- 1Department of Mechanical Engineering, Federal University of Petroleum Resources, Effurun, Nigeria
- 2Department of Mechanical Engineering Technology, University of Johannesburg, Johannesburg, South Africa
- 3Department of Mechanical Engineering, Olabisi Onabanjo University, Ago Iwoye, Nigeria
- 4Department of Mechanical and Aeronautical Engineering, University of Pretoria, Pretoria, South Africa
- 5Department of Mechanical Engineering, University of Science and Culture, Tehran, Iran
- 6Department of Computer Engineering, Olabisi Onabanjo University, Ago Iwoye, Nigeria
Among the modern computational techniques, Artificial Neural Network (ANN) and Adaptive Neuro-Fuzzy Inference System (ANFIS) are preferred because of their ability to deal with non-linear modelling and complex stochastic dataset. Nondeterministic models involve some computational complexities while solving real-life problems but would always produce better outcomes. For the first time, this study utilized the ANN and ANFIS models for modelling tobacco seed oil methyl ester (TSOME) production from underutilized tobacco seeds in the tropics. The dataset for the models was obtained from an earlier study which focused on design of the experiment on TSOME production. This study is an an exposition of the influence of transesterification parameters such as reaction duration (T), methanol/oil molar ratio (M:O), and catalyst dosage on the TSOME/biodiesel yield. A multi-layer ANN model with ten hidden layers was trained to simulate the methanolysis process. The ANFIS approach was further implemented to model TSOME production. A comparison of the formulated models was completed by statistical criteria such as coefficient of determination (R2), mean average error (MAE), and average absolute deviation (AAD). The R2 of 0.8979, MAE of 4.34468, and AAD of 6.0529 for the ANN model compared to those of the R2 of 0.9786, MAE of 1.5311, and AAD of 1.9124 for the ANFIS model. The ANFIS model appears to be more reliable than the ANN model in predicting TSOME production in the tropics.
Introduction
Notable properties associated with methyl and ethyl esters of oils such as renewability, environmental friendliness, and others, have propelled researchers and scientific communities to substitute such renewable fuels for diesel fuel in diesel and automotive engines (cars, trucks, farm machinery, marine vessels, and even aircraft) (Enweremadu and Mbarawa, 2009; Huang et al., 2012; Huang et al., 2019; Jayaprabakar et al., 2019; Shrivastava and Verma, 2019). In time past, clean and sparkling oily feedstock has been a preferred option for investigation by researchers in terms of biodiesel production since the oils possess the shortest reaction time and do not need pre-treatment prior to the base transesterification (Giwa et al., 2010). However, non-edible seed oils from castor, rubber, jatropha, tobacco seed, and others have been considered for biodiesel production globally. This consideration is associated with non-edible oils as they can lessen the cost of biodiesel production. Among non-edible oils, tobacco seed oil (TSO) seemed appealing for biofuel production which is often observed to possess close basic properties with diesel fuel (Andrianov et al., 2010). In addition, the literature (Giannelos et al., 2002; Moser, 2009) remarked on the viability of biodiesel production from TSO.
The tobacco plant is readily available as it is abundant in over 118 nations globally (Usta, 2005). There is a remarkable growth in tobacco cultivation. The African tobacco plantation grew from 440,000 tons in 2003 to 650,000 tons in 2012. Also, the 20 top-ranked countries (global) in the production of tobacco in 2012 consisted of Malawi (sixth), Tanzania (eight), Zimbabwe (ninth), Zambia (sixteenth), and Mozambique (seventeenth) position (Hu and Lee, 2015). Figure 1 depicts the tobacco production capacity of some countries in 2018 (Statista, 2020), and that of Nigeria in 2017 (FAO statistics, 2008). As observed, China was the largest producer in 2018 while the tobacco plantation in Nigeria was higher compared to those of other countries. There is a need to revamp tobacco farming to meet the feasible production for a biorefinery in the nearest future.
The leaf from the tobacco plant and its seed possess commercial values. For example, the tobacco industry adopts leaves for cigarette production, but alternate use of tobacco by-product is at the top gear (Grisan et al., 2016). The authors further remarked that researchers are projecting alternate application of tobacco such as biofuel. Such reports might be attributed to people’s awareness of the dangerous implication of cigarette smoking (Usta et al., 2011). However, the by-product, which is tobacco seeds contains no nicotine which can be employed for tobacco-based biodiesel production. This will reduce undesirable implications on the health and well-fare of the populace and promote the socio-economic balance in Africa and the world at large (Hu and Lee, 2015).
Refs. (Uzun et al., 2012; Samuel et al., 2020a; 2020b) hinted that optimization is a prerequisite to boost yield and biofuel production in a biorefinery plant. Among high-level optimization techniques, a breed of Artificial Neural Network (ANN) and the Fuzzy Logic (FL), which gives the hybrid algorithm, Adaptive Neuro-Fuzzy Inference System (ANFIS), has been the preferred option in terms of problem-solving, the combination has numerical advantages discussed elsewhere (Okwu and Adetunji, 2018). The ANFIS is a controlling data-driven and adaptive computational tool having the capability of mapping non-linear and complex data (Gupta and Sharma, 2014). On the contrary, the limitation of ANN is its black box which fails to link input parameters with the response. Jang and Sun (1995) linked the failure of the black box technique of the ANN model to the inability of the model to accommodate linguistically information unswervingly. On the other hand, Yaghoobi et al. (2016) attributed the superiority of the ANFIS model to its capacity to handle lapses in the ANN model.
