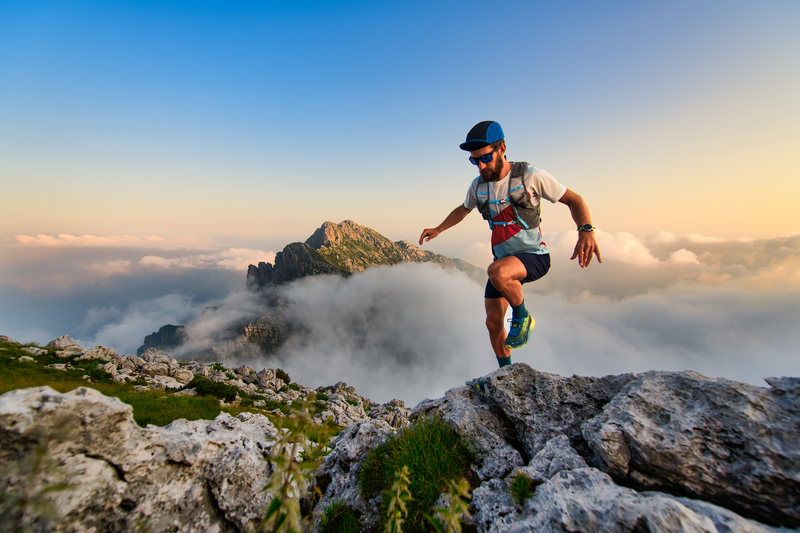
95% of researchers rate our articles as excellent or good
Learn more about the work of our research integrity team to safeguard the quality of each article we publish.
Find out more
ORIGINAL RESEARCH article
Front. Energy Res. , 23 March 2021
Sec. Sustainable Energy Systems
Volume 9 - 2021 | https://doi.org/10.3389/fenrg.2021.651586
This article is part of the Research Topic Policy-Oriented Optimization for Urban Energy-X Nexus View all 8 articles
To investigate the nexus between natural gas consumption, global carbon dioxide (CO2) emissions, and technological innovation, this study employs a balanced panel dataset of 73 countries for the period 1990–2019 based on the fixed effect and random effect estimation methods. Considering potential heterogeneity in the natural gas-CO2 nexus, this study divides the 73 countries into regional comprehensive economic partnership (RCEP) countries and non-RCEP countries for comparative analysis. The main findings indicate that natural gas consumption can significantly promote CO2 emissions for the full sample and non-RCEP countries, and improved technological innovation can help alleviate CO2 emissions from natural gas consumption. In the RCEP countries, technological innovation can improve the carbon emission reduction effect of natural gas. Furthermore, economic growth and global CO2 emissions show an inverted U-shaped relationship, which confirms the environmental Kuznets curve hypothesis. Finally, several policy implications are provided to reduce global CO2 emissions and promote green recovery in the post-epidemic era.
In recent years, the world has witnessed unparalleled economic growth. Simultaneously, this rapid growth has been accompanied by abundant energy consumption and global CO2 emissions. According to statistics from BP (formerly British Petroleum) (BP, 2020), the world’s total primary energy consumption increased nearly twofold between 1990 and 2019, from 7,820.7 million tons of oil equivalent (Mtoe) to 13,301.4 Mtoe, respectively. This rapidly increasing energy consumption has imposed tremendous environmental pressures on the world, particularly from the huge amount of carbon dioxide (CO2) emissions released into the atmosphere (Dong et al., 2018a). Based on statistics from BP (2020), global CO2 emissions have increased approximately 1.6 times in the past few decades from 21,331.5 million tons (Mt) in 1990 to 34,169.0 Mt in 2019. The increasing global CO2 emissions and the associated global warming have ignited worldwide concerns. Thus, many scholars have focused on the factors driving global CO2 emissions (Chebbi et al., 2011; Shahzad et al., 2017; Mahmood et al., 2019). In the meantime, a widely accepted pathway to alleviate the environmental pressure is to expand natural gas consumption, which is considered relatively cleaner, high-efficiency, and low-carbon transmit energy (Dong et al., 2019; Zhao et al., 2020a; Jiang et al., 2020). Accordingly, several scholars have studied the impact of natural gas consumption on CO2 emissions (Peters et al., 2011; Dong et al., 2017; Dong et al., 2018b). For example, Jiang et al. (2021) propose that natural gas can significantly reduce carbon abatement cost and thus curb CO2 emissions. Moreover, with rapid advances in technology, many scholars have recognized the environmentally friendly effect of technological innovation. However, to the best of our knowledge, most studies on the relationship between natural gas consumption and CO2 emissions are based on a national or regional level, and very few researches have investigated the natural gas-CO2 nexus from a global perspective. Furthermore, prior literature has often examined the impacts of natural gas consumption and technological innovation on CO2 emissions respectively. For example, some scholars investigate the impact of natural gas consumption on CO2 emissions, but not considering the effect of technological innovation in the nexus (Alkhathlan and Javid, 2013; Saboori and Sulaiman, 2013; Dong et al., 2018d). In addition, there are some studies that only focus on the nexus between technological innovation on CO2 emissions which ignore the impact of natural gas consumption on CO2 emissions (York et al., 2003; Irandoust, 2016; Yii and Geetha, 2017). However, to the best of our knowledge, very few studies have systematically investigated the nexus between natural gas consumption, CO2 emission, and technological innovation under a unified framework.
Furthermore, with rapid globalization, countries around the world are becoming more closely linked, in both international trade and environmental governance. Recently, on November 15, 2020, 15 member countries covering the ten Association of Southeast Asian Nations (ASEAN) countries and China, Japan, South Korea, Australia, and New Zealand formally signed the Regional Comprehensive Economic Partnership (RCEP) agreement, thus forming the world’s largest Free Trade Agreement (FTA). The agreement covers the largest participating population in the world, the most diverse membership structure, and the largest development potential. The signing of the RCEP will not only reshape the rules of economics and trade in the Asia-Pacific region, but will also have spillover effects on the construction of a new energy system in the Asia-Pacific region. For example, Japan and South Korea have relatively higher energy efficiency and more advanced technological innovation due to their higher levels of natural gas consumption and energy dependence. Thus, the technology spillover effect among the countries which sign the RCEP agreement might be stronger. Furthermore, most RCEP countries have relatively higher environmental regulations, which might influence the development of domestic natural gas market reforms and air pollution. These factors might cause a structural shock in the nexus between natural gas consumption, CO2 emissions, and technological innovation. However, the existing literature that further discuss the regional heterogeneity mainly focuses on the income level of countries or geographical positions. For example, Dong et al. (2020a) investigate the relationship between renewable energy consumption and global CO2 emissions based on countries with different income levels. Thus, to the best of our knowledge, few studies have investigated the potential heterogeneity in the nexus between natural gas, CO2 emissions, and technological innovation between the RCEP countries and non-RCEP countries.
