- 1Institute of Technical Thermodynamics, RWTH Aachen University, Aachen, Germany
- 2Institute of Energy and Climate Research—Energy Systems Engineering (IEK-10), Forschungszentrum Jülich GmbH, Jülich, Germany
- 3Energy and Process Systems Engineering, Department of Mechanical and Process Engineering, ETH Zürich, Zürich, Switzerland
National energy models provide decarbonization strategies. Most national energy models focus on costs and greenhouse gas emissions only. However, this focus carries the risk that burdens shift to other environmental impacts. Energy models have therefore been extended by life-cycle assessment (LCA). Furthermore, deep decarbonization is only possible by targeting all high-emission sectors. Thus, we present a holistic national energy model that includes high-emission sectors and LCA. The model provides detailed environmental impacts for electricity, heat, and transport processes in Germany for meeting the climate targets up to 2050. Our results show that renewable energies and storage are key technologies for decarbonized energy systems. Furthermore, sector coupling is crucial and doubles electricity demand. Our LCA shows that environmental impacts shift from operation to infrastructure highlighting the importance of an impact assessment over the full life cycle. Decarbonization leads to many environmental cobenefits; however, it also increases freshwater ecotoxicity and depletion of metal and mineral resources. Thus, holistic planning of decarbonization strategies should also consider other environmental impacts.
Highlights
Sector-coupled national energy system integrating life-cycle assessment.
Transition path in Germany until 2050 for a greenhouse gas reduction target of 85%.
Renewable energies, storage, and sector-coupling are the key for a low-carbon economy.
There are many cobenefits of decarbonization, but 5 out of 16 impacts increase.
Considering solely greenhouse gas emissions shifts burden to other impacts.
1 Introduction
Climate change mitigation in line with the 1.5°C goal requires a massive reduction of greenhouse gas (GHG) emissions (IPCC, 2018). Until now, reduction measures mainly focus on the electricity sector through the integration of low-carbon technologies. However, electricity production accounts only for approximately 40% of GHG emissions in the G20 countries (Olivier et al., 2017), 25% in EU-28 (Eurostat, 2018), and 38% in Germany (Federal Ministry for the Environment, 2018). Thus, GHG emissions can only be massively reduced by also targeting other high-emission sectors, such as heating and transportation (Ruhnau et al., 2019).
In low-carbon energy systems, the sectors electricity, heat, and transportation are interconnected via electricity. This sector coupling makes the development of a decarbonization strategy challenging (DeCarolis et al., 2017). To capture this challenge, decarbonization strategies can be developed based on national energy system models (Mirakyan and de Guio, 2015).
Decarbonization strategies created with national energy system models envision a vast expansion of low-carbon technologies. Although these low-carbon technologies perform well regarding direct GHG emissions, they lead to indirect GHG emissions due to construction (McDowall et al., 2018) and might induce other environmental impacts, such as ecotoxicity and resource depletion (Rauner and Budzinski, 2017; Algunaibet and Guillén-Gosálbez, 2019). Thus, employing low-carbon technologies for GHG reductions may shift burden to other environmental impacts (Algunaibet and Guillén-Gosálbez, 2019). As GHG emissions reduce, these other environmental impacts gain more importance (Rauner and Budzinski, 2017).
To consider other environmental impacts, energy system models have recently been extended by life-cycle assessments (LCAs) (Ringkjøb et al., 2018). For example, Turconi et al. (2014) investigated the environmental impacts of the Danish electricity sector for different scenarios of heat and power cogeneration and biomass. Kouloumpis et al. (2015) assessed environmental impacts for 16 scenarios for the United Kingdom electricity sector up to 2070. Berrill et al. (2016) presented an LCA of 44 European electricity scenarios, from conventional energy systems using carbon capture and storage and systems based mostly on renewable generation. García-Gusano et al. (2016) calculated environmental impacts of a business-as-usual scenario for the electricity sector of Norway up to 2050. Rauner and Budzinski (2017) used a multiobjective optimization to include environmental impacts in an expansion problem of the German electricity system. Algunaibet and Guillén-Gosálbez (2019) used a multiobjective optimization to quantify the burden-shifting potential of GHG emission reductions of the electricity system of the United States.
These energy system models provide a comprehensive view on environmental impacts by including LCA, but they focus on the electricity sector only. Thus, cross-sectoral interconnectivity is neglected.
Volkart et al. (2017) combined a cross-sectoral energy system model with LCA to evaluate three scenarios for the Swiss energy transition. The used energy system model, however, considers no spatial resolution, i.e., no electricity grid, and only low time-resolution with six time steps. Recently, Blanco et al. (2020) performed ex-post LCA of the JRC-EU-TIMES model, including multiple sectors; however, the LCA is not part of the optimization. Also worth mentioning is the work of (Gençer et al., 2020), in which they developed the SESAME-tool to evaluate and compare energy transition pathways; however, SESAME does not include optimization. In the work of Li et al. (2020), they developed a new modeling approach to optimize the carbon loop of biomass and carbon capture and storage for the carbon neutrality of Switzerland in 2050. However, Li et al. (2020) focus on climate change and do not include an holistic LCA.
Lopion et al. (2018) reviewed energy system models and concluded that four elements are required to provide holistic insight for national GHG reduction strategies: 1) cross-sectoral technologies (Pregger et al., 2013; Lopion et al., 2018); 2) high temporal resolution (Lopion et al., 2018; Mallapragada et al., 2018; Victoria et al., 2019); 3) high spatial resolution (Haller et al., 2012; Lopion et al., 2018; Victoria et al., 2019); and 4) evaluating environmental impacts holistically, for which LCA is best suited (Lopion et al., 2018; Ringkjøb et al., 2018). Most energy models reviewed by Lopion et al. (2018) aim only for cost-optimal GHG reduction strategies but do not consider other environmental impacts (Spittler et al., 2019), e.g., REMIX (Gils et al., 2017), TIMES (Loulou et al., 2016), and OSeMOSYS (Howells et al., 2011).
A model is required that includes all four elements to derive optimal pathways to reduce GHG emissions while considering other environmental impacts.
In Section 2, we propose the sector-coupled energy system model SecMOD to optimize the transition of a national energy system providing all four required elements: cross-sectoral technologies, high spatial and temporal resolution, and holistic environmental assessment by LCA. The model includes the sectors with most GHG emissions (Federal Ministry for the Environment, 2018): electricity, heat, and private transportation.
We compute environmental impacts for electricity, heat, and private transportation for the optimal, sector-coupled energy transition in Germany for the period 2020–2050 for the German GHG reduction goal of 85% until 2050 compared to 1990, Section 3. We show that considering cross-sectoral interconnectivity increases burden shifting significantly compared to previous studies examining only the electricity sector (Turconi et al., 2014; Rauner and Budzinski, 2017). The computed environmental impacts provide comprehensive life cycle inventories (LCIs) for electricity, heat, and transportation. In Section 4, we summarize and conclude.
2 Methodology of SecMOD
The sector-coupled energy system model (SecMOD), established in this article, calculates the cost-optimal transition of a national energy system under GHG constraints. For a holistic evaluation of resulting energy systems, SecMOD incorporates LCA using the categories of the Environmental Footprint 2.0 life-cycle impact assessment (LCIA) (Castellani et al., 2018). Here, we parameterize SecMOD for the German energy transition from 2016 until 2050.
In Section 2.1, we give a concise overview of SecMOD. In Section 2.2, we present the LCA integrated into SecMOD. The detailed model formulation is given in Supplementary Material.
2.1 Modeling of the Sector-Coupled Energy System
SecMOD computes a transition path toward a low-carbon energy system by an economic optimization satisfying GHG-reduction targets. SecMOD is formulated as a linear programming (LP) problem in GAMS (GAMS, 2016) and solved with CPLEX 12.9.0.0 (IBM, 2016).
In SecMOD, the energy system is locally resolved by nodes. At each node and time step, demands need to be fulfilled. In the current version of SecMOD, the demands are electricity, centralized and decentralized heating on four temperature levels (domestic heating, and industrial heat below 100°C, between 100–400°C, and above 400°C), and private short- and long-distant transportation. The electricity demand is based on Egerer (2016); the heating demand is based for households on Federal Statistical Office (2015) and industry on Öko-Institut and Fraunhofer ISI (2015); the demand for private transportation is taken from Federal Motor Transport Authority (2017). We assume constant demands for electricity and heating, besides sector-coupling technologies. The heating demand can be reduced by thermal insulation. For the transportation demand, we assume a slight increase till 2030 and then a constant demand. The detailed demand structure is given in Supplementary Material.
