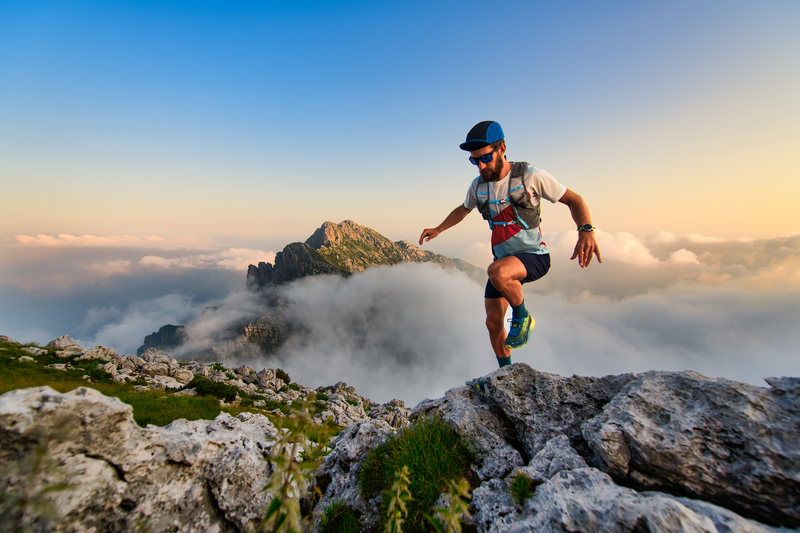
94% of researchers rate our articles as excellent or good
Learn more about the work of our research integrity team to safeguard the quality of each article we publish.
Find out more
ORIGINAL RESEARCH article
Front. Energy Res. , 23 October 2020
Sec. Sustainable Energy Systems
Volume 8 - 2020 | https://doi.org/10.3389/fenrg.2020.00212
This article is part of the Research Topic Energy Market and Energy Transition: Dynamics and Prospects View all 22 articles
Energy is both a basic resource needed for economic growth and an essential tool for economic recovery. The topic of resilience is becoming increasingly prominent in the energy-economic domain and has also entered policy discourse. Yet the measuring method of resilience based on post-disruption events and the relationship between energy consumption and economic recovery are far from settled. This paper develops the idea of resilience and proposes a model to evaluate the economic recovery ability of an economy from the perspective of energy consumption. It also proposes a decoupling model to address the impact of energy-related elements on economic recovery. These ideas are then used for a preliminary empirical analysis of 14 countries against the context of the 2007–2008 financial crisis. The analysis showed that developing countries generally performed better than developed countries, that energy consumption is not a necessity for promoting economic recovery, and that energy-economic decoupling has a positive effect on economic recovery.
Energy is a basic resource needed for economic growth and is an essential tool for economic recovery. Numerous studies have demonstrated the important relationship between energy consumption and economic recovery (Mahadevan and Asafu-Adjaye, 2007; Tang et al., 2016; Destek and Aslan, 2017). Currently, researchers have extended the idea of resilience into the energy-economic domain in order to measure how quickly local and regional economies could recover from shocks and emergencies. The application of resilience into energy-economic study is also in line with the International Energy Agency's (IEA's) definition of national energy security: “short-term energy security focuses on the ability of the energy system to react promptly to sudden changes in the supply–demand balance” (IEA, 2020).
According to the dissipative structure theory, an energy system is an open complicated system, with non-linear interactions among the inner elements. Prior experience showed that the linear management method usually leads to lower social value. In recent decades, widespread and persistent disruptions (e.g., energy shortages, energy price fluctuations, climate change, and environmental pollution, etc.) have consistently demonstrated the failure of single-target optimization of energy systems. With the increase of such failures, we need to seek new solutions to the growing energy security problems beyond traditional approaches. Under this circumstance, researchers have begun to focus on the idea of resilience, an approach related to philosophy and pragmatism. It is supposed that resilience will be used to seek solutions for addressing these problems.