Relevant studies on the TSO biodiesel have been reported by researchers. For instance, Veljkovic et al. (2016) indicated the feasibility of a two‐way process on biodiesel production from TSO having high FFA. Usta et al. (2011) indicated suitable for the antioxidant for TSOME. Sharma et al. (2020) explored RSM for modelling the diesel engine performance parameters of IC engines fuelled with TSOME and diesel blends. However, published literature on ANN-ANFIS based modelling of biodiesels from non-edible oils is scarce. A hybrid of ANN and ANFIS was adopted to optimize biodiesels from sorrel oil (Ishola et al., 2019), palm kernel oil (Betiku et al., 2018), Vitis vinifera seed oil (Hariram et al., 2019), and waste cooking oil, aided with a prepared heterogeneous catalyst (Betiku and Ishola, 2020).
To the best of the authors’ knowledge, a hybrid tool such as ANN integrated with the ANFIS has not been explored to model transesterification parameters of biodiesel production from TSO. On the contrary, the hybrid tool has been employed in other engineering applications. For instance, a handful of exploration of ANN and ANFIS in the distributed system (Okwu and Adetunji, 2018), mechanical properties of concrete (Boğa et al., 2013), wind speed sensor (Rahman and Rahim, 2016), grade estimation (Tahmasebi and Hezarkhani, 2012; Amirkhani et al., 2015), and under convective hot air dryer (Kaveh et al., 2018), sheet hydroforming process (Yaghoobi et al., 2016), etc., have appeared in the literature. Also, the adoption of ANN and ANFIS models are relevant since it strengthens the performance of the model and enables robust modeling for effective productivity and sustainability. Unfortunately, the perusal of the survey showed that there are (1) no established ANN model for TSOME production and (2) comparison capacity of hybrid models such as RSM and ANN models for TSOME’s parameters in the literature are scarce. Hence, there is a need to truncate the lapses in the knowledge of such reports and establish robust models models capable of promoting sustainable production of biodiesel alongside tobacco production in developing countries.
Materials and Method
Data Preparation and Modelling Technique
Creative and intelligent techniques should be adopted for modeling and optimizing limited nonlinear as well as ill-defined engineering problems such as the methylic process. To end this, the ANN and ANFIS were employed herein to model the methylic biodiesel production from TSO on a lab scale. Figure 2 portrays tobacco seeds (TS) as a by-product from leaves of tobacco, and the TS are small but compact together (Giannelos et al., 2002). TSO comprises linoleic acid (C18:2; 73.19 wt %), oleic acid (C18:1; 7.97 wt %), and palmitic acid (C16:0; 11.73 wt %) (Figure 3). As detected in Figures 3 and 4, the overall saturated and unsaturated fatty acids contained in TSO were 73.43 and 26.57 wt %, respectively. TSO having high saturated fatty acid content has a tendency to improve the cetane number of the fuel (Samuel et al., 2020a).
The efficacy of ANN and ANFIS models are indicated in biodiesel productions from palm kernel oil (Betiku et al., 2018), Vitis vinifera seed oil (Hariram et al., 2019), waste cooking oil (Najafi et al., 2018). The theory associated with the models can be found elsewhere (Ighose et al., 2017). Table 1 highlights the databases utilized for the development of ANN and ANFIS models (Waheed et al., 2015).
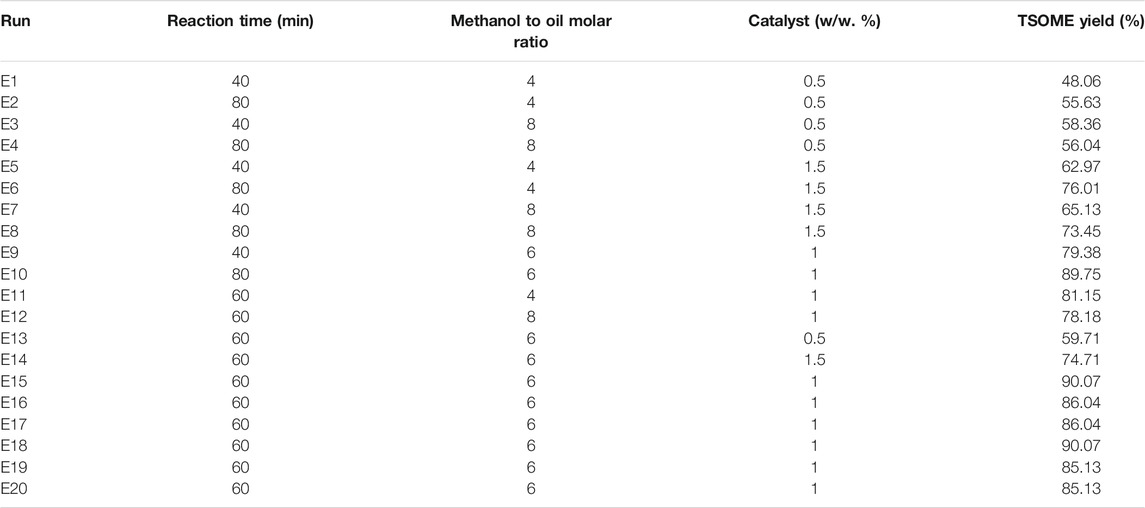
TABLE 1. Dataset for TSOME (Waheed et al., 2015).
