- 1School of Management, Nanjing University of Posts and Telecommunications, Nanjing, China
- 2School of Economics and Management, Harbin Engineering University, Harbin, China
With the increasing difficulties associated with heating, the new energy industry has become the mainstay for property development. The effective diffusion of leading technologies supplies a social edge for enterprise core technologies, and this is also a necessary topic for industrial transformation and optimization. Within the international context of energy conservation and emission reduction, the scientific and in-depth study of the diffusion mechanisms underlying leading technologies in the new energy industry have vital theoretical significance for the promotion of the diffusion of leading technologies. Based on the introduction of the Bass model and one extension model, this paper constructs the diffusion model of the new energy industry’s leading technology and analyzes its diffusion mechanism. The identified mechanism indicates that in the case of imperfect market and policy environments, the diffusion of the leading technology of the new energy industry is mainly influenced by the “expected utility” of innovators and the “actual utility” of imitators. The diffusion of the leading technology in innovator enterprises of the new energy industry is mainly affected by the “expected utility,” while the diffusion in imitator enterprises is affected by the “actual utility.” These influences are verified by simulation analysis. Based on the diffusion mechanism, several suggestions are presented for the promotion of the diffusion mechanism of leading technology, with the aim to provide references for the government, industry associations, and enterprises for relevant decision-making.
Introduction
The 24th Meeting of the Parties to the United Nations Framework Convention on Climate Change (UNFCCC) (COP24) was held in Katowice, Poland, from December 2–14, 2018. This is not only the most important meeting after the Paris Climate Conference, but also a key opportunity for determining whether the goals of the Paris Agreement can be achieved. The rules outlined in the Paris Agreement call for an increase of global climate action, and climate finance was the focus of the conference. Director General of Greenpeace International, Jennifer Morgan, called on all countries to shoulder the responsibilities entrusted by the times, and furthermore called for timely and clear climate improvement actions. China has made outstanding achievements in climate improvement actions over the past few years, and has thus reached its 2020 emission reduction target three years earlier. Although the coal consumption has risen again since then, China promised that its greenhouse gas emissions will peak at around 2030. This means that China will continue to increase its efforts toward energy upgrades over the next 10 years, and therefore, will face greater responsibilities and more reform costs (Liu et al., 2017; Zheng and Wang, 2019).
Technology is one of the main manifestations of knowledge. Today, competitiveness is mainly reflected by knowledge, and its efficient diffusion leads toward social progress, enterprise upgrading, and industrial development (Kumar and Agarwala, 2016). The leading technology represents the main technology that can represent the development of the society as a while during a certain period of time. At the same time, it can cause major changes in the technology systems of advanced technology and emerging technologies (Wang et al., 2017). Steam power technology was the leading technology from the end of the 18th century to the middle of the 19th century, while metallurgical technology and power technology were the leading technologies from the middle of the 19th century to the beginning of the 20th century. The technology group that includes electronic technology, biotechnology, and information technology was the leading technologies of the early 20th century (Sun et al., 2016). Since science and technology are rapidly developing in the 21st century, under the background of low-carbon economy, new energy technologies will undoubtedly be part of the leading technologies. The effective diffusion of leading technologies presents a source of social benefits for technological innovation for a country or region (Ortiz, 2019). Technology diffusion is a sub-process of innovation, but technology diffusion is also a completely independent activity process of technology and economic integration (Nicholas et al., 2015). Technology would not impact the economy without the proliferation of innovation. Therefore, effectively promoting the diffusion of the leading technologies of the new energy industry and mastering the diffusion mechanism, based on the leading technologies of the new energy industry, have become strategic issues that need to be resolved over the course of Chinese economic development.
Leading technology is the advanced technology and emerging technology that can represent the main direction of social development in a certain period and cause significant changes in the technology system. As we all know, steam power technology is the leading technology from the end of 18th century to the middle of 19th century; Metallurgical technology and electric power technology are the leading technologies from the mid-19th century to the early 20th century; The technology group including electronic technology, biotechnology and information technology, is the leading technology in the early 20th century. With the rapid development of science and technology in the 21st century, new energy technology under the background of low-carbon economy has undoubtedly become one of the leading technologies. With the development of the times, the new energy industry has entered the 21st century, and is rising rapidly all over the world. It has been listed as one of the nine strategic emerging industries in China. At the macro level, all the industrial chains related to new energy belong to the category of new energy industry; At the micro level, only the production and R & D of new energy belong to the new energy industry.
