/j.apenergy.2014.04041
Laínez, J. M., Pérez-Fortes, M., Bojarski, A. D., and Puigjaner, L. (2011). "Raw materials supply,” in Syngas From Waste, editor L. Puigjaner (London: Springer), 23–54.
Lee, R., Homan, S., Dowell, N. M., and Brown, S. (2019). A closed-loop analysis of grid scale battery systems providing frequency response and reserve services in a variable inertia grid. Appl. Energy 236, 961–972. doi: 10.1016/j.apenergy.2018.12.044
Mogensen, M. B. (2020). Materials for reversible solid oxide cells. Curr. Opin. Electrochem. 21, 265–273. doi: 10.1016/j.coelec.2020.03.014
Motylinski, K., Kupecki, J., Numan, B., Hajimolana, Y. S., and Venkataraman, V. (2021). Dynamic modelling of reversible solid oxide cells for grid stabilization applications. Energy Convers. Manage. 228:113674. doi: 10.1016/j.enconman.2020.113674
Olsen, K. P., Zong, Y., You, S., Bindner, H., Koivisto, M., and Gea-Bermúdez, J. (2020). Multi-timescale data-driven method identifying flexibility requirements for scenarios with high penetration of renewables. Appl. Energy 264:114702. doi: 10.1016/j.apenergy.2020.114702
Paulus, M., and Borggrefe, F. (2011). The potential of demand-side management in energy-intensive industries for electricity markets in germany. Appl. Energy 88, 432–441. doi: 10.1016/j.apenergy.2010.03.017
Pérez-Fortes, M., Laínez-Aguirre, J. M., Bojarski, A. D., and Puigjaner, L. (2014). Optimization of pre-treatment selection for the use of woody waste in co-combustion plants. Chem. Eng. Res. Design 92, 1539–1562. doi: 10.1016/j.cherd.2014.01.004
Perna, A., Minutillo, M., Lavadera, A. L., and Jannelli, E. (2018). Combining plasma gasification and solid oxide cell technologies in advanced power plants for waste to energy and electric energy storage applications. Waste Manage. 73, 424–438. doi: 10.1016/j.wasman.2017.09.022
S2Biom (2016). Delivery of Sustainable Supply of Non-food Biomass to Support a Resource-Efficient Bioeconomy in Europe. Technical report, European commission.
Sigurjonsson, H. Æ., and Clausen, L. R. (2018). Solution for the future smart energy system: a polygeneration plant based on reversible solid oxide cells and biomass gasification producing either electrofuel or power. Appl. Energy 216, 323–337. doi: 10.1016/j.apenergy.2018.02.124
Skytte, K. (1999). The regulating power market on the nordic power exchange nord pool: an econometric analysis. Energy Econ. 21, 295–308. doi: 10.1016/s0140-9883(99)00016-x
SOLIDpower (2017).SOLIDpower expands production capacity, SOFC runtime record. Fuel Cells Bull. 2017:9. doi: 10.1016/S1464-2859(17)30421-2
The European Parliament And The Council Of The European Union (2018). Directive (eu) 2018/851 of the European Parliament and of the Council.
Wang, C., Akkurt, N., Zhang, X., Luo, Y., and She, X. (2020). Techno-economic analyses of multi-functional liquid air energy storage for power generation, oxygen production and heating. Appl. Energy 275:115392. doi: 10.1016/j.apenergy.2020.115392
Wang, L., Zhang, Y., Li, C., Pérez-Fortes, M., Lin, T.-E., Maréchal, F., et al. (2020). Triple-mode grid-balancing plants via biomass gasification and reversible solid-oxide cell stack: concept and thermodynamic performance. Appl. Energy 280:115987. doi: 10.1016/j.apenergy.2020.115987
Keywords: biomass-to-chemical, grid balancing, biomass-to-electricity, reversible solid-oxide cell, economic feasibility, plant capital expenditure target
Citation: Zhang Y, Wang N, Li C, Pérez-Fortes M, Duan L, Van herle J, Maréchal F, Lin T-E, Wang L and Yang Y (2021) Triple-Mode Grid-Balancing Plants via Biomass Gasification and Reversible Solid-Oxide Cell Stack: Economic Feasibility Evaluation via Plant Capital-Cost Target. Front. Energy Res. 9:659154. doi: 10.3389/fenrg.2021.659154
Received: 27 January 2021; Accepted: 12 March 2021;
Published: 14 April 2021.
Edited by:
Chao Long, Cranfield University, United KingdomReviewed by:
Haoshui Yu, Massachusetts Institute of Technology, United StatesCopyright © 2021 Zhang, Wang, Li, Pérez-Fortes, Duan, Van herle, Maréchal, Lin, Wang and Yang. This is an open-access article distributed under the terms of the Creative Commons Attribution License (CC BY). The use, distribution or reproduction in other forums is permitted, provided the original author(s) and the copyright owner(s) are credited and that the original publication in this journal is cited, in accordance with accepted academic practice. No use, distribution or reproduction is permitted which does not comply with these terms.
*Correspondence: Ligang Wang, bGlnYW5nLndhbmdAbmNlcHUuZWR1LmNu
Disclaimer: All claims expressed in this article are solely those of the authors and do not necessarily represent those of their affiliated organizations, or those of the publisher, the editors and the reviewers. Any product that may be evaluated in this article or claim that may be made by its manufacturer is not guaranteed or endorsed by the publisher.
Research integrity at Frontiers
Learn more about the work of our research integrity team to safeguard the quality of each article we publish.