The comprehensive information on the employed materials, biodiesel development methodology, and analytical techniques could also be found elsewhere (Waheed et al., 2015). Reaction time, A (40, 60, and 80°C), methanol/oil molar ratio, B (4:1, 6:1, and 8:1), and catalyst amount, C (0.5, 1.0, and 1.5 wt %) were adopted as input variables to the hybrid models. The biodiesel yield/TSOME’s yield was separately adopted as the response of the models. To implement the predictive models, MATLAB® R2019a was employed. The established models for the prediction were the ANN technique based on a multilayer perceptron (MLP) algorithm integrated with the ANFIS.
ANN and ANFIS Modelling
ANN Modelling
The ANN modelling was systematically conducted by using the dataset presented in Table 1. The steps involved in the ANN modelling for TSOME production are presented in the Supplementary Figure S1. The optimum model implemented based on the ANN technique focused on three key steps: (1) optimum neuron value, (2) selection of the appropriate training algorithm, and (3) model testing and validation. Twenty samples of the dataset were considered for the experiment, with 70% allocated for sample training, 15% samples used for validation, and 15% of samples engaged for testing. Utilizing the presented experimental data, the Levenberg–Marquardt (LM) ANN fitting tool and Logistic Sigmoid Activation Transfer Function 3–10–1 (number of the input layer, neurons in the hidden layer, and output layer nodes) model were applied as portrayed in the Supplementary Figure S2. The LM back propagation highlights the process of data training, network inputs, and target/response standardized with a fitted maximum and minimum level. The literature provides details on the adoption of the standardization limits (Aghbashlo et al., 2016; Okpalaeke et al., 2020; Okwu et al., 2020).
ANFIS Modelling
The ANFIS is a high-level creative algorithm obtained by combining the quantitative strength of ANN and the qualitative strength of Fuzzy Logic. The architecture of the ANFIS showing the input variables is presented in the Supplementary Figure S3. The step presented in the fuzzification was such that the crisp value of inputs and output variables was transformed into linguistic terminologies leading to the sectioning of the membership function plot for easy and accurate analysis. The triangular membership function is selected for the input parameters as portrayed in Figure 5. It can be observed that the reaction time (40–80 min), methanol/oil molar ratio (4–8), and catalyst amount (0.5–1.5 wt %) are presented in Figures 5A–C, respectively. The trapezoidal membership function was preferred for the yield of TSOME (Figure 5).
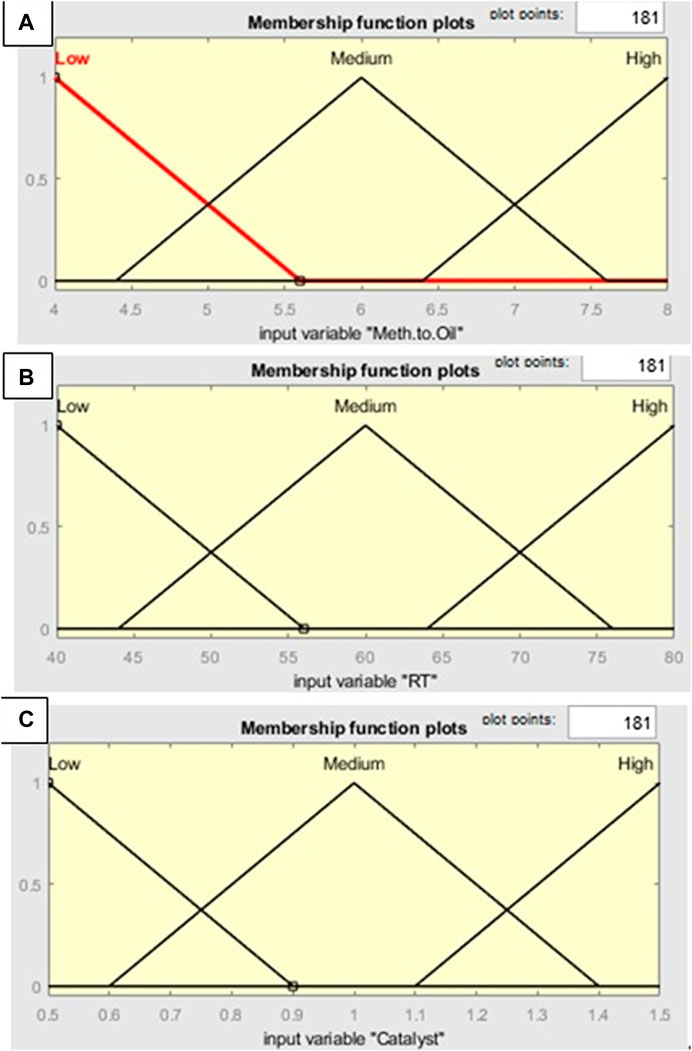
FIGURE 5. Membership function of (A) reaction time, (B) methanol/oil molar ratio and (C) catalyst amount.
The characteristics fuzzy instruction indicated by Ighose et al. (2017) have been accosted for this study. Table 2 presents the number of membership functions and their ranges. From this, fuzzy rules were formulated as given in Table 3 using the input output-data pair specified in Table 1. Thereafter, the defuzzification the yield was completed and presented in Figure 6. To predict the yield of TSOME, a Mamdani-type fuzzy inference system IF-THEN rules were implemented.