Scholars from different countries often have a different understanding of leading technologies because of their different perspectives. The main views can be divided into two classes. A number of researchers justify the meaning of the dominant technology from a single technology. In this context, dominant technology refers to the stable state of an existing technology system that may be broken into a certain period, and that will trigger technological changes, innovations, and even industrial changes in different fields. The technology of a series activity features a decisive significance for technological advancement, and is the premise and key force for the development of a new technology system (Ahn, 2018; Nascimento et al., 2009). Leading technology has several distinct characteristics, such as its advanced nature and leading nature, which conjointly becomes the elementary condition for dominant market norms (Sharif and Kabir, 1967). Aleksios suggested that the emergence of a dominant technology could be a watershed within technology and business evolution. As a result, the determination of dominant technology is expanded if an enterprise effectively solves the problems associated with technological uncertainty and enterprise maturity. The process of the determinant dominant technology is typically between changes within the nature of technology, changes in the competitive position, and changes in shopper cluster adoption ways (Giovangis and Skiadas, 1999; Jun et al., 2002; Jun, 2018). Other researchers support the connotation of leading technology from the level of technology integration. The alleged dominant technology could be the product technology system enterprises and customers expect within the dynamical market setting at a relevant time (Narin, 1994; Joung and Kim, 2017; Hu et al., 2018). Furthermore, the leading technologies could unite related technologies, which may lead to a combination of the replacement leading technology or a new technological system. This can evoke technological modification, cause changes within the technology-economic model, and may induce a replacement semi-permanent economic cycle (Jaffe et al., 1993; Jaffe and Trajtenberg, 1999; Néstor and María Teresa, 2015). A number of researchers perceive this technology as the dominant design, i.e., during a certain period, several single technological innovations are integrated. The interaction between technology and the market is reflected by merchandise, where single technologies are dominant designs.
In recent years, researchers have conducted connected analyses on leading technologies and technology diffusion in the new energy industry. Liu used patents as research objects and utilized input-output analysis methods to build a patent citation matrix, using the Python programming language. The matrix calculates the induction and influence coefficients that enable the identification of core and cutting-edge technologies. The results showed that in the new energy industry, the cutting-edge technology field is the research and development of marine energy technology, while the core technology is solar energy technology, which has a greater impact on the development of the entire new energy field (Liu et al., 2017). Sun used the Kaya equation to determine the core standards of key technologies for new energy industry-energy security and emission reduction. Sun further analyzed the external and internal environment of the Chinese new energy industry technology promotion with a technology diffusion model (Sun et al., 2016). Kumar constructed a technology diffusion model for renewable new energy by discussing multiple innovations, energy efficiency, and support policies for new energy technologies (Kumar and Agarwala, 2016). Malone used existing information to assess governmental strategies and to link the diffusion of new energy technologies to the national surroundings. Empirical research in the United States, Brazil, and Sweden showed that the combination of technological transformation and national environment promotes the successful diffusion of major energy technologies (Malone et al., 2017). Edsand used a comprehensive framework of system functions and landscape factors to identify and analyze many obstacles that hinder the diffusion of wind energy technology in Colombia. Edsand confirmed the value of landscape analysis, and pointed out that landscape factors should be used as endogenous factors to promote energy technology diffusion (Edsand, 2017). Stucki studied how different types of policies affect the diffusion of new energy technologies among enterprises. Stucki investigated 1,200 new energy companies of Switzerland and found that energy taxes are an effective policy tool that aids the diffusion of new energy technologies among enterprises (Stucki and Woerter, 2016).
The related ideas of the Bass model cannot be used to verify the analysis direction of the diffusion theory; however, using them can provide an additional search basis for the diffusion theory. Therefore, the Bass model has landmark significance for the research of the diffusion model. In the decades following the appearance of the Bass model, most reported diffusion models were based on it. Bass combined a predictive consumer product diffusion model with a logistic model (Bass, 1969). The resulting model assumes that new product companies are affected by the above two modes of communication, which is based on Rogers’five classifications of companies: innovative adopters, early adopters, early majority, late majority, and laggard (Bass, 1980). In terms of the extension of the Bass model for technology diffusion, the technology innovation model (TIM) proposed by Tse not only added the factor of new technology product suppliers, but also proposed that for new technology holders, curiosity is a key driving force behind successful technology diffusion (Tse and Lau, 1997). Chen introduced more microscopic details, and established a new Bass extension model based on the theory of supply and demand in the Tse model. This new Bass extension includes differential equations of three state variables X, Y, and Z, and is commonly referred to as the XYZ model (Chen, 2008). In his research, Chen pointed out that the essence of technology diffusion is the decision of whether technology suppliers trade or not, based on profit, and whether potential adopters adopt or not, based on utility maximization. This is particularly important for the decision of technology suppliers, which may impact the final result of the diffusion. At the same time, Chen proposed that the value representation of technology products is the realized actual utility and the potential expected utility. Yu-Heng Chen used BASS model to present the technological S-curves and identified the optimal patent strategy (Chen et al., 2011). Baur article used the methodology of System Dynamics and the theory of Bass model to develop a model of the German photovoltaic market for small plants on private houses and tests public policies (Baur and Uriona, 2018). According to BASS Model, Kapur developed a parsimonious and innovative model that captures the dynamics of new product diffusion in the recent high-technology markets (Kapur et al., 2019). Singhal examined the problem of stochasticity in predicting the adoption growth pattern of technological innovations based on BASS model (Singhal et al., 2020).