2.1.1 Technology and Model Overview
To fulfill the demands, SecMOD can choose between various technologies (sector-coupling technologies are highlighted in bold-italic). For private transportation, SecMOD includes the following vehicle technologies:
diesel (and synthetic diesel), gasoline, natural gas (and synthetic methane), battery electric, plug-in hybrids, and fuel cells.
For centralized and decentralized heating, SecMOD includes the following technologies:
domestic thermal insulation, electrode boilers, gas boilers, oil boilers, heat pumps, and heat cogeneration from power plants.
For electricity demand, SecMOD includes the following technologies:
biomass plants, combined-cycle power plants, coal plants, gas plants, geothermal generation, H2-electrolysis with H2-fuel cell, lignite plants, lithium-ion batteries, nuclear plants, oil plants, power-to-methane, power-to-diesel, pumped-hydro-storage plants, run-of-river power plants, photovoltaics, transmission grids, waste incineration plants, and wind (onshore and offshore).
The primary data source for technology parameters, such as costs, is German Energy Agency (2018); all data sources are given in Supplementary Material. Climate change itself can affect the performance of technologies in a positive or negative manner (Cronin et al., 2018). This effect is not reflected in our model. For our model scope in central Europe, the review of Cronin et al. (2018) suggests that the impact of climate change should be the strongest for bioenergy, which, however, is not expandable in our model. For investment costs and efficiencies, projections of future development are implemented, to model cost degression for specific technologies; see Supplementary Material. Time series for renewable generation and conventional availability are taken from Egerer (2016). The potential for renewable generation, capacities for onshore wind, offshore wind, and roof-top photovoltaics is regionally limited. Regional limits are included for onshore wind energy with values based on McKenna et al. (2015), for offshore wind on Gerhardt et al. (2015), and for photovoltaics on Mainzer et al. (2014). The limits are based on available area for each municipality considering political restrictions. The resulting overall limits are 698 GWon onshore wind, 36 GWof offshore wind, and 235 GWpv PV. The overall limits are stated as maximal installed nominal capacity.
The power sector includes the high-voltage transmission grid based on ELMOD-DE from 2012 (Egerer, 2016) and is updated to 2016. The German transmission grid is modeled by 416 nodes and 661 power lines. Following ELMOD-DE, SecMOD represents network flows with the DC-load-flow approximation by Overbye et al. (2004), which linearizes the AC-power flow. For this study, we spatially aggregate the nodes to 18 regions proposed by the German Energy Agency, Dena Grid Study II (2010). For grid expansion, power lines can be upgraded to a higher voltage level or new power lines can be installed in parallel.
As objective, we minimize the total annualized costs assuming a discount rate of 5% as in the JRC-EU-TIMES model (Simoes et al., 2013). Investment costs in technologies are annualized over their economic lifetime also with an interest rate of 5%; see Supplementary Material. The economic objective is minimized being subject to GHG reductions taken from Federal Ministry for the Environment (2018). Besides climate impact, 15 further environmental impacts are assessed, but not constrained.
System boundaries include German sectors for power, heating, and private vehicles which account for 77% (690 Mt CO2-eq) of the total GHG emissions in 2016. Therefore, we scale the GHG-reduction targets by 77%. Thus, GHG emissions are reduced by 85% compared to 1990 (966 Mt CO2-eq) until absolute emissions reach 144 Mt CO2-eq in 2050.
For GHG-reduction targets, we consider operational climate impacts; see Section 2.2. The operational climate impacts comprise direct CO2-eq emissions from flue-gas stacks and exhausts. In addition, we include indirect upstream emissions from fuel supply, e.g., mining of energy carriers. Current policies for emission reductions only target direct emissions. However, both direct and indirect emissions need to be constrained to cover the entire climate impact of operation (Lopion et al., 2018). Thus, our emission targets are more ambitious than current policies as we incorporate the whole operational impact by life-cycle thinking.
2.1.2 Transition Path via a Rolling Horizon Approach
To calculate the transition path till 2050, SecMOD is modeled as a multiperiod problem considering eight consecutive investment periods with a horizon of five years each, in line with state-of-the-art energy system models (Lopion et al., 2018).
In general, transition paths can be optimized either with perfect or myopic foresight (Heuberger et al., 2018; Lopion et al., 2018). Next, we discuss our approach to optimize the transition path. Perfect foresight solves the whole optimization problem at once with complete information on all future parameters, whereas myopic foresight solves the investment periods sequentially with only current information on parameters (Ringkjøb et al., 2018). Perfect foresight leads to an optimal transition path; however, it is computationally demanding and does not capture reality, where future parameters are uncertain. While myopic foresight reduces the computation time, it leads only to a near-optimal transition path (Heuberger et al., 2018). In SecMOD, we employ a rolling-horizon strategy with myopic foresight as decomposition method of the problem with perfect foresight (Grossmann, 2012). In the rolling-horizon strategy, the optimization is carried out over a limited horizon which covers only few investment periods. After each optimization, the variables of the first investment period within the horizon are fixed, and the next investment periods are recalculated with updated myopic foresight. Here, we set the myopic foresight to 15 years. Preliminary studies with SecMOD have shown that the transition paths are almost the same with perfect foresight and 15 years of myopic foresight within the rolling-horizon strategy. The rolling-horizon strategy is described in detail in Supplementary Material.
2.1.3 Time Representation
Another challenge for energy system models is sufficient time resolution to capture the volatile nature of renewable energies (Mallapragada et al., 2018). SecMOD, therefore, employs hourly time resolution. To reduce computational effort, the two-step method from Bahl et al. (2018) is employed as the so-called primal heuristic to calculate near-optimal feasible solutions. In the first step, SecMOD solves a time-aggregated synthesis optimization in each investment period of the rolling horizon to expand the existing capacity. As aggregated time series, SecMOD considers an adjustable number of typical periods with typical time slices. Here, we choose to represent every investment period by 10 typical periods with 12 typical time steps each. In the second step, after each synthesis optimization, the operation is optimized. The operational optimization is conducted with the full hourly resolution and with capacities fixed of the current investment period. The operational optimization ensures that the energy system is feasible and thus can fulfill the energy demand of the original time series. If the operational optimization reveals that the energy system is not feasible, additional power, heat, or transportation capacities are added and the operational optimization is repeated. For details, see Supplementary Material. Importantly, the operational optimization is more accurate in terms of costs and GHG emissions as the calculated operational costs and GHG emissions from the time-aggregated synthesis optimization in the first step. In summary, the two-step optimization allows SecMOD to provide a feasible near-optimal solution for the hourly resolved problem.
The model formulation in SecMOD corresponds to the state-of-the-art in energy system models with its formulation as linear programming, time- and spatial-resolution, optimization horizon, and technology richness (Ringkjøb et al., 2018). To holistically evaluate environmental impacts of the transition path, SecMOD expands energy system modeling by LCA as presented in the following.
2.2 Life-Cycle Assessment
LCA is a holistic methodology for the environmental assessment of products and services, taking into account their entire life cycle (JRC European Commission, 2010). The life cycle includes all activities from cradle to grave: the extraction of raw materials, transportation, production and product use, recycling, treatment, and final disposal of waste. Through the life cycle, the activities result in material and energy flows that are exchanged with the environment. In LCA, the material and energy flows are collected and interpreted regarding their environmental impacts.
The ISO 14040 (2006) and ISO 14044 (2006) defined and standardized the LCA methodology. Next, we discuss the general goal and scope definition for our study of low-carbon transition pathways, including the definition of system boundaries, functional unit, life-cycle inventory (LCI), and methods for life-cycle impact assessment (LCIA).
2.2.1 Goal, System Boundaries, Functional Unit, and Multifunctionality
Our LCA pursues two main goals: 1) to determine environmental burden shifting caused by the German low-carbon transition path and 2) to provide specific environmental impacts for the services power, heat, and private transportation in 10-year steps according to the German low-carbon transition path till 2050; see Supplementary Material.
Based on the two goals of our study, the system boundary includes all processes associated with the low-carbon transition path of the German energy system. The considered German energy system contains the sectors power, heating, and private transportation, while international electricity trade is not considered. Neglecting electricity trade reduces the burden for modeling and computation but also impacts the results as discussed in Section 3.3.
In LCA, services or products are compared based on a so-called functional unit that quantifies the function of the investigated product systems and serves as a basis for comparison (ISO 14040, 2006; ISO 14044, 2006). The function of the considered energy system is to provide the services power, heat, and private transportation. To determine the environmental impacts along the low-carbon transition path, we use the functional units: power: 1 MWhpower, heat: 1 MWhheat, and private transportation: 1 vkm (vehicle kilometer), for the German energy system.