The mathematical expression that forecasts the behavior of the dependent parameters was expressed with the aid of Eq. 1 (Quesada et al., 2019).
where mr, nr is the number of rules and number of independent parameters while
Assessment of the Development Models
The precision of both ANN and ANFIS models were investigated with the aid of Eqs. 2–6 as applied by Samuel et al. (2020b).
where Ei,e, Pi,p, and n are the experimental value, predicted value, and several data, respectively. MSE is the mean standard error, MAE is the mean average error, RMSE is the root mean square error, AD is an average deviation, and SEP is the standard error of prediction.
Results and Discussion
ANN Modelling
Employing MATLAB® R2019a, the prediction of TSOME’s yield using ANN has been effectively implemented in this study. Table 2 highlights the grouped available dataset. As obtained, the first sample collection includes 70% dataset for training, 15% for testing, and another 15% for validation. The iteration provided an unsatisfactory result for the first iteration since the MSE value for the training was high. If R-value is close to unity with low MSE values, it becomes appropriate and good for prediction. The iteration performance value was observed until the best values of R and MSE were obtained as shown in Table 4, with the best training value of 0.9909. To achieve the best value as presented in Table 2, the iteration was conducted repeatedly. The best solution was observed for training, testing, and validation at the twenty-fifth iteration with the lowest MSE value and the highest R values.
Figure 7 presents the relationship between the output and target data. As observed, the straight lines correlate the data. Also, the real and expected correlation coefficients (R) are 0.9909 (training), 0.9181 (testing) and 0.9267 (validation). Therefore, in terms of correlation, the prediction of ANN is significant with an overall value of 0.8941, as presented in Figure 8. Figure 9 depicts the best validation performance drawn from the curve of a different epoch. As shown, the best validation performance is 286.02 at the epoch of 1. Error histogram is another tool used to obtain more information about the neural network Figure 10 portrays the error histogram attained for the regression curves for the chosen network. As seen, a good signal of the values of outliers is obtained.
ANFIS Modelling
Figure 11 depicts the architecture of the developed ANFIS model of the membership functions for three inputs (reaction time, methanol/oil molar ratio, and catalyst amount). Figure 12 displays the actual yield and those of ANN and ANFIS models. As seen, the yield predicted by the ANFIS model is closer to the actual TSOME yield compared to the ANN model. Also, the effectiveness of the models was further substantiated through the plots of experimental values against model predicted values (Figure 13). As seen in Figures 13A,B, the linear equations such as (0.9104x + 9.1009) and (1.0401x − 2.6601) are detected to be appropriate for the variations of ANN predicted and actual TSOME yields and ANFIS predicted and actual TSOME yields. The high regression coefficients (R2) of 0.9048 in the ANN model and 0.9496 in the ANFIS model imply that over 90.5 and 95% of the data fit into the respective models. Hence, the ANFIS model could predict the yield better than the ANN model with high accuracy. Similar reports were reported by researchers elsewhere (Betiku and Ishola, 2020; Giwa et al., 2020).
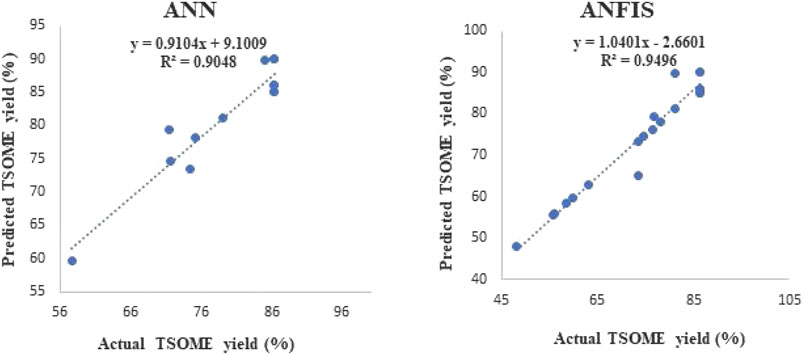
FIGURE 13. Comparison of actual and predicted ANN and ANFIS yield: (A) Actual and ANN predicted yields and (B) actual and ANFIS predicted yields.
Figures 14A–F present the response surface plot of transesterification parameters on the ANFIS predicted TSOME yield in comparison with RSM predicted yield. As observed in Figures 14A,D, TSOME yield remarkably increased from a methanol/oil molar ratio of 4–6 and reaction time of 40–60 min. The yield decreased outside these ranges. Similar observations were documented in the literature by other researchers (Encinar et al., 2010; Betiku et al., 2018). The reduction in TSOME’s yield can be related to the interference of the separation between the esters and glycerol (Samuel et al., 2019). Tabatabaei et al. (2015) and Dhar and Kirtania (2009) hinted that suitable methanol needs to be employed in the transesterification process to minimize the cost of production. Pugazhendhi et al. (2020) attributed the enhanced yield at the range of reaction temperature to maximum activation energy attained during this period. The researchers further stressed that the reduction in TSOME yield at the high temperature is due to the reversal effect of the transesterification reaction. Figures 14 B,E demonstrate the yield of TSOME as a function of catalyst amount and reaction duration (RT). As noticed, TSOME yield remarkably increased from a catalyst amount of 0.5–1.0 wt % and reaction time (RT) of 40–60 min. The yield decreased outside these ranges. A similar observation was reported by researchers elsewhere (Rodrigues et al., 2009; Waheed et al., 2015). This reduction in the TSOME’s yield is ascribed to the triglyceride in the TSO contributing to the soap formation rather than yield enhancement. Figures 14 C,F depict the yield of TSOME as a function of methanol/oil molar ratio (MR) and catalyst amount. As seen, TSOME yield remarkably increased from a catalyst amount of 0.5–1.1 wt % and MR of 4–6. Beyond these ranges, the yield decreased. This observation is further corroborated by Musa (2016) and Dhingra et al. (2016) emphasized that high catalyst amount reduced the yield of TSOME.