Many researchers have recognized the necessity of the recent energy industry within the economic development pattern (Li et al., 2021; Ullah et al., 2021; Minuto et al., 2021). Related problems with the new energy industry, with regard to technology diffusion, have received increasing attention from researchers. Analyses of the mechanism of technology diffusion, which supports completely different eventualities, remains in its infancy (Pakravan and MacCarty, 2021; Rezaei-Moghaddam and Far, 2019). Therefore, this paper uses the Bass model to explore the leading technology diffusion mechanism of the new energy industry. The aim is to improve and enrich the theoretical system of technological innovation, which has reference value for new energy companies that face technical decisions. As the new energy industry is an emerging industry, the relevant market environment and policy environment are not perfect, even after the relevant technologies have been integrated into the market at the R&D stage. This restricts their development to a certain extent. Therefore, this paper sets the diffusion of the leading technology of the new energy industry in the background of an imperfect market environment and imperfect policy environment. This imperfect market and policy environment mainly shows that the social infrastructure related to the new energy industry is currently still in its construction period. This makes it unable to provide an external guarantee for the leading technology diffusion of the new energy industry. The atmosphere of relevant technical R&D is slack, and the imperfect infrastructure and the lack of talents lead to the failure to form a good market environment, scientific and technological environment, policies, and systems.
Materials and Methods
Compared with other technologies, the leading technology of new energy industry has more obvious characteristics. In their related research, scholars pointed out that the leading technology of new energy industry has the characteristics of complexity, guidance and externality. The reasons are as follows: 1) The top technology, advanced technology or the integration of multiple technologies are the basis for establishing the leading technology of new energy industry. Therefore, the leading technology of new energy industry has the characteristics of high complexity. 2) At the industrial level, the leading technology of new energy industry represents the development direction of the internal technology frontier of the industry, and plays a guiding role in the formation and development of the country’s future industry, and is conducive to the upgrading of the internal technology of the industry and realizing the leapfrog development. Therefore, the leading technology of new energy industry has guiding characteristics. 3) Due to its relatively important position, the leading technology and related technology of new energy industry are relatively easy to spread and spill over to other departments or fields, which reflects the externality of the leading technology of new energy industry. In the process of model selection and formula derivation, we fully consider these three characteristics of energy technology.
As mentioned before, the incomplete situation of environment is mainly manifested in the construction period of social infrastructure, the relative lack of relevant technical R&D personnel and R&D atmosphere, which can not provide external guarantee for the leading technology diffusion of new energy industry. In this case, the diffusion process of new energy industry’s leading technology is similar to the adoption process of new products in bass diffusion model, so this paper chooses Bass model to study the diffusion mechanism of new energy industry’s leading technology under the incomplete environment. The original hypothesis of the BASS model is expressed as (1):
Let
The transformed expression (2) is the basic expression of the Bass model.
Let
The basic expression of the Bass model is used to enrich the impact of external environmental factors on the diffusion of leading technologies in new energy industry. Because the diffusion of the leading technology of new energy industry will be greatly affected by the external environment, the paper adds environmental elements to the diffusion model of the leading technology of new energy industry. Considering that scholars use markets and policies as environmental factors when they use the Bass model to study technology diffusion within the industry, the research in this paper mainly considers the environmental impact of market environment and policy environment on the dominant technology diffusion of new energy industries. The paper comprehensively considers the innovation coefficient
Substituting (4) and (5) into the basic expression of the Bass model (2) yields (6), and after collation, it yields (7):
From Equation 7, when
Let Y be the diffusion ratio of a new technology; E is the environmental factor of the heterogeneous diffusion policy; S is the expected utility of the market;
Equation 8 represents that diffusion depends on the technology absorber as an innovator and imitator. The innovators are the first companies to try new technologies. Their decision basis is based only on the expected market utility of the technology. The bigger is
Results
Leading Technology Diffusion Mechanism of the New Energy Industry Based on the Bass Model
Based on the above analysis, this paper proposes the diffusion mechanism of the leading technology of the new energy industry. The expected utility S of the innovator and the actual utility X of the imitator exert a decisive influence on the large-scale diffusion of the leading technology of the new energy industry. When technology-holding companies choose to promote new technologies, as the first group of innovators who boldly try new technologies, enterprises decide whether to adopt these leading technologies based on their expected market utility. Because of the complexity of the dominant technology, the cost of adopting a technology that includes information from multiple fields will be higher than the cost of adopting a general technology. In this situation, at the early stage when a new technology enters the market, because of the imperfect market environment, the transaction mechanism related to the leading technology has not yet been completed. Therefore, the technology absorbing party has to pay a higher cost. Companies with stronger comprehensive strength commonly have more abundant capital and can thus bear the cost of technology adoption. Because of the imperfect policy environment, relevant policies related to the leading technologies of the new energy industry have not yet been promulgated. Alternatively, there may be regulations but no relevant implementation plans have been introduced yet, which has hindered the market-oriented development of this technology. The professional and technical personnel of stronger enterprises has the ability to accelerate the process of technology marketization and let new technologies quickly enter a profitable state. Moreover, companies with stronger comprehensive strength as innovators can also provide imitators with higher practical utility X, thus promoting the diffusion of leading technologies in the new energy industry.
The diffusion of dominant technologies into imitator enterprises depends on the difference between the actual utility X of the innovator enterprise and the expected utility S of the imitator enterprise itself. Following the innovator, the imitator enterprise determines the expected market utility of the leading technology based on the actual utility generated by the innovator enterprise, and then decides whether to adopt it. Leading technologies will be shared by multiple industries because of their externalities. Because of the differences between industries, companies will base different standards when determining their expected utility. In this case, the scientific evaluation system of a new technology within the enterprise plays a key role. If companies can scientifically and comprehensively consider new technologies, their risk of adopting new technologies will greatly decrease. Therefore, the scientific evaluation of new technologies is conducive to the diffusion of leading technologies in imitating enterprises. In contrast, inconsiderate adoption will lead to technology application failures in the enterprise, which will interrupt the subsequent diffusion of leading technologies. Therefore, the expected utility S of the innovator and the actual utility X of the imitator are clearly the prerequisites for promoting the diffusion of the leading technology of the new energy industry.
