- 1School of Electrical Engineering, Chongqing University, Chongqing, China
- 2Department of Electronic and Electrical Engineering, University of Strathclyde, Glasgow, United Kingdom
- 3State Grid Henan Electric Power Research Institute, Zhengzhou, China
The problem of wind power curtailment (WPC) during winter heating periods in China’s “Three-North regions” is becoming worse. Wind power heating, though being an effective way to increase wind power consumptions, is constrained by high electric heating costs under a peak-to-valley electricity price pattern. This study develops a real-time price (RTP) decision model which adjusts the time-varying RTPs within an acceptable range of heating users based on the WPC distribution over a particular dispatch day. The lower RTPs accompanying the higher WPC can guide the electric heating user side equipped with regenerative electric boilers (REBs) to actively increase REB imports to absorb additional wind generation. Then, the demand side response using REBs under the RTP scheme is optimized to minimize the total heating cost met by electric heating users while assisting in the large-scale wind generation accommodation. The total heating costs and WPC reductions under different heating scenarios are compared and discussed alongside the effectiveness of the RTP-based demand side management in terms of reducing the WPC and heating costs and increasing the feasibility of wind power heating during winter heating periods.
Introduction
By the end of 2019, the cumulative installed capacity of wind power in China reached 210 GW, and the annual national wind generation was about 405.7 TWh (National Energy Administration, 2020). Though the total installed capacity ranked first in the world, the severe issue of wind power curtailment (WPC) restricts China from becoming the largest wind power utilization country. The “Three-North regions” of China having abundant and concentrated wind energy resources are suffering from the most significant WPC, accounting for 98% of the national WPC of 41.9 TWh in 2017 (National Energy Administration, 2020). This is mainly because the installed capacity of renewable energy in the “Three-North regions” is inversely correlated with the local electricity demand. As of the end of 2017, grid-connected wind farms in the “Three-North regions” contributed to about 74.23% of the national total installed capacity and had a wind power penetration rate as high as 70%, while the local electricity consumption reached 36% of the national volume only.
In particular, the electricity generation mix of the “Three-North regions” is dominated by thermal power, most of which is supplied by combined heat and power (CHP) units. In winter heating periods, the mode of “ordering power by heat” reduces the ability of thermoelectric units to adjust their electrical power outputs, resulting in a more significant WPC phenomenon (Liu, 2013; Lü et al., 2013; Yao et al., 2018). The CHP units providing 92% of the total installed capacity of thermal power in Jilin Province must increase their minimum export limits to meet the heat demand required during winter heating periods. In addition, heat demand peaks generally coinciding with electricity demand troughs further reduce the feasible region of wind power acceptance and compress the space for wind power to access the local grids.
Given actual characteristics of the “Three-North regions,” improving the flexibility of CHP units is expected to significantly benefit for the wind power integration (Li et al., 2015; Cui et al., 2016; Xie et al., 2016; Li et al., 2018a; Li et al., 2020). A chance-constrained programming-based scheduling model was developed in (Li et al., 2020) to deal with the insufficient flexibility of an integrated energy system with CHP units. To address the problem of the CHP economic emission dispatch, a two-stage approach combining multi-objective optimization with integrated decision-making was proposed in (Li et al., 2018a). With the deployment of electric boiler and thermal energy storage, a coordinated wind power accommodating dispatch model was developed in (Cui et al., 2016) to improve the operational flexibility of CHP units. In addition, heating with wind power, as an effective solution to the WPC problem, has been significantly promoted in China in recent years (Comprehensive Department of National Energy Administration, 2015). The principle of the wind power heating mode and its role and prospects in improving the grid’s capability of wind power accommodation during off-peak periods were investigated in (Wang et al., 2013).
Energy storage systems (ESSs) can convert energy between multiple forms and enable the time shift of a particular energy form, permitting an effective coordination between power supply and demand (Chen et al., 2015; Gifford et al., 2020; Fan et al., 2019). Nowadays, regenerative electric boiler (REB) as one of the heat storage technologies has been deployed to alleviate the WPC (Xu and Jiang, 2015; Cui et al., 2016). An integrated energy system including wind farms, REBs, and ESS was modeled in (Xu and Jiang, 2015) where the WPC was minimized taking into account the ESS scale and the operating conditions of REBs. The China’s energy “13th Five-Year Plan” anticipated expanding the scale of the wind power heating mode and promoting the integration of REBs with electric heating (Energy Administration of Jilin Province, 2017). In order to maximize wind power consumptions, the import of heat storage is expected to exactly track the WPC. However, the peak-to-valley pattern of electricity price currently adopted in the “Three-North regions” affects the user’s choices of winter heating methods and thus the extent to which the heat storage can assist in the wind power accommodation.