The environmental impacts of the electricity used in the heating and private transportation sector are accounted for the electricity sector as well as to the heating or private transportation sector, respectively. Synthetic natural gas can be used in the power, heating, and private transport sectors. We assume that the produced synthetic natural gas is fed into the natural gas grid. We, therefore, allocate the environmental impact of synthetic natural gas to individual sectors in proportion to the amount of used natural gas.
In LCA, processes are often multifunctional producing more than one product. Some of the existing conventional power plants in SecMOD generate both electricity and heat (combined heat and power). Multifunctionality is a well-known problem that can be solved using established methods such as system expansion, avoided burden and allocation of physical relationships or other criteria such as mass, energy, or economic value (ISO 14040, 2006; JRC European Commission, 2011). Here, we assume heat as waste and assign all environmental impacts to the electricity generation. This choice does not affect the transition pathway and has a minor impact on the distribution of the environmental impacts on the heating and electricity sector. In general, the contribution of heat from cogeneration is low for the considered low-carbon transition path: 4 and 0.5% of the heating demand in 2016 and 2050, respectively.
2.2.2 Life-Cycle Inventory
The life-cycle inventory (LCI) contains all mass and energy balances within the system boundaries required for the LCA. SecMOD computes the flows between the units to fulfill the final demand. For the considered technologies themselves (see Section 2.1), the LCI datasets are based on the LCI database ecoinvent version 3.5 APOS (Wernet et al., 2016). We chose the ecoinvent system model APOS to consistently consider recyclable materials. Recycling of materials is an important issue in energy system design due to the extensive infrastructure. Recycling rates for all metals are given in Supplementary Material. For all other materials, we assume treatment and final waste disposal documented in Supplementary Material with the LCI data. The LCI data of novel technologies missing in ecoinvent are modeled based on literature data with ecoinvent as background system. The modeled LCI datasets are described in detail in Supplementary Material.
In general, we distinguish between infrastructural and operational LCI datasets. The operational LCI datasets describe impacts resulting from the operation of a technology, e.g., due to the consumption and combustion of fuels, and auxiliaries for maintenance. The operational LCI datasets quantify the environmental impact per functional unit: MWhpower, MWhheat, and vkm. In contrast, the infrastructural LCI datasets of a technology cover the construction and subsequent dismantling, potential recycling, treatment, and final disposal of waste. The infrastructure of the technology is assessed based on the installed capacity in MW or the number of vehicles. This distinction between infrastructure and operation enables differentiating between environmental impacts that arise on-site and those that occur (partly) outside of Germany. Furthermore, the operational impacts are used to constrain the German GHG emissions in SecMOD. The current reduction targets are taken from the Federal Ministry for the Environment (2018).
From the synthesis optimization, we determine the infrastructural LCI datasets of existing and added capacity of each technology, for each investment period. To distribute the environmental burdens over the investment periods, the infrastructural LCI datasets are annualized over the minimum of lifetime and 30 years; see Section 2.1. As in Rauner and Budzinski (2017), we assume a discount rate of 0% for the LCI datasets. A discount rate of 0% implies that future environmental damage is equivalent to current damage.
The operational optimization calculates operational LCI datasets of all technologies for each hour of operation within the investment period. The infrastructural and operational LCI datasets are subsequently classified into the sectors power, heat, and private transportation based on the functional units.
An important issue for LCA in sector-coupled energy system models is double counting (Lenzen, 2008). Double counting occurs since LCI datasets contain energy-related environmental impacts from production steps in Germany. However, energy-related environmental impacts of the German industry are already included within the national energy model. As a result, such energy-related environmental impacts would be double counted. For example, electricity needed to manufacture German vehicles is considered as part of the industrial demands. However, the same electricity is also part of the LCI datasets of vehicles. In SecMOD, we solve the problem of double counting by deleting impacts in the LCI, which we already account for in the German energy system following the approach of Volkart et al. (2018) and Reinert et al. (2021).
2.2.3 Life-Cycle Impact Assessment
LCI data provides the basis for the life-cycle impact assessment (LCIA). In the LCIA phase, all mass and energy flows are analyzed concerning the resulting environmental impacts. For the low-carbon transition path, the main goal is the reduction of GHG emissions, i.e., the climate impact. However, besides climate impacts, the energy system leads also to other environmental impacts. To identify potential environmental trade-offs and to determine burden shifting, according to the first goal of our study, we consider 15 additional environmental impacts, Table 1. We assess the environmental impacts according to the LCIA-method Environmental Footprint 2.0 recommended by the European Commission’s Joint Research Center (Castellani et al., 2018). Environmental Footprint 2.0 is the latest recommended method by the European Commission implemented in the LCI database ecoinvent. The recommended environmental impacts are classified into three quality levels (JRC European Commission, 2011) and updated in Castellani et al. (2018) regarding completeness, relevance, robustness, transparency, applicability, acceptance, and suitability. The quality classification of each environmental impact (Table 1) should be considered when interpreting LCA results.
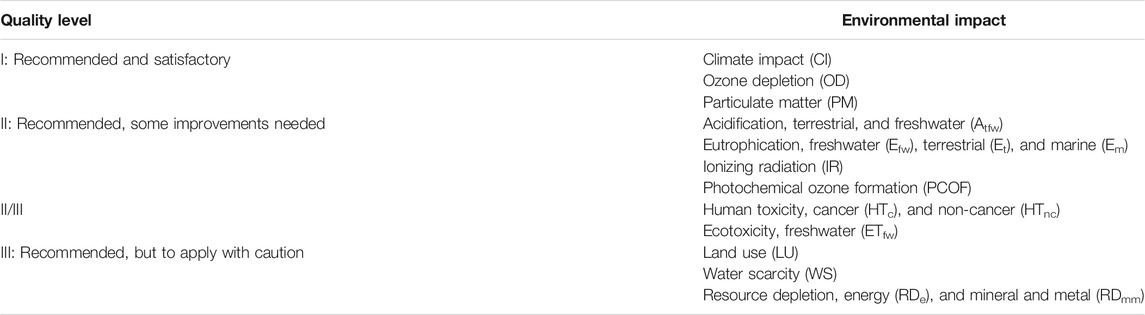
TABLE 1. Considered environmental impacts in SecMOD following the recommendation of the European Commission’s Joint Research Centre in the European context, sorted by their quality level (JRC European Commission, 2011; Castellani et al., 2018).
According to the second goal of our study, we determine the specific environmental impacts of the energy services in the sector electricity, heating, and private transportation. For this purpose, we also calculate the annualized infrastructural environmental impacts due to installed capacities based on the LCIs from the synthesis optimization. The operational optimization calculates the operational environmental impacts on an hourly basis. Infrastructural and operational environmental impacts are subsequently assigned to the sectors power, heat, and private transportation.
Specific environmental impacts are achieved by dividing the total impacts of each sector by the energy output of the corresponding sector in MWhpower, MWhheat, and vkm.
3 Transition Pathway of the German Energy System From SecMOD
In SecMOD, we optimize the transition pathway to a low-carbon energy system. In Section 3.1, we present the complete low-carbon transition pathway of the German energy system until 2050. The transition pathway results from an economic optimization with constrained GHG emissions. In Section 3.2 we show the environmental impacts for the provided energy services: electricity, heat, and transportation. The transition pathway is optimal under the given model assumptions that, however, represent only one possible transition scenario among many possibilities. Key assumptions are discussed in Section 3.3.
3.1 Energy-Transition Pathway
Emission reductions of 85% by 2050 are possible considering the sector-coupled German energy system without international electricity trade, Figure 1. In the first investment periods, emissions are reduced in the power and especially in the heating sector. The decarbonization of the transportation sector starts later in the transition. In 2050, to achieve the 85% reduction target, the transportation sector is almost carbon-neutral and GHG emissions from both the heating and electricity sectors are reduced to approximately one-third of the emissions in 2016.
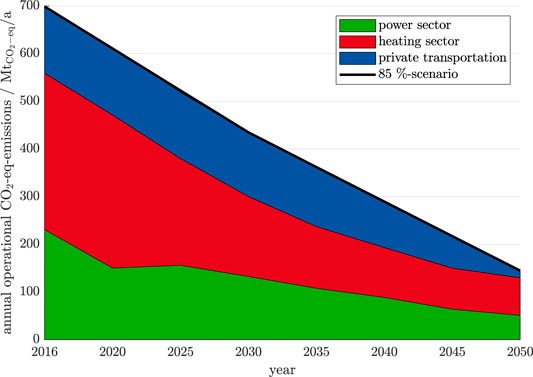
FIGURE 1. Operational GHG emissions for the transition path considering the 85% reduction target compared to 1990 (966 Mt CO2-eq). The 85%-Scenario is based on the targets of the German Federal Government of a 55% reduction by 2030, 70% by 2040, and 80–95% reduction by 2050. We assume a linear reduction of emission limits. The emissions are assigned to the power, heating, and private transportation sector.