Comparative Evaluation of ANN and ANFIS Models
Figure 15 depicts the statistical indices of ANN and ANFIS models. As noticed, the higher R2 (0.978613), lower values of RMSE (3.0635), SEP (4.1370), MAE (1.5311), and AAD (1.9124) for the ANFIS model compared to those of R2 (0.8979), RMSE (7.1026), SEP (9.5916), MAE (4.3446) and AAD (6.0529) for ANN model, establish the superiority of the ANFIS model over the ANN model.
Validation of Optimized Condition for TSOME
Table 5 summarizes the optimal conditions for TSOME. As seen, the yield of TSOME (90.11%) was optimum at the methanol/oil molar ratio of 5.99/1, catalyst dosage of 1.10 wt %, and reaction time of 77.6 min (Waheed et al., 2016). The justification test using the optimized experimental factors produced a predicted for the hybrid yield (90.15%). The average error between the RSM based predicted and that of hybrid model were 0.066 and 0.004%. The validation results showed that the hybrid model developed was precise since the percentages of error in prediction were in a good pact.
Hybrid Models for TSOME and its Comparison with Literature
Table 5 summarizes the ANN-ANFIS models of TSOME yield. As observed, the models of TSOME are comparable with those in the literature (Betiku et al., 2018; Najafi et al., 2018; Hariram et al., 2019). The variation in the hybrid models of the present study with those in literature might be ascribed to transesterification conditions and topologies associated with the ANN model.
Table 6 highlights the overview of R2 of ANN and ANFIS models in the literature and TSOME. As observed, the R2 value of ANN and ANFIS models for TSOME are comparable to those of literature but those of ANN model are lesser than ANFIS (Betiku et al.,2018; Hariram et al., 2019; Betiku and Ishola, 2020).
Fuel Properties of TSOME Produced
Supplementary Table S2 recapitulates the basic properties of TSOME formed. As detected, the density of TSOME (891.1 kg/m3) concurred with the EN 41214 (850–900 kg/m3) standard, though somewhat higher than B0 (850 kg/m3). TSOME having higher density compared to B0 will not significantly influence brake fuel consumption when injected (Niculescu et al., 2019).
The KVs of TSOME (3.87 mm/s2) agreed with the ranges of ASTM D6751 (1.9–6.0 mm2/s) and EN14214 (3.5–5.0 mm2/s), however, it was higher than that of fossil diesel (3.61 mm2/s). Fuels with modest values of KVs will safeguard complete combustion requiring to enhance engine power and reduction in the exhaust emission profile (Samuel and Gulum, 2019). Flash point (FLAP) of TSOME (126°C) is higher than that of diesel fuel (75°C), and it certified the safety requirements for both international standards. A higher value of FLAP diminishes the risk of fire and this property is a benefit of biodiesel over fossil diesel.
The lower calorific value (LCV) of TSOME (42.53 MJ/kg) was lower than that of B0 (43.78 MJ/kg). HSOME possessing LCV can lead to an upsurge in the brake-specific consumption (Xue et al., 2011; Adaileh and AlQdah, 2012; Samuel et al., 2020b).
Conclusion
The study established the application of the ANN model in comparison with the hybrid ANFIS in the modelling of transesterification parameters of biodiesel production from TSO. The suitability of TSOME produced was also verified for its appropriateness in internal combustion engines. The efficacy of the ANN and ANFIS models was assessed based on the statistical indices such as R2, MAE, and AD. The R2 of 0.8979, MAE of 4.3446, and AD of 6.0529 for the ANN model compared to those of the R2 of 0.9786, MAE of 1.5311, and AD of 1.9124 for the ANFIS model. The ANFIS model appears to be more reliable than the ANN model in predicting TSOME production for the tropics. The analysis of basic properties indicated that the produced TSOME is comparable to that of fossil diesel. To get a robust study in the nearest future, (i) other transesterification variables namely stirring speed and reaction time and (ii) the second law of thermodynamics can be explored on the TSOME production can be further investigated.
Data Availability Statement
The original contributions presented in the study are included in the article/Supplementary Material, further inquiries can be directed to the corresponding author.
Author Contributions
OS provided the dataset and wrote the part of the first draft of the manuscript. MO and LT modelled the dataset and wrote part of the first draft of the manuscript. SG and ZO wrote the final draft of the manuscript, which was proofread, improved, and edited by MS. The concept of the study was envisioned by SG and SO.
Conflict of Interest
The authors declare that the research was conducted in the absence of any commercial or financial relationships that could be construed as a potential conflict of interest.
Acknowledgments
The authors are grateful to Pastor Akinola Omotayo and Tayo of Food Science and Technology, Federal University of Agriculture, Abeokuta, Nigeria, for their assistance during the research work.
Supplementary Material
The Supplementary Material for this article can be found online at: https://www.frontiersin.org/articles/10.3389/fenrg.2020.612165/full#supplementary-material.