To fill the academic gaps discussed above, this study first investigates the impact of natural gas consumption on global CO2 emissions by employing a balanced panel dataset for 73 countries covering the period 1990–2019. Then we systematically conduct an empirical analysis of the role of technological innovation in natural gas-CO2 emissions. Furthermore, to detect whether heterogeneity exists in the nexus between natural gas consumption, CO2 emissions, and technological innovation, this study divides the full panel data into two subsamples, the RCEP countries and the non-RCEP countries, and conducts a comparative analysis based on the fixed effect (FE) and random effect (RE) estimation methods. Accordingly, this study contributes to the existing literature in the following three aspects: 1) This study systematically investigates the nexus between natural gas consumption, global CO2 emissions, and technological innovation by putting the three factors into a unified framework. On the one hand, the impact of the natural gas consumption and technological innovation on global CO2 emissions are investigated from the respective coefficient in the empirical framework; on the other hand, the cross impact of the technological innovation and natural gas consumption is examined. The estimation results are not only conducive to better understanding the impact of natural gas consumption and technological innovation on CO2 emissions, but are also beneficial for policymakers to formulate precise policies to mitigate environmental degradation; 2) this study divides the full panel into two groups (i.e., the RCEP countries and the non-RCEP countries) for a heterogeneous analysis by conducting Dumitrescu-Hurlin (D-H) panel causality test among the two different subsamples. The results provide new evidence for policymakers to implement specific policies that are conducive to carbon reduction; and 3) this study also examines the environmental Kuznets curve (EKC) hypothesis by introducing the quadratic term of economic growth into the empirical model, and the estimation results provide a reference for policymakers to formulate policies that promote green recovery in the post-epidemic era.
The remainder of this study is organized as follows. Literature Review and Research Gap presents the existing relevant literature. Empirical Model and Data presents the empirical model and data. Estimation Strategy provides the estimation strategy. Empirical Results reports the empirical estimation results. Conclusion and Policy Implications concludes this study and provides some implications.
In recent years, a growing body of scholars has focused on the relationship between natural gas and CO2 emissions. For instance, Lotfalipour et al. (2010) explore the causal relationship between natural gas consumption and carbon emissions in Iran and find a unidirectional causality running from natural gas consumption to CO2 emissions. Similar results are reported by Pereira and Pereira (2010), who investigate the nexus of natural gas consumption and CO2 emissions in Portugal. However, some researchers, such as Alkhathlan and Javid (2013) for Saudi Arabia, Dong et al. (2018d) for 14 Asia-Pacific countries, and Saboori and Sulaiman (2013) for Malaysia, uncover a bidirectional causality between natural gas consumption and CO2 emissions. Furthermore, Shearer et al. (2014) investigate the impact of natural gas consumption on CO2 emissions in the United States, and find that increased natural gas consumption could induce more CO2 emissions and thus delay the process of decarbonization. They also claim that the promoting effect of natural gas consumption on CO2 emissions is attributed to it delaying deployment of renewable energy technologies. However, some other researchers obtain the opposite conclusion, which indicates natural gas can reduce CO2 emissions. For example, based on the data for China for the period 1965–2016, Dong et al. (2018b) find a significant mitigating effect of natural gas consumption on CO2 emissions. They insist that natural gas is a relatively cleaner transmit fuel, which can curb CO2 emissions effectively. However, they also propose that in the long run, the mitigation effect of CO2 emissions will shrink because it is composed primarily of methane which would produce substantial CO2 emissions. Their findings are confirmed by Dong et al. (2017) for BRIC countries, Su et al. (2017) for Singapore, and Xie (2014) for China. Thus, to date, the relationship between natural gas consumption and CO2 emissions is still controversial among scholars.
The impact of technological innovation on CO2 emissions has attracted the attention of numerous researchers in the past few decades. Most scholars believe technological innovation is conducive to reducing CO2 emissions. For example, by using the VECM and TYDL granger causality tests, Yii and Geetha (2017) find a negative correlation between technological innovation and CO2 emissions. Based on the STIRPAT model, York et al. (2003) have explored the influencing factors of CO2 emissions and conclude that technological innovation can significantly reduce CO2 emissions. Their results are consistent with Irandoust (2016), Zhao et al. (2010), and Zhao et al. (2013), who investigate the relationship between technological innovation and CO2 emissions based on the LMDI, ARDL, and VAR models, respectively. However, Wang et al. (2017a) propose that the impact of technological innovation on CO2 emissions varies according to different levels of economic development. Specifically, technological innovation could reduce CO2 emissions under high economic development but increase CO2 emissions under low economic development. Their findings are supported by Chen and Lee (2020) for 96 countries and Cheng et al. (2019) for OECD countries. Based on the literature review above, the existing studies on the relationship between technological innovation and CO2 emissions are mainly based on time series data, which cannot capture the individual effect information among countries. Thus, in this study, we employ the commonly used estimation methods, (i.e. FE and RE) that are appropriate for panel data to investigate the impact of technological innovation on global CO2 emissions.
In addition to the above factors, (i.e. natural gas consumption and technological innovation), some other variables are frequently presented as influencing factors on CO2 emissions. These factors include mainly economic growth, industrial structure upgrading, population, trade openness, and urbanization level. Specifically, many scholars have widely investigated the EKC hypothesis proposed by Grossman and Krueger (1993) (Iwata et al., 2012; Baek, 2015; Bilgili et al., 2016; Zhang et al., 2017; Dong et al., 2018c). This hypothesis depicts an inverted U-shaped relationship between economic growth and CO2 emissions. Specifically, according to the EKC hypothesis, further economic growth can improve environmental degradation after an economy has reached an adequate level of economic growth (Kaika and Zervas, 2013). As for industrial structure upgrading, it has been verified that industrial structure upgrading is a significant driving factor of CO2 emissions (Li et al., 2017; Chen et al., 2019; Tian et al., 2019), and most studies indicate that industrial structure upgrading can reduce CO2 emissions significantly. In terms of population, Dong et al. (2018a) and Li et al. (2017) propose that population can significantly contribute to CO2 emissions, a proposition supported by Dong et al. (2020b), Ghazali and Ali (2019), and Wang et al. (2019). In contrast, Wang et al. (2017b) oppose the above arguments and reveal a negative correlation between population size and CO2 emissions. Furthermore, many researchers have examined the impact of trade openness on CO2 emissions. For example, Ahmed et al. (2017), Ansari et al. (2020), and Bernard and Mandal (2016) find that trade openness has a positive impact on CO2 emissions. However, based on a dataset for OECD countries covering the period 1960–2013, Gozgor (2017) reveals that trade openness affects CO2 emissions negatively in the long run. This mitigation effect is evident in many other studies such as that by Ho and Iyke (2019) for central and eastern European countries and that by Lv and Xu (2019) for middle-income countries. Many other scholars have also attested to the significant impact of urbanization level on CO2 emissions (Martínez-Zarzoso and Maruotti, 2011; Zhang and Lin, 2012; Sadorsky, 2014; Shahbaz et al., 2016; Liu and Bae, 2018). Additionally, some other factors are considered to be the driving forces of CO2 emissions, such as foreign direct investment (FDI) (Omri et al., 2014; Bakhsh et al., 2017; Haug and Ucal, 2019), industrialization (Li and Lin, 2015; Xu and Lin, 2015; Liu and Bae, 2018), and lifestyle change (Wei et al., 2007; Feng et al., 2009; Li et al., 2019). Notably, these factors are not taken into the empirical model in this study.