Prior research on energy resilience originates from both ecology resilience and engineering resilience, spanning across general energy systems and some specific systems (O'Brien and Hope, 2010), such as hydropower systems (Afgan and Veziroglu, 2012), electricity systems (Molyneaux et al., 2012; Kharrazi et al., 2015; Ibrahim et al., 2016), and energy infrastructure systems (Ouyang et al., 2012; Alderson et al., 2015). The definitions of energy resilience vary according to different research goals, and these definitions can be generally classified into two categories; the first highlights resilience as the capability to resist disturbances coming from outside and which helps the target system to recover as fast as possible (O'Brien, 2009; Korhonen and Snäkin, 2015; Arghandeh et al., 2016), and the second one emphasizes the core ability of resilience as adaption, including adaption to strikes and the involution of the system itself (O'Brien and Hope, 2010; Skea, 2010).
In terms of research method, a majority of prior research employed a qualitative research method to explore the specific resilience value of target energy systems (DiMase et al., 2015; Hosseini et al., 2016), the key elements that can affect the level of an energy system's resilience (McLellan et al., 2012), and conceptual frameworks that can help to normalize resilience management (Sharifi and Yamagata, 2015; Xie et al., 2018). Little research has investigated energy resilience from the perspective of economic recovery (Rose, 2007; Briguglio et al., 2009). Given that energy security is not only important for economic growth, but also the foundation of national security, it is vital to quantify the relationship between energy resilience and economic recovery. Therefore, to fill the above research gaps, this paper aims to propose a set of models to evaluate energy-economic resilience based on post-disruption events. Further, data from 14 countries will be used to demonstrate the usefulness of our model.
The rest of this paper is organized as follows. The next section reviews the literature on measuring models that have been used to investigate economic recovery and energy resilience. Section Methodology introduces our proposed model to compute resilience and losses to the economy and energy, as well as their inner relationship. Section Case Study presents a case study of 14 countries to illustrate the model's applicability in future. Finally, Section Discussion and Conclusion concludes the paper and makes suggestions for future research.
Over the past few years, the impact of economic resilience on disasters has drawn more and more attention from both researchers and policymakers. At the early stage, the qualitative method was widely used to investigate the necessity to embed resilience into energy systems, the elements that affect energy resilience, and the framework that guides resilience assessment (Sharifi and Yamagata, 2016; Child et al., 2018). However, compared to the qualitative method, it is still too early to use a quantitative method to examine the above topic. Nevertheless, several quantitative methods have been used in prior research and will be introduced below.
One of the quantitative methods is setting up indicators or employing a proxy for resilience; Molyneaux et al. applied resilience to an electricity system by setting up a composite resilience index which consists of seven indicators (Molyneaux et al., 2016). Korhonen et al. used diversity as a proxy of energy system resilience (Korhonen and Snäkin, 2015). Hosseini et al. (2016) identified four domains of resilience: organizational, social, economic, and engineering. Blum et al. set up a resilience index from the angle of economic operation capacity to handle energy-related effects (Blum and Legey, 2012).
The second method is to define resilience through comparisons with historical data; Afgan and Cvetinovic (2013) defined the resilience index R as the integral value of the sustainability index between the time point of a sudden change in respective indicators and the time point when it resumes a stable state value, as follows:
where Wi is the weighting coefficient and qi is an indicator. A similar method is also used in research on natural gas supply resilience, network resilience (Dassisti and Carnimeo, 2013; Rabbani et al., 2015), and some other deterministic models related to energy resilience (Mulyono, 2015; Ibrahim et al., 2016).
Another representative quantitative method used for resilience evaluation is based on the input-output (IO) model. Usually an IO model is combined with integrating linear programming; He et al. developed a resilience model which helps to estimate the maximum level of energy import reduction that an economy can endure (He et al., 2017). Sato evaluated energy resilience by measuring diversity from the perspective of both direct and embodied energy supplies (Sato et al., 2015). Based on the multi-regional IO model, they attempted to use the Shannon-Weaver index and a cosine similarity as a complementary index to evaluate the supply diversity of 134 countries. With the same method, Kharrazi et al. evaluated the electricity diversity in production-based and consumption-based trade networks (Kharrazi et al., 2015).