Until 2050, the resulting energy system is proven to be feasible by operational optimization with a time-resolution of 1 h. The total annualized costs increase by a factor of three compared to current costs (Figure 2). The costs increase mainly due to the demand for storage capacity. Storage is necessary to cope with the volatile nature of renewable generation. The required amount of storage needed is high due to the considered high temporal and spatial resolution. Storage demand is often underestimated in energy system models which are not spatially resolved (Heuberger et al., 2020). In addition, Victoria et al. (2019) showed that storage demand increases strongly for reduction targets beyond 80%. For a decarbonized European energy system in 2050, Child et al. (2018) predicted a cost-share of storage of about 30%.
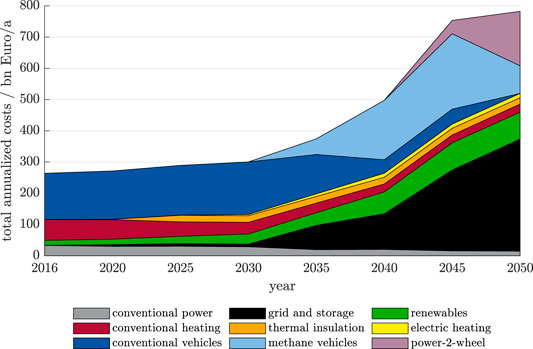
FIGURE 2. Total annualized costs for the energy-transition path reaching the 85% reduction target. Technologies are lumped for clarity of presentation. Power-2-wheel includes battery electric vehicles, fuel cell vehicles, and synthetic diesel.
In the energy transition, energy services are provided by different technologies since increasingly tighter emission constraints from 2016 to 2050 require the change to low-carbon technologies. First, we discuss the heating sector, Section 3.1.1. We then regard private transportation, Section 3.1.2. Finally, we describe the electricity sector that becomes increasingly important in low-carbon energy systems, Section 3.1.3.
3.1.1 Heating Sector
The first measure to reduce GHG emissions in the heating sector is to reduce heating demand by thermal insulation (Figure 3). Thermal insulation is already known as a main measure of energy transition in the heating sector (Ifeu, Fraunhofer IEE., and Consentec, 2019). In SecMOD, investment rates for all technologies are unlimited; i.e., no budget limit is given. Thus, a rapid technology shift is possible for thermal insulation. Starting from 2016, oil boilers are replaced by more efficient gas boilers. Beginning 2025, heating is increasingly electrified. First, the supply of domestic and industrial low-temperature heating is electrified by heat pumps. Starting 2030, electrification of industrial medium-temperature heating starts with electrode boilers, but with slower deployment rates. In the year 2040, all oil boilers are dismantled. In 2050, 75% of the heating demand is directly electrified or reduced by thermal insulation. In SecMOD, we assume that industrial, high-temperature heat demand above 400°C cannot be directly electrified. Still, starting 2035, the heating sector is further indirectly electrified by synthetic natural gas; see Figure 5. In summary, measures to decarbonize the heating sector are as follows. First, low-temperature domestic heating demand is reduced by thermal insulation. Second, heat supply is directly electrified via heat-pumps and electric boilers. Third, high-temperature heat supply is electrified indirectly by synthetic natural gas.
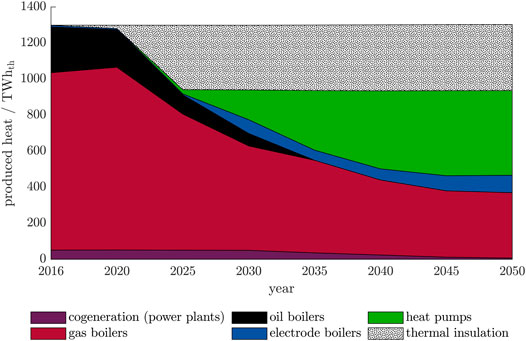
FIGURE 3. Provided heating demand in Germany during the energy transition from 2016 to 2050. Thermal insulation reduces overall domestic heating demand. Temperature levels of the heating demand as well as centralized and decentralized heating demands are lumped for clarity of presentation. Gas boilers can be fueled by (synthetic) natural gas.
3.1.2 Transportation Sector
Significant decarbonization of the private transportation sector occurs late compared to the other sectors, Figure 4, due to higher abatement costs. During the first investment periods, the ratio between gasoline and diesel vehicles shifts toward more diesel vehicles, as diesel has lower specific GHG emissions in operation compared to gasoline vehicles. Until 2030, all gasoline vehicles are replaced by diesel vehicles. Natural gas vehicles act as transition technology, due to even lower specific GHG emissions in operation at slightly higher costs. Starting in 2030, natural gas vehicles provide increasing parts of the transportation demand. Until 2040, about 85% of the demand is provided by natural gas vehicles. Beginning in 2040, a large share of battery electric vehicles (BEV) start to replace the remaining diesel vehicles and already old natural gas vehicles, integrating more renewable electricity directly in the transportation sector. Also beginning from 2040, synthetic diesel is partly used in remaining diesel vehicles. In 2050, about 80% of the transportation demand is provided by battery electric vehicles, while about 15% of the demand is still provided by natural gas vehicles. In SecMOD, battery electric vehicles can only replace a certain share of conventional technologies (45% in 2016 and 82% in 2050), as long-distant transportation is limited due to battery capacities: see Supplementary Material. In 2045, fuel cell vehicles also begin to replace the remaining natural gas vehicles, as low-carbon, long-distant transportation technology. To account for costs of charging infrastructure, the deployment of fuel cell and battery electric vehicles leads to a parallel investment in this infrastructure; see Supplementary Material. For conventional vehicles, cost of charging infrastructure is included within fuel costs. In summary, decarbonization of the private transportation sector starts later than the decarbonization of the heating sector due to higher costs and employs a mix of technologies to cover short- and long-distance transportation.
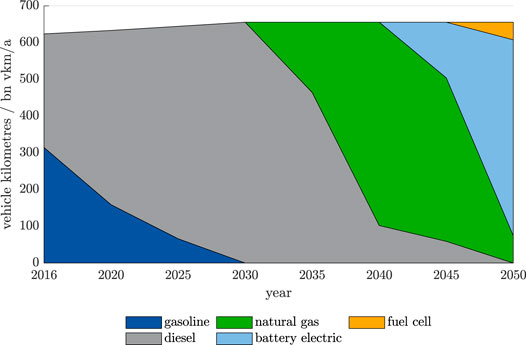
FIGURE 4. Provided transportation service per vehicle type. Natural gas vehicles can be fueled by (synthetic) natural gas.
3.1.3 Electricity Sector
The decarbonization of both transportation and, in particular, heating heavily depends on low-carbon electricity, Figure 5. SecMOD computes that about one-third of the electricity is provided by renewable generation in Germany in 2016, which is close to the actual value of 32% from Fraunhofer ISE (Burger, 2017). To decarbonize the electricity sector, almost all conventional power plants continuously phase-out, except for gas-power plants. At the same time, these conventional power plants are substituted by renewable electricity production. Between 2025 and 2050, the electricity demand almost doubles to 1,100 TWh (excluding storage input and output).
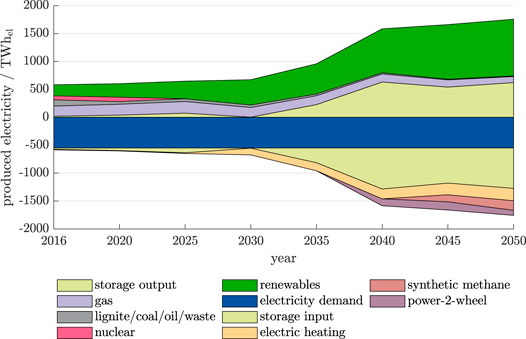
FIGURE 5. Produced electricity (positive) and consumed electricity in cross-sectoral technologies (negative) in the German energy transition. Gas includes gas turbines as well as combined cycle gas turbines. Power-2-wheel includes battery electric vehicles, fuel cell vehicles, and synthetic diesel.