Simulation of the Diffusion of Leading Technology in the New Energy Industry
In the paper, MATLAB software was used to simulate the diffusion mechanism of the new energy industry leading technology. MATLAB software is widely used for numerical calculations. According to its literal meaning (i.e., “matrix laboratory,”) it can form a visual graph of a matrix calculation, numerical analysis, and other processes, thus making the modeling and simulation experiment of a nonlinear dynamic system more convenient and efficient. Therefore, this paper uses MATLAB software to simulate the diffusion model of new energy industry’s leading technology, to clarify the diffusion process of the new energy industry’s leading technology.
The simulation in this paper is divided into two parts: First, the effect of the expected utility of innovators on the number of innovator enterprises is simulated; second, the effect of the difference between the actual utility and the expected utility on the imitator enterprises is simulated.
In the first part of the simulation, the total number of innovators is 400. To explore the diffusion of the leading technology of the new energy industry in government oriented, market-oriented, and hybrid-oriented modes, this paper simulates the diffusion curves for v = 0, w = 0.5, v = 0.5, w = 0, and v = 0.5, w = 0.5, respectively. Based on the research of Hao, the expected utility s of innovators is increased, taking 0.3 and 0.5. The simulation experiment contains random characteristics; therefore, in the process of numerical simulation, this paper uses two groups of parameters to repeat the experiment 10,000 times. Each time, 50 time steps are run, and the average value of the cumulative number of innovators in each time step is taken. This yields the curve of innovators’ changes caused by expected utility changes of innovators, as shown in Figure 1.
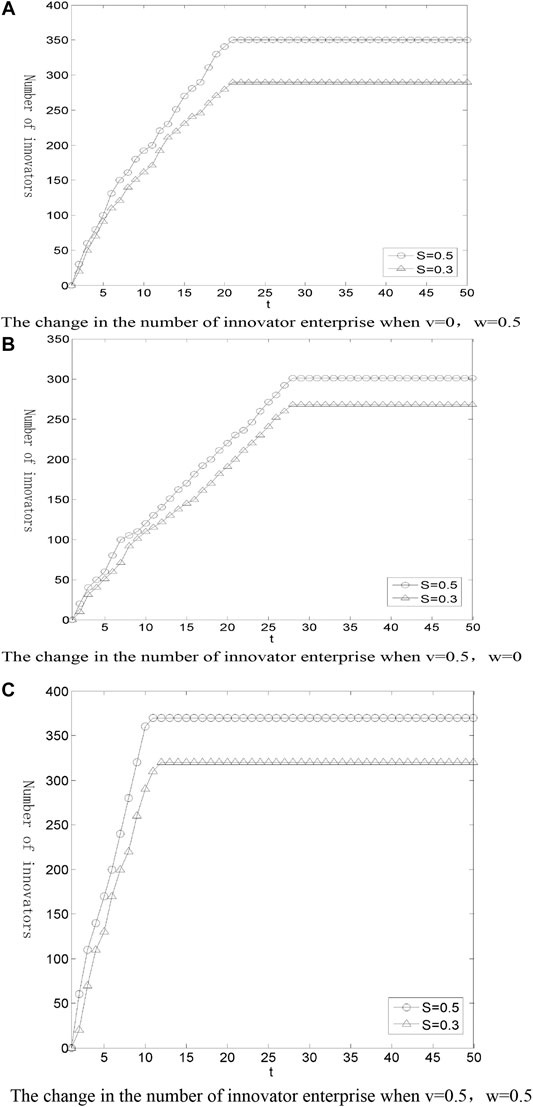
FIGURE 1. The influence of the innovator’s expected utility S on the innovator enterprise. (A) The change in the number of innovator enterprise when v = 0, w = 0.5. (B) The change in the number of innovator enterprise when v = 0.5, w = 0. (C) The change in the number of innovator enterprise when v = 0.5, w = 0.5.
To study the influence of the difference between the actual utility of innovators and the expected utility of imitators on the number of imitators, this paper assumes a total number of imitators of 400, and the diffusion curves are simulated for v = 0, w = 0.5, v = 0.5, w = 0.5, and v = 0.5, w = 0.5. The paper sets the innovator’s actual utility x = 0.5, and the imitator’s expected utility s to 0.8 and 0.6, respectively. Because the simulation experiment will contain certain random characteristics, in the process of the numerical simulation, this paper uses two groups of parameters to repeat the experiment 10,000 times. Each time, 50 time steps are run, the average value of the accumulated number of imitator enterprises in each time step is calculated. Then, the curve of imitator enterprise changes caused by the difference between the actual utility and the expected utility is obtained, as shown in Figure 2.
