- Business School, Shandong University, Weihai, China
This study explores the impact of public concerns on green innovation in China’s automotive industry and examines whether the effect varies based on firm size, ownership, and time phase. The study investigates 151 automobile enterprises and provides a novel, large-scale, and data-based perspective and estimation method for exploring critical factors of green innovation. By applying transition probabilities matrix (TPM) model, this paper finds that for different-sizes automotive enterprises there are significant differences in innovation sustainability, non-innovation sustainability, and liquidity between innovation and non-innovation, and such differences also exist for state-owned and non-state-owned enterprises. Then, based on the dynamic panel random probit (DPRP) model, the paper further analyzes the possible reasons for these differences, and particularly focuses on exploring the impact of public environmental concern on the environmental technology innovation. The empirical results show: 1) public concerns encourages green innovation emerging in all automotive firms, but only affects innovation persistence in medium and large companies. 2) public concerns encourages non-innovator state-owned companies to become innovators and motivates them to maintain continuous innovation. 3) the impact of public concerns changes over time. In the periods of 2002–2007 and 2012–2013, the role of public concerns is not significant. However, in the 2007–2012 period, public concerns significantly stimulate enterprises to move from non-innovators to innovators and promotes continuous innovation.
Introduction
Global warming, which is caused by relentless increases in greenhouse gas, especially CO2 emissions, has become a key challenge for the society worldwide (Liu et al., 2020a). Alongside with the rapid economic growth, China has overtaken the United States as the world’s largest CO2 emitter in around 2007 (Fu et al., 2021); According to the International Energy Agency (IEA), China’s CO2 emissions accounted for 29% of the world’s total in 2019. Energy consumption in the transportation industry has become one of the significant contributor to the rapid increase in China’s CO2 emissions (Song et al., 2019). With hindsight, experiences in a large number of developing countries show that the growth rates of emissions in the transportation industry are faster than those of aggregate CO2 emissions (Loo and Li, 2012; Li et al., 2019). At the same time, among the different modes of transportation, road transport is the biggest contributor to CO2 emissions in many countries (Cai et al., 2011; Solaymani, 2019). In China, total carbon emissions from the freight industry increased 25.81 times from 3.7352 Mt in 1988 to 96.4158 Mt in 2016, and the road freight was the sector with the largest increase in carbon emissions, with a 119.38-fold increase (Lv et al., 2019).
Related statistics depict that the energy consumption of the transportation industry has been grown steadily and rapidly in China (Zhu et al., 2020). As a consequence, the environmental pollution caused by automobile industry is serious in China, and the emission of the automobile industry has been recognized as a key culprit for haze and photochemical smog pollution (Lu et al., 2021). Therefore, promoting green innovation in the automotive industry is imperatively important for controlling greenhouse gas emissions and improving the atmospheric environment (Gohoungodji et al., 2020).
Green innovation is also named as environmental innovation and sustainable innovation (Schiederig et al., 2012; Meng et al., 2020). However, it has yet formed a widely recognized definition of green innovation. Existing literature has proposed different definitions based on various perspectives and theoretical underpinnings. One strand of literature defines the green innovation with a specific emphasis on the object, content and objective of green innovation. For example, both Chen (2008) and Liao (2017) hold a similar view that green innovation involves hardware innovation or software innovation activities related to green products or processes, such as technology innovation in energy conservation, energy saving, pollution prevention, waste recycling, green product design or enterprise environmental management. Tarnawska (2013) argued that the goal of enterprises green innovation is to improve resource use efficiency, reduce production costs and enhance competitiveness of the enterprises. Another strand of literature defined green innovation based on the innovation effect. For example, Ghisetti et al. (2017) defined green innovation as the solution for new or important products (or services) and processes that reduce the consumption of natural resources and the release of harmful substances throughout the life cycle. Berrone et al. (2013) proposed that green innovation refers to the development of products, processes and services that can reduce environmental hazards by using new methods to deal with emissions, recycle or reuse waste, and find cleaner sources of energy. Combing the above two points of view, Schiederig et al. (2012) concluded that green innovation is driven by economic or ecological benefits, guided by meeting market demand or achieving market competitiveness, and results in reducing negative environmental impact.
Present literature on green innovation in the automobile industry mainly focuses on the following aspects. On the one hand, some literature have concerned on the poor innovation motivation of automobile enterprise. The poor innovation motivation can be attributed to various factors. First, environmental externality may deter enterprises from engaging green innovation activities. To be specific, investment and efforts to mitigate climate change and reduce air pollution exhibit a strong positive environmental externality, and the benefits received by climate change contributors (enterprises) are usually significantly lower than those received by the society (Gohoungodji et al., 2020; Qi et al., 2021), which discourages enterprises’ concerns and commitments in green innovation. Second, inadequate green innovation capacity is another key impediment for green innovation. In some circumstances, some first movers of green innovation in the automotive industry does not generate apparent price advantages or performance features due to the inadequate capacity, which discourages green innovation incentives (Penna and Geels, 2015; Gupta and Barua, 2018). Third, a mismatch between innovation and traditional system is another barrier for simulating and fostering green innovation (Hao et al., 2019). In the process of green innovation, some new elements are usually incompatible with the rules, infrastructure, users’ custom of the traditional or existing socioeconomic and technological systems, which may lead to a high sunk cost (Freeman, 1995; Gupta and Barua, 2018).
On the other hand, in views of the poor internal incentive, some scholars have studied how to use external factors to motivate green innovation in the automobile industry. Existing literature focuses on the effect of three aspects, including industrial policy, environmental policy and market demand on green innovation. For example, based on the number of new energy vehicles patents in China’s automobile industry, Liu et al. (2020b) found that China’s industrial policy has a significant impact on green innovation in the automobile industry. Cristina De Stefano et al. (2016) studied innovation in the automotive industry from the perspective of climate change policy and argued that it is necessary for automotive companies to continue to innovate in product stewardship in order to survive in a carbon-constrained market. Using data from 145 companies belonging to automotive parts manufacturing sector in Spanish, Leal-Rodríguez et al. (2018) links market orientation, green innovation and enterprise performance and finds that market positioning is a key factor for green innovation and maintaining a company's competitive advantage.
To summarize the above, most of the existing literature focuses on the poor innovation motivation of automobile enterprise and the effect of policy and market demand on stimulating green innovation. However, some scholars began to study the impact of public attention on green innovation in the automobile industry. Public concerns is an important concept in risk perception, communication and management literature, and a reason for policy attention (Fellenor et al., 2020). Penna and Geels (2015) argued that with the advent and prevalence of big data technologies such as social media, the external pressure from the public and the media has become an important driving force to promote green innovation in the automotive industry. Olson (2013) argued that China is the top automobile production and consumption market and the largest greenhouse gases emitter in the world. It is of great importance to evaluate the influence of public concerns on the green innovation of China's automobile industry. Empirical evidence of China is valuable to shed the light of the effects of public environmental concerns on green innovation in developing countries.
This study aims to explore the effect of public concerns on green innovation of automobile enterprises in China. Three specific research questions are investigated. 1) Does public concerns motivate automobile companies to transform from non-innovators (without green patents) to innovators (with green patents)? For most companies, the first patent is more difficult to obtain than subsequent ones (Cefis, 2003), making that transformation more challenging. 2) Does public concerns improve innovation commitment of automobile companies? Some studies have shown that companies with experience of innovation activities are of high possibility to engage innovation in future developments (Raymond et al., 2010), which can be attributed to state dependence (Ayllón and Radicic, 2019). This study also aims to examine whether public concerns will increase the probability that a company will pursue further innovation. 3) Does the effect of public concerns vary depending on enterprises’ attributes such as enterprise size, ownership, and time phase? First, public concerns may take heterogenous effects on different enterprises. For example, small and medium enterprises usually have to face more impediments and challenges to innovation than large enterprises (Raymond et al., 2010). Second, ownership is an important determining factor of green innovation. The intention and capacity of green innovation of state-owned companies may generally differ from those of private or foreign-owned companies (Hu et al., 2020). Third, the effect of public concerns on green innovation may show temporal characteristics at different stages. For example, according to the dialectic issue life cycle (DILC) model (Geels and Penna, 2015; Sillak and Kanger, 2020), the public’s response to environmental issues occurs and vary in several typical phrases. Thus, we study the impact of public concerns on green innovation at different stages.