The electricity users in a long-standing consumption mode are reluctant to adjust their consumption behavior to match the load schedule. In order to promote their active participation in the load shaping, an attractive price signal needs to be offered as an incentive (Ketterer, 2014; Shen et al., 2016). Given a reasonable price elasticity of demand, the demand side response (DSR) to short-term variations of electricity prices plays an increasingly important role in balancing short-term supply and demand, especially during peak periods, and in dealing with fluctuations of renewable power outputs (Khodayar and Shahidehpour, 2013; De Jonghe et al., 2014; Sánchez de la Nieta et al., 2014; Shafie-khah et al., 2015). The demand side self-adjustment is more economical and practical than investing in the power supply side to meet the peak demand. Therefore, encouraging users to actively switch to the wind power heating mode is expected to be a practical and direct way to increase wind power consumptions during winter heating periods. This can be realized by reducing real-time electricity prices (RTPs) in response to WPC signals, which guides the users to actively increase the electric heating demands to consume the additional wind generation. The introduction of the DSR under the RTP scheme not only permits an active demand side management (DSM) for the WPC alleviation (Sioshansi and Short, 2009; Ai et al., 2016; Shin et al., 2017; Schmidt and Linder, 2020) but can also reduce heating costs of users, increasing their attractiveness of the electric heating in cost compared to the conventional heating. An RTP decision method has been proposed in (Li et al., 2018b) which constructed a bi-level optimal scheduling model to maximize the system profit.
Most research related to RTPs only sets a single electricity price signal in response to WPC. From the perspective of supply and demand in electricity markets, the article develops an RTP decision model to adjust the time-varying RTPs based on the scale and temporal distribution of WPC in a dispatch day. Then, the responses of electric heating with REBs under the RTP scheme are optimized to minimize the total electric heating cost while realizing the large-scale accommodation of wind generation. The daily heating costs and WPC reductions under four particular heating scenarios are evaluated to verify the effectiveness of the proposed RTP decision model for DSM in terms of reducing the electric heating cost and increasing wind power consumptions.
This article is structured as follows: Theoretical Basis of RTP Decision Model for DSM section introduces the theoretical basis of the RTP decision model for DSM in the wind power heating mode; RTP Decision Model Based on WPC Distribution section describes the RTP adjustment based on WPC distributions; DSR Model Under RTP Scheme for Wind Power Heating section develops a DSR model using REBs under the RTP scheme; Results and Model Validation section evaluates the performance of the proposed models in reducing the WPC and heating costs; and Conclusion and Future Work section presents conclusions and recommendations for further work.
Theoretical Basis of RTP Decision Model for DSM
Wind Power Accommodation by Electric Heating With Heat Storage
The daily curves of total available wind power outputs and electrical and heat demands during a typical high-wind winter heating period of 2015–2016 in a particular province in the “Three-North regions” are shown in Figure 1. The feasible region of wind power acceptance denoted by the area between blue dashed lines is determined by the rise of the electrical demand above the minimum export limit of the thermal power unit. The exceedance of available wind power outputs over the feasible region, that is, denoted by the yellow area, must be curtailed so as to avoid violating the minimum export limit of the thermal power unit. It can be seen that the large-scale WPC mostly coincides with electrical demand troughs at night, while there still exists a small amount of WPC in some particular time slots in the daytime. The high WPC is mainly caused by the mismatch in daily profiles between available wind power outputs and electrical demands.
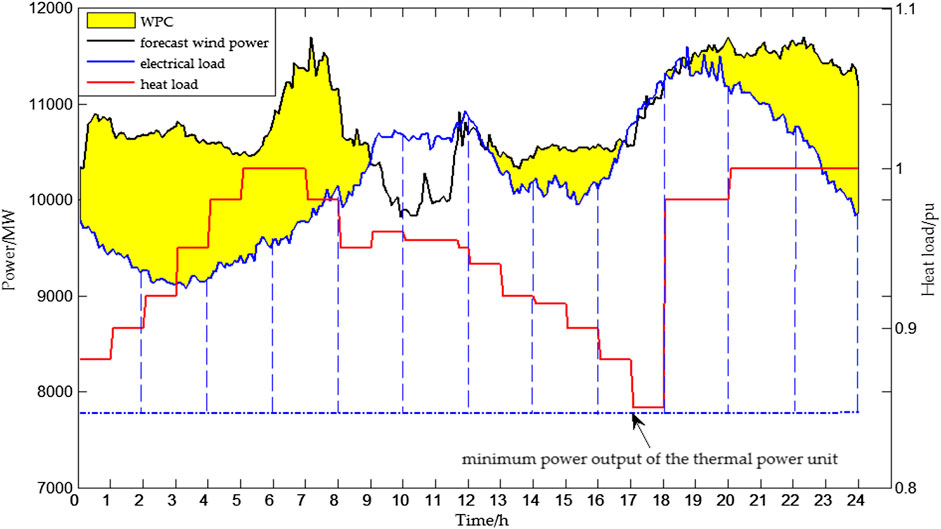
FIGURE 1. Daily profiles of total wind power (MW), electrical load (MW), and heat load (p.u.) in a particular province during a typical high-wind winter heating period of 2015–2016.