The preceding studies have investigated the impact of natural gas consumption on CO2 emissions. However, certain research gaps still exist. First, most studies over the natural gas-CO2 emissions nexus are based on the national or regional level. To the best of our knowledge, very few researches have systematically investigated the relationship between natural gas consumption and CO2 emissions from a global perspective, which could provide more generalized evidence for policymakers to formulate appropriate policies to reduce CO2 emissions. Second, the existing literature often ignores heterogeneity in the natural gas-CO2 emissions nexus. Based on the literature review, the studies that consider the heterogeneity in the nexus between natural gas consumption and CO2 emissions mainly focus on the income levels or the geographical positions of countries. However, very few studies have investigated the difference in the impact of natural gas consumption on CO2 emissions between the RCEP countries and the non-RCEP countries. Third, it is evident that most studies over the nexus between natural gas consumption, technological innovation, and CO2 emissions investigate the impact of natural gas consumption and technological innovation on CO2 emissions respectively. And to the best of our knowledge, very few studies have examined the nexus between the three variables in a unified framework. Furthermore, few scholars have explored the impact of technological innovation on the natural gas-CO2 emissions nexus, which is not conducive to clearly analyzing the causal relationships between natural gas consumption, CO2 emissions, and technological innovation.
To empirically explore the causal natural gas-CO2 nexus, this study examines the impact of natural gas consumption and technological innovation on global CO2 emissions based on the static panel model. Based on the conventional STIRPAT model reformulated by Dietz and Rosa (1997), we construct an econometric model as follows: CO2 emissions are utilized as the dependent variable, while natural gas consumption and technological innovation are used as the main independent variables. Following the discussion in Studies on the Determinants of CO2Emissions, this study further introduces economic growth, industrial structure upgrading, trade openness, population, and urbanization level as control variables. Furthermore, to verify the EKC hypothesis of global CO2 emissions, this study also introduces the square term of economic growth. The multivariate framework is presented as follows:
where subscripts i and t represent the country and year, respectively. CO2 indicates the CO2 emissions of various countries, NGC denotes natural gas consumption, Tec refers to technological innovation, Pgdp means economic growth, Ind represents industrial structure upgrading, Pop indicates the population of each country, Tra refers to trade openness, and Urb represents the urbanization level.
To eliminate the effect of variable dimension as well as the potential heteroscedasticity problem of the data series, this study takes the natural logarithm of all variables in Eq. 1, which is shown as follows:
where
In addition, to systematically explore the influence of technological innovation on the impact of natural gas consumption in global CO2 emissions, we further introduce the interaction term of technological innovation and natural gas consumption on the basis of Eq. 2; thus, Eq. 2 can be rewritten as follows:
where
In this study, a balanced panel dataset covering 73 countries for the period 1990–2019 is utilized to investigate the impact of natural gas consumption and technological innovation on global CO2 emissions, yielding a total of 2,190 observations. Notably, due to data limitations, other countries are not considered. Furthermore, with the signing of the RCEP, the world’s largest free trade agreement, it is important to explore the differences in the impact of natural gas consumption and technological innovation on CO2 emissions between RCEP and non-RCEP countries. Thus, the 73 countries are divided into RCEP countries (12 countries) and non-RCEP countries (61 countries); the specific countries are highlighted in Table A1 in the Appendix.
The data on CO2 emissions (denoted as CO2) and natural gas consumption (denoted as NGC) of each country are obtained from the BP Statistical Review of World Energy (BP, 2020). Technological innovation (denoted as Tec) is measured by the ratio of GDP to energy consumption, where the data on energy consumption are from BP (2020), while the data on GDP are obtained from the World Bank. (2020). Economic growth (denoted as Pgdp) is measured by per capita GDP, industrial structure upgrading (denoted as Ind) is measured by the ratio of the added value of tertiary industry to secondary industry, population (denoted as Pop) is measured by the population of each country, trade openness (denoted as Tra) is measured by the ratio of total import and export trade to GDP; that is, trade dependence; and urbanization level (denoted as Urb) is measured by the proportion of the urban population to total population. The data on economic growth, industrial structure upgrading, population, trade openness, and urbanization level are collected from the World Bank. (2020). The description of the variables is presented in Table 1, while the descriptive statistics (i.e., mean value, standard deviation, maximum value, and minimum value) of all the selected variables are shown in Table 2.
Technically, the estimation strategies in this study consist mainly of four steps: 1) Both the Breusch-Pagan Lagrange multiplier (LM) test and Pesaran cross-section dependence (CD) test are utilized to examine cross-sectional dependence within the panel data (see Cross-Sectional Dependence Tests); 2) the Pesaran cross-sectionally augmented Dickey-Fuller (CADF) and cross-sectionally augmented Im, Pesaran, and Shin (CIPS) panel unit root tests are utilized to examine the stationarity of each variable (see Panel CADF and CIPS Unit Root Tests); 3) the impacts of natural gas consumption and technological innovation on global CO2 emissions are investigated by employing the FE and RE estimation methods simultaneously (see FE and RE Estimates); and 4) the causal nexus between the three main variables is explored by the Dumitrescu-Hurlin (D-H) panel causality test (see Dumitrescu–Hurlin Panel Causality Test).
To the best of our knowledge, the panel data used in this study may have the problem of potential cross-sectional dependence, which can cause inconsistent and invalid estimates (Grossman and Krueger, 1995; Zhao et al., 2020b). Moreover, in the current era of economic integration, complete independence is almost impossible. To effectively achieve the targeted objectives, this study first conducts cross-sectional dependence tests within the panel data.
The three most commonly used cross-sectional dependence test methods include the Breusch-Pagan LM test, the Pesaran scaled LM test, and the Pesaran CD test. By comparing the advantages and disadvantages of these three methods, this study employs the Breusch-Pagan LM test and the Pesaran CD developed by Breusch and Pagan (1980) and Pesaran (2004), respectively, to conduct cross-sectional dependence tests; the specific statistics are expressed in Eqs 4Eqs 5, respectively.