To sum up, the above three quantitative analysis methods have been widely adopted by researchers. However, the issues of energy consumption and economic recovery from a resilience point of view have not been clarified enough. Models involve economic-energy characteristics only consider economic characteristics, such as economic impacts on disruptions (Rose et al., 2018) and investment optimization (Fang and Sansavini, 2017; Nezamoddini et al., 2017). In this work, we propose the enhancement of energy economic resilience post a disaster event. Particularly, we employ resilience loss to develop a model to address economy recovery with consideration of energy consumption.
Measuring the resilience loss of a target system after a disruptive event is fundamental to assess the relationship between economic recovery and energy consumption. As is shown in Figure 1, the resilience loss is calculated by Equation (2), in which RL is a two-dimension vector represented by the shadow area.
where, RL: Average resilience loss during period T (from t0 to t1)
t0: Start time of disruption
t1: End time of disruption
T: The whole period from time t0 to t1
Et: The expected value based on historical data
Qt: The actual value
Qt0: The actual value from time t0 to t1
Δ: Performance attenuation
λ: Level of recovery.
RL is the average integral value of the difference between Et and Qt. δ represents performance attenuation which indicates the largest deviation of a target system when experiencing disruption during time T. λ describes the recovery level of the system after the disruption, which is relative to its basic performance.
Although the calculation of resilience loss can provide a way to measure the basic resilience state caused by a disruption, it cannot reflect the interrelationship between economic recovery and energy consumption. Given this, we modify the model from Blum and Legey (2012) to measure the resilience loss of energy consumption and electricity consumption. Also, a number of studies have shown that economic growth relies heavily on electricity consumption (Shahbaz et al., 2017; Zhang et al., 2017), so the relative impact of economic recovery on the change of energy as well as electricity consumption during a certain period will be established. From the perspective of energy consumption and economic growth, we define the changing ratios as follows:
(1) If the energy-related factors have no impact on economic recovery, then ε = 0
(2) If the energy-related factors have an impact on economic recovery, then 0 < |ε | <1.
(3) If the energy-related factors affect economic recovery in the same proportion, then |ε| = 1.
(4) If the effects are amplified, then |ε |>1.
Furthermore, taking different energy efficiencies of different countries/regions into account, we take the method of resilience coupling/decoupling to evaluate recovery performance. Decoupling is originated from environmental kuznets curve (EKC), which is applied in economic growth and environmental problems research. In this paper, the term decoupling is applied to economic growth and energy resilience during a post-disruption period. By improving Vehmas' model (Vehmas et al., 2007), six possible scenarios are established in this paper and each scenario is placed in the coordinate axis according to its resilience performance. These scenarios, shown in Figure 2, are: absolute decoupling, relative decoupling, expensive coupling, recessive decoupling, recessive coupling, and strong coupling.
As shown in Figure 2, from the best-case category (I) to the worst-case category (VI), each category is determined by three parameters: ΔRLGDP, RLen, and Δ(RLen/RLGDP). When Δ(RLen/RLGDP) is <0, it is decoupling in the lower area of the diagonal line including “I,” “II,” and “IV;” in these areas the energy efficiency can be improved. When Δ(RLen/RLGDP) is more than 0, it is coupling in the up area of the diagonal line including “III,” “V,” and “VI.” Each area can be further divided into three categories according to the value of ΔRLGDP and RLen. Taking area “I” as an example, the ΔRLGDP increases with a decrease of RLen and Δ(RLen/RLGDP), which is the most ideal state of all six states with the best performance of output and efficiency. The explanation of the six categories are listed in Table 1.
Fourteen countries whose total GDP exceeds 70% of the world's GDP in 2018 were examined, and their final energy consumption and final electricity consumption account for over 65 and 70% of world's total, respectively, in 2018. The Middle East is excluded because of its current situation of unrest. In addition, South Africa and Singapore are included as South Africa is supposed to be a promising economy, and Singapore, as a resource-poor country, represents developed countries that performed well during the 2007–2008 financial crisis. The expected value Et is based on historical data using the method of regression, and fitting degree is supposed to be more than 95%. All the data is from the (IEA) and World Bank.