It is noted that heat demands and available wind power outputs are shown to have similar diurnal trends where heat demand peaks mostly coincide with high wind power outputs. This permits the use of the electric heating with heat storage to meet heat demands while increasing wind power consumptions. The otherwise curtailed wind power can supply the electric heating demand and/or charge the heat storage during electrical off-peak periods; then, the stored heat is released to the users when needed, which alleviates the constraint of “ordering power by heat” and greatly improves the system flexibility.
REBs are widely used for regenerative electric heating due to their advantages of long service life (20–25 years), simple structure, high heating efficiency, intelligent control, and zero labor cost. There are two main types of REBs, that is, a solid REB and a water REB. The former is studied in this article due to its small footprint, large heat storage capacity per unit volume, and no additional ancillary equipment.
Electric Heating Users With Wind Power Heating
Heating as a kind of livelihood guarantee project requires a high reliability of heat supply. With expanding the scale of the wind power heating mode, the intermittency of wind generation requires the introduction of high-cost conventional generation to ensure the supply reliability. However, the current low prices of fossil fuels make the conventional heating method more attractive to heat users, which slow-paced the development of the electric heating. The contradiction between the electric heating demands and the cost of wind power heating has become the focus of market feasibility studies. To deal with this issue, the study develops an RTP decision model where the time-varying RTPs are adjusted to reflect the scale of predicted WPC during a particular dispatch day and guide electric heating users to actively increase their needs to consume the additional wind generation.
The demand change of a single user has little impact on an entire system. In order to facilitate the DSM, the study integrates the electric heating users within a particular area(s) into a single user set where each user is considered to share the same heating behavior. In addition, it is presumed here that the daily WPC will fully meet the heating demand with the operation of REBs.
RTP Decision Model Based on WPC Distribution
Before a particular dispatch day, wind farms will provide a system operator (SO) with their day-ahead wind power forecasts. Then, the SO combines available wind power forecasts with the electrical demand forecast curve to predict the hourly WPC in the dispatch day, based on which hourly RTPs are determined subject to a set of constraints and issued prior to the dispatch day. The relatively lower RTPs accompanying higher WPC forecasts can encourage a user set to choose the time of heating supplied by the otherwise curtailed wind power and conduct day-ahead transactions according to its heat demand.
Given day-ahead predictions of available wind power outputs Pwind.f(t) (MW) and electrical demands Pload(t) (MW), the WPC forecast Pqf(t) (MW) at each 1-hour time step t within the dispatch day is determined by Eq. 1, avoiding the violation of the minimum export limit Ph.min (MW) of the thermal power unit.
From a range of factors such as electricity prices, weather, and national economy, electricity prices are the dominant factor affecting electricity consumptions of users. The electricity has the common attribute of general goods, that is, a contrary relationship between demand and price, as shown in Figure 2, where D0 and C0 represent the quantity and the price transacted at an equilibrium point, respectively (the supply curve that determines the equilibrium point is not plotted for brevity).
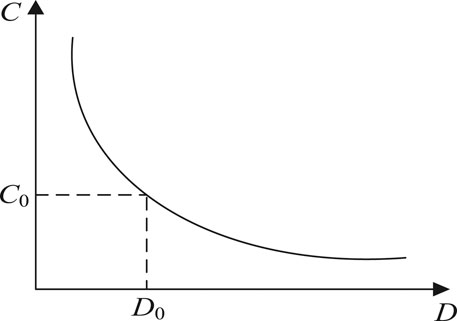
FIGURE 2. Typical demand curve with quantity D0 and price C0 being transacted at the equilibrium point.
The demand curve in Figure 2 can also describe the negative correlation between RTPs and WPC forecasts within a dispatch day, which is approximated here by Eq. 2.
where Ci ($/kWh) denotes the RTP at the ith (i = 1, … , n) time step where wind farms are forecast to be curtailed by Di (kWh); CB ($/kWh) is the benchmark value of RTPs equaling the sum of Ci; and Ci* denotes the per unit RTP relative to CB which inversely depends on Di. Furthermore, Ci should be maintained within a reasonable range below Cimax ($/kWh):
In addition, it must be profitable for a wind farm to inject the otherwise curtailed wind power onto the grid. This means that Ci paid by the user set should be greater than the incremental operating cost cw of the wind farm (i.e., 0.0087 $/kWh in this study):
When there is no WPC at a particular time step, the RTP will not be issued and no transactions will occur at that time step.