Notably, the two stochastic variables described above are distributed as standard normal under the null hypothesis when
Following the previous discussion, this study employs an updated panel unit root test that incorporates cross-sectional dependence. Notably, the first-generation panel unit root test is invalid when cross-sectional dependence exists within the panel data. Accordingly, this study selects the Pesaran CIPS test proposed by Pesaran (2007) for the panel stationarity test. The test statistic for the Pesaran CIPS is presented as follows:
where
Here, CADFi is the t-statistics in the CADF regression defined by Eq. 7.
Considering the potential existence of cross-sectional dependence in the panel data, this study employs the FE and RE estimation methods. Moreover, the FE and RE estimation methods could avoid the problem of omitted variables by controlling the individual effect in the panel data. Specifically, the FE model is appropriate for the conditions under which the individual factors are correlated with certain independent variables, while the RE model applies to the situation where the individual factors are unrelated to all the independent variables. Furthermore, to distinguish which estimation method is suitable for the panel data, the specification test principle proposed by Hausman (1978), namely the Hausman test, is utilized in this study. According to Baltagi (2006), when analyzing an empirical model using panel data, Hausman test is a usual and effective tool to select appropriate estimation method. This viewpoint is shared by many other scholars (Baltagi et al., 2003; Frondel and Vance, 2010; Amini et al., 2012). Thus, employing the Hausman test for model specification is valid in this study. And the null hypothesis of the Hausman test is non-correlation between individual factors and the independent variables, which indicates the RE estimation is more effective for the panel data. Additionally, to examine whether heterogeneity exists in the impact of natural gas consumption on CO2 emissions, this study further divides the full sample into two subsamples, the RCEP countries and the non-RCEP countries, and makes a comparative analysis by estimating the natural gas-CO2 emissions nexus in the two subsamples, respectively.
Exploring the causality direction between natural gas consumption, technological innovation, and CO2 emissions is particularly useful for policymakers to formulate specific policies. Considering cross-sectional dependence, this study employs the D-H panel causality test proposed by Dumitrescu and Hurlin (2012) to investigate the causal nexus between the variables. This test can be conducted irrespective of T > N or N > T and also deals with heterogeneity in the slope coefficients and cross-sectional dependence (Khan et al., 2020).
The results of the cross-sectional dependence tests for the full panel data and the two subsamples are all reported in Table 3. From the table, the statistics for both the Breusch-Pagan LM tests and the Pesaran CD tests are all higher than the critical values at the 1% significance level, which means the null hypotheses, (i.e. no cross-sectional dependence) are all rejected for the full panel and the groups of RCEP and non-RCEP countries. Accordingly, the existence of cross-sectional dependence is valid and should be considered in the following estimation procedures.
Considering the existence of cross-sectional dependence in the full panel and the two subsamples, the panel CADF and CIPS unit root tests are utilized to examine the stability of the relevant series; the results are listed in Table 4. As the table shows, for the global panel sample, the series data are not all stable at the level, whereas the first-differentiated variables are all stable due to the significant statistics of the two panel unit root tests. This indicates that the selected variables in the global panel are integrated of order, [i.e. I (1)], which ensures stable estimations of the empirical model. Similarly, with respect to the data for RCEP countries, all the selected variables are all integrated of order and thus valid for model construction and empirical analysis. The same test results are evident for the variables of the non-RCEP countries. To conclude, the linear empirical model is valid in the full panel and the two subsamples, respectively, due to the same order of integration of the selected variables in all samples.
Following the discussions above, this study estimates the natural gas-CO2 nexus for the global panel, and the results are displayed in Table 5. Furthermore, to explore the impact of technological innovation on the natural gas-CO2 nexus, we also re-estimate the empirical model by introducing the cross term of natural gas consumption and technological innovation into the empirical framework. The results of the FE and RE estimations are listed in the second and fourth columns, respectively. Also, the results of the Hausman tests are reported in the table. The p-value of the Hausman test statistic indicates that the null hypothesis, (i.e. no correlation between the individual effect and independent variables) is rejected. Thus, the FE estimates are considered as the benchmark results of the empirical model for the global panel. Notably, the results of the RE estimates are basically consistent with those of the FE estimates, which imply that the estimation results for the global panel are robust and reliable.
As for the natural gas-CO2 nexus, it is evident that to date, natural gas consumption has a significant positive impact on global CO2 emissions. Although natural gas is relatively cleaner transition energy, compared with other traditional energy, its utilization is still accompanied by increasing CO2 emissions. Thus, increased natural gas consumption is not conducive to carbon reduction. However, when the impact of technological innovation on natural gas-CO2 emissions is considered, that is, the cross term of natural gas consumption and technological innovation is introduced into the empirical model, the positive impact of natural gas consumption on CO2 emissions is no more significant. This means the implementation of technological innovation can offset the negative effect of natural gas consumption on CO2 emissions to some extent. Furthermore, the negative value of the coefficient of the cross term implies that the promoting effect of natural gas consumption on CO2 emission would decline as technological innovation is developed. This might be because that when technological innovation is advanced, the utilization efficiency of natural gas consumption will improve, which will greatly reduce the amount of total energy use keeping other production activities constant. Furthermore, technological innovation could optimize the process of natural gas use, thus promoting cleaner production and mitigating CO2 emissions.
As for the control variables, the coefficients of the control variables are all statistically significant, and their signs are basically in line with the actual conditions. Specifically, technological innovation can significantly reduce global CO2 emissions. This is rational because the progress of technological innovation can improve energy efficiency and thereby reduce energy use while maintaining the current production level. Furthermore, an advance in technological innovation is conducive to promoting other alternative clean energy, which is conducive to environmental improvement. Moreover, the positive coefficient of economic growth and the negative coefficient of its quadratic term provide evidence of the EKC hypothesis in the globe, which indicates global CO2 emissions initially increase and then decline after economic growth crosses a certain value, (i.e. turning point). Notably, both industrial structure upgrading and trade openness can reduce global CO2 emissions, according to their negative coefficients in the empirical model. This is because both of them can accelerate the transformation of industrial structure from a carbon-intensive heavy industry to a low-carbon and high value-added service one. However, the positive estimated coefficients of population and urbanization level indicate positive correlations between population, urbanization level, and CO2 emissions, respectively. Specifically, population promotes more CO2 emissions mainly from two aspects: on the one hand, population growth results in more demand for energy consumption, especially for countries with low energy efficiency; on the other hand, rapid population growth may cause the destruction of forests and even change land utilization patterns, both of which are not conducive to carbon reduction. In terms of urbanization, the positive relationship between urbanization and CO2 emissions reveals that the current urbanization process is not environmentally friendly. The reason might be that urbanization gives rise to more production and the establishment of more structures and industrial units, and the expansion of production necessitates more energy consumption, which would induce more CO2 emissions.
Table 6 exhibits the estimation results for the RCEP countries and non-RCEP countries. From the table, the p-values of the Hausman test statistics for the two subsamples reject the null hypothesis of the Hausman tests at the 1 and 5% significance levels, respectively. Thus, similar to the global panel above, the FE model is preferred as the benchmark estimation for both the RCEP and non-RCEP countries.