The contributions of this study are as follows. First, the perspective of public environmental concern is introduced to the research domain of industrial green innovation, which enrich the research paradigms of green innovation. Green innovation studies primarily focus on the economy, policy, and technology aspects, whilst the effect of public concerns is largely omitted. The rapid development of social media platforms has enabled public environmental concern become a key driving force for promoting green innovation in different industries. Estimation results of this study shed light on the effect of public environmental concern on green innovation. Second, a novel quantitative-based evaluation framework based on multi-sourced data is proposed to explore the effect of public concerns on industrial green innovation. By using transition probabilities matrix (TPM) model, two important measurable indicators of innovation dynamics in Chinese automotive enterprises, including innovation transition and innovation persistence, are systematically investigated in this study. By using dynamic panel random probit (DPRP) model, this study quantitatively analyzes the impact of public attention on industrial green innovation, which complements the current case studies and deepens the understanding of external pressures to promote corporate green innovation.
The rest of this paper is organized as follows. Section Theoretical Framework and Hypotheses introduces theoretical framework and research hypotheses. Section Research Methods illustrates the research methods. Section Indicators and Data describes variables and data. Section Empirical Results presents the empirical results and robustness checks. Section Discussion and Conclusion concludes the study.
Theoretical Framework and Hypotheses
This section reviews relevant theories and research hypotheses are proposed.
Impact of Public Concerns on Green Innovation Emerging
The public’s concerns on environmental issues essentially form an either formal or informal supervision on pollution emitter (enterprises), and such supervision involves a variety of stakeholders, including environmental protection enthusiasts, community residents, consumers, non-governmental organizations, etc. (Blackman and Bannister, 1998). By influencing the election and voting, the public’s concerns on environmental issues can promote the legislation of environmental policy in some countries (Kahn, 2007).
In addition to the supervision effects, public environmental concerns can forester green innovation by affect consumer’s behavior and lifestyle (Leonidou et al., 2017). The public environmental concerns of consumers can be transformed to buying preference for green product (Rugman and Verbeke, 1998). Therefore, enterprises with a good reputation of green innovation can improve the sales and competitiveness by meeting the demand of consumers (Revell et al., 2010). Environmental policy can promote enterprises’ green innovation in the short term, whilst the changes of consumer’s behavior and lifestyle toward environment-friendly ones are the sustainable force to encourage green innovation (Geels, 2010; Lin et al., 2019).
As to China’s situation, this study assumes that environmental problems affect the regular life to people, thereby changing the cognitive roles of the public (e.g., raising public concerns on environmental issues) and promoting the emergence of green innovations. Thus, the following assumption hypothesis is proposed:
H1: Public concerns about environmental issues encourages green innovation emerging in Chinese automobile companies.
Impact of Public Concerns on the Persistence of Green Innovation
If the previous technological innovative activities of enterprises can significantly increase the probability of performing innovative practices in the future, then the continuity of technological innovative activities is considered persistent (Cefis, 2003; Clausen et al., 2012). Triguero and Córcoles (2013) further developed this concept in the context of conditional probability; that is, technological innovation sustainability refers to the probability of technological innovation in year
The theory of planned behavior (TPB) was proposed by Ajzen (1985), who incorporated perceived behavioral control into the theory of reasoned action. According to the TPB, the main determinant of behavioral intention is attitude (Ajzen, 1991). Attitude results from behavioral assessment while behavior and result are functions of behavioral attitude. The TPB has been successfully used to explain both general pro-environmental behavior (Chao, 2012) and specific behaviors such as organic food purchasing (Yazdanpanah and Forouzani, 2015), cleaner production technology adoption (Zhang et al., 2013), and environmental activism (Fielding et al., 2008). Thus, in the context of global warming and serious air pollution in China, the public will focus on measures to tackle pollution, including green innovation (Hu et al., 2020). Green products are environmentally friendly and can help to reduce pollution problems (Yu and Han, 2019), so the public will increase their demand for green products based on rational health considerations. In other words, public concerns about green innovative activities often indicates a potential demand for green products.
Two theories have been proposed for the relationship between market demand and technological innovation: the “demand-pull model” and the “technological innovation and demand interaction.” Demand-pull model theory refers to the phenomenon that occurs when enterprises conduct technological innovative activities to earn high profits as they face actual or potential high market demand (Schmookler, 2013). Technological innovation and demand interaction theory holds that the potential market demand can stimulate enterprises’ technological innovation activities. With forthcoming solutions to technical problems, product functions have rapidly increased; these new functions continue to stimulate and guide changes in market demand, and strong demand can entice enterprises to continue technological innovation for improved functionality (Mowery and Rosenberg, 1979). This suggests the following hypothesis:
H2: Public concerns on environmental issues positively affects the sustainability of green innovation.
Time Characteristic Factor
Penna and Geels (2012) established a DILC model by combining insights into life cycle theory. They argued that external pressure can be divided into five stages performed by different major players based on qualitative research of the repositioning of United States carmakers in response to global warming issues (1943–1985) and traffic accidents/safety issues (1900–1995) (Penna and Geels, 2012; Geels and Penna, 2015). In the first three stages, companies are reluctant to undergo substantive changes to solve their problems because they are “locked in” to four industry-specific systems: 1) industry beliefs and mentality, 2) identity and mission, 3) regulations and formal policies, and 4) competence and technical knowledge. Companies utilize social culture and political strategies to resist this pressure. When environmental problems affect the economic environment, the life cycle moves through the last two stages. In the fourth stage, the mounting public concerns pushes the issue toward the macro-political stage. Moreover, radical legislative policies will essentially change the economic framework. In the fifth stage, environmental issues affect the preferences of mass consumers, thereby resulting in strong market demand for new technologies.
On the basis of DILC theory, the following hypotheses are proposed:
H3a: The impact of public concerns on green innovation in China’s automobile industry varies over time.
H3b: The impact of public concerns on the persistence of green innovation in China’s automobile industry varies over time.
Influence of Enterprise Characteristic Factors
Consumers want to buy high-quality and safe products, but they also want to know if they are produced in a responsible way. These aspects are related to Corporate Social Responsibility (CSR). Corporate Social Responsibility is often defined as “the concept of a company on a voluntary basis that incorporates social and environmental issues into its business operations and interaction with stakeholders.” Therefore, socially responsible practices include environmental responsibility measures related to natural resources management and eco-innovation implementation (Kesidou and Demirel, 2012).
However, corporate social responsibility may vary depending on different industries, companies, and departments (Cramer, 2005). The theory of corporate social responsibility shows that large companies should take a high degree of social responsibility (McWilliams and Siegel, 2000). In China, state-owned enterprises should take more social responsibility (Garde Sánchez et al., 2017). Zeng et al. (2011) believes that in China, the influence of environmental organizations and media attention on state-owned enterprises is much greater than that on non-state-owned enterprises. In fact, current national plans and policies related to sustainable development, such as energy conservation in China, are mainly concentrated on the regulation of state-owned enterprises (Kostka et al., 2013). Therefore, the following assumptions are made:
H4a: Public concerns has a greater impact on promoting green innovation in large enterprises than in small companies.
H4b: Public concerns has a greater impact on innovation persistence in large enterprises than in small companies.
H4c: Public concerns has a greater impact on promoting green innovation of state-owned enterprises than non-state-owned enterprises.
H4d: Public concerns has a greater impact on innovation persistence of state-owned enterprises than non-state-owned enterprises.