DSR Model Under RTP Scheme for Wind Power Heating
Given the REB installation providing a user set with a certain amount of controllable demand, the user set will actively shape its demand curve to match available wind power or WPC forecasts so as to exploit the price opportunity under the RTP scheme described in RTP Decision Model Based on WPC Distribution section. The user set can respond to RTP signals by increasing electricity consumptions at times of low RTPs which result from high WPC forecasts, and vice versa. The DSR using REBs under the RTP scheme not only helps to put additional wind generation onto the grid but also reduces heating costs met by users. From the perspective of the user set, this section will develop a DSR model using REBs to minimize the total heating cost by optimizing the heating behavior under the RTP scheme.
Objective Function
The objective function of the DSR model is the total electric heating cost of a user set in a particular dispatch day:
where ΔDi (kWh) denotes the WPC reduction consumed by the REBs at the ith time step. The imports of the REBs supplied by otherwise curtailed wind power at each time step in the dispatch day are determined to minimize the objective function F. It is noted that the DSR model developed here deals with the optimization of the REB imports only, while the REB exports are not simulated in this work.
Constraint Condition
The daily electricity consumption needed for electric heating, denoted by Eh (kWh), limits the total volume of WPC that can be alleviated on a particular dispatch day:
where W is the heat load index (W/m2), Shot is the heating area (m2), Th is the daily heating duration (h), and η is the electrothermal conversion efficiency. In addition, ΔDi is smaller than the WPC volume Di at the ith time step:
From the perspective of the user set, the implementation of RTPs should not increase the average electricity price met by the user set during the day. This is formulated by Eq. 8 as
where Cav represents the average electricity price ($/kWh) before the price adjustment. In addition, the import rate Pen.in(t) (MW) of an REB at time step t must not exceed its maximum import limit Pin.N (MW):
A flowchart describing the optimization of the REB imports under the RTP scheme is shown in Figure 3.
As a comparison to the wind power heating cost, the conventional heating cost for the heating area Shot (m2) is also estimated here based on the conventional heating price Cr ($/m2) for an entire heating season and then evenly spread to each day within the season.
Results and Model Validation
The developed DSR model using REBs under the RTP scheme for the wind power heating is validated here based on four particular heating days with various WPC distributions (2015.12.18, 2016.01.21, 2016.02.22, and 2016.04.03). The following four different heating scenarios are simulated for each day to evaluate the performance of the proposed approach in putting additional wind generation onto the grid and reducing heating costs met by the user set.
Scenario 1: Conventional heating with a constant conventional heating price;
Scenario 2: Wind power heating under peak-to-valley electricity price scheme with REB importing at off-peak time;
Scenario 3: Wind power heating under peak-to-valley electricity price scheme with REB tracking WPC;
Scenario 4: Wind power heating under the RTP scheme with REB tracking WPC.
The 5-minute average available wind power output forecasts and actual demands at a provincial level in the “Three-North regions” over each day are converted into 1-hour average data in line with the adopted time step length. The province studied here currently employs peak-to-valley electricity prices for electric heating, as shown in Table 1 (Jilin Province Price Bureau, 2018). The total area of the 15 residential districts with the heat demand across the province is about 3 million m2. Given technical parameters of a solid REB and the residential district heating as listed in Tables 2, 3, respectively, it is evaluated by Eq. 6 that each 0.2 million m2 residential district needs to be equipped with 11 REBs. Therefore, it is presumed here that a total of 165 REBs are installed within the whole province.
Simulation Results of the RTP Decision Model
Figure 4 shows the daily curves of WPC forecasts on the four heating days and their corresponding RTPs determined by the RTP decision model (for the exact RTP values, the reader is referred to Table A1 in Appendix). The WPC is shown to not only happen in off-peak periods but may also occur at times of normal or even peak electrical demands. This means that REBs simply importing during electrical demand troughs cannot ensure the WPC to be minimized. Therefore, it is necessary to implement the RTP scheme that guides the user set to charge REBs in response to the WPC, that is, tracking the WPC.