Due to the high electricity demand caused by sector coupling and distributed renewable generation, the grid capacity is almost doubled. To cope with the volatile nature of renewable electricity in 2050, about
The storage periods are predominantly short and thus used to shift wind and solar electricity within a day. For longer storage periods and further decarbonization, synthetic natural gas is produced starting 2035. An amount of
To decarbonize the electricity sector, a rapid expansion of solar power, onshore wind, and offshore wind is required, Figure 6. First, regions with high full load hours per year for wind and solar power are exploited. The capacities in these regions are, however, limited; see the Supplementary Material. Thus, with the increasing need for renewable electricity, regions with lower full load hours are also employed. Therefore, overall full load hours decline by about 35%, Figure 6. The capacity limits for solar power and offshore wind are reached in 2040, for onshore wind in 2045. Thus, the full capacity by renewable electricity production is utilized. In 2045 and 2050, the same amount of low-carbon electricity is produced. In 2050, the low-carbon electricity is used more efficiently by direct electrification, however, with higher investment costs. The decline in full load hours and the high demand for short-term storage capacities are major contributors to burden shifting, as discussed next.
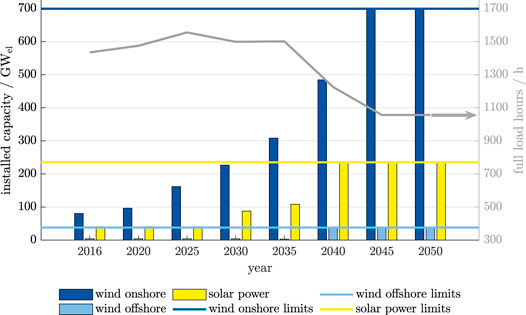
FIGURE 6. Installed capacity of onshore wind, offshore wind, and solar power, and full load hours per year for all investment periods.
3.2 LCA of the Energy-Transition Pathway
Here, we discuss the environmental impacts per functional unit: MWhpower, MWhheat, and vehicle kilometer (vkm). The environmental impacts of future years are shown using 2016 as reference. The total environmental impacts include the operation and infrastructure of technologies, cf. Section 2.2. In general, we observe a shift in the origin of environmental impacts: in 2050, infrastructure contributes 41–98% to the impacts in the electricity sector, 10–58% in the heating sector, and 38–98% in the transportation sector, Supplementary Material. Importantly, the GHG reduction target of 85% in operational emissions corresponds only to a total reduction of 70% if both operational and infrastructural emissions are accounted for. This drastic trend from impacts from operation to infrastructure shows that both politics and energy system models must include LCA to assess environmental impacts holistically over the full life cycle.
In the following, we first discuss environmental impacts from each sector. Tables with the most important LCA results for 2016–2050 are given in Supplementary Material. In detail, we provide tables with the following:
specific environmental impacts per functional unit,
technology-specific contributions to the environmental impacts of the electricity sector in 2050,
share of the infrastructural environmental impacts in each sector, and
share of the environmental impacts due to electricity used in the heating and transportation sector.
The present analysis is based on static LCA for the background processes. Thus, future improvements of technology and their production are not reflected. Current work therefore aims at integrating dynamic LCA in SecMOD to capture such changes in the background system.3.2.1 Electricity Sector
In the electricity sector, the reduction of GHG emissions leads to cobenefits; thus, other environmental impacts are reduced. In 2050, environmental impacts are reduced most for the depletion of energy resources (RDe, 78%), eutrophication freshwater (Efw, 90%), and ionizing radiation (IR, 93%). In total, 11 of 16 environmental impacts are reduced by 10–92% within the power sector in 2050, Figure 7. The fact that reductions of GHG emissions lead to cobenefits is in line with other studies and has recently been discussed in detail by Luderer et al. (2019).
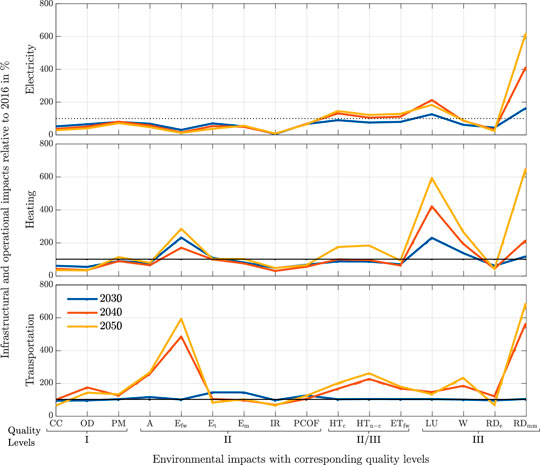
FIGURE 7. Operational and infrastructural environmental impacts for 1 MWhpower electricity, MWhheat heating, and for 1 vkm of private transportation. The environmental impacts of 2016 are used as reference (dotted black line: 2016 = 100%). For abbreviations and quality levels, see Table 1. Electricity used in heating and transportation sector is accounted for in these sectors.
However, the low-carbon transition of the electricity sector results in significant increases in other environmental impacts in 2050: metal and minerals resource depletion RDmm increases by 520%, ecotoxicity fresh water ETfw by 30%, human toxicities HTc and HTn−c by 45 and 20%, and land use LU by 80%. Environmental impacts increase due to the expansion of stationary batteries and low-carbon electricity generation technologies (see Section 3.1), with a contribution 7–51% for batteries, 8–48% for wind, and 12–66% for photovoltaics; see Supplementary Material. All environmental impacts that significantly increased are at quality level II/III-III indicating high uncertainty. Thus, there is a need to improve LCA and LCIA methods to clarify the extent of increasing environmental impacts.
3.2.2 Heating Sector
In the heating sector, six out of 16 environmental impacts are lower than in 2016, whereas four stay nearly the same in 2050 (≤15%). With rising shares of heat pumps and electrode boilers, the environmental impacts of heating are strongly determined by the environmental impacts of the electricity system. In 2050, environmental impacts in the heating sector are dominated by electricity used with values of up to 90% in metal and minerals resource depletion RDmm. Metal and minerals resource depletion RDmm increases by 550% and land use LU by 490%. Furthermore, freshwater eutrophication Efw increases by 185%.
3.2.3 Transportation Sector
Private transportation shows a slower transition than the other sectors. The initial shift from gasoline to diesel vehicles changes environmental impacts for 1 vkm of private transportation by less than 50% by 2030, due to the similarity of the fuel types. The shift from diesel to natural gas vehicles after 2035 improves all environmental impacts. However, starting 2040, the transportation sector shifts to battery electric vehicles, small shares of synthetic diesel, and fuel cell cars. Therefore, by 2050, we observe a decrease in climate impact by about 45% and, however, also a significant increase in freshwater eutrophication by 490% and in metal and minerals resource depletion RDmm by 590%. Again, it is important to consider the quality levels of increased environmental impacts. The increase of metals and minerals resource depletion and freshwater eutrophication has low quality levels. Electricity used in the transportation sector in 2050 has only minor effects on specific environmental impacts (≤15%), as impacts are dominated by infrastructure.
3.2.4 Effects of Sector-Coupling
Our results are in line with other studies which focused only on the electricity sector. For the electricity sector in Denmark, Turconi et al. (2014) showed a reduction of all environmental impacts besides metal depletion (“Green Scenario 2030”). Metal depletion worsens by about 50%. In our multisector investigation of Germany, we also observe a reduction in all environmental impacts in 2030 except metal and minerals depletion and land use. Metal and minerals depletion increases by about 65% and land use by 25%.
Until 2030, the sectors are not yet highly coupled, storage capacities are still small, and renewable sites with low full load hours have not yet been employed. Therefore, until 2030, studies only investigating the electricity sector with low spatial and temporal resolution lead to similar results as our study considering multiple sectors and high resolution.
Also Rauner and Budzinski (2017) showed, in their most environmentally friendly scenario for 2050, increases in the same environmental impacts as in our study: metal depletion, land occupation, and ecotoxicity. However, the authors found an increase in metal depletion by merely 200%, whereas in our study the increase is about 520%. The comparison shows that only considering the electricity sector underestimates environmental impacts due to an increasing electricity demand caused by sector-coupling technologies, in particular after 2030 for the German case study.
3.3 Discussion of the Results
All energy models attempt to represent reality. The larger and more complex the energy systems under consideration are, the more assumptions are needed. Besides, models that predict the future require more far-reaching assumptions, e.g., the development of efficiencies and costs. Here, we not only investigate the complex German energy system, but also look 30 years into an uncertain future. Accordingly, our model is based on many assumptions, which we carefully reviewed and documented.
Here, we discuss the influence of the three most important assumptions.