Research Methods
Transition Probabilities Matrix Model
Several studies on the determinants of innovation have focused on the distribution of innovative and non-innovative enterprises in a given period. However, the distribution of a cross-section may refer to the coexistence of different transfer modes because enterprises may change from one innovative status to another over time. In general, this dynamic change is distinguished by an autoregressive process and TPM model. However, the time series of patent data is short, and biased estimates of the persistence of technological innovation are obtained using an autoregressive process. In addition, patent data are affected by several other factors, except for one-period lagged variables. Thus, the regression coefficient cannot fully reflect the extent of persistence. TPM can simultaneously consider cross-sectional and time series information and can thus effectively reflect the continuity of technological innovation. Thus, the TPM method is utilized in this study.
TPM is an important concept in the Markov chain. The basic assumption is that the probability distribution of the system status of the
Where
Accordingly, TPM
All TPMs satisfy the following two conditions: Eq. 1 the elements of the matrix are non-negative numbers and less than or equal to 1, and Eq. 2 the sum of the elements of each row equal 1. That is,
Patent data are used to investigate industrial green innovation and establish two patent statuses based on relevant patents (i.e., without patents and with at least one patent). Therefore, TPM would be expressed as follows:
Specifically, this study focuses on the probability that a company changes from being a non-innovator to an innovator
Dynamic Panel Random Probit Model
The dynamic panel random probit model is used to analyze whether public concerns affects industrial green innovation. In this model, the probability of an event depends on its outcome at the previous time node and non-observed heterogeneity. Moreover, the model allows “status dependency” in the innovation process. Cross-multiplying terms of public concerns (
where
According to Cefis and Marsili (2015), a random effects model is selected for estimation as the dynamic panel random probit model assumes that individual effects are independent of explanatory variables.
Indicators and Data
Dependent Variable
The dependent variable
The innovation output (patents for automobile emission reduction, using the IPC numbers in Dechezleprêtre et al. (2015)) is applied to reflect whether the company is an innovator in the field of energy conservation. At the same time, represent innovation as a stochastic process of the two statuses to reflect the dynamic changes in enterprises. In
In addition to the aforementioned patent data, data from automobile companies must be obtained to calculate each company’s transition probabilities. For information on how to obtain data of automobile enterprises and how to match enterprises and patents, please refer to Supplementary Material 3.
Independent Variables
Public attention: Traditional public attention measurements can be divided into two approaches, namely, the most important problem (MIP) test and the media coverage index across the country. The MIP test provides respondents with a series of representative investigative questions for answers on what they consider the most important issue in the country at present. Subsequent information is collected, and the questions that are more important than others are summarized. McCombs and Zhu (1995) argued that the MIP test can force testers to respond to a limited number of questions that are prepared in advance. Henry and Gordon (2001) claimed that the MIP test has methodological deficiencies, while Wlezien (2005) argued that the MIP test has reliability and validity problems.
Considering the limitations of MIP, some scholars have proposed a dynamic media coverage index as the measurement method. The media coverage index refers to the number of instances of media coverage on a particular problem within a period. Public and media attention is generally believed to be strongly relevant, and the media coverage index is a lower cost, more flexible approach than the MIP test. However, some researchers have questioned the causal relationship between media coverage and public concern; that is, whether the media reports attract public attention or public concern leads to media coverage.
To address the shortcomings of the two methods, researchers have proposed a method of measuring public attention using search engines. Ripberger (2011) used Google for public attention because this search engine possesses the largest market share in the United States. Therefore, this study selects Baidu, which has the largest market share in China.
The Baidu index is a data-sharing platform based on the behavior data of a massive number of Internet users. Baidu uses search volume as the database for analyzing and calculating the weighted sum of each keyword in web search frequency. Search users can determine the search scale of a keyword and the spatial distribution of Internet users using the Baidu index (Zhao et al., 2015). In this study, the search volume in the Baidu index of the keywords “climate change,” “global warming,” “greenhouse effect,” “air pollution,” and “haze” is used as the proxy variable for public concern in each year.
Control Variables
Regulation: Government policy usually plays an important role in green innovation. However, the number of government policies alone is insufficient for evaluating this role. The emergence of new energy vehicles primarily deals with environmental problems; thus, environmental regulations reflect the role of the government. At present, most scholars measure environmental regulations from a governance point of view (Brunnermeier and Cohen, 2003; Wu et al., 2011) but with less concern for the effectiveness of the regulations. Ben Kheder and Zugravu-Soilita (2008) used Energy/GDP to measure the extent of environmental regulation. They claimed that the advantage of using this indicator is the capability of measuring the actual impact of the government’s rules and terms on the environment. Given the superiority of the index, this study uses Energy/GDP to measure the degree of environmental regulation; that is, smaller Energy/GDP indicates stricter environmental regulations. GDP refers to the national gross domestic product (unit: billion yuan), and energy is the national energy consumption over the years (unit: million tons of standard coal).
R&D: R&D investment is an important factor in patent output. In the 11 years of the data set, nearly 80% of enterprises have no R&D investment. This phenomenon may be caused by two things. First, a large number of small-scale enterprises lack R&D departments, R&D funds, innovation capability, or demand for technological innovation. Second, some companies do not report the cost of R&D. In this case, it is unable to take the log of R&D input. Thus, use R&D expenditure divided by sales to represent R&D density.
Age: Company age is calculated by
Moreover, enterprise size is classified in China Industry Business Performance Database (CIBPD); specifically, one represents large enterprises, two denotes medium enterprises, and three represents small enterprises.
In addition, state-owned and non-state-owned enterprises are distinguished by verifying H5 (a/b). State-owned enterprises are set to 1, whereas non-state-owned enterprises are set to 0.
Time Division
Multi-angle indicators should be considered when dividing periods using the DILC model. The indicators used in this study include media attention, government concern, vendor response, and patent activity. The sources of these indicators are described as follows.
(1) Media attention: This indicator is represented by the number of newspaper articles related to environmental change. Search titles in the newspaper database of China National Knowledge Infrastructure (http://www.cnki.net/) for news containing the following keywords: “climate change,” “global warming,” “greenhouse effect,” “air pollution,” and “haze.”
(2) Government concern: Use the “advanced search” command in the Peking University Law database (http://www.pkulaw.cn/) to locate full texts of the central laws and regulations that contain at least one of the five keywords mentioned above.
(3) Vendor response: According to automotive media studies in China, vertical websites, where automobile manufacturers publish their announcements, have the greatest impact on new car purchase decisions. This study selects the following four representative vertical sites: Car Home (http://www.autohome.com.cn/), Pacific Car Network (http://www.pcauto.com.cn/), Sina Car (https://auto.sina.com.cn/), and Transaction Car Network (http://www.yiche.com/). The number of articles with topics including at least one of the above-mentioned keywords on different websites each year measures the vendor response.
(4) Patent activity: The data sources and collection procedures of enterprises patent activities, which are introduced specifically in the section of Supplementary Material 3.
A visual examination of the plotted time series provides a quantitatively-based stage division method. Based on a comprehensive investigation (see Supplementary Material 1), this study divided the green innovation period of the automobile industry in China into the following four stages: 2000–2002, 2002–2007, 2007–2012, and 2012–2013. The division follow the way proposed by Penna and Geels (2015), who used a DILC model to research the development of the United States automotive industry. As shown in Supplementary Material 1, four variables demonstrate the similar time trend. These stages serve as the foundation for studying the effect of public concerns on green innovation in different periods.
Data
Information on green information is obtained from Chinese patent database (CPD).
According to seven innovation directions provided by Dechezleprêtre et al. (2015), automotive emission reduction technology and every relevant International Patent Classification (IPC) patent number can be found (See Supplementary Material 2). Then, by searching the IPC from 1985 to 2014 in the CPD this study obtained 27,932 patent data from 3,303 agencies. The total number of patents eligible for green innovation represents the enterprise’s annual innovation statues.
Automobile enterprise characteristic data are obtained from the China Industry Business Performance Database (CIBPD). CIBPD is established by the National Bureau of Statistics, and the data are mainly from sample enterprises’ quarterly and annual reports to the local statistical bureau. Specific methods for screening automotive enterprises as well as the steps for matching data in different databases are described in the Supplementary Material 3. After data collection and matching, 151 automobile enterprises are identified that applied at least once for energy-saving automotive technology between 2003 and 2013.