References
Adaileh, W. M., and AlQdah, K. S. (2012). Performance of diesel engine fuelled by a biodiesel extracted from a waste cocking oil. Energy Procedia. 18, 1317–1334. doi:10.1016/j.egypro.2012.05.149
Aghbashlo, M., Tabatabaei, M., Hosseinpour, S., Hosseini, S. S., Ghaffari, A., Khounani, Z., et al. (2016). Development and evaluation of a novel low power, high frequency piezoelectric-based ultrasonic reactor for intensifying the transesterification reaction. Biofuel Res. J. 3, 528–535. doi:10.18331/BRJ2016.3.4.7
Amirkhani, S., Nasirivatan, S., Kasaeian, A. B., and Hajinezhad, A. (2015). ANN and ANFIS models to predict the performance of solar chimney power plants. Renew. Energy. 83, 597–607. doi:10.1016/j.renene.2015.04.072
Andrianov, V., Borisjuk, N., Pogrebnyak, N., Brinker, A., Dixon, J., Spitsin, S., et al. 2010). Tobacco as a production platform for biofuel: overexpression of Arabidopsis DGAT and LEC2 genes increases accumulation and shifts the composition of lipids in green biomass. Plant Biotechnol. J. 8, 277–87. doi:10.1111/j.1467-7652.2009.00458.x
Betiku, E., and Ishola, N. B. (2020). Optimization of sorrel oil biodiesel production by base heterogeneous catalyst from kola nut pod husk: neural intelligence‐genetic algorithm versus neuro‐fuzzy‐genetic algorithm. Environ. Prog. Sustain. Energy. 39, e13393. doi:10.1002/ep.13393
Betiku, E., Osunleke, A. S., Odude, V. O., Bamimore, A., Oladipo, B., Okeleye, A. A., et al. (2018). Performance evaluation of adaptive neuro-fuzzy inference system, artificial neural network and response surface methodology in modeling biodiesel synthesis from palm kernel oil by transesterification. Biofuels. doi:10.1080/17597269.2018.1472980
Boğa, A. R., Öztürk, M., and Topcu, I. B. (2013). Using ANN and ANFIS to predict the mechanical and chloride permeability properties of concrete containing GGBFS and CNI. Compos. B Eng. 45, 688–696. doi:10.1016/j.compositesb.2012.05.054
Dhar, B. R., and Kirtania, K. (2009). Excess methanol recovery in biodiesel production process using a distillation column: a simulation study. Chem. Eng. Res. Bull. 13, 55–60. doi:10.3329/cerb.v13i2.3538
Dhingra, S., Bhushan, G., and Dubey, K. K. (2016). Development of a combined approach for improvement and optimization of karanja biodiesel using response surface methodology and genetic algorithm. Front. Energy. 7, 495–505. doi:10.1007/s11708-013-0267-5
Encinar, J. M., Martínez, G., González, J. F., and Pardal, A. C. (2010). “Transesterification of rapeseed oil with methanol in the presence of various co-solvents,” in Proceedings, Venice 2010 third international symposium on energy from biomass and waste, Venice, Italy, November 8–11, (Italy: CISA, Environmental Sanitary Engineering Centre) 2010, 1–17.
Enweremadu, C. C., and Mbarawa, M. (2009). Technical aspects of production and analysis of biodiesel from used cooking oil–a review. Renew. Sustain. Energy Rev. 13, 2205–2224. doi:10.1016/j.rser.2009.06.007
Food and Agriculture Organization Statistics (2008). Nigeria: tobacco, production quantity (tons). Available at: http://faostat.fao.org (Accessed August 13, 2020).
Giannelos, P. N., Zannikos, F., Stournas, S., Lois, E., and Anastopoulos, G. (2002). Tobacco seed oil as an alternative diesel fuel: physical and chemical properties. Ind. Crop. Prod. 16, 1–9. doi:10.1016/S0926-6690(02)00002-X
Giwa, S., Chuah, L. A., and Adam, N. M. (2010). Investigating “egusi” (Citrullus Colocynthis L.) seed oil as potential biodiesel feedstock. Energies. 3, 607–618. doi:10.3390/en3040607
Giwa, S., Sharifpur, M., Goodarzi, M., Alsulami, H., and Meyer, J. (2020). Influence of base fluid, temperature, and concentration on the thermophysical properties of hybrid nanofluids of alumina–ferrofluid: experimental data, modeling through enhanced ANN, ANFIS, and curve fitting. J. Therm. Anal. Calorim. doi:10.1007/s10973-020-09372-w
Grisan, S., Polizzotto, R., Raiola, P., Cristian, S., Ventura, F., di Lucia, F., et al. (2016). Alternative use of tobacco as a sustainable crop for seed oil, biofuel, and biomass. Agron. Sustain. Dev. 36, 55. doi:10.1007/s13593-016-0395-5
Gupta, A., and Sharma, D. (2020). A survey on stock market prediction using various algorithms. 2nd international conference on finance, economics, management and IT business.