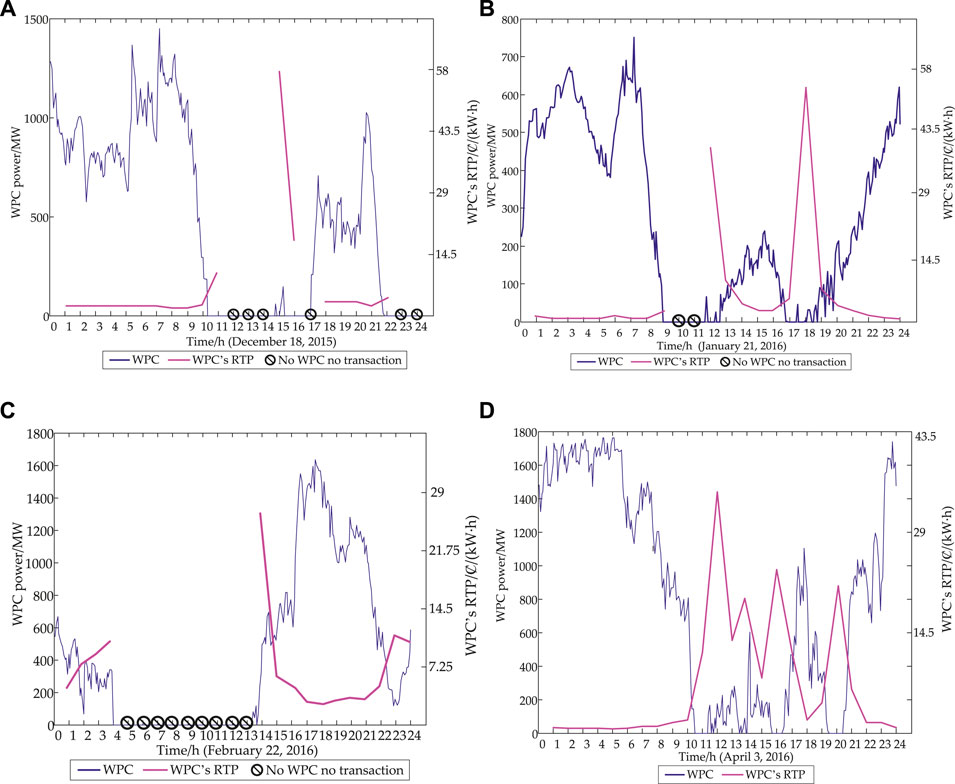
FIGURE 4. Daily curves of WPC (MW) and corresponding RTPs (C//kWh) on (A) 2015.12.28, (B) 2016.01.21, (C) 2016.02.22, and (D) 2016.04.03.
Evaluation on WPC Reductions and Heating Costs
In Scenario 4, the time and rates at which the REBs import are optimized to minimize the total heating cost. Figure 5 shows the aggregation of the optimized imports of REBs along with the remaining WPC in Scenario 4 on 2016.01.21 and 2016.02.22 where the REB imports greatly increase wind power consumptions which would otherwise be curtailed during off-peak and peak periods, respectively. To illustrate the advantages of the DSR model under the RTP scheme adopted in Scenario 4, total WPC reductions and total heating costs over each day estimated under the four heating scenarios are compared in Figure 6 and Table 4.
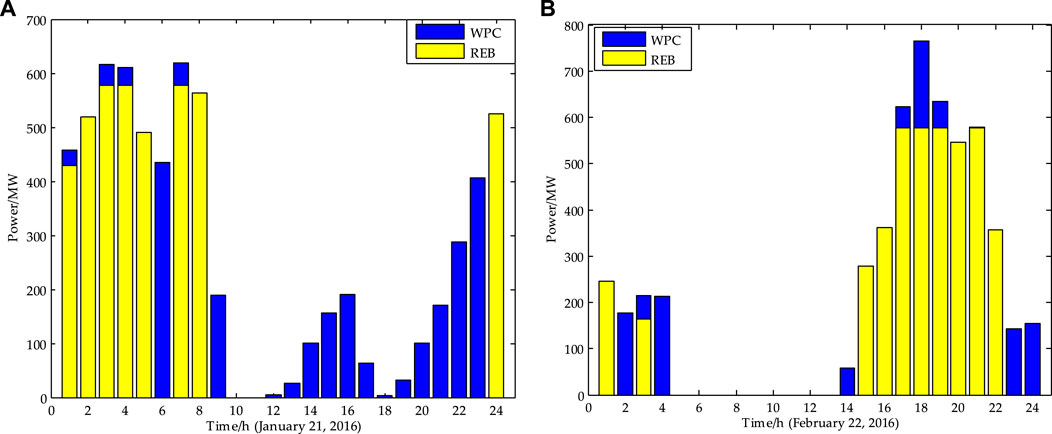
FIGURE 5. Aggregation of optimized REB imports (MW) and remaining WPC (MW) under Scenario 4 on (A) 2016.01.21 and (B) 2016.02.22.
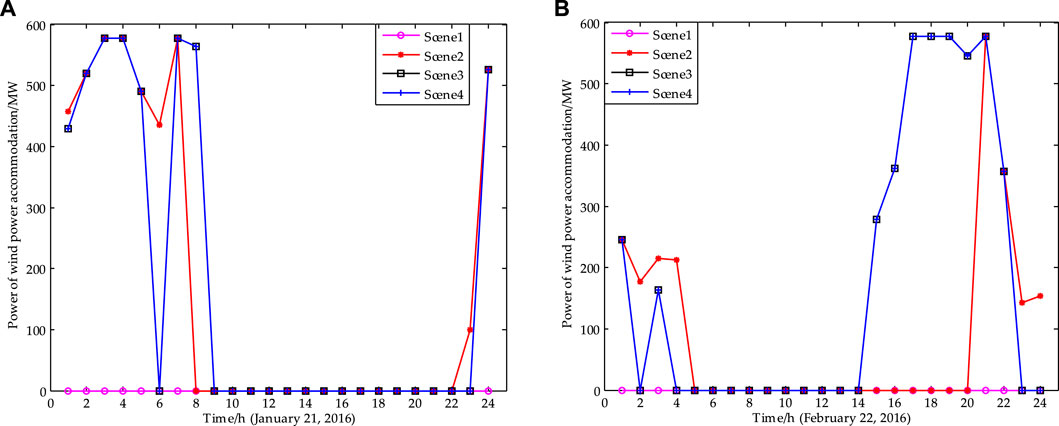
FIGURE 6. WPC reductions (MW) under the four heating scenarios on (A) 2016.01.21 and (B) 2016.02.22.