As for the RCEP countries, the coefficient of natural gas consumption is not significant, which indicates that increased natural gas consumption would not contribute to CO2 emissions. This might be because several RCEP countries, (e.g. Japan, South Korea, and Singapore) have relatively foreign energy dependence, and this promotes these countries to have relatively higher technology levels. Furthermore, under the Kyoto Protocol and the Paris Agreement, these developed countries bear greater responsibility for reducing carbon emissions, which contributes a lot to the higher level of energy efficiency and technology development domestically. Thus, the signing of the RCEP would expand the technology spillovers among the relevant countries, which would neutralize the supposed positive effects of natural gas consumption on CO2 emissions. However, after introducing the cross term of natural gas consumption and technological innovation into the empirical model, we find that natural gas consumption can significantly reduce CO2 emissions, which further confirms the reinforcement effect of technology spillovers in the RCEP countries. It is also noteworthy that trade openness has a positive impact on CO2 emissions in the RCEP countries. Thus, the signing of the RCEP would change the negative correlation between trade openness and CO2 emissions. This may be because the signing of the RCEP would expand the volume of international trade of the relevant countries, thereby increasing production in a country, which would inevitably induce more energy consumption and CO2 emissions. Therefore, at least in the short term, trade openness would contribute to CO2 emissions in the RCEP countries, and this provides evidence for integrated environmental governance in the post-RCEP era. Additionally, the estimation results of other control variables are consistent with those for the global panel, which also coincides with the actual conditions of the RCEP countries.
With respect to the non-RCEP countries, the significance and signs of the estimated coefficients are similar to the estimation results for the full panel data. This may be because most global countries are different from the countries that sign the RCEP agreement. Thus, the estimation results for the non-RCEP countries are similar to that for the global panel, which state the general rule over the nexus between natural gas consumption, technological innovation, and CO2 emissions. To conclude, the impact of natural gas consumption and technological innovation on CO2 emissions is different between the RCEP and non-RCEP countries, which indicates significant heterogeneity in the natural gas-CO2 nexus. Therefore, policymakers should formulate specific policies to reduce CO2 emissions.
The results of the D-H panel causality test across different regions are displayed in Table 7. To clearly show the causality flows between natural gas consumption, technological innovation, and CO2 emissions, this study also depicts the causality movements in Figure 1. As the figure shows, bidirectional causality runs between any two of the above three variables for the global panel data and the non-RCEP countries. However, for the RCEP countries, unidirectional causality runs only from technological innovation to CO2 emissions, natural gas consumption to CO2 emissions, and technological innovation to natural gas consumption, respectively.
On the one hand, the varying causal relationships between natural gas consumption, technological innovation, and CO2 emissions again verifies the existence of heterogeneity in the natural gas-CO2 nexus. On the other hand, policymakers should take the heterogeneous impact of natural gas consumption on CO2 emissions into consideration and formulate specific policies to improve environment quality. For example, the technological spillover effects are reinforced in the RCEP countries, which provides evidence for policymakers to formulate policies to promote the development of technological innovation in the RCEP countries. Simultaneously, the positive correlation between trade openness and CO2 emissions indicates that at least in the short term, trade openness would contribute to CO2 emissions in the RCEP countries, which also suggests integrated environmental governance in the post-RCEP era.
To investigate the impact of natural gas consumption on global CO2 emissions and explore the role of technological innovation in the natural gas-CO2 nexus, this study employs a balanced panel dataset for 73 countries for the period 1990–2019 for empirical analysis. Considering the cross-sectional dependence in the panel data, this study utilizes the FE and RE methods in the process of estimation. Furthermore, to examine heterogeneity in the nexus between natural gas consumption, technological innovation, and CO2 emissions, we also conduct an empirical analysis for the RCEP countries and non-RCEP countries, respectively. This study makes several interesting findings, as follows:
First, natural gas consumption has a significant positive impact on global CO2 emissions. However, technological innovation could reduce or even neutralize the environmental degradation effect of natural gas consumption to some extent. Specifically, the marginal impact of natural gas consumption on CO2 emissions would decline with a higher level of technological innovation.
Second, this study verifies the EKC hypothesis between economic growth and global CO2 emissions, by indicating an inverted U-shaped relationship between economic growth and global CO2 emissions. That is to say, global CO2 emissions increase initially and then decline after economic growth crosses a certain value, (i.e. turning point).
Third, heterogeneity exists in the natural gas-CO2 emissions nexus between the RCEP countries and non-RCEP countries. The technological spillover effects are reinforced in the RCEP countries, which provides evidence for policymakers to formulate specific policies in a certain country. However, the positive correlation between trade openness and CO2 emissions indicates that at least in the short term, trade openness would contribute to CO2 emissions in the RCEP countries, which also suggests integrated environmental governance in the post-RCEP era.
Based on the above findings, several policy implications are highlighted as follows:
First, it is necessary to promote technological innovation, not only for its direct negative impact on global CO2 emissions, but also for its spillover effect on the natural gas-CO2 nexus. Thus, the governments should encourage enterprises to improve their production process, especially for cleaner technology advancement. For example, it is advisable to provide targeted subsides or tax reductions for technology research and development.
Second, given that the EKC hypothesis is valid in the globe, countries should continue to accelerate their economic growth and promote their economic structure into a low-carbon development mode. However, the COVID-19 epidemic which broke out at the end of 2019 has caused great damage to the world economy. Given that both technological innovation and trade openness can significantly reduce global CO2 emissions, the technology trade deserves special attention to advance productivities for a post-epidemic “green recovery.” For example, the global policymakers should encourage the development of technology trade among countries. Some effective measures include reducing the tariff to lower technology trade barriers, providing subsidies to encourage more technology trade. Furthermore, countries should strengthen environmental protection experience communication and cooperation, especially in the post-epidemic era where countries are closely linked with each other.
Third, considering that the technological spillover effects in the natural gas-CO2 nexus are more reinforced in the RCEP countries, the relevant countries should attach more importance to technological innovation in the process of carbon reduction. Furthermore, given that trade openness would contribute to CO2 emissions in the RCEP countries, the relevant countries should restrict the production of high-carbon products to guide the market and promote the development of high value-added and low-carbon industries, thereby realizing the transformation of trade structure to a low-carbon mode.
However, it is noteworthy that this study provides only preliminary empirical evidence on the natural gas-CO2 emissions nexus, and some limitations still exist. One limitation is related to the specific impact mechanism between natural gas consumption and CO2 emissions. In addition to the heterogeneous effects, in future research, we also can explore the mediating effect mechanism between natural gas consumption and global CO2 emissions. Furthermore, it would be interesting to add more independent variables and estimate their potential impacts on global CO2 emissions, such as foreign direct investment, industrialization, lifestyle change and so on; however, they are not considered in this study due to data missing in some countries. Additionally, since the RCEP agreement is signed not long ago, the data do not cover the period after the signing of the RCEP agreement. Thus, in future research, it will be interesting to employ a dataset that covers more updated data to examine whether the signing of the RCEP agreement can influence the nexus between natural gas consumption, technological innovation, and CO2 emissions.