Hariram, V., Bose, A., and Seralathan, S. (2019). Dataset on optimized biodiesel production from seeds of Vitis vinifera using ANN, RSM and ANFIS. Data Brief. 25, 104298. doi:10.1016/j.dib.2019.104298
Huang, D., Zhou, H., and Lin, L. (2012). Biodiesel: an alternative to conventional fuel. Energy Procedia. 16, 1874–1885. doi:10.1016/j.egypro.2012.01.287
Hu, T. W., and Lee, A. H. (2015). Tobacco control and tobacco farming in African countries. J. Publ. Health Pol. 36, 41–51. doi:10.1057/jphp.2014.47
Huang, D., Zhou, H., and Lin, L. (2019). Biodiesel: an alternative to conventional fuel. Energy Procedia. 16, 1874–1885. doi:10.1016/j.egypro.2012.01.287
Ighose, B. O., Adeleke, I. A., Damos, M., Junaid, H. A., Okpalaeke, K. E., and Betiku, E. (2017). Optimization of biodiesel production from Thevetia peruviana seed oil by adaptive neuro-fuzzy inference system coupled with genetic algorithm and response surface methodology. Energy Convers. Manag. 132, 231–240. doi:10.1016/j.enconman.2016.11.030
Ishola, N. B., Okeleye, A. A., Osunleke, A. S., and Betiku, E. (2019). Process modeling and optimization of sorrel biodiesel synthesis using barium hydroxide as a base heterogeneous catalyst: appraisal of response surface methodology, neural network and neuro-fuzzy system. Neural Comput. Appl. 31, 4929–4943. doi:10.1007/s00521-018-03989-7
Jang, J. S., and Sun, C. T. (1995). Neuro-fuzzy modeling and control. Proc. IEEE. 83, 378–406. doi:10.1109/5.364486
Jayaprabakar, J., Dawn, S., Ranjan, A., Priyadharsini, P., George, R., Sadaf, S., et al. (2019). Process optimization for biodiesel production from sheep skin and its performance, emission and combustion characterization in CI engine. Energy. 174, 54–68. doi:10.1016/j.energy.2019.02.140
Kaveh, M., Sharabiani, V. R., Chayjan, R. A., Taghinezhad, E., Abbaspour-Gilandeh, Y., and Golpour, I. (2018). ANFIS and ANNs model for prediction of moisture diffusivity and specific energy consumption potato, garlic and cantaloupe drying under convective hot air dryer. Inf. Process. Agric. 5, 372–387. doi:10.1016/j.inpa.2018.05.003
Moser, B. R. (2009). Biodiesel production, properties, and feedstocks. In Vitro Cell. Dev. Biol. Plant. 45, 229–266. doi:10.1007/s11627-009-9204-z
Musa, I. A. (2016). The effects of alcohol to oil molar ratios and the type of alcohol on biodiesel production using transesterification process. Egypt. J. Pet. 25, 21–31. doi:10.1016/j.ejpe.2015.06.007
Najafi, B., Ardabili, S. F., Shamshirband, S., Chau, K. W., and Rabczuk, T. (2018). Application of ANNs, ANFIS and RSM to estimating and optimizing the parameters that affect the yield and cost of biodiesel production. Eng. Appl. Comput. Fluid Mech. 12, 611–624. doi:10.1080/19942060.2018.1502688
Niculescu, R., Clenci, A., and Iorga-Siman, V. (2019). Review on the use of diesel–biodiesel–alcohol blends in compression ignition engines. Energies. 12 (7), 1194. doi:10.3390/en12071194
Okpalaeke, K. E., Ibrahim, T. H., Latinwo, L. M., and Betiku, E. (2020). Mathematical modeling and optimization studies by Artificial neural network, genetic algorithm and response surface methodology: a case of ferric sulfate–catalyzed esterification of Neem (Azadirachta indica) seed oil. Front. Energy Res. 8, 614621. doi:10.3389/fenrg.2020.614621
Okwu, M. O., and Adetunji, O. (2018). A comparative study of artificial neural network (ANN) and adaptive neuro-fuzzy inference system (ANFIS) models in distribution system with nondeterministic inputs. Int. J. Eng. Bus. Manag. 10, 1–17. doi:10.1177/1847979018768421
Okwu, M. O., Samuel, O. D., Otanocha, O. B., Tartibu, L. K., Omoregbee, H. O., and Mbachu, V. M. (2020). Development of ternary models for prediction of biogas yield in a novel modular biodigester: a case of fuzzy Mamdani model (FMM), artificial neural network (ANN), and response surface methodology (RSM). Biomass Convers. Biorefin. doi:10.1007/s13399-020-01113-1
Pugazhendhi, A., Alagumalai, A., Mathimani, T., and Atabani, A. E. (2020). Optimization, kinetic and thermodynamic studies on sustainable biodiesel production from waste cooking oil: an Indian perspective. Fuel. 273, 117725. doi:10.1016/j.fuel.2020.117725
Quesada, L., Pérez, A., Godoy, V., Peula, F. J., Calero, M., and Blázquez, G. (2019). Optimization of the pyrolysis process of a plastic waste to obtain a liquid fuel using different mathematical models. Energy Convers. Manag. 188, 19–26. doi:10.1016/j.enconman.2019.03.054
Rahman, M. A., and Rahim, A. H. M. A. (2016). “Performance evaluation of ANN and ANFIS based wind speed sensor-less MPPT controller,” in 2016 5th international conference on informatics, electronics and vision (ICIEV), Dhaka, Bangladesh, May 13–14, 2016, Dhaka, Bangladesh: Institute of Electrical and Electronic Engineers (IEEE).