Empirical Results
Descriptive Analysis
Table 1 presents the descriptive statistics of each variable of 151 automobile enterprises in this study. The maximum number of patents related to car emission reduction is 170, and the minimum is 0, with a mean value of only 2.416, which implies that the green innovation capacity of most companies is very low. The average public concerns is 609.2, which indicates that the average of each enterprise concerned is approximately 609 times and the variance is 438.8. This finding shows considerably different degrees of public concerns.
Table 2 shows that the proportion of companies with innovative behavior increases, which is a positive situation. When enterprises innovate continuously, they become powerful and strong in the market. Accordingly, green innovation in the macro-environment can be accelerated.
Transition Probabilities
Tables 3 shows the transition probabilities of companies of different sizes and different ownership properties.
In terms of persistence (e.g., innovative and non-innovative statuses), the rate of sustained innovative status is highest in large enterprises (67.8%), followed by that in medium enterprises (51.61%), with small enterprises the least (31.82%). Accordingly, sustainability of non-innovative status declines as enterprise scale increases from small to large. Small enterprises present high probability of sustaining their non-innovative status at 73.63%, whereas medium and large companies are at 68.75% and 53.49%, respectively. Considering different ownerships, the rate of sustained innovative status in non-state-owned enterprises (88%) is two times as high as that in state-owned enterprises (44.04%). However, the rate of sustained non-innovative status in non-state-owned enterprises (75%) is also 8% higher than that in state-owned enterprises (67.03%). Accordingly, non-state enterprises exhibit a stronger tendency to sustain their status and continue innovations.
Concerning the liquidity between innovative and non-innovative statuses, the probabilities of innovation for small, medium, and large enterprises are 26.37%, 31.25%, and 46.51%, respectively, based on enterprise scale. Small enterprises exhibit high probability of withdrawing from innovation. Transition probabilities are 68.18%, 48.39%, and 32.2% for small, medium, and large enterprises, respectively. With regard to different ownerships, state-owned enterprises (32.97%) have higher probability of entering innovative status than non-state-owned enterprises (25%). Meanwhile, state-owned enterprises (55.96%) exhibit higher probability of retreating from innovation than non-state-owned enterprises (12%).
When comparing innovation entrance and withdrawal, large companies have higher probability of changing from non-innovative status to innovative status (46.51%), whereas they exhibit low probability of changing from innovative status to non-innovative status (32.2%). Small and medium companies demonstrate the opposite performance, which indicates that small enterprises encounter difficulties when attempting or sustaining green innovations because of limited resources (Geroski et al., 1997). Considering different ownerships, state-owned enterprises have higher probability of entering and withdrawing from innovation than non-state-owned enterprises. This finding shows that state-owned enterprises possess advantages in policy support and financing channels, and they can easily accomplish green innovation (Choi et al., 2011).
Regression Results
Innovations of Different Sizes
This paper uses dynamic panel random probit models, including the innovative status in the previous year, the initial innovative status of companies, and a set of control variables, to measure the impact of public concerns on the green innovation of enterprises. The regression results of different size enterprises, different ownership forms, and different periods are shown in Table 4.
In the models including all companies, the coefficient of innovative status in the previous year
When enterprises are classified by size, strong heterogeneity of innovative status exists. The coefficient of
Innovation of Different Ownerships
This paper primarily analyzes state-owned companies because the sample size of non-state-owned companies is extremely small, and the Rho value of the model is considerably low. The results are presented in Table 4.
For state-owned enterprises, the coefficient of
Phase Analysis
The results of the phase analysis are shown in Table 5. Due to the small sample size in the period 2000–2002, we omitted this period in the specific analysis. In the period of 2007–2012, the coefficient of
The above empirical analysis tests the hypothesis respectively, and the test results are summarized in Table 6.
Robustness Analysis
As previously mentioned, the Baidu index of related keywords can be used to represent public concerns. However, the resulting robustness is sensitive to the appropriate selection of keywords. The impact of variations in keywords and different methods of measuring public concerns should be analyzed to ensure the robustness of the tests. Thus, the following procedure is conducted.
Changing Public Concerns’ Proxy Variable
(1) Using the Baidu index, keywords are replaced by “sewage,” “green,” “energy saving,” “energy consumption,” “emission reduction,” “sustainable,” “new energy,” and “green.” The new empirical results (See Tables 7, 8) are similar to those reported.
(2) The “public environmental awareness” score of China’s public environmental protection livelihood index (state environmental protection administration and China Environmental Culture Promotion Association) is used as an indicator of public concerns. The index surveys public perceptions of food safety, drinking water pollution, air pollution, waste disposal, greening, noise pollution, pollution of rivers and lakes, sustainable development, land pollution, global warming, land desertification, thinning of the ozone layer, species reduction, and 14 other issues using field surveys and telephone surveys. However, the index only includes data obtained from 2005 to 2007. Thus, conduct the analysis with the three-year data for the corresponding years of green innovation of enterprises. Although the model involves lagged variables with a small sample size, the positive impact of public concerns on green innovation and the role of enterprise scale can still be proven (See Table 9). However, the impact of corporate ownership and time heterogeneity cannot be proven because of the lack of data.
(3) According to the World Values Survey (http://www.worldvaluessurvey.org), the use of the 2000–2004, 2005–2009, and 2010–2013 rounds of surveys is related to the issue of environmental awareness in the study; two are selected as representative issues: “Aims of country: Enterprises choice” and “Protecting environment vs. economic growth.” In terms of the small number of environmental awareness issues, this study assigns weights of 50% to each of the two problems, thereby calculating the Chinese public awareness of environmental protection level on the basis of each round of survey per year for the index of environmental awareness. The results are similar to those obtained using the Baidu index; however, the coefficients become large. This result is related to the high scores in the Baidu index (100–several thousand) compared to the much smaller World Values Survey data (typically ranging from 1 to 10) (See Table 9).
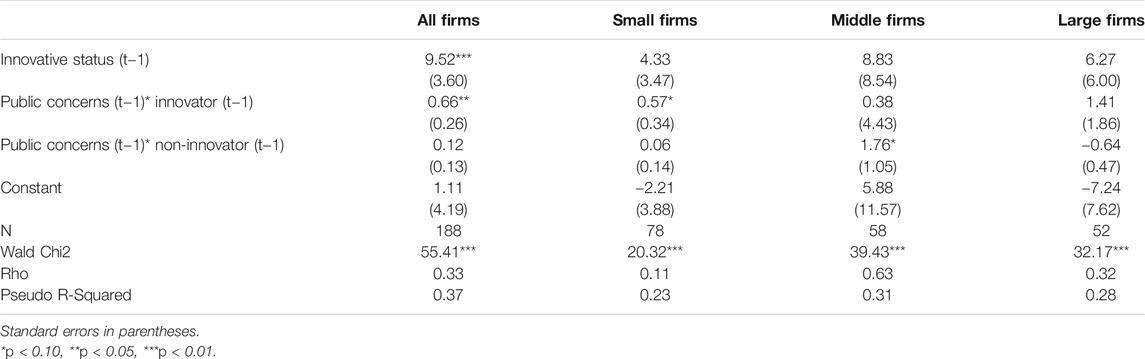
TABLE 7. The effect of enterprise size on green innovation based on Baidu index with keywords replaced.