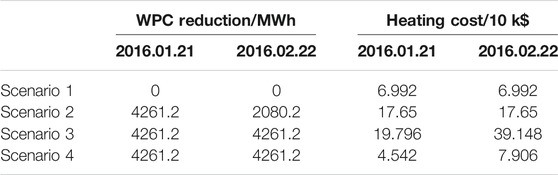
TABLE 4. Total WPC reductions (MWh) and heating costs (10 k$) under four heating scenarios on two particular days.
The wind power heating mode employed in Scenarios 2–4 is mostly shown to achieve a maximum of 4261.2 MWh WPC reduction with the help of REBs. However, simply charging REBs at off-peaks in Scenario 2 may decrease the REB performance in the WPC reduction depending on the temporal distribution of WPC. For example, only 2080.2 MWh otherwise curtailed wind generation is absorbed by REBs on 2016.02.22 where the WPC mostly occurs during peak demands (see Figure 4C).
Compared to the conventional heating method in Scenario 1, the peak-to-valley electricity price pattern adopted in Scenarios 2 and 3 leads to significant electric heating costs. With the implementation of the RTP scheme in Scenario 4, total electric heating costs are largely reduced to the levels close to or even smaller than the total conventional heating cost. The significantly decreased electric heating costs will make the electric heating with REBs more attractive, expanding the scale of the wind power heating mode. In addition, the total heating cost on 2016.02.22 is higher than the total cost on 2016.01.21 under Scenario 4. This is because the WPC occurs at such fewer time steps that higher RTPs are issued on 2016.02.22. Among all heating scenarios, Scenario 4 using REBs to track the WPC under the RTP scheme achieves the greatest WPC reduction and an acceptable total heating cost.
The additional wind generation put onto the grid can reduce fossil fuel consumptions of conventional thermal power plants and thus increase the environmental benefit. The revenue associated with the environmental benefit received by wind farms can contribute to the use-of-system charge paid to the SO who is responsible for the reliable system operation. The win-win situation of wind farms, network owners, and electric heating users illustrates the feasibility of implementing the DSR with REBs under the RTP scheme as proposed in this work.
Conclusion and Future Work
The electric heating demand management permitted by the heat storage combined with a suitable electricity price incentive is expected to assist in the development of the wind power heating mode during winter heating periods. This study has developed a real-time price (RTP) decision model to introduce an RTP scheme taking into account a negative correlation between the RTP and the wind power curtailment (WPC) within a particular dispatch day. The time-varying RTPs not only met the acceptable range of electric heating users but also covered the cost of a wind farm to put otherwise curtailed wind power onto the grid. Given electric heating users being equipped with regenerative electric boilers (REBs), a demand side response (DSR) model using REBs under the RTP scheme was developed to minimize the total electric heating cost by charging REBs at lower RTPs so as to alleviate the higher WPC. The developed RTP decision and DSR models have been tested on four particular heating days at a provincial level in the “Three-North regions” in China. Compared to the currently adopted peak-to-valley price pattern, the RTPs reflecting the WPC distribution in a dispatch day permitted the electric heating users with REBs to exploit the price opportunity while helping to increase wind power consumptions. Furthermore, the implementation of the RTP scheme largely reduced the total electric heating cost met by users to the level close to or even smaller than the conventional heating cost, which may increase the attractiveness of the electric heating with REBs in cost, and in turn, expand the scale of the wind power heating mode. In addition, the DSR model optimizing the REB imports to track the lower RTPs has maximized the WPC reduction in this work, while simply charging the REBs during electrical demand troughs may degrade the WPC alleviation depending on the temporal distribution of WPC.
Building on the present work, the integrated energy system modeled here should be extended to incorporate other renewable energy resources such as photovoltaic energy and reflect transmission constraints on both heat and electricity. This will permit to estimate the most suitable REB locations within the system that maximize the use of renewable energy. Furthermore, capital and operating expenditures of REBs will be compared with the heating cost saving of users relative to the conventional heating. The cost–benefit analysis can indicate the investment return and payback period, assessing the economic feasibility of deploying REBs from the perspective of heating users. In addition, the proposed RTP-based demand side management for the wind or renewable power heating will be further tested on other cities or provinces, for example, in the “Three-North regions,” which are suffering from the WPC problem in heating seasons.