The original contributions presented in the study are included in the article/Supplementary Material, further inquiries can be directed to the corresponding author.
YD:Conceptualization, Methodology, Validation, Investiga- tion, Writing - original draft, Writing—review and editing. JZ:Datacuration,Methodology,Software. JD:Conceptualization, Writing—review and editing.
The article is supported by the National Social Science Foundation of China (Grant No. 18VDL017) and the Innovation Methods Special Foundation of the Chinese Ministry of Science and Technology (Grant No. 2018IMO40100). Certainly, all remaining errors are our own.
The author declares that the research was conducted in the absence of any commercial or financial relationships that could be construed as a potential conflict of interest.
Ahmed, K., Rehman, M. U., and Ozturk, I. (2017). What drives carbon dioxide emissions in the long-run? Evidence from selected South Asian Countries. Renew. Sust. Energ. Rev. 70, 1142–1153. doi:10.1016/j.rser.2016.12.018
Alkhathlan, K., and Javid, M. (2013). Energy consumption, carbon emissions and economic growth in Saudi Arabia: an aggregate and disaggregate analysis. Energ. Policy 62, 1525–1532. doi:10.1016/j.enpol.2013.07.068
Amini, S., Delgado, M. S., Henderson, D. J., and Parmeter, C. F. (2012). Fixed vs random: the Hausman test four decades later. Essays in honor of Jerry Hausman. Bingley, United Kiingdom: Emerald Group Publishing Limited. doi:10.1108/S0731-9053(2012)0000029021
Ansari, M. A., Haider, S., and Khan, N. A. (2020). Does trade openness affects global carbon dioxide emissions: evidence from the top CO2 emitters. Manag. Environ. Qual. 31, 32–53. doi:10.1108/MEQ-12-2018-0205
Baek, J. (2015). A panel cointegration analysis of CO2 emissions, nuclear energy and income in major nuclear generating countries. Appl. Energy 145, 133–138. doi:10.1016/j.apenergy.2015.01.074
Bakhsh, K., Rose, S., Ali, M. F., Ahmad, N., and Shahbaz, M. (2017). Economic growth, CO2 emissions, renewable waste and FDI relation in Pakistan: new evidences from 3SLS. J. Environ. Manage. 196, 627–632. doi:10.1016/j.jenvman.2017.03.029
Baltagi, B. H., Bresson, G., and Pirotte, A. (2003). Fixed effects, random effects or Hausman–Taylor? A pretest estimator. Econ. Lett. 79, 361–369. doi:10.1016/S0165-1765(03)00007-7
Baltagi, B. H. (2006). Estimating an economic model of crime using panel data from North Carolina. J. Appl. Econom. 21, 543–547. doi:10.1002/jae.861
Bernard, J., and Mandal, S. K. (2016). The impact of trade openness on environmental quality: an empirical analysis of emerging and developing economies. WIT Trans. Ecol. Environ. 203, 195–208. doi:10.2495/EID160181
Bilgili, F., Kocak, E., and Bulut, Ü. (2016). The dynamic impact of renewable energy consumption on CO2 emissions: a revisited Environmental Kuznets Curve approach. Renew. Sust. Energ. Rev. 54, 838–845. doi:10.1016/j.rser.2015.10.080
BP (2020). BP statistical review of world energy 2020 . Available at: http://www.bp.com/en/global/corporate/energy-economics/statistical-review-of-world-energy/downloads.html
Breusch, T. S., and Pagan, A. R. (1980). The Lagrange multiplier test and its applications to model specification in econometrics. Rev. Econ. Stud. 47, 239–253. doi:10.2307/2297111
Chebbi, H. E., Olarreaga, M., and Zitouna, H. (2011). Trade openness and CO2 emissions in Tunisia. Middle East. Dev. J. 3, 29–53. doi:10.1142/S1793812011000314
Chen, J., Yuan, H., Tian, X., Zhang, Y., and Shi, F. (2019). What determines the diversity of CO2 emission patterns in the Beijing-Tianjin-Hebei region of China? An analysis focusing on industrial structure change. J. Clean. Prod. 228, 1088–1098. doi:10.1016/j.jclepro.2019.04.267
Chen, Y., and Lee, C. C. (2020). Does technological innovation reduce CO2 emissions? Cross-country evidence. J. Clean. Prod. 121550. doi:10.1016/j.jclepro.2020.121550
Cheng, C., Ren, X., and Wang, Z. (2019). The impact of renewable energy and innovation on carbon emission: an empirical analysis for OECD countries. Energ. Proced. 158, 3506–3512. doi:10.1016/j.egypro.2019.01.919
Dietz, T., and Rosa, E. A. (1997). Effects of population and affluence on CO2 emissions. P. Natl. Acad. Sci. 94, 175–179. doi:10.1073/pnas.94.1.175
Dong, K., Sun, R., and Hochman, G. (2017). Do natural gas and renewable energy consumption lead to less CO2 emission? Empirical evidence from a panel of BRICS countries. Energy 141, 1466–1478. doi:10.1016/j.energy.2017.11.092
Dong, K., Hochman, G., Zhang, Y., Sun, R., Li, H., and Liao, H. (2018a). CO2 emissions, economic and population growth, and renewable energy: empirical evidence across regions. Energ. Econ. 75, 180–192. doi:10.1016/j.eneco.2018.08.017
Dong, K., Sun, R., and Dong, X. (2018b). CO2 emissions, natural gas and renewables, economic growth: assessing the evidence from China. Sci. Total Environ. 640-641, 293–302. doi:10.1016/j.scitotenv.2018.05.322
Dong, K., Sun, R., Jiang, H., and Zeng, X. (2018c). CO2 emissions, economic growth, and the environmental Kuznets curve in China: what roles can nuclear energy and renewable energy play? J. Clean. Prod. 196, 51–63. doi:10.1016/j.jclepro.2018.05.271
Dong, K., Sun, R., Li, H., and Liao, H. (2018d). Does natural gas consumption mitigate CO2 emissions: testing the environmental Kuznets curve hypothesis for 14 Asia-Pacific countries. Renew. Sust. Energ. Rev. 94, 419–429. doi:10.1016/j.rser.2018.06.026
Dong, K., Dong, X., and Sun, R. (2019). How did the price and income elasticities of natural gas demand in China evolve from 1999 to 2015? The role of natural gas price reform. Petrol. Sci. 16, 685–700. doi:10.1007/s12182-019-0320-z
Dong, K., Dong, X., and Jiang, Q. (2020a). How renewable energy consumption lower global CO2 emissions? Evidence from countries with different income levels. World Econ. 43, 1665–1698. doi:10.1111/twec.12898