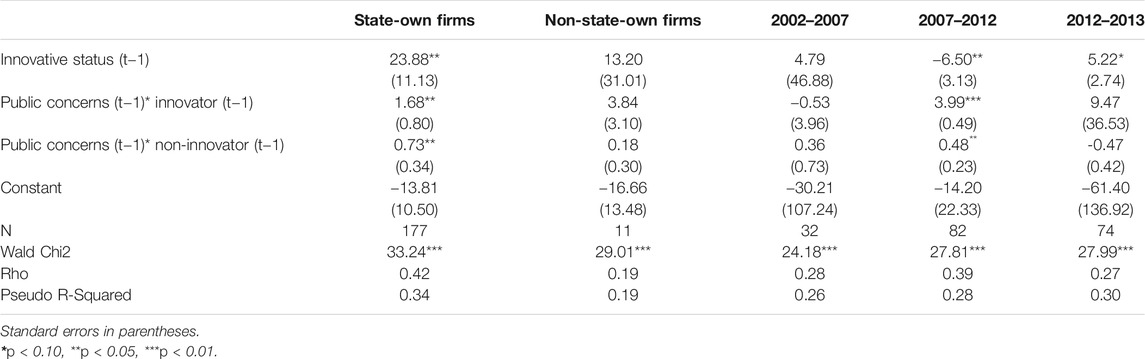
TABLE 8. The effect of ownerships and stages on green innovation based on Baidu index with keywords replaced.
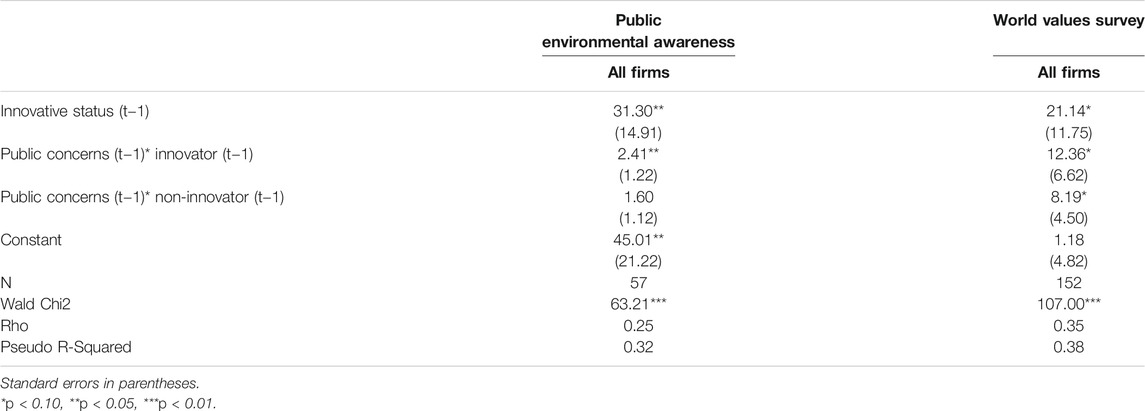
TABLE 9. the effect of public concerns on green innovation based on Public Environmental Awareness data and World Values Survey data.
Using Data of Listed Companies
The research data is mainly sourced for Chinese industrial enterprises databased and most of the enterprises in the database are non-listed companies. Therefore, the use of such data may lead to biased estimation results. In order to test the robustness of the research findings, this study used the data of listed companies for robustness check. The data of listed companies, including patent data and enterprise characteristic data, are sourced from CSMAR database. In this paper, all the 81 automobile manufacturing enterprises data are sourced from the database covering the period between 2003–2013. The statistic description of these companies is shown in Table 10. Then, based on the dynamic panel random probit model, the data of listed companies are empirically analyzed. The specific empirical results are shown in Tables 11, 12. It can be found that for listed companies, the public concerns takes heterogenous effects on innovation depending on the size of enterprise. Public concerns only has a statistically significant effect on the innovation sustainability of large and medium-sized companies, and this effect will not affect small companies. Public concerns has different effects on enterprises with different ownership. Public concerns only has a significant positive effect on the innovation sustainability and the transformation of innovation state of state-owned enterprises. In addition, in different time stages, the role of public concerns is different. Only in the period of 2007–2012, public concerns will significantly promote enterprises to carry out green innovation.
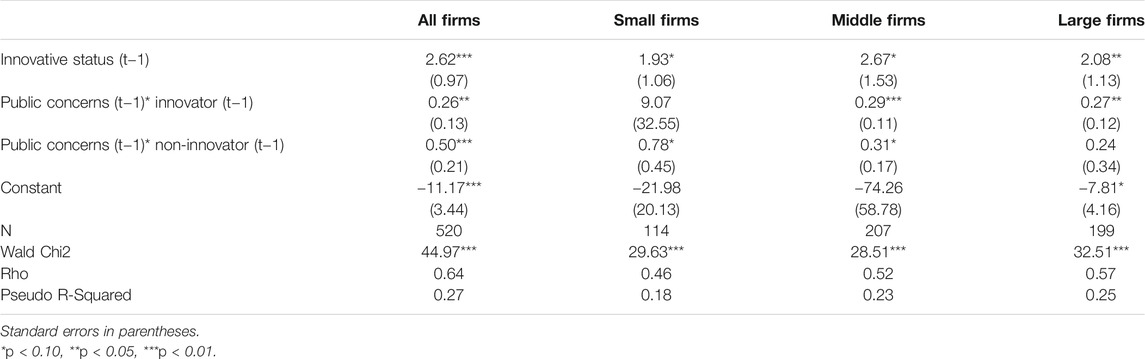
TABLE 11. The effect of public concerns on green innovation of different size of listed automobile enterprises.
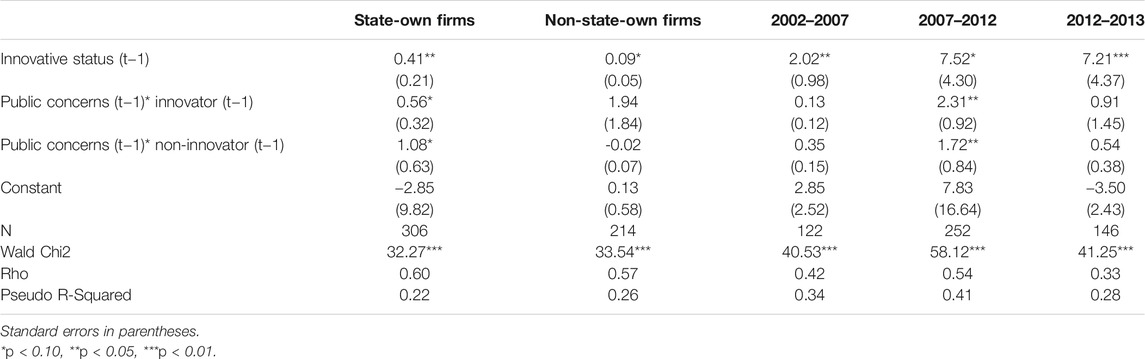
TABLE 12. The effect of public concerns on green innovation of listed automobile enterprises with different ownership and for different time periods.
Discussion and Conclusion
Conclusion
This study analyzes the impact of public concerns on green innovation of Chinese automobile companies and examines whether the effect varies depending on enterprise size, ownership, and time phase.
Firstly, obtain data for 151 automobile enterprises by matching the data of the CIBPD and the CPD. TPM is then used to analyze the sustainability of company innovation. Subsequently, use a dynamic panel random probit model to analyze the impact of public concerns on the green innovation of enterprises. Afterward, use a DILC model to illustrate that the impact of public concerns on green innovation in the automobile industry varies with time.
Based on TPM, which describes the dynamic change in innovation status, the results show that for persistence (e.g., innovative and non-innovative status), innovation sustainability increases as company size increases from small to large. In contrast, the sustainability of non-innovative status decreases as company size increases from small to large. For different ownership forms, non-state-owned companies exhibit higher probability of maintaining their present innovative status than state-owned companies. Specifically, if the enterprise is an innovator (non-innovator) in the previous period, its likelihood of remaining an innovator (non-innovator) in the subsequent period is high with few dynamic changes.
Concerning the liquidity between innovative and non-innovative statuses based on company scale, the probability of becoming innovative increases with increases in company size from small to large. On the contrary, the probability of withdrawing from innovation decreases with an increase in company size from small to large. In terms of ownership, state-owned companies have higher probability of entering or withdrawing from innovative status than non-state-owned companies.