3.3.1 System Boundaries
SecMOD combines many features to model the German energy system as accurate as possible, such as high spatial and temporal resolution, and the most important sectors. This accurate representation of time and space leads to large demand for storage capacity, which is the main cost driver of the low-carbon energy system, cf. Section 3.1. For the considered sectors, we assume constant or rising demands. Behavioral change might reduce these demands, which directly reduces system costs and environmental impacts. In addition, further sectors can influence the system costs and impacts, such as the chemical industry as potential large consumer of renewable energy and/or producer of fuel and storage provider.
In SecMOD, we consider the German energy system without international electricity trade. However, we expect that international electricity trade would reduce the storage demand and total annualized costs as suggested by Weitemeyer et al. (2016), Child et al. (2018), Louis et al. (2020), and Breyer et al. (2020). For example, Child et al. (2018) found that costs can be reduced by about 10% for a highly integrated European electricity system. Storage demand and total annualized costs can further be slightly reduced by exploiting storage capacities of battery electric vehicles (Weitemeyer et al., 2016; Victoria et al., 2019).
Our modeled German energy system can only import conventional fuels, i.e., natural gas, gasoline, and diesel. However, in a low-carbon world, synthetic fuels are likely to be produced abroad and transported to energy-importing countries like Germany (Verkehrswende, 2018; Hank et al., 2020). Synthetic fuels could enable the transition toward low-carbon energy systems, still based on current infrastructure without large changes (The Royal Society, 2019). Thereby, synthetic fuels might reduce costs. Furthermore, synthetic fuels could also reduce the environmental impacts such as NOx and soot (Deutz et al., 2018).
In summary, we expect that international trade of electricity would reduce costs. Further costs reductions could arise for reducing demand by behavioral change, storage in BEVs, and import of synthetic fuels. In addition, consideration of further sectors might reduce costs due to synergies or impose further costs and burden as added consumer.
3.3.2 Annualization
Technologies are usually annualized over their lifetime. We use an interest rate of 5% for annualization. Both parameters, time of annualization, and interest rate, are fixed over the entire optimization horizon. These parameters have a high impact on the total annualized costs and also on technology preference since they define the balance between operation and investment cost. However, the importance of operational costs declines in low-carbon energy system. Thus, the right balance between operation and investment costs becomes less important.
We also use annualized infrastructural impacts in the LCA. Without annualized impacts, the impacts would show peaks in the year of construction. However, these annualized impacts have no effect on the transition pathway, but only on the representation of results. Still, when interpreting results, we must take into account that environmental impacts actually occur in the year of construction.
3.3.3 Technology Parameters
80% of the total annualized costs in 2050 are due to the investment costs of just five technologies: battery storage, battery electric vehicles, natural gas vehicles, onshore wind, and photovoltaics. Thus, cost predictions for these technologies have significant impact on the total annualized costs.
To evaluate a potential cost range, we collected more optimistic cost projections, Table 2. These cost projections reduce the total annualized costs in 2050 by over 40% to about 460 bn €/a (Table 2 and cf. Figure 2). Thereby the total annualized costs increase only by a factor of 1.7, instead of 3, compared to current costs. Thus, the economic development of technologies has a large influence on the future costs.
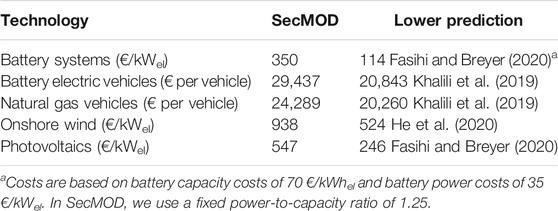
TABLE 2. Comparison of investment costs of specific technologies in SecMOD and more optimistic cost projections in 2050.
The ecological development of technologies can have a similar positive effect on our environmental results. For example, Cox et al. (2018) showed that climate impact of future battery electric vehicles might decrease by 75%, mainly due to cleaner resources. Our results show the strong need for low-carbon technologies with reduced needs for metal and minerals and less freshwater eutrophication. Hopefully, technology development will reduce both costs and environmental impacts.
All assumptions, and these three assumptions in particular, have a significant influence on the quantitative results. We believe that all our assumptions are well in line with the state-of-the-art and, thus, reasonable. Still, we recognize the uncertainty and focus our conclusion on underlying trends.
4 Conclusion
We propose the national energy system model SecMOD. SecMOD considers the electricity sector, the domestic and industrial heating sector, and the private transportation sector in Germany. SecMOD optimizes the low-carbon transition of a national energy system with high spatial and temporal resolution and further includes LCA. SecMOD is parameterized for the German energy system; however, we believe that key findings and trends are valid for all energy systems in industrialized countries under transition.
We show an optimized transition to achieve the German GHG reduction goal of 85% until 2050 compared to 1990. To achieve the GHG reduction, three elements are key: deployment of low-carbon technologies, storage, and electrification of all sectors. The electrification of all sectors doubles the electricity demand to 1,100 TWh/a. To cope with the volatile and distributed nature of renewable energies, doubled electricity grid capacity and large storage capacity are necessary. We show that the energy transition is feasible, but the total cost increases by a factor of 3. However, these high costs might be reduced significantly by international trade of electricity, import of synthetic fuels, and technology development. Using alternative learning curves reduces the increase already to a factor of 1.7.
In future, operation contributes less to overall environmental impacts, while infrastructural environmental impacts are gaining more importance. This shift to infrastructural impacts shows that energy system models have to include LCA to assess environmental impacts holistically and detect burden shifting.
We derive specific impacts for 1 MWhpower of power, MWhheat heat, and 1 vkm of transportation, for the computed energy transition satisfying the GHG-emission targets.
For the power sector, we observe many cobenefits of the energy transition: 11 of 16 environmental impacts decrease. However, some environmental impacts also increase: for instance, the transition increases the depletion of metal and minerals by a factor of 6, compared to 2016. The comparison with other studies shows that considering only the electricity sector underestimates the specific environmental impacts, also, for the electricity sector. Thus, all sectors need to be considered, even if only electricity supply is of interest.
In the heating and transportation sector, also only few impacts increase with the largest increase for metals and minerals resource depletion, freshwater eutrophication, and land use. These impacts are critical for future technology development.
To avoid burden-shifting, national GHG-reduction strategies should consider the mentioned environmental impacts rather than focusing on climate change only. The observed increases concern mainly impacts with high uncertainty. Further research in LCA should improve LCIA methods to reduce uncertainty for these important environmental impacts.
Data Availability Statement
The original contributions presented in the study are included in the article/Supplementary Material; further inquiries can be directed to the corresponding author.
Author Contributions
NB and SD drafted the article, wrote the paper, were involved in study conception, performed the analysis, and developed the model and optimization method. CR, NN, and EK collected data, contributed to tool analysis, and contributed to the model and optimization method. MH and DH drafted the article and performed critical revision. AB was involved in drafting the article, study conception, and critical revision.
Conflict of Interest
The authors declare that the research was conducted in the absence of any commercial or financial relationships that could be construed as a potential conflict of interest.
Acknowledgments
The authors thank Dana Aleff, Erik Dickhof, Lukas Dörpinghaus, Anton Gemassner, Jonas Grossmann, Niklas Groll, Tobias Gülker, Lisa Neumaier, Sophia Schröer, and Maximilian Staib for their contributions to the model framework.
Supplementary Material
The Supplementary Material for this article can be found online at: https://www.frontiersin.org/articles/10.3389/fenrg.2021.621502/full#supplementary-material.
References
Algunaibet, I., and Guillén-Gosálbez, G. (2019). Life cycle burden-shifting in energy systems designed to minimize greenhouse gas emissions: novel analytical method and application to the United States. J. Clean. Prod. 229, 886–901. doi:10.1016/j.jclepro.2019.04.276
Bahl, B., Söhler, T., Hennen, M., and Bardow, A. (2018). Typical periods for two-stage synthesis by time-series aggregation with bounded error in objective function. Front. Energ. Res. 5, 35. doi:10.3389/fenrg.2017.00035
Berrill, P., Arvesen, A., Scholz, Y., Gils, H., and Hertwich, E. (2016). Environmental impacts of high penetration renewable energy scenarios for Europe. Environ. Res. Lett. 11. doi:10.1088/1748-9326/11/1/014012
Blanco, H., Codina, V., Laurent, A., Nijs, W., Maréchal, F., and Faaij, A. (2020). Life cycle assessment integration into energy system models: an application for Power-to-Methane in the EU. Appl. Energ. 259, 114160. doi:10.1016/j.apenergy.2019.114160
Breyer, C., Bogdanov, D., Aghahosseini, A., Gulagi, A., Child, M., Oyewo, A. S., et al. (2018). Solar photovoltaics demand for the global energy transition in the power sector. Prog. Photovolt Res. Appl. 26, 505–523. doi:10.1002/pip.2950
Breyer, C., Bogdanov, D., Aghahosseini, A., Gulagi, A., and Fasihi, M. (2020). On the techno-economic benefits of a global energy interconnection. Econ. Energ. Environ. Pol. 9, 83–103. doi:10.5547/2160-5890.9.1.cbre
Burger, B. (2017). Stromerzeugung in Deutschland im Jahr 2016. Freiburg, Germany: Fraunhofer-Institut für Solare Energiesysteme ISE.