Dong, K., Dong, X., and Ren, X. (2020b). Can expanding natural gas infrastructure mitigate CO2 emissions? Analysis of heterogeneous and mediation effects for China. Energ. Econ. 90, 104830. doi:10.1016/j.eneco.2020.104830
Dumitrescu, E. I., and Hurlin, C. (2012). Testing for Granger non-causality in heterogeneous panels. Econ. Model. 29, 1450–1460. doi:10.1016/j.econmod.2012.02.014
Feng, K., Hubacek, K., and Guan, D. (2009). Lifestyles, technology and CO2 emissions in China: a regional comparative analysis. Ecol. Econ. 69, 145–154. doi:10.1016/j.ecolecon.2009.08.007
Frondel, M., and Vance, C. (2010). Fixed, random, or something in between? A variant of Hausman's specification test for panel data estimators. Econ. Lett. 107, 327–329. doi:10.1016/j.econlet.2010.02.007
Ghazali, A., and Ali, G. (2019). Investigation of key contributors of CO2 emissions in extended STIRPAT model for newly industrialized countries: a dynamic common correlated estimator (DCCE) approach. Energ. Rep. 5, 242–252. doi:10.1016/j.egyr.2019.02.006
Gozgor, G. (2017). Does trade matter for carbon emissions in OECD countries? Evidence from a new trade openness measure. Environ. Sci. Pollut. R. 24, 27813–27821. doi:10.1007/s11356-017-0361-z
Grossman, G. M., and Krueger, A. B. (1995). Economic growth and the environment. The Quarterly Journal of Economics 110, 353–377. doi:10.2307/2118443
Grossman, G. M., and Krueger, A. B. (1993). “Environmental impacts of A North American free trade agreement,” in The US-Mexico free trade agreement. Editor P. Barber (Cambridge, MA, United States: MIT Press). doi:10.3386/w3914
Haug, A., and Ucal, M. (2019). The role of trade and FDI for CO2 emissions in Turkey: Nonlinear relationships. Energ. Econ. 81, 297–307. doi:10.1016/j.eneco.2019.04.006
Hausman, J. A. (1978). Specification tests in econometrics. Econometrica 46, 1251–1272. doi:10.2307/1913827
Ho, S., and Iyke, B. (2019). Trade openness and carbon emissions: evidence from central and eastern European countries. Rev. Econ. 70, 41–67. doi:10.1515/roe-2018-0001
Irandoust, M. (2016). The renewable energy-growth nexus with carbon emissions and technological innovation: evidence from the Nordic countries. Ecol. Indicat. 69, 118–125. doi:10.1016/j.ecolind.2016.03.051
Iwata, H., Okada, K., and Samreth, S. (2012). Empirical study on the determinants of CO2 emissions: evidence from OECD countries. Appl. Econ. 44, 3513–3519. doi:10.1080/00036846.2011.577023
Jiang, H., Dong, X., Jiang, Q., and Dong, K. (2020). What drives China's natural gas consumption? Analysis of national and regional estimates. Energ. Econ. 87, 104744. doi:10.1016/j.eneco.2020.104744
Jiang, H. D., Xue, M. M., Dong, K. Y., and Liang, Q. M. (2021). How will natural gas market reforms affect carbon marginal abatement costs? Evidence from China. Econ. Syst. Res. 33, 1–22. doi:10.1080/09535314.2020.1868410
Kaika, D., and Zervas, E. (2013). The Environmental Kuznets Curve (EKC) theory—Part A: concept, causes and the CO2 emissions case. Energ. Pol. 62, 1392–1402. doi:10.1016/j.enpol.2013.07.131
Khan, Z., Ali, S., Umar, M., Kirikkaleli, D., and Jiao, Z. (2020). Consumption-based carbon emissions and international trade in G7 countries: the role of environmental innovation and renewable energy. Sci. Total Environ. 730, 138945. doi:10.1016/j.scitotenv.2020.138945
Li, J., Zhang, D., and Su, B. (2019). The impact of social awareness and lifestyles on household carbon emissions in China. Ecol. Econ. 160, 145–155. doi:10.1016/j.ecolecon.2019.02.020
Li, K., and Lin, B. (2015). Impacts of urbanization and industrialization on energy consumption/CO2 emissions: does the level of development matter?. Renew. Sust. Energ. Rev. 52, 1107–1122. doi:10.1016/j.rser.2015.07.185
Li, W., Wang, W., Wang, Y., and Qin, Y. (2017). Industrial structure, technological progress and CO2 emissions in China: analysis based on the STIRPAT framework. Nat. Hazards 88, 1545–1564. doi:10.1007/s11069-017-2932-1
Liu, X., and Bae, J. (2018). Urbanization and industrialization impact of CO2 emissions in China. J. Clean. Prod. 172, 178–186. doi:10.1016/j.jclepro.2017.10.156
Lotfalipour, M. R., Falahi, M. A., and Ashena, M. (2010). Economic growth, CO2 emissions, and fossil fuels consumption in Iran. Energy 35, 5115–5120. doi:10.1016/j.energy.2010.08.004
Lv, Z., and Xu, T. (2019). Trade openness, urbanization and CO2 emissions: dynamic panel data analysis of middle-income countries. J. Int. Trade. Econ. Dev. 28, 317–330. doi:10.1080/09638199.2018.1534878
Mahmood, H., Maalel, N., and Zarrad, O. (2019). Trade openness and CO2 emissions: evidence from Tunisia. Sustainability-Basel 11, 3295. doi:10.3390/su11123295
Martínez-Zarzoso, I., and Maruotti, A. (2011). The impact of urbanization on CO2 emissions: evidence from developing countries. Ecol. Econ. 70, 1344–1353. doi:10.1016/j.ecolecon.2011.02.009
Omri, A., Nguyen, D. K., and Rault, C. (2014). Causal interactions between CO2 emissions, FDI, and economic growth: evidence from dynamic simultaneous-equation models. Econ. Model. 42, 382–389. doi:10.1016/j.econmod.2014.07.026
Pereira, A. M., and Pereira, R. M. M. (2010). Is fuel-switching a no-regrets environmental policy? VAR evidence on carbon dioxide emissions, energy consumption and economic performance in Portugal. Energ. Econ. 32, 227–242. doi:10.1016/j.eneco.2009.08.002
Pesaran, M. H. (2007). A simple panel unit root test in the presence of cross‐section dependence. J. Appl. Economet. 22, 265–232. doi:10.1002/jae.951
Pesaran, M. H. (2004). General diagnostic tests for cross section dependence in panels. Cambridge working papers in economics. doi:10.17863/CAM.5113
Peters, L., Hussain, A., Follmann, M., Melin, T., and Hägg, M. B. (2011). CO2 removal from natural gas by employing amine absorption and membrane technology—a technical and economical analysis. Chem. Eng. J. 172, 952–960. doi:10.1016/j.cej.2011.07.007