Data Availability Statement
The original contributions presented in the study are included in the article/Supplementary Material; further inquiries can be directed to the corresponding author.
Author Contributions
QL performed the experiment and simulation; JL contributed to the conception of the work; ZH performed analysis and prepared the manuscript; FF performed analysis and revised the manuscript; and WT assisted in analysis with constructive discussions.
Funding
This research was supported by National Natural Science Foundation of China (NSFC) (51707016).
Conflict of Interest
The authors declare that the research was conducted in the absence of any commercial or financial relationships that could be construed as a potential conflict of interest.
Appendix
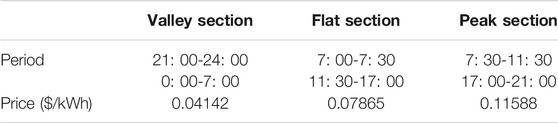
TABLE A1. Exact RTP values (C//kWh) on (a) 2015.12.18, (b) 2016.01.21, (c) 2016.02.22, and (d) 2016.04.03.
References
Ai, X., Zhou, S., and Zhao, Y. (2016). Study on time of use pricing of user side considering wind power uncertainty. Power Syst. Technol. 40 (5), 1529–1535. doi:10.13335/j.1000-3673.pst.2016.05.035 [in Chinese, with English summary]
Chen, L., Xu, F., Wang, X., Min, Y., Ding, M., and Huang, P. (2015). Implementation and effect of thermal storage in improving wind power accommodation. Proc. CSEE 35 (17), 4283–4290. doi:10.13334/j.0258-8013.pcsee.2015.17.001 [in Chinese, with English summary]
Comprehensive Department of National Energy Administration (2015). Notice on the work of clean heating using wind power (No. 306, 2015). Available at: http://zfxxgk.nea.gov.cn/auto87/201506/t20150615_1938.htm (Accessed June 5, 2015) [in Chinese, with English summary]. [Online]
Cui, Y., Chen, Z., Yan, G., and Tang, Y. (2016). Coordinated wind power accommodating dispatch model based on electric boiler and CHP with thermal energy storage. Proc. CSEE 36 (15), 4072–4081. [in Chinese, with English summary]. doi:10.13334/j.0258-8013.pcsee.152406
De Jonghe, C., Hobbs, B. F., and Belmans, R. (2014). Value of price responsive load for wind integration in unit commitment. IEEE Trans. Power Syst. 29 (2), 675–685. doi:10.1109/tpwrs.2013.2283516
Energy Administration of Jilin Province (2017). Notice on issuing the “13th Five-Year plan” for energy development in Jilin. Available at: http://nyj.jl.gov.cn/ghjh/20170522/852.html(Accessed May 22, 2017) [in Chinese, with English summary]. [Online]
Fan, F., Xu, H., and Kockar, I. (2019). “Utilisation of energy storage to improve distributed generation connections and network operation on Shetland Islands,” in Proceedings of 25th international conference on electricity distribution, Madrid, Spain, June 3, 2019 (CIRED 2019 Conference), 1764, 1–5.
Beijing GasHeating Engineering Design Institute (2010). City thermal net design criterion CJJ34-2010. Beijing, China: China Architecture & Building Press, 21–30.
Gifford, J., Ma, Z., and Davenport, P. (2020). Thermal analysis of insulation design for a thermal energy storage silo containment for long-duration electricity storage. Front. Energy Res. 8, 99. doi:10.3389/fenrg.2020.00099
Jilin Province Price Bureau (2018). Notice on further clarifying the relevant issues concerning the clean heating price policy of the province. Available at: http://wjj.jl.gov.cn/scjgjg/zyynyjg_67230/dj/201802/t20180223_3716147html(Accessed February 23, 2018) [in Chinese, with English summary]. [Online]
Ketterer, J. C. (2014). The impact of wind power generation on the electricity price in Germany. Energy Econ. 44, 270–280. doi:10.1016/j.eneco.2014.04.003
Khodayar, M. E., and Shahidehpour, M. (2013). Stochastic price-based coordination of intrahour wind energy and storage in a generation company. IEEE Trans. Sustain. Energy 4 (3), 554–562. doi:10.1109/tste.2012.2228284
Li, Y., Wang, C., Li, G., Wang, J., Zhao, D., and Chen, C. (2020). Improving operational flexibility of integrated energy system with uncertain renewable generations considering thermal inertia of buildings. Energy Convers. Manag. 207, 112526. doi:10.1016/j.enconman.2020.112526
Li, Y., Wang, J., Zhao, D., Li, G., and Chen, C. (2018a). A two-stage approach for combined heat and power economic emission dispatch: combining multi-objective optimization with integrated decision making. Energy 162, 237–254. doi:10.1016/j.energy.2018.07.200
Li, Y., Yang, Z., Li, G., Mu, Y., Zhao, D., Chen, C., et al. (2018b). Optimal scheduling of isolated microgrid with an electric vehicle battery swapping station in multi-stakeholder scenarios: a bi-level programming approach via real-time pricing. Appl. Energy 232, 54–68. doi:10.1016/j.apenergy.2018.09.211
Li, Z., Wu, W., Shahidehpour, M., Wang, J., and Zhang, B. (2015). Combined heat and power dispatch considering pipeline energy storage of district heating network. IEEE Trans. Sustainable Energy 7 (1), 12–22. doi:10.1109/TSTE.2015.2467383
Liu, J. (2013). Basic issues of the utilization of large-scale renewable power with high security and efficiency. Proc. Chin. Soc. Electr. Eng. 33 (16), 2–8. doi:10.3969/j.issn.2095-0802.2014.11.031 [in Chinese, with English summary].
Lü, Q., Wang, W., Han, S., Yuan, S., Zhang, J., and Li, W. (2013). A new evaluation method for wind power curtailment based on analysis of system regulation capability. Power Syst. Technol. 37 (7), 1887–1894. [in Chinese, with English summary]. doi:10.1016/S0924-8579(07)70847-6
National Energy Administration (2020). Wind power grid operation in the first half of 2018. Available at: http://www.nea.gov.cn/201802/01/c_13694 2234.htm (Accessed February 1, 2020) [in Chinese, with English summary]. [Online]
Sánchez de la Nieta, A. A. S., Contreras, J., Muñoz, J. I., and O'Malley, M. (2014). Modeling the impact of a wind power producer as a price-maker. IEEE Trans. Power Syst. 29 (6), 2723–2732. doi:10.1109/tpwrs.2014.2313960
Schmidt, M., and Linder, M. (2020). A novel thermochemical long term storage concept: balance of renewable electricity and heat demand in buildings. Front. Energy Res. 8, 137. doi:10.3389/fenrg.2020.00137
Shafie-khah, M., Heydarian-Forushani, E., Golshan, M. E. H., Moghaddam, M. P., Sheikh-El-Eslami, M. K., and Catalão, J. P. S. (2015). Strategic offering for a price-maker wind power producer in oligopoly markets considering demand response exchange. IEEE Trans. Ind. Inf. 11 (6), 1542–1553. doi:10.1109/tii.2015.2472339
Shen, J., Jiang, C., Liu, Y., and Wang, X. (2016). A microgrid energy management system and risk management under an electricity market environment. IEEE Access 4, 2349–2356. doi:10.1109/access.2016.2555926
Shin, H., Lee, D., and Baldick, R. (2017). An offer strategy for wind power producers that considers the correlation between wind power and real-time electricity prices. IEEE Trans. Sustainable Energy 9 (2), 695–706. doi:10.1109/TSTE.2017.2757501
Sioshansi, R., and Short, W. (2009). Evaluating the impacts of real-time pricing on the usage of wind generation. IEEE Trans. Power Syst. 24 (2), 516–524. doi:10.1109/tpwrs.2008.2012184
Wang, C., Li, Q., and Xie, G. (2013). Evaluation of wind power heating in facilitating wind power integration capability during valley load period. Electr. power 46 (12), 100–106. [in Chinese, with English summary]. doi:10.4028/www.scientific.net/amr.953-954.501
Xie, D., Lu, Y., Sun, J., Gu, C., and Li, G. (2016). Optimal operation of a combined heat and power system considering real-time energy prices. IEEE Access 4, 3005–3015. doi:10.1109/access.2016.2580918
Xu, M., and Jiang, D. (2015). Research on energy performance and economic analysis of wind power heating system. Energy China 37 (8), 42–47. doi:10.3969/j.issn.1003-2355.2015.08.008 [in Chinese, with English summary]
Keywords: demand side management, electric heating, real-time electricity price, regenerative electric boiler, wind power heating
Citation: Li Q, Li J, Huang Z, Fan F and Teng W (2021) A Real-Time Electricity Price Decision Model for Demand Side Management in Wind Power Heating Mode. Front. Energy Res. 9:634603. doi: 10.3389/fenrg.2021.634603
Received: 28 November 2020; Accepted: 12 January 2021;
Published: 26 February 2021.
Edited by:
Yang Li, Northeast Electric Power University, ChinaReviewed by:
Chen Liang, Nanjing University of Information Science and Technology, ChinaShaoyan Li, North China Electric Power University, China
Copyright © 2021 Li, Li, Huang, Fan and Teng. This is an open-access article distributed under the terms of the Creative Commons Attribution License (CC BY). The use, distribution or reproduction in other forums is permitted, provided the original author(s) and the copyright owner(s) are credited and that the original publication in this journal is cited, in accordance with accepted academic practice. No use, distribution or reproduction is permitted which does not comply with these terms.
*Correspondence: Qiang Li, NDE5NzM3MTAwQHFxLmNvbQ==