The dynamic panel random probit model is used to analyze the impact of public concerns on green innovation of Chinese automobile companies and examines whether the effect varies depending on enterprise size, ownership, and time phase. The results show the following:
In terms of company scale, the innovative status of medium and large companies is sustainable. Public concerns only affects the continuous innovation of medium and large companies, whereas small companies exhibit low probability of sustained innovation as a result of public concerns. However, public concerns encourages companies of all sizes to experience innovation threshold breakthroughs and green innovation.
For different ownerships, once innovation of state-owned companies begins, their innovative status becomes sustainable. Public concerns can monitor state-owned companies for continuous innovation and enable them to experience breakthroughs and accelerate the pace of green innovation.
Considering different periods, the innovative status of companies is not sustainable in the 2007–2012 period. This situation may be related to the financial crisis. Moreover, the total amount of corporate capital decreases. Thus, the company can no longer innovate in the current period because of the previous period of innovation. Public concerns relaxes the company as it cuts innovation funding and monitors and encourages the company to sustain green innovation. In the periods of 2002–2007 and 2012–2013, the innovative status of companies is continuous, and enterprises’ R&D plays a significant role; however, the role of public concerns is minimal.
Public Policy Implications
About the green transformation of automobile industry in China, the result of this paper reveals that it has not been conducted for most enterprises, no matter divided by scale (26.37% of small businesses, 31. 25% of medium-sized and 46. 51% of large-scale enterprises), or by ownership (25% of non-state-owned enterprises and 32.97% of state-owned enterprises). For this situation, first, government shall realize the severe situation, and there is a long way to go for the greenization of the automobile industry in China. The enterprises’ green innovation can be encouraged or promoted through measures such as government procurement, subsidies and stronger regulation etc. Second, government should realize the differences in enterprises’ size and ownership during making policies, and more supporting policies shall be given to small enterprises and non-nationalized enterprises which with low green transformation probability. Third, public concerns is a very important driving force for enterprises’ green innovation. Therefore, the government can strengthen the public’s environmental awareness through various methods, and promote the enterprise's green transformation through external pressure.
About the innovation persistence, 68.18% of small enterprises will quit innovation even if they had green innovation in last time phase. It shows that it is not easy to maintain an innovative state for them. Innovation requires a high demand for R&D capability and fund support, but both of them are great disadvantages for small enterprises. Therefore, the government can play the positive role in personnel training, employment policy making, technology platform construction etc., and try to solve the problem of difficult financing for small enterprises.
Managerial Implications
The findings can be used by enterprises to consider how public concerns may impact their business decisions about enterprise activities, such as public concerns will facilitate the green transformation of enterprises. Furthermore, it will reinforce persistent innovation for large and medium enterprises, which demonstrates that the public has potential power to affect enterprise behavior.
Currently, environment issues are the core factors for the competitiveness in product markets (McDonagh and Prothero, 2014). This research is capable of assisting managers to exploit the implements, so as to acquire the competitive advantage of the market. For instance, the enterprise can take actions as the followings:
(1) The empirical results show that public attention is the key driving force to promote green innovation in enterprises. Meanwhile, according to the TPB theory referred in this paper, public concerns about green innovative activities often indicates a potential demand for green products. Therefore, before making a decision, the enterprise can have a good command of the development tendency of public opinions by virtue of online forum, Weibo as well as other social media, from which it can obtain the public opinions, reaching an agreement with the public before making a decision. What the enterprise observes for the perception of the public provides an opportunity of taking the public aspiration and the public concerns into consideration in the early stage of innovation and development, so as to bring the most potential benefits to the users in the future, acquiring the competitive advantage.
(2) However, according to the conclusion of this study, the impact of public concerns on automobile enterprises of different sizes and ownerships varies. The innovation of large and middle-sized enterprises and state-owned enterprises is more sustainable, which may be due to their stronger ability to bear innovation failures. If these enterprises want to be more competitive in the future market, they should analyze the public concerns to predict the possible green development trend of the industry and invest in innovation of this regard. For small enterprises and non-state-owned enterprises, green innovation is less sustainable, which may be related to financial constraints. Therefore, the main objective of such enterprises is to imitate existing green products, which can help them survive in the market by coping with increasingly strict environmental policies at a lower cost of innovation.
Limitations and Future Research
Although this paper comprehensively analyzes the impact of public concerns on the green innovation of enterprises, some limitations still exist.
Frist, public is a comprehensive concept. Future research should classify the public. For example, the public can be divided into corporate stakeholders (investors, creditors, suppliers, customers, etc.) and general public with no corporate interests and study the influencing mechanism of different actors on green innovation of enterprises.
Second, due to the data accessibility restrictions and using IPC of patent to identify “green” innovation, patents are used as indicators of innovation. But it is questionable. After viewing other literature, it is obvious to find that “eco-labeling product certification” as an index of “Green product innovation” (Lin et al., 2014), “ISO 14001 certification” is used as an index for “Green process innovation” (Lin et al., 2014) in some articles on measure “Green Innovation” and take other industries as research objects. But these data are not available in the automobile industry database. However, it is possible to use it in other industries in the future.
Data Availability Statement
The original contributions presented in the study are included in the article/Supplementary Material, further inquiries can be directed to the corresponding author.
Author Contributions
Writing and method: YL. Data and review: ZW.
Conflict of Interest
The authors declare that the research was conducted in the absence of any commercial or financial relationships that could be construed as a potential conflict of interest.
Supplementary Material
The Supplementary Material for this article can be found online at: https://www.frontiersin.org/articles/10.3389/fenrg.2021.623638/full#supplementary-material.
Acknowledgments
The authors are grateful for the financial support from the MOE (Ministry of Education in China) Project of Humanities and Social Sciences (No.18YJC840041), National Natural Science Foundation of China (NO. 71904009), the Postdoctoral Science Foundation of China (NO. 2019M652415), Young Scholars Program of Shandong University, Weihai, and Scientific Research Fund for Graduate Students of Business School of Shandong University. Besides, the authors also thank the anonymous reviewers for insightful comments that helped us improve the quality of the paper.
References
Ajzen, I. (1985). From intentions to actions: a theory of planned behavior, Action control. Berlin, Germany: Springer, 11–39.
Ajzen, I. (1991). The theory of planned behavior. Organ. Behav. Hum. Decis. Process. 50, 179–211. doi:10.1016/0749-5978(91)90020-t
Ayllón, S., and Radicic, D. (2019). Product innovation, process innovation and export propensity: persistence, complementarities and feedback effects in Spanish firms. Appl. Econ. 51, 3650–3664. doi:10.1080/00036846.2019.1584376
Ben Kheder, S., and Zugravu-Soilita, N. (2008). The pollution haven hypothesis: a geographic economy model in a comparative study. FEEM Working. 73. doi:10.2139/ssrn.1266705
Berrone, P., Fosfuri, A., Gelabert, L., and Gomez-Mejia, L. R. (2013). Necessity as the mother of 'green' inventions: institutional pressures and environmental innovations. Strat. Mgmt. J. 34, 891–909. doi:10.1002/smj.2041
Blackman, A., and Bannister, G. J. (1998). Community pressure and clean technology in the informal sector: an econometric analysis of the adoption of propane by traditional Mexican brickmakers. J. Environ. Econ. Manag. 35, 1–21. doi:10.1006/jeem.1998.1019
Brunnermeier, S. B., and Cohen, M. A. (2003). Determinants of environmental innovation in United States manufacturing industries. J. Environ. Econ. Manag. 45, 278–293. doi:10.1016/s0095-0696(02)00058-x
Cai, B., Cao, D., Liu, L., Zhou, Y., and Zhang, Z. (2011). China transport CO2 emission study. Adv. Clim. Change Res. 3, 197–203.