Saboori, B., and Sulaiman, J. (2013). Environmental degradation, economic growth and energy consumption: evidence of the environmental Kuznets curve in Malaysia. Energ. Pol. 60, 892–905. doi:10.1016/j.enpol.2013.05.099
Sadorsky, P. (2014). The effect of urbanization on CO2 emissions in emerging economies. Energ. Econ. 41, 147–153. doi:10.1016/j.eneco.2013.11.007
Shahbaz, M., Loganathan, N., Muzaffar, A. T., Ahmed, K., and Jabran, M. A. (2016). How urbanization affects CO2 emissions in Malaysia? The application of STIRPAT model. Renew. Sust. Energ. Rev. 57, 83–93. doi:10.1016/j.rser.2015.12.096
Shahzad, S. J. H., Kumar, R. R., Zakaria, M., and Hurr, M. (2017). Carbon emission, energy consumption, trade openness and financial development in Pakistan: a revisit. Renew. Sust. Energ. Rev. 70, 185–192. doi:10.1016/j.rser.2016.11.042
Shearer, C., Bistline, J., Inman, M., and Davis, S. J. (2014). The effect of natural gas supply on US renewable energy and CO2 emissions. Environ. Res. Lett. 9, 094008. doi:10.1088/1748-9326/9/9/094008
Su, B., Ang, B. W., and Li, Y. (2017). Input-output and structural decomposition analysis of Singapore's carbon emissions. Energ. Pol. 105, 484–492. doi:10.1016/j.enpol.2017.03.027
Tian, X., Bai, F., Jia, J., Liu, Y., and Shi, F. (2019). Realizing low-carbon development in a developing and industrializing region: impacts of industrial structure change on CO2 emissions in southwest China. J. Environ. Manage. 233, 728–738. doi:10.1016/j.jenvman.2018.11.078
Wang, C. J., Wang, F., Zhang, X. L., Yang, Y., Su, Y. X., Ye, Y. Y., et al. (2017a). Examining the driving factors of energy related carbon emissions using the extended STIRPAT model based on IPAT identity in Xinjiang. Renew. Sustain. Energ. Rev. 67, 51–61. doi:10.1016/j.rser.2016.09.006
Wang, Y., Kang, Y., Wang, J., and Xu, L. (2017b). Panel estimation for the impacts of population-related factors on CO2 emissions: a regional analysis in China. Ecol. Indic. 78, 322–330. doi:10.1016/j.ecolind.2017.03.032
Wang, S., Wang, J., Li, S., Fang, C., and Feng, K. (2019). Socioeconomic driving forces and scenario simulation of CO2 emissions for a fast-developing region in China. J. Clean. Prod. 216, 217–229. doi:10.1016/j.jclepro.2019.01.143
Wei, Y. M., Liu, L. C., Fan, Y., and Wu, G. (2007). The impact of lifestyle on energy use and CO2 emission: an empirical analysis of China’s residents. Energ. Policy 35, 247–257. doi:10.1016/j.enpol.2005.11.020
World Bank (2020). World development indicators https://databank.worldbank.org/source/world-development-indicators/preview/on.
Xie, S. C. (2014). The driving forces of China’s energy use from 1992 to 2010: an empirical study of input–output and structural decomposition analysis. Energ. Pol. 73, 401–415. doi:10.1016/j.enpol.2014.05.035
Xu, B., and Lin, B. (2015). How industrialization and urbanization process impact on CO2 emissions in China: evidence from nonparametric additive regression models. Energ. Econ. 48, 188–202. doi:10.1016/j.eneco.2015.01.005
Yii, K. J., and Geetha, C. (2017). The nexus between technology innovation and CO2 emissions in Malaysia: evidence from granger causality test. Energ. Proced. 105, 3118–3124. doi:10.1016/j.egypro.2017.03.654
York, R., Rosa, E. A., and Dietz, T. (2003). STIRPAT, IPAT and ImPACT: analytic tools for unpacking the driving forces of environmental impacts. Ecol. Econ. 46, 351–365. doi:10.1016/S0921-8009(03)00188-5
Zhang, B., Wang, B., and Wang, Z. (2017). Role of renewable energy and non-renewable energy consumption on EKC: evidence from Pakistan. J. Clean. Prod. 156, 855–864. doi:10.1016/j.jclepro.2017.03.203
Zhang, C., and Lin, Y. (2012). Panel estimation for urbanization, energy consumption and CO2 emissions: a regional analysis in China. Energ. Pol. 49, 488–498. doi:10.1016/j.enpol.2012.06.048
Zhao, J., Jiang, Q., and Dong, K. (2020a). Income inequality and natural gas consumption in China: do heterogeneous and threshold effects exist?. Aust. Econ. Pap. 59, 1–21. doi:10.1111/1467-8454.12222
Zhao, J., Jiang, Q., Dong, X., and Dong, K. (2020b). Would environmental regulation improve the greenhouse gas benefits of natural gas use? A Chinese case study. Energ. Econ. 87, 104712. doi:10.1016/j.eneco.2020.104712
Zhao, M., Tan, L., Zhang, W. G., Ji, M. H., Liu, Y., and Yu, L. Z. (2010). Decomposing the influuencing factors of industrial carbon emissions in Shanghai using the LMDI method. Energy 35, 2505–2510. doi:10.1016/j.energy.2010.02.049
Keywords: carbon emissions, natural gas consumption, technological innovation, RCEP and non-RCEP countries, global analysis
Citation: Dou Y, Zhao J and Dong J (2021) Re-Estimating the Impact of Natural Gas on Global Carbon Emissions: The Role of Technological Innovation. Front. Energy Res. 9:651586. doi: 10.3389/fenrg.2021.651586
Received: 10 January 2021; Accepted: 03 February 2021;
Published: 23 March 2021.
Edited by:
Qi Liao, University of Lisbon, PortugalReviewed by:
Jiang Hongdian, Beijing Institute of Technology, ChinaCopyright © 2021 Dou, Zhao and Dong. This is an open-access article distributed under the terms of the Creative Commons Attribution License (CC BY). The use, distribution or reproduction in other forums is permitted, provided the original author(s) and the copyright owner(s) are credited and that the original publication in this journal is cited, in accordance with accepted academic practice. No use, distribution or reproduction is permitted which does not comply with these terms.
*Correspondence: Jiajia Dong, amQyMDIwQGJ1LmVkdQ==
Disclaimer: All claims expressed in this article are solely those of the authors and do not necessarily represent those of their affiliated organizations, or those of the publisher, the editors and the reviewers. Any product that may be evaluated in this article or claim that may be made by its manufacturer is not guaranteed or endorsed by the publisher.
Research integrity at Frontiers
Learn more about the work of our research integrity team to safeguard the quality of each article we publish.