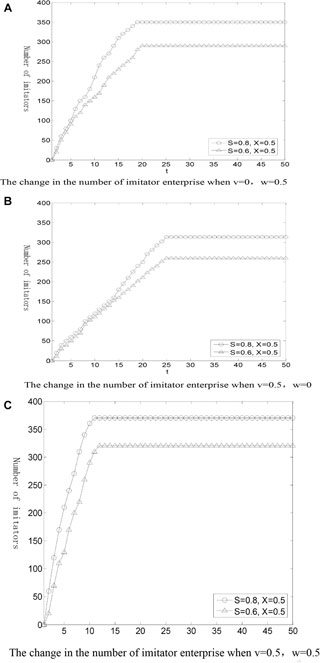
FIGURE 2. Influence of the difference between the expected utility S and the actual utility X of the imitator enterprise on the number of imitator enterprises. (A) The change in the number of imitator enterprise when v = 0, w = 0.5. (B) The change in the number of imitator enterprise when v = 0.5, w = 0. (C) The change in the number of imitator enterprise when v = 0.5, w = 0.5.
Discussion
Figure 1 shows that the expected utility of innovators has a more obvious impact on the peak number of innovators and the time to reach the peak. When v = 0.5, w = 0, see Figure 1A, the number of innovators grows slowest, and the peak number of innovators is lowest. This shows that because of the imperfect policy environment (e.g., the relevant policies of the new energy industry leading technology have not been issued, or only relevant laws and regulations but no specific implementation plan have been issued), the market-oriented development of technology is hindered. This results in the slow diffusion progress of new energy industry leading technologies. When v = 0, w = 0.5, see Figure 1B, the growth rate of the number of innovators is average, and the peak of the number of innovators is also average. This shows that because of the imperfect market environment, the transaction mechanism related to the leading technology has not been completed, and the technology absorbing party has to pay a high cost. Only when enterprises with high comprehensive strength take the lead as innovators, the leading technology of the new energy industry can spread smoothly. When v = 0.5, w = 0.5, see Figure 1C, the number of innovators grows fastest, and the peak number of innovators is highest. This shows that when the development of both market environment and policy environment is good, an enterprises with strong comprehensive strength can obtain the relevant information of the leading technology of new energy industry in time and can complete the transaction quickly with the support of a relevant policy environment. Under the support of market conditions, the leading technology of new energy industry can be quickly translated into production and relevant products can be improved continuously. This promotes the leading technology of the new energy industry effective diffusion of operation. Therefore, the results of the simulation verify that the higher expected utility of innovators can promote the diffusion of new energy leading technology.
Figure 2 indicates that the difference between the actual utility of innovators and the expected utility of imitators exerts a significant impact on the peak number of imitators and the time to reach the peak. When v = 0.5, w = 0, see Figure 2A, the growth rate of imitator enterprises is slowest, and the peak value of imitator enterprises is lowest. This shows that because of the imperfect policy environment, the actual utility of innovator enterprises is low. Consequently, imitator enterprises cannot obtain favorable information to adopt technology, and thus, the expected utility of imitator enterprises for leading technology is also low. This hinders the diffusion of leading technology in the new energy industry. When v = 0, w = 0.5, see Figure 2B, the growth rate of imitator enterprises and the peak of imitator enterprises are average. This shows that because of the relative improvement of the policy environment, several innovators can obtain better benefits after adopting the leading technology. However, because of the relatively backward development of the market environment, if the enterprises determine the expected utility only according to market demand, they cannot produce higher expected utility for the leading technology. Therefore, the objective and scientific evaluation mechanism for the new technology enables enterprises to obtain a more reasonable expected utility, thus promoting the effective diffusion of leading technologies in the new energy industry. When v = 0.5, w = 0.5, see Figure 2C, the number of imitators grows fastest, and the peak number of imitators is highest. This shows that a better market environment and policy environment generally enable innovator enterprises to obtain higher actual utility. The broad market prospect and strong policy support can cause the imitator enterprises to produce higher estimated utility, thus accelerating the rapid and effective diffusion of the leading technology of the new energy industry. Therefore, the simulation results verify that the difference between the actual utility of innovators and the expected utility of imitators can promote the diffusion of new energy leading technologies.
Conclusion
This paper first builds a diffusion model of the leading technology of the new energy industry based on the introduction of the Bass model and its extended model. Furthermore, the diffusion mechanism of the leading technology of the new energy industry is analyzed. The diffusion of leading technologies is mainly affected by the “expected utility” of innovator firms and the “actual utility” of imitator firms, and has been verified via simulation analysis. Based on the results, relevant countermeasures and suggestions are proposed from the perspectives of governments, industry associations, and enterprises. These suggestions provide scientific and effective guidance for the diffusion of leading technologies in the new energy industry.
The government can promote the diffusion of leading technologies in the new energy industry in the following two ways:
1) Formulate and promulgate patent protection policies and regulations related to leading technology products. The aim is to improve the policy environment and market environment for the diffusion of leading technologies in new energy industry, which can ensure the effective diffusion of leading technologies. Because of the complexity of the dominant technology and the lack of relevant policies and regulations, the technical transaction process of dominant technology is relatively long. A variety of conditions that lead to transaction failures will occur during this period. The government should assume the active role of the intellectual property protection mechanism and establish a dual system of patent administrative protection and judicial protection, i.e., a “dual-track system” protection mode. The government should cooperate with administrative agencies, including patents, industry and commerce, technical supervision, and customs, to gradually form a unified evaluation standard for various cases of intellectual property transactions. Furthermore, the government should exercise sanctions against infringers in infringement disputes by exercising administrative power. The various regulations of the patent protection law should be improved and implemented, so that law enforcement agencies can protect the interests of rights holders through judgments in accordance with laws and regulations. This not only ensures the compliance of transactions, but also suppresses malicious illegal acts, thus effectively promoting the diffusion of leading technologies in the new energy industry.