Rodrigues, S., Mazzone, L. C. A., Santos, F. F. P., Cruz, M. G. A., and Fernandes, F. A. N. (2009). Optimization of the production of ethyl esters by ultrasound assisted reaction of soybean oil and ethanol. Braz. J. Chem. Eng. 26, 361–366. doi:10.1590/S0104-66322009000200013
Samuel, O. D., and Gulum, M. (2019). Mechanical and corrosion properties of brass exposed to waste sunflower oil biodiesel‐diesel fuel blends. Chem. Eng. Commun. 206 (5), 682–682.
Samuel, O. D., Boye, T. E., and Enweremadu, C. C. (2020a). Financial and parametric study of biodiesel production from hemp and tobacco seed oils in modified fruit blender and prediction models of their fuel properties with diesel fuel. Bioresour. Technol. Rep. 12, 100599. doi:10.1016/j.biteb.2020.100599
Samuel, O. D., Okwu, M. O., Amosun, S. T., Verma, T. N., and Afolalu, S. A. (2019). Production of fatty acid ethyl esters from rubber seed oil in hydrodynamic cavitation reactor: study of reaction parameters and some fuel properties. Ind. Crop. Prod. 141, 111658. doi:10.1016/j.indcrop.2019.111658
Samuel, O., Okwu, M., Oyejide, O., Taghinezhad, E., Afzal, A., and Kaveh, M. (2020b). Optimizing biodiesel production from abundant waste oils through empirical method and grey wolf optimizer. Fuel. 281, 118701. doi:10.1016/j.fuel.2020.118701
Sharma, A., Singh, Y., Singh, N., Singla, A., Ong, H., and Chen, H. C. (2020). Effective utilization of tobacco (Nicotiana Tabaccum) for biodiesel production and its application on diesel engine using response surface methodology approach. Fuel. 273, 117793. doi:10.1016/j.fuel.2020.117793
Shrivastava, P., and Verma, T. (2019). An experimental investigation into engine characteristics fueled with Lal ambari biodiesel and its blends. Therm. Sci. Eng. Prog. 17, 100356. doi:10.1016/j.tsep.2019.100356
Statista (2020). Global tobacco production 1990-2018. Available at: https://www.statista.com/statistics/261189/global-tobacco-production-since-1980 (Accessed August 10, 2020).
Tabatabaei, M., Karimi, K., Sárvári Horváth, I., and Kumar, R. (2015). Recent trends in biodiesel production. Biofuel Res. J. 2 (3), 258–267.
Tahmasebi, P., and Hezarkhani, A. (2012). A hybrid neural networks-fuzzy logic-genetic algorithm for grade estimation. Comput. Geosci. 42, 18–27. doi:10.1016/j.cageo.2012.02.004
Usta, N., Aydoğan, B., Çon, A., Uğuzdoğan, E., and Özkal, S. (2011). Properties and quality verification of biodiesel produced from tobacco seed oil. Energy Convers. Manag. 52, 2031–2039. doi:10.1016/j.enconman.2010.12.021
Usta, N. (2005). Use of tobacco seed oil methyl ester in a turbocharged indirect injection diesel engine. Biomass Bioenergy. 28, 77–86. doi:10.1016/j.biombioe.2004.06.004
Uzun, B. B., Kılıç, M., Özbay, N., Pütün, A. E., and Pütün, E. (2012). Biodiesel production from waste frying oils: optimization of reaction parameters and determination of fuel properties. Energy. 44, 347–351. doi:10.1016/j.energy.2012.06.024
Veljkovic, V., Lakic´evic, S., Stamenkovic, O., Todorovic, Z., and Lazic, M. (2006). Biodiesel production from tobacco (Nicotiana tabacum L.) seed oil with a high content of free fatty acids. Fuel. 85, 2671–2675. doi:10.1016/j.fuel.2006.04.015
Waheed, M., Samuel, O., Bolaji, B., and Dairo, O. (2015). “RSM optimization of biodiesel production from tobacco seed oil,” in Renewable energy and sustainable development. (New York: Nova Science Publishers), 85–101
Xue, J., Grift, T. E., and Hansen, A. C. (2011). Effect of biodiesel on engine performances and emissions. Renew. Sustain. Energy Rev. 15 (2), 1098–1116. doi:10.1016/j.rser.2010.11.016
Keywords: artificial neural network, adaptive neuro-fuzzy inference system, biodiesel, modeling, tobacco seed oil
Citation: Samuel OD, Okwu MO, Tartibu LK, Giwa SO, Sharifpur M and Jagun ZOO (2021) Modelling of Nicotiana Tabacum L. Oil Biodiesel Production: Comparison of ANN and ANFIS. Front. Energy Res. 8:612165. doi: 10.3389/fenrg.2020.612165
Received: 30 September 2020; Accepted: 03 December 2020;
Published: 22 January 2021.
Edited by:
Tunde Victor Ojumu, Cape Peninsula University of Technology, South AfricaReviewed by:
Baskar Gurunathan, St. Joseph’s College of Engineering, IndiaAnping Wang, Guizhou Normal University, China
Copyright © 2021 Samuel, Okwu, Tartibu, Giwa, Sharifpur and Jagun.. This is an open-access article distributed under the terms of the Creative Commons Attribution License (CC BY). The use, distribution or reproduction in other forums is permitted, provided the original author(s) and the copyright owner(s) are credited and that the original publication in this journal is cited, in accordance with accepted academic practice. No use, distribution or reproduction is permitted which does not comply with these terms.
*Correspondence: Solomon O. Giwa, solomon.giwa@oouagoiwoye.edu.ng; Olusegun D. Samuel, samuel.david@fupre.edu.ng