Castellani, V., Diaconu, E., Fazio, S., Sala, S., Schau, E. M., Secchi, M., et al. (2018). “Supporting information to the characterisation factors of recommended EF Life Cycle Impact Assessment methods,” in New methods and differences with ILCD volume 28888 of EUR, Scientific and technical research series. Luxembourg, Netherlands: Publications Office of the European Union
Child, M., Bogdanov, D., and Breyer, C. (2018). The role of storage technologies for the transition to a 100% renewable energy system in Europe. Energy Proced. 155, 44–60. doi:10.1016/j.egypro.2018.11.067
Cox, B., Mutel, C. L., Bauer, C., Mendoza Beltran, A., and van Vuuren, D. P. (2018). Uncertain environmental Footprint of current and future battery electric vehicles. Environ. Sci. Technol. 52, 4989–4995. doi:10.1021/acs.est.8b00261
Cronin, J., Anandarajah, G., and Dessens, O. (2018). Climate change impacts on the energy system: a review of trends and gaps. Climatic Change 151, 79–93. doi:10.1007/s10584-018-2265-4
DeCarolis, J., Daly, H., Dodds, P., Keppo, I., Li, F., McDowall, W., et al. (2017). Formalizing best practice for energy system optimization modelling. Appl. Energy 194, 184–198. doi:10.1016/j.apenergy.2017.03.001
Dena Grid Study II (2010). Integration of renewable energy sources in the German power supply system from 2015–2020 with an outlook to 2025. Berlin, Germany: German Energy Agency-Dena.
Deutz, S., Bongartz, D., Heuser, B., Kätelhön, A., Schulze Langenhorst, L., Omari, A., et al. (2018). Cleaner production of cleaner fuels: wind-to-wheel-environmental assessment of CO2-based oxymethylene ether as a drop-in fuel. Energy Environ. Sci. 11, 331–343. doi:10.1039/c7ee01657c
Egerer, J. (2016). Open source electricity model for Germany (ELMOD-DE). Available at: http://hdl.handle.net/10419/129782 (Accessed March 13, 2021).
Fasihi, M., and Breyer, C. (2020). Baseload electricity and hydrogen supply based on hybrid PV-wind power plants. J. Clean. Prod. 243, 118466. doi:10.1016/j.jclepro.2019.118466
Federal Ministry for the Environment, Nature Conservation and Nuclear Safety (2018). Climate action in figures: facts, trends and incentives for German climate policy nature conservation and nuclear safety. Bonn, Germany: Federal Ministry for the Environment.
Federal Motor Transport Authority (2017). Verkehr in Kilometern der deutschen Kraftfahrzeuge im Jahr 2016: Kurzbericht (German). Wiesbaden, Germany: Federal Motor Transport Authority.
Federal Statistical Office (2015). Bevölkerung und Erwerbstätigkeit: haushalte und Familien-ergebnisse des Mikrozensus (German). Wiesbaden, Germany: Federal Statistical Office of Germany.
GAMS (2016). General algebraic modeling system version 27.3. Washington, DC: GAMS Development Corporation.
García-Gusano, D., Iribarren, D., Martín-Gamboa, M., Dufour, J., Espegren, K., and Lind, A. (2016). Integration of life-cycle indicators into energy optimisation models: the case study of power generation in Norway. J. Clean. Prod. 112, 2693–2696. doi:10.1016/j.jclepro.2015.10.075
Gençer, E., Torkamani, S., Miller, I., Wu, T. W., and O’Sullivan, F. (2020). Sustainable energy system analysis modeling environment: analyzing life cycle emissions of the energy transition. Appl. Energy 277, 115550. doi:10.1016/j.apenergy.2020.115550
Gerhardt, N., Sandau, F., Scholz, A., Hahn, H., Schumacher, P., Sager, C., et al. (2015). Interaktion EE-Strom, Wärme und Verkehr: analyse der Interaktion zwischen den Sektoren Strom, Wärme/Kälte und Verkehr in Deutschland (German). Bremerhaven, Germany: IWES.
German Energy Agency (2018). Dena-Leitstudie Integrierte Energiewende: impulse für die Gestaltung des Energiesystems 2050. Berlin, Germany: DENA.
Gils, H. C., Scholz, Y., Pregger, T., Luca de Tena, D., and Heide, D. (2017). Integrated modelling of variable renewable energy-based power supply in Europe. Energy 123, 173–188. doi:10.1016/j.energy.2017.01.115
Grossmann, I. (2012). Advances in mathematical programming models for enterprise-wide optimization. Comput. Chem. Eng. 47, 2–18. doi:10.1016/j.compchemeng.2012.06.038
Haller, M., Ludig, S., and Bauer, N. (2012). Decarbonization scenarios for the EU and MENA power system: considering spatial distribution and short term dynamics of renewable generation. Energy Policy 47, 282–290. doi:10.1016/j.enpol.2012.04.069
Hank, C., Sternberg, A., Köppel, N., Holst, M., Smolinka, T., Schaadt, A., et al. (2020). Energy efficiency and economic assessment of imported energy carriers based on renewable electricity. Sustain. Energy Fuels 4, 2256–2273. doi:10.1039/d0se00067a
He, G., Lin, J., Sifuentes, F., Liu, X., Abhyankar, N., and Phadke, A. (2020). Rapid cost decrease of renewables and storage accelerates the decarbonization of China’s power system. Nat. Commun. 11, 2486. doi:10.1038/s41467-020-17706-3
Heuberger, C., Bains, P., and Mac Dowell, N. (2020). The EV-olution of the power system: a spatio-temporal optimisation model to investigate the impact of electric vehicle deployment. Appl. Energy 257, 113715. doi:10.1016/j.apenergy.2019.113715
Heuberger, C., Staffell, I., Shah, N., and Mac Dowell, N. (2018). Impact of myopic decision-making and disruptive events in power systems planning. Nat. Energy 3, 634–640. doi:10.1038/s41560-018-0159-3
Howells, M., Rogner, H., Strachan, N., Heaps, C., Huntington, H., Kypreos, S., et al. (2011). OSeMOSYS: the open source energy modeling system. Energy Policy 39, 5850–5870. doi:10.1016/j.enpol.2011.06.033
IBM (2016). IBM ILOG and CPLEX optimization and studio and CPLEX user’s and manual and version 12 and release 7. New York, NY: IBM.
Ifeu, Fraunhofer IEE., and Consentec (2019). Building sector efficiency: a crucial component of the energy transition: a study commissioned by agora energiewende. Berlin, Germany: Agora Energiewende.
IPCC (2018). Global Warming of 1.5 C above pre-industrial levels and related global greenhouse gas emission pathways in the context of strengthening the global response to the threat of climate change, sustainable development, and efforts to eradicate poverty. Incheon, Republic of Korea: IPCC.
ISO 14040 (2006). International organization for standardization. Environmental management: life cycle assessment-principles and framework. London, United Kingdom: ISO.
ISO 14044 (2006). International organization for standardization. Environmental management-life cycle assessment-requirements and guidelines. London, United Kingdom: ISO.
JRC European Commission (2011). International reference life cycle data system (ILCD) handbook-recommendations for life cycle impact assessment in the European context from the European commission. Luxembourg, Netherlands: Publications Office of the European Union.
JRC European Commission (2010). European commission-joint research centre-international reference life cycle data system (ILCD) handbook-general guide for life cycle assessment-detailed guidance. Luxembourg, Netherlands: Publications Office of the European Union.