2) Focus on cultivating innovator companies in the new energy industry and enhancing their overall strength to increase the expected utility of imitator companies. Because of the high cost of technology adoption and the imperfect market environment, few companies have actively adopted the leading technologies of the new energy industry. Therefore, the government should select specific companies with high-tech R&D and application capabilities to focus on training, and policy support for technology promotion at both the national and local levels. For example, by providing funds and tax reductions, companies with stronger comprehensive strength should be encouraged to take the lead in technology research and development and technology introduction, to thus increase the comprehensive strength of enterprises and increase their profits as innovator companies in the transformation of leading technologies in the new energy industry. Furthermore, the expected utility of imitator companies should be increased to adopt leading technologies, and these companies should play a leading and demonstrative role in the adoption of leading technologies. Consequently, they can promote the rapid diffusion of leading technologies within the new energy industry.
Industry associations can promote the diffusion of leading technologies in the new energy industry in the following two ways:
1) Industry associations should strive to promote the division of labor and cooperation between innovators and imitators in the industry, to increase the expected utility of innovators and the actual utility of imitators. For fast-developing innovator companies, resources should be allocated with a focus on technological breakthroughs to encourage them to play a demonstrative role for adopting leading technologies. Imitator companies with relatively weak overall strength should be encouraged to strengthen and innovate. Exchange meetings or forums should be held in the name of industry associations to promote technological exchanges and cooperation between innovator and imitator companies. Furthermore, imitator companies should be guided to selectively adopt leading technologies based on their own strengths, thus promoting the stable diffusion of new technologies.
2) Establish an information service platform within the industry to promote the flow of information related to the leading technology of the new energy industry; consequently, imitator companies can quickly obtain information on how the innovator companies use the leading technology. Industry associations should establish a dedicated information service platform, integrate information resources related to the leading technology of the new energy industry, and establish a service organization that provides the latest information both in China and internationally. Furthermore, domestic new energy companies should be enabled to grasp important information related to the leading technology in a timely manner to correctly predict the effectiveness of leading technologies. Industry associations should also provide technical services for new energy companies, and gradually build and improve the dynamic information service system related to the leading technology of the new energy industry. Moreover, industry associations should promote the exchange of relevant information both within China and internationally, and ensure the accurate transmission of relevant utility information about the leading technology, with the goal to ensure the process of leading technology diffusion in the new energy industry.
Enterprises should promote the diffusion of leading technologies in the new energy industry with regard to the following two aspects:
Enterprises should start to establish a sound on-the-job training system for employees, improve their own technology application capabilities, increase confidence in the industrialization of leading technology, and increase the expected utility of companies for adopting leading technologies in the new energy industry. New energy enterprises should combine their own technical and production characteristics to formulate a continuing education plan for professional and technical personnel in R&D. The technical personnel should choose the content of continuing education based on the needs to enhance the scientific research capabilities of the technical personnel, thus improving the enterprise’s technology application capabilities. Enterprises should smoothly integrate the leading technology of the new energy industry into the production process. At the same time, relevant departments of new energy companies should actively seek opportunities for project cooperation with Chinese and foreign institutions of higher learning. Young and middle-aged professional and technical backbone-employees should be sent to colleges and universities for further studies, to thus improve the technical strength and scientific research level of the enterprise. The adaptability of technology will increase the expected utility of the leading technology of the new energy industry and thus ensure the smooth diffusion of the leading technology of the new energy industry.
New energy enterprises should establish an effective technology evaluation system, which will enable them to accurately evaluate the effectiveness of leading technologies in the new energy industry based on their own characteristics. Because of the complicated marketization process of leading technologies of the new energy industry, information asymmetry will inevitably occur in the process of technology trading and use. If enterprises determine the expected utility of dominant technology based on the actual utility of an innovator enterprise, an effective technology evaluation system needs to be established. An effective technology evaluation system can also improve the operating efficiency of the technology market, save game funds, time, and energy for both parties of the transaction. Therefore, core enterprises in the industry should lead the establishment of a utility feedback platform to provide specific basis and judgment standards for the adoption and improvement of leading technologies in the new energy industry. At the same time, enterprises of the new energy industry should organize technical staff to establish a technology evaluation system based on their own strengths and enterprise development plans. This can ensure the scientific evaluation of the leading technology, shorten the time of the technology evaluation stage, and promote the smooth implementation of the leading technology adoption process. Consequently, the rapid and effective diffusion of leading technologies in the new energy industry is promoted.