Fourteen countries whose total GDP exceeded 70% of the world's GDP in 2018 were examined; their final energy consumption and final electricity consumption account for over 65 and 70% of world's total consumption, respectively, in 2018. According to the aforementioned model, the resilience loss for GDP, total final energy consumption, and total final electricity consumption of the 14 countries are shown in Figure 3.
From the results, we can see that the countries were grouped into three categories: almost unaffected (China, India, and Indonesia), almost recovered (South Africa, Korea, Singapore, Brazil, and Germany), and unrecovered (France, the United States, Canada, Japan, the United Kingdom, and Russia). Aligned with the RLen and RLel, it is assumed that the GDP growth rate of China was affected a little bit during the 2007–2008 financial crisis, which exceeded the historical expectation by an average rate of 4% per year. Accordingly, the total energy consumption and total electricity consumption increased by 2.7 and 4.5%, respectively. This means that during the year 2007–2018, China's electricity consumption exceeded the expected value significantly. As to India and Indonesia, their GDP were barely affected. India's total final energy consumption increased slightly, and Indonesia decreased slightly. Both countries had significant increases in total final electricity consumption. Obviously, apart from Russia, the developing countries performed better than the developed countries.
Performance attenuation and recovery level are used to indicate the scale of impacts from the financial crisis. As is shown in Figure 4, the following points were concluded: (1) Countries that suffered more have a relatively poor recovery performance, except for Germany. Although Germany was hard-hit by this financial crisis, it performed much better than other countries at the end of 2018; (2) Developing countries (except Russia) generally performed better than developed countries in terms of both performance attenuation and recovery level.
Germany's astonishing recovery was due to increased trade with developing countries, especially China, which offset its trade reduction with other countries. Further, Germany took a powerful economic stimulus plan focusing on infrastructure investment and tax relief to help small- and medium-sized enterprises. As for Russia, the oil price fluctuation affected RLGDP a lot, which is a reflection of “Dutch disease” and the result of its irrational industry infrastructure.
Results of inter-resilience of economic recovery to energy/electricity consumption are shown in Figure 5. It can be seen that in terms of εen, there are four countries whose figures are not in the reasonable scope (from −1 to 1): China, India, Indonesia, and Russia. For the first three countries, they had a much faster GDP growth than the total final energy consumption. Russia's GDP has sharply reduced by nearly 9% per year while having only about a 1% increase per year in energy consumption. Considering both εenand εel, Russia's capability of handling energy-related problems is clearly insufficient.
According to the results, 14 countries were sorted into the six areas as shown in Figure 6; these 14 countries are spread out over five areas. Strong coupling and recessive coupling are occupied by Russia and Brazil, respectively; Russia shows the worst resilience performance during this period, with an increasingly decaying economic recovery performance yet without a decline in total energy consumption. Considering the fixed assets investment and the sharp decrease of RLel, Russia's overdependence on resources export suffered a lot during this period because of the energy price fluctuation and the shrinking energy demand.
Indonesia lies in the most desirable condition-absolute decoupling. This is mainly because the Indonesian government paid more attention to domestic demand rather than export. They also paid more attention to high-tech industry rather than labor-intensive industry, meaning quality and efficiency of GDP growth were stable. China and India are in the relative decoupling area; the two prominent fast-growing economies benefit from both expanding domestic demand and their multilateral trade strategies. South Africa and all developed countries are in the recessive decoupling state, although South Africa is not the same as other countries because it is a developing country with a relatively low fixed assets investment percentage (around 20% in 2018). But for long-term economic development, a low fixed assets investment may lead to a lack of development motivation.
One of the main findings in our study is that developing countries' economic resilience is better than developed countries when faced with a national crisis, excluding the ones who overly rely on resource extraction. In particular, overreliance on energy/electricity consumption is shown to considerably weaken the ability of the economy to mitigate crisis. Hence, continually decreasing energy reliance will be a promising way to enhance economic resilience.