Khalili, S., Rantanen, E., Bogdanov, D., and Breyer, C. (2019). Global transportation demand development with impacts on the energy demand and greenhouse gas emissions in a climate-constrained world. Energies 12, 3870. doi:10.3390/en12203870
Kouloumpis, V., Stamford, L., and Azapagic, A. (2015). Decarbonising electricity supply: is climate change mitigation going to be carried out at the expense of other environmental impacts?. Sustain. Prod. Consum. 1, 1–21. doi:10.1016/j.spc.2015.04.001
Lenzen, M. (2008). Double-counting in life cycle calculations. J. Ind. Ecol. 12, 583–599. doi:10.1111/j.1530-9290.2008.00067.x
Li, X., Damartzis, T., Stadler, Z., Moret, S., Meier, B., Friedl, M., et al. (2020). Decarbonization in complex energy systems:a study on the feasibility of carbon neutrality for Switzerland in 2050. Front. Energy Res. 8, 549615. doi:10.3389/fenrg.2020.549615
Lopion, P., Markewitz, P., Robinius, M., and Stolten, D. (2018). A review of current challenges and trends in energy systems modeling. Renew. Sust. Energy Rev. 96, 156–166. doi:10.1016/j.rser.2018.07.045
Louis, J.-N., Allard, S., Kotrotsou, F., and Debusschere, V. (2020). A multi-objective approach to the prospective development of the European power system by 2050. Energy 191, 116539. doi:10.1016/j.energy.2019.116539
Loulou, R., Lehtilä, A., Kanudia, A., Remme, U., and Goldstein, G. (2016). Documentation for the TIMES model part II: energy technology systems analysis programme. Available at: http://www.iea-etsap.org/web/Documentation.asp (Accessed March 13, 2021).
Luderer, G., Pehl, M., Arvesen, A., Gibon, T., Bodirsky, B. L., de Boer, H. S., et al. (2019). Environmental co-benefits and adverse side-effects of alternative power sector decarbonization strategies. Nat. Commun. 10, 5229. doi:10.1038/s41467-019-13067-8
Mainzer, K., Fath, K., McKenna, R., Stengel, J., Fichtner, W., and Schultmann, F. (2014). A high-resolution determination of the technical potential for residential-roof-mounted photovoltaic systems in Germany. Solar Energy 105, 715–731. doi:10.1016/j.solener.2014.04.015
Mallapragada, D. S., Papageorgiou, D. J., Venkatesh, A., Lara, C. L., and Grossmann, I. E. (2018). Impact of model resolution on scenario outcomes for electricity sector system expansion. Energy 163, 1231–1244. doi:10.1016/j.energy.2018.08.015
McDowall, W., Rodriguez, B., Usubiaga, A., and Fernández, J. (2018). Is the optimal decarbonization pathway influenced by indirect emissions? Incorporating indirect life-cycle carbon dioxide emissions into a European TIMES model. J. Clean. Prod. 170, 260–268. doi:10.1016/j.jclepro.2017.09.132
McKenna, R., Hollnaicher, S., OstmanLeye, v. d. P., and Fichtner, W. (2015). Cost-potentials for large onshore wind turbines in Europe. Energy 83, 217–229. doi:10.1016/j.energy.2015.02.016
Mirakyan, A., and de Guio, R. (2015). Modelling and uncertainties in integrated energy planning. Renew. Sust. Energy Rev. 46, 62–69. doi:10.1016/j.rser.2015.02.028
Öko-Institut and Fraunhofer ISI. (2015). Klimaschutzszenario 2050–2. Endbericht: (German). Berlin, Germany: Öko-Institut e.V and Fraunhofer ISI.
Olivier, J., Schure, K., and Peters, J. (2017). Trends in global CO2 and total greenhouse gas emissions: summary of 2017 report. The Hague, Netherlands: PBL Netherlands Environmental Assessment Agency.
Overbye, T. J., Cheng, X., and Sun, Y. (2004). “A comparison of the AC and DC power flow models for LMP calculations,” in 37th Annual Hawaii International Conference on System Sciences, 2004, Big Island, HI, Jan 5–8, 2004 (New York, NY: IEEE), 1–9.
Pregger, T., Nitsch, J., and Naegler, T. (2013). Long-term scenarios and strategies for the deployment of renewable energies in Germany. Energy Policy 59, 350–360. doi:10.1016/j.enpol.2013.03.049
Rauner, S., and Budzinski, M. (2017). Holistic energy system modeling combining multi-objective optimization and life cycle assessment. Environ. Res. Lett. 12, 124005. doi:10.1088/1748-9326/aa914d
Reinert, C., Deutz, S., Minten, H., Dörpinghaus, L., von Pfingsten, S., Baumgärtner, N., et al. (2021). “Environmental impacts of the future german energy system from integrated energy systems optimization and dynamic life cycle assessment,” in Proceedings of 30th European Symposium on computer aided process engineering (ESCAPE 2020) (virtual), Milano, Italy, May 24–27, 2020 (Amsterdam, Netherlands: Elsevier).
Ringkjøb, H., Haugan, P., and Solbrekke, I. (2018). A review of modelling tools for energy and electricity systems with large shares of variable renewables. Renew. Sustain. Energy Rev. 96, 440–459. doi:10.1016/j.rser.2018.08.002
Ruhnau, O., Bannik, S., Otten, S., Praktiknjo, A., and Robinius, M. (2019). Direct or indirect electrification? A review of heat generation and road transport decarbonisation scenarios for Germany 2050. Energy 166, 989–999. doi:10.1016/j.energy.2018.10.114
Simoes, S., Nijs, W., Ruiz, P., Sgobbi, A., Radu, D., Bolat, P., et al. (2013). The JRC-EU-TIMES model: assessing the long-term role of the SET plan energy technologies volume 26292 of EUR, Scientific and technical research series. Luxembourg, Netherlands: Publications Office of the European Office.
Spittler, N., Gladkykh, G., Diemer, A., and Davidsdottir, B. (2019). Understanding the current energy paradigm and energy system models for more sustainable energy system development. Energies 12, 1584. doi:10.3390/en12081584
The Royal Society (2019). Sustainable synthetic carbon based fuels for transport: policy briefing. London, United Kingdom: The Royal Society.
Turconi, R., Tonini, D., Nielsen, C., Simonsen, C., and Astrup, T. (2014). Environmental impacts of future low-carbon electricity systems: detailed life cycle assessment of a Danish case study. Appl. Energy 132, 66–73. doi:10.1016/j.apenergy.2014.06.078
Verkehrswende, A. (2018). The future cost of electricity-based synthetic fuels: conclusions drawn by Agora Verkehrswende and Agora Energiewende. Berlin, Germany: Agora Verkehrswende, Agora Energiewende and Frontier Economics.
Victoria, M., Zhu, K., Brown, T., Andresen, G. B., and Greiner, M. (2019). The role of storage technologies throughout the decarbonisation of the sector-coupled european energy system. Energy Convers. Manag. 201, 111977. doi:10.1016/j.enconman.2019.111977
Volkart, K., Mutel, C. L., and Panos, E. (2018). Integrating life cycle assessment and energy system modelling: methodology and application to the world energy scenarios. Sustain. Prod. Consum. 16, 121–133. doi:10.1016/j.spc.2018.07.001
Volkart, K., Weidmann, N., Bauer, C., and Hirschberg, S. (2017). Multi-criteria decision analysis of energy system transformation pathways: a case study for Switzerland. Energy Policy 106, 155–168. doi:10.1016/j.enpol.2017.03.026
Weitemeyer, S., Kleinhans, D., Wienholt, L., Vogt, T., and Agert, C. (2016). A European perspective: potential of grid and storage for balancing renewable power systems. Energy Technol. 4, 114–122. doi:10.1002/ente.201500255
Keywords: integrated assessment modeling, burden shifting, energy transition, linear programming, life-cycle assessment, design and operation optimization
Citation: Baumgärtner N, Deutz S, Reinert C, Nolzen N, Kuepper LE, Hennen M, Hollermann DE and Bardow A (2021) Life-Cycle Assessment of Sector-Coupled National Energy Systems: Environmental Impacts of Electricity, Heat, and Transportation in Germany Till 2050. Front. Energy Res. 9:621502. doi: 10.3389/fenrg.2021.621502
Received: 26 October 2020; Accepted: 19 January 2021;
Published: 08 April 2021.
Edited by:
Fu Zhao, Purdue University, United StatesReviewed by:
Brian Tarroja, University of California, Irvine, United StatesStanislav Martinat, Institute of Geonics (ASCR), The Czech Republic
Copyright © 2021 Baumgärtner, Deutz, Reinert, Nolzen, Kuepper, Hennen, Hollermann and Bardow This is an open-access article distributed under the terms of the Creative Commons Attribution License (CC BY). The use, distribution or reproduction in other forums is permitted, provided the original author(s) and the copyright owner(s) are credited and that the original publication in this journal is cited, in accordance with accepted academic practice. No use, distribution or reproduction is permitted which does not comply with these terms.
*Correspondence: André Bardow, abardow@ethz.ch
†These authors have contributed equally to this work