The contributions of this paper are as follows: 1) As an emerging industry, the new energy industry has important strategic significance for replacing the existing oil, coal, and other traditional energies, energy conservation, and environmental protection. Research on the diffusion of the leading technology in the new energy industry provides a theoretical basis for the healthy development and industrial optimization of the new energy industry. 2) From the industrial level, this paper explores both the development law and diffusion mechanism of the leading technology in the new energy industry, which are conducive to improving and enriching the theoretical system of technological innovation. 3) This paper uses simulation analysis to verify the diffusion mechanism of leading technologies in the new energy industry. This approach effectively improved the diffusion level of leading technology in the new energy industry and provides a reference for energy upgrading. The main limitation of this study is that only one model is used to study the mechanism of technology diffusion. In future research, the author will continue to integrate the classic technology diffusion model to more deeply analyze the mechanism of leading technology diffusion in the new energy industry. Furthermore, simulation software is used to simulate the technology diffusion in this paper, which may be different from the actual situation. Practical cases and statistical data will be used to verify the diffusion mechanism in various situations.
Data Availability Statement
The original contributions presented in the study are included in the article/Supplementary Material, further inquiries can be directed to the corresponding author.
Author Contributions
HW is responsible for the writing of the article and the construction of the theoretical model, while BS is responsible for the revision and polishing of the article.
Funding
This research was funded by National Natural Science Fund under Grant, grant numbers 71774035 and 71372020. Research Fund for Humanities and social sciences of Nanjing University of Posts and Telecommunications, grant number is XK0014520010.
Conflict of Interest
The authors declare that the research was conducted in the absence of any commercial or financial relationships that could be construed as a potential conflict of interest.
References
Ahn, Y. S. (2018). Dominant Design Technology Strategy Based on Open Innovation: High Skewed Propeller(HSP) Design and Production System of Hyundai Heavy Industries Co.[J]. J. Inf. Tech. Appl. Manag. 25 (1), 1–17.
Bass, F. M. (1980). The Relationship between Diffusion Rates, Experience Curves, and Demand Elasticities for Consumer Durable Technological Innovation[J]. J. Business 11, 57–67.
Bass, F. M. (1969). A New Product Growth for Model Consumer Durables. Manag. Sci. 15, 215–227. doi:10.1287/mnsc.15.5.215
Baur, L., and Uriona, M. (2018). Diffusion of Photovoltaic Technology in Germany: A Sustainable success or an Illusion Driven by Guaranteed Feed-In Tariffs? Energy 150, 289–298. doi:10.1016/j.energy.2018.02.104
Chen, X. (2008). Extended Research on Bass model[D]. Dalian: Doctoral dissertation of Jinan University.
Chen, Y.-H., Chen, C.-Y., and Lee, S.-C. (2011). Technology Forecasting and Patent Strategy of Hydrogen Energy and Fuel Cell Technologies. Int. J. Hydrogen Energ. 36 (12), 6957–6969. doi:10.1016/j.ijhydene.2011.03.063
Edsand, H.-E. (2017). Identifying Barriers to Wind Energy Diffusion in Colombia: A Function Analysis of the Technological Innovation System and the Wider Context. Tech. Soc. 49, 1–15. doi:10.1016/j.techsoc.2017.01.002[
Giovangis, A. N., and Skiadas, C. H. (1999). A Stochastic Logistic Innovation Diffusion Model Studying the Electricity Consumption in greece and the United States[J]. Technol. Forecast. Soc. Change 61, 235–246.
Hu, J., Li, S., Yao, Y., Yu, L., Yang, G., and Hu, J. (2018). Patent Keyword Extraction Algorithm Based on Distributed Representation for Patent Classification. ENTROPY 20 (2), 104–116. doi:10.3390/e20020104
Jaffe, A. B., Trajtenberg, M., and Henderson, R. (1993). Geographic Localization of Knowledge Spillovers as Evidenced by Patent Citations. Q. J. Econ. 108, 577–598. doi:10.2307/2118401
Jaffe, A. B., and Trajtenberg, M. (1999). International Knowledge Flows: Evidence from Patent Citations. Econ. Innovation New Tech. 8, 105–136. doi:10.1080/10438599900000006
Joung, J., and Kim, K. (2017). Monitoring Emerging Technologies for Technology Planning Using Technical Keyword Based Analysis from Patent Data. Technol. Forecast. Soc. Change 114, 281–292. doi:10.1016/j.techfore.2016.08.020
Jun, D. B., Kim, S. K., Park, Y. S., Park, M. H., and Wilson, A. R. (2002). Forecasting Telecommunication Service Subscribers in Substitutive and Competitive Environments. Int. J. Forecast. 18, 561–581. doi:10.1016/s0169-2070(02)00067-5
Jun, S. (2018). Patent Mining Using Beta Regression and R Text Mining. Jkiis 28 (4), 383–387. doi:10.5391/jkiis.2018.28.4.383
Kapur, P. K., Panwar, S., and Singh, O. (2019). Modeling Two-Dimensional Technology Diffusion Process under Dynamic Adoption Rate. JMM 14 (3), 717–737. doi:10.1108/jm2-06-2018-0088
Kumar, R., and Agarwala, A. (2016). Renewable Energy Technology Diffusion Model for Techno-Economics Feasibility. Renew. Sust. Energ. Rev. 54, 1515–1524. doi:10.1016/j.rser.2015.10.109
Li, Y., Chen, Y., and Wang, Q. (2021). Evolution and Diffusion of Information Literacy Topics. Scientometrics 12, 1–30. doi:10.1007/s11192-021-03925-y
Liu, J.-h., Meng, Z., and Jiang, Z.-h. (2017). Analysis on Core Technologies and Cutting-Edge Technologies of New Energy Based on Input-Output Method. Proced. Eng. 174, 1036–1045. doi:10.1016/j.proeng.2017.01.256
Malone, E., Hultman, N. E., Anderson, K. L., Romeiro, V., and Romeiro, V. (2017). Stories about Ourselves: How National Narratives Influence the Diffusion of Large-Scale Energy Technologies. Energ. Res. Soc. Sci. 31, 70–76. doi:10.1016/j.erss.2017.05.035
Minuto, F. D., Lazzeroni, P., Borchiellini, R., Olivero, S., Bottaccioli, L., and Lanzini, A. (2021). Modeling Technology Retrofit Scenarios for the Conversion of Condominium into an Energy Community: An Italian Case Study. J. Clean. Prod. 282, 124536. doi:10.1016/j.jclepro.2020.124536
Nascimento, P. T. D., Yu, A. S. O., Nigro, F., et al. (2009). “The Case of Magneti Marelli Brasil: Endogenous and Exogenous Factors in Local Dominant Technology Development[J],” in Proceedings of Portland International Conference on Management of Engineering and Technology, 3122–3132.