Another important insight from our study in section Case Study is that boosting domestic demand and strengthening multilateral trading can yield improvements to economic resilience. The governments may be able to exploit this to enhance their economic resilience and energy security, by expanding domestic demand and re-designing the cross-border trade interdependencies.
In this work, we have proposed a novel approach to evaluate the impact of energy consumption on economic recovery from a perspective of resilience. The proposed models are shown to yield problems in the format of decoupling status. The proposed framework can provide valuable decision-support for policy-makers to study the impact of energy consumption on economic recovery, and also guide decision-makers on how to improve the resilience of an economy.
One of the main findings in our case study is that, during the 2007–2008 financial crisis, developing countries were less affected and had better recovery performance than developed countries, except for Russia. Russia is overly dependent on energy export, and its energy efficiency is much lower than most other countries. Furthermore, in terms of developed countries, Korea and Singapore, located in Asia, have better recovery performance than other countries. Germany had experienced an astonishing recovery compared to all the other countries because of its successful trade strategy shift to Asian countries and its efficient tax policy.
For future studies, an alternative multiregional resilience framework can be explored, which classifies the driving factors behind promoting economic recovery based on production-consumption patterns in the energy supply chain. Renewable energy consumption is also playing an increasingly significant role in economic productivity; researchers can quantitatively investigate the role of renewable energy in improving economic recovery, and ultimately improving the resilience of an economy.
Publicly available datasets were analyzed in this study. This data can be found here: https://www.worldbank.org.
ZG takes charge the conceptualization, formal analysis, and the original draft writing of this paper. YW is responsible of data curation, methodology construction, and funding acquisition of this paper. WP is in charge of data calculation, analyzing the results, writing-review and editing, and the validation of this paper. All authors contributed to the article and approved the submitted version.
This research was supported by the Philosophy and Social Science Planning Project of Guangdong Province (Grant No. GD19YGL08), the MOE (Ministry of Education in China) Project of Humanities and Social Sciences (Grant No. 20JYC630026), the National Social Science Fund (Grant No. 18ZDA403), and the National Natural Science Foundation of China (Grant No. 71901080).
The authors declare that the research was conducted in the absence of any commercial or financial relationships that could be construed as a potential conflict of interest.
Afgan, N., and Veziroglu, A. (2012). Sustainable resilience of hydrogen energy system. Int. J. Hydrogen Energy 37, 5461–5467. doi: 10.1016/j.ijhydene.2011.04.201
Afgan, N. H., and Cvetinovic, D. B. (2013). Resilience evaluation of the South East European natural gas routes system catastrophe. Int. J. Eng. Innovative Technol. 2013, 175–180.
Alderson, D. L., Brown, G. G., and Carlyle, W. M. (2015). Operational models of infrastructure resilience. Risk Anal. 35, 562–586. doi: 10.1111/risa.12333
Arghandeh, R., Von Meier, A., Mehrmanesh, L., and Mili, L. (2016). On the definition of cyber-physical resilience in power systems. Renew. Sustain. Energy Rev. 58, 1060–1069. doi: 10.1016/j.rser.2015.12.193
Blum, H., and Legey, L. F. L. (2012). The challenging economics of energy security: ensuring energy benefits in support to sustainable development. Energy Econ. 34, 1982–1989. doi: 10.1016/j.eneco.2012.08.013
Briguglio, L., Cordina, G., Farrugia, N., and Vella, S. (2009). Economic vulnerability and resilience: concepts and measurements. Oxford Dev. Stud. 37, 229–247. doi: 10.1080/13600810903089893
Child, M., Koskinen, O., Linnanen, L., and Breyer, C. (2018). Sustainability guardrails for energy scenarios of the global energy transition. Renew. Sustain. Energy Rev. 91, 321–334. doi: 10.1016/j.rser.2018.03.079
Dassisti, M., and Carnimeo, L. (2013). A small-world methodology of analysis of interchange energy-networks: the European behaviour in the economical crisis. Energy Policy 63, 887–899. doi: 10.1016/j.enpol.2013.09.