Cefis, E. (2003). Is there persistence in innovative activities? Int. J. Ind. Organ. 21, 489–515. doi:10.1016/s0167-7187(02)00090-5
Cefis, E., and Marsili, O. (2015). Crossing the innovation threshold through mergers and acquisitions. Res. Pol. 44, 698–710. doi:10.1016/j.respol.2014.10.010
Chao, Y.-L. (2012). Predicting people's environmental behaviour: theory of planned behaviour and model of responsible environmental behaviour. Environ. Educ. Res. 18, 437–461. doi:10.1080/13504622.2011.634970
Chen, Y.-S. (2008). The driver of green innovation and green image - green core competence. J. Bus. Ethics. 81, 531–543. doi:10.1007/s10551-007-9522-1
Choi, S. B., Lee, S. H., and Williams, C. (2011). Ownership and firm innovation in a transition economy: evidence from China. Res. Pol. 40, 441–452. doi:10.1016/j.respol.2011.01.004
Clausen, T., Pohjola, M., Sapprasert, K., and Verspagen, B. (2012). Innovation strategies as a source of persistent innovation. Ind. Corp. Change. 21, 553–585. doi:10.1093/icc/dtr051
Cramer, J. (2005). Experiences with structuring corporate social responsibility in Dutch industry. J. Clean. Prod. 13, 583–592. doi:10.1016/j.jclepro.2003.12.008
Cristina De Stefano, M., Montes-Sancho, M. J., and Busch, T. (2016). A natural resource-based view of climate change: innovation challenges in the automobile industry. J. Clean. Prod. 139, 1436–1448. doi:10.1016/j.jclepro.2016.08.023
Dechezleprêtre, A., Neumayer, E., and Perkins, R. (2015). Environmental regulation and the cross-border diffusion of new technology: evidence from automobile patents. Res. Pol. 44, 244–257. doi:10.1016/j.respol.2014.07.017
Fellenor, J., Barnett, J., Potter, C., Urquhart, J., Mumford, J. D., and Quine, C. P. (2020). 'Real without being concrete': the ontology of public concern and its significance for the social amplification of risk framework (SARF). J. Risk Res. 23, 20–34. doi:10.1080/13669877.2018.1501598
Fielding, K. S., McDonald, R., and Louis, W. R. (2008). Theory of planned behaviour, identity and intentions to engage in environmental activism. J. Environ. Psychol. 28, 318–326. doi:10.1016/j.jenvp.2008.03.003
Freeman, C. (1995). The ‘national system of innovation’ in historical perspective. Camb. J. Econ. 19, 5–24.
Fu, R., Jin, G., Chen, J., and Ye, Y. (2021). The effects of poverty alleviation investment on carbon emissions in China based on the multiregional input-output model. Technol. Forecast. Soc. Change. 162, 120344. doi:10.1016/j.techfore.2020.120344
Garde Sánchez, R., Rodríguez Bolívar, M. P., and López Hernández, A. M. (2017). Corporate and managerial characteristics as drivers of social responsibility disclosure by state-owned enterprises. Rev Manag Sci. 11, 633–659. doi:10.1007/s11846-016-0199-7
Geels, F. W. (2010). Ontologies, socio-technical transitions (to sustainability), and the multi-level perspective. Res. Pol. 39, 495–510. doi:10.1016/j.respol.2010.01.022
Geels, F. W., and Penna, C. C. R. (2015). Societal problems and industry reorientation: elaborating the dialectic issue lifecycle (DILC) model and a case study of car safety in the United States (1900-1995). Res. Pol. 44, 67–82. doi:10.1016/j.respol.2014.09.006
Geroski, P. A., Van Reenen, J., and Walters, C. F. (1997). How persistently do firms innovate? Res. Pol. 26, 33–48. doi:10.1016/s0048-7333(96)00903-1
Ghisetti, C., Mancinelli, S., Mazzanti, M., and Zoli, M. (2017). Financial barriers and environmental innovations: evidence from EU manufacturing firms. Clim. Pol. 17, S131–S147. doi:10.1080/14693062.2016.1242057
Gohoungodji, P., N'Dri, A. B., Latulippe, J.-M., and Matos, A. L. B. (2020). What is stopping the automotive industry from going green? a systematic review of barriers to green innovation in the automotive industry. J. Clean. Prod. 277, 123524. doi:10.1016/j.jclepro.2020.123524
Gupta, H., and Barua, M. K. (2018). A framework to overcome barriers to green innovation in SMEs using BWM and fuzzy topsis. Sci. Total Environ. 633, 122–139. doi:10.1016/j.scitotenv.2018.03.173
Hao, Z., Qi, W., Gong, T., Chen, L., and Shen, Z.-J. M. (2019). Innovation uncertainty, new product press timing and strategic consumers. Omega. 89, 122–135. doi:10.1016/j.omega.2018.09.011
Henry, G. T., and Gordon, C. S. (2001). Tracking issue attention: specifying the dynamics of the public agenda. Publ. Opin. Q. 65, 157–177. doi:10.1086/322198
Hu, C., Mao, J., Tian, M., Wei, Y., Guo, L., and Wang, Z. (2020). Distance matters: investigating how geographic proximity to ENGOs triggers green innovation of heavy-polluting firms in China. J. Environ. Manag. 279, 111542. doi:10.1016/j.jenvman.2020.111542
Huergo, E., and Jaumandreu, J. (2004). Firms' age, process innovation and productivity growth. Int. J. Ind. Organ. 22, 541–559. doi:10.1016/j.ijindorg.2003.12.002
Kahn, M. E. (2007). Do greens drive Hummers or hybrids? Environmental ideology as a determinant of consumer choice. J. Environ. Econ. Manag. 54, 129–145. doi:10.1016/j.jeem.2007.05.001
Kesidou, E., and Demirel, P. (2012). On the drivers of eco-innovations: empirical evidence from the United Kingdom. Res. Pol. 41, 862–870. doi:10.1016/j.respol.2012.01.005
Kostka, G., Moslener, U., and Andreas, J. (2013). Barriers to increasing energy efficiency: evidence from small-and medium-sized enterprises in China. J. Clean. Prod. 57, 59–68. doi:10.1016/j.jclepro.2013.06.025
Leal-Rodríguez, A. L., Ariza-Montes, A. J., Morales-Fernández, E., and Albort-Morant, G. (2018). Green innovation, indeed a cornerstone in linking market requests and business performance. Evidence from the Spanish automotive components industry. Technol. Forecast. Soc. Change. 129, 185–193. doi:10.1016/j.techfore.2017.07.021
Leonidou, L. C., Christodoulides, P., Kyrgidou, L. P., and Palihawadana, D. (2017). Internal drivers and performance consequences of small firm green business strategy: the moderating role of external forces. J. Bus. Ethics. 140, 585–606. doi:10.1007/s10551-015-2670-9
Li, Y., Du, Q., Lu, X., Wu, J., and Han, X. (2019). Relationship between the development and CO2 emissions of transport sector in China. Transport. Res. Transport Environ. 74, 1–14. doi:10.1016/j.trd.2019.07.011
Liao, W.-W. (2017). A study on the correlations among environmental education, environment-friendly product development, and green innovation capability in an enterprise. Eurasia J. Math. Sci. Technol. Educ 13, 5435–5444. doi:10.12973/eurasia.2017.00841a
Lin, H., Zeng, S. X., Ma, H. Y., Qi, G. Y., and Tam, V. W. Y. (2014). Can political capital drive corporate green innovation? Lessons from China. J. Clean. Prod. 64, 63–72. doi:10.1016/j.jclepro.2013.07.046
Lin, W.-L., Cheah, J.-H., Azali, M., Ho, J. A., and Yip, N. (2019). Does firm size matter? Evidence on the impact of the green innovation strategy on corporate financial performance in the automotive sector. J. Clean. Prod. 229, 974–988. doi:10.1016/j.jclepro.2019.04.214
Liu, K., Xue, M., Peng, M., and Wang, C. (2020a). Impact of spatial structure of urban agglomeration on carbon emissions: an analysis of the Shandong Peninsula, China. Technol. Forecast. Soc. Change. 161, 120313. doi:10.1016/j.techfore.2020.120313
Liu, L., Zhang, T., Avrin, A.-P., and Wang, X. (2020b). Is China's industrial policy effective? An empirical study of the new energy vehicles industry. Technol. Soc. 63, 101356. doi:10.1016/j.techsoc.2020.101356
Loo, B. P. Y., and Li, L. (2012). Carbon dioxide emissions from passenger transport in China since 1949: implications for developing sustainable transport. Energy Pol. 50, 464–476. doi:10.1016/j.enpol.2012.07.044
Lu, J., Li, B., Li, H., and Al-Barakani, A. (2021). Expansion of city scale, traffic modes, traffic congestion, and air pollution. Cities 108, 102974. doi:10.1016/j.cities.2020.102974
Lv, Q., Liu, H., Yang, D., and Liu, H. (2019). Effects of urbanization on freight transport carbon emissions in China: common characteristics and regional disparity. J. Clean. Prod. 211, 481–489. doi:10.1016/j.jclepro.2018.11.182
McCombs, , and Zhu, J.-H. (1995). Capacity, diversity, and volatility of the public agenda: trends from 1954 to 1994. Publ. Opin. Q. 59, 495–525. doi:10.1086/269491
McDonagh, P., and Prothero, A. (2014). Sustainability marketing research: past, present and future. J. Market. Manag. 30, 1186–1219. doi:10.1080/0267257x.2014.943263
McWilliams, A., and Siegel, D. (2000). Corporate social responsibility and financial performance: correlation or misspecification? Strat. Mgmt. J. 21, 603–609. doi:10.1002/(sici)1097-0266(200005)21:5<603::aid-smj101>3.0.co;2-3
Meng, Q., Wang, Y., Zhang, Z., and He, Y. (2020). Supply chain green innovation subsidy strategy considering consumer heterogeneity. J. Clean. Prod. 281, 125199. doi:10.1016/j.jclepro.2020.125199
Mowery, D., and Rosenberg, N. (1979). The influence of market demand upon innovation: a critical review of some recent empirical studies. Res. Pol. 8, 102–153. doi:10.1016/0048-7333(79)90019-2
Olson, E. L. (2013). Perspective: the green innovation value chain: a tool for evaluating the diffusion prospects of green products. J. Prod. Innovat. Manag. 30, 782–793. doi:10.1111/jpim.12022
Penna, C. C. R., and Geels, F. W. (2015). Climate change and the slow reorientation of the American car industry (1979-2012): an application and extension of the dialectic issue lifecycle (DILC) model. Res. Pol. 44, 1029–1048. doi:10.1016/j.respol.2014.11.010
Penna, C. C. R., and Geels, F. W. (2012). Multi-dimensional struggles in the greening of industry: a dialectic issue lifecycle model and case study. Technol. Forecast. Soc. Change. 79, 999–1020. doi:10.1016/j.techfore.2011.09.006
Qi, G., Jia, Y., and Zou, H. (2021). Is institutional pressure the mother of green innovation? Examining the moderating effect of absorptive capacity. J. Clean. Prod. 278, 123957. doi:10.1016/j.jclepro.2020.123957
Raymond, W., Mohnen, P., Palm, F., and van der Loeff, S. S. (2010). Persistence of innovation in Dutch manufacturing: is it spurious? Rev. Econ. Stat. 92, 495–504. doi:10.1162/rest_a_00004
Revell, A., Stokes, D., and Chen, H. (2010). Small businesses and the environment: turning over a new leaf? Bus. Strat. Environ. 19, 273–288. doi:10.1002/bse.628
Ripberger, J. T. (2011). Capturing curiosity: using Internet search trends to measure public attentiveness. Pol. Stud. J. 39, 239–259. doi:10.1111/j.1541-0072.2011.00406.x
Rugman, A. M., and Verbeke, A. (1998). Corporate strategies and environmental regulations: an organizing framework. Strat. Mgmt. J. 19, 363–375. doi:10.1002/(sici)1097-0266(199804)19:4<363::aid-smj974>3.0.co;2-h
Schiederig, T., Tietze, F., and Herstatt, C. (2012). Green innovation in technology and innovation management-an exploratory literature review. R. Manag. 42, 180–192. doi:10.1111/j.1467-9310.2011.00672.x
Sillak, S., and Kanger, L. (2020). Global pressures vs. local embeddedness: the de-and restabilization of the Estonian oil shale industry in response to climate change (1995-2016). Enviro. Inno. Soc. Trans. 34, 96–115. doi:10.1016/j.eist.2019.12.003
Solaymani, S. (2019). CO2 emissions patterns in 7 top carbon emitter economies: the case of transport sector. Energy. 168, 989–1001. doi:10.1016/j.energy.2018.11.145
Song, Y., Zhang, M., and Shan, C. (2019). Research on the decoupling trend and mitigation potential of CO2 emissions from China’s transport sector. Energy 183, 837–843. doi:10.1016/j.energy.2019.07.011
Tarnawska, K. (2013). Eco-innovations–tools for the transition to green economy. Econ. Manag. 18, 735–743. doi:10.5755/j01.em.18.4.4485
Triguero, Á., and Córcoles, D. (2013). Understanding innovation: an analysis of persistence for Spanish manufacturing firms. Res. Pol. 42, 340–352. doi:10.1016/j.respol.2012.08.003
Wlezien, C. (2005). On the salience of political issues: the problem with 'most important problem'. Elect. Stud. 24, 555–579. doi:10.1016/j.electstud.2005.01.009
Wooldridge, J. M. (2005). Simple solutions to the initial conditions problem in dynamic, nonlinear panel data models with unobserved heterogeneity. J. Appl. Econ. 20, 39–54. doi:10.1002/jae.770
Wu, R., Zhang, S., Yu, D. W., Zhao, P., Li, X., Wang, L., et al. (2011). Effectiveness of China's nature reserves in representing ecological diversity. Front. Ecol. Environ. 9, 383–389. doi:10.1890/100093
Yazdanpanah, M., and Forouzani, M. (2015). Application of the Theory of Planned Behaviour to predict Iranian students' intention to purchase organic food. J. Clean. Prod. 107, 342–352. doi:10.1016/j.jclepro.2015.02.071
Yu, W., and Han, R. (2019). The impact of pollution taxation on competitive green product choice strategies. Comput. Ind. Eng. 137, 106012. doi:10.1016/j.cie.2019.106012
Zeng, S. X., Meng, X. H., Zeng, R. C., Tam, C. M., Tam, V. W. Y., and Jin, T. (2011). How environmental management driving forces affect environmental and economic performance of SMEs: a study in the Northern China district. J. Clean. Prod. 19, 1426–1437. doi:10.1016/j.jclepro.2011.05.002
Zhang, B., Yang, S., and Bi, J. (2013). Enterprises' willingness to adopt/develop cleaner production technologies: an empirical study in Changshu, China. J. Clean. Prod. 40, 62–70. doi:10.1016/j.jclepro.2010.12.009
Zhao, Y., Gao, X., and Jiang, B. (2015). The urban network connection of three provinces in northeast China based on Baidu Index. Econ. Geogr. 35, 32–37. doi:10.13249/j.cnki.sgs.2019.07.011
Keywords: green innovation, public concerns, innovation transition, innovation persistence, China’s automotive industry, large-scale web search data
Citation: Li Y and Wang Z (2021) Will Public Environmental Concerns Foster Green Innovation in China’s Automotive Industry? An Empirical Study Based on Multi-Sourced Data Streams. Front. Energy Res. 9:623638. doi: 10.3389/fenrg.2021.623638
Received: 30 October 2020; Accepted: 06 January 2021;
Published: 19 February 2021.
Edited by:
Bai-Chen Xie, Tianjin University, ChinaReviewed by:
Xingping Zhang, North China Electric Power University, ChinaZhan-Ming Chen, Renmin University of China, China
Copyright © 2021 Li and Wang. This is an open-access article distributed under the terms of the Creative Commons Attribution License (CC BY). The use, distribution or reproduction in other forums is permitted, provided the original author(s) and the copyright owner(s) are credited and that the original publication in this journal is cited, in accordance with accepted academic practice. No use, distribution or reproduction is permitted which does not comply with these terms.
*Correspondence: Zhicheng Wang, 201916299@mail.sdu.edu.cn