Néstor, D. B., and María Teresa, C. C. (2015). The Diffusion of Patented Oil and Gas Technology with Environmental Uses: A Forward Patent Citation Analysis[J]. Energy Policy 83, 267–276. doi:10.1016/j.enpol.2015.03.001
Nicholas, A., Lyda, B., and Jack, A. N. (2015). Dominant Designs, Innovation Shocks, and the Follower's Dilemma[J]. Strateg. Manag. J. 36 (2), 216–234.
Ortiz, M. R. (2019). Leading-Following with Theory and Technology. Nurs. Sci. Q. 32 (4), 326. doi:10.1177/0894318419867328
Pakravan, H., and MacCarty, N. (2021). An Agent-Based Model for Adoption of Clean Technology Using the Theory of Planned Behavior. J. Mech. Des. 143, 021402. doi:10.1115/1.4047901
Rezaei-Moghaddam, K., and Far, S. (2019). The Impact Assessment of Technologies Diffusion: a Mixed Methods Analysis. Chem. Biol. Tech. Agric. 6, 24. doi:10.1186/s40538-019-0162-3
Sharif, M. N., and Kabir, C. (1967). A Generalized Model for Forecasting Technological Substitution[J]. Technol. Forecast. Soc. Change 8, 353–364.
Singhal, S., Anand, A., and Singh, O. (2020). Studying Dynamic Market Size-Based Adoption Modeling & Product Diffusion under Stochastic Environment. Technol. Forecast. Soc. Change 161, 120285. doi:10.1016/j.techfore.2020.120285
Stucki, T., and Woerter, M. (2016). Intra-firm Diffusion of green Energy Technologies and the Choice of Policy Instruments. J. Clean. Prod. 131, 545–560. doi:10.1016/j.jclepro.2016.04.144
Sun, M., Gao, C., Jia, C., Ni, F. D. F., and Zhang, J. (2016). The Selection and Promotion of Core Technology to China's Energy Goals. Energ. Proced. 104, 233–238. doi:10.1016/j.egypro.2016.12.040
Tse, H.-F., and Lau, C.-P. (1997). Long-Term Effect of Right Ventricular Pacing on Myocardial Perfusion and Function. J. Am. Coll. Cardiol. 29 (4), 744–749. doi:10.1016/s0735-1097(96)00586-4
Ullah, N., Mugahed Al-Rahmi, W., Alzahrani, A. I., Alfarraj, O., and Alblehai, F. M. (2021). Blockchain Technology Adoption in Smart Learning Environments. SUSTAINABILITY 13, 1801. doi:10.3390/su13041801
Wang, Z.-X., Zheng, H.-H., Pei, L.-L., and Jin, T. (2017). Decomposition of the Factors Influencing export Fluctuation in China's New Energy Industry Based on a Constant Market Share Model. ENERGY POLICY 109 (109), 22–35. doi:10.1016/j.enpol.2017.06.050
Keywords: new energy industry, leading technology, technology diffusion, bass model, diffusion mechanism
Citation: Wang H and Sun B (2021) Diffusion Mechanism of Leading Technology in the New Energy Industry Based on the Bass Model. Front. Energy Res. 9:586787. doi: 10.3389/fenrg.2021.586787
Received: 04 August 2020; Accepted: 20 May 2021;
Published: 31 May 2021.
Edited by:
Joni Jupesta, Research Institute of Innovative Technology for the Earth, JapanCopyright © 2021 Wang and Sun. This is an open-access article distributed under the terms of the Creative Commons Attribution License (CC BY). The use, distribution or reproduction in other forums is permitted, provided the original author(s) and the copyright owner(s) are credited and that the original publication in this journal is cited, in accordance with accepted academic practice. No use, distribution or reproduction is permitted which does not comply with these terms.
*Correspondence: Hongying Wang, wanghongying0723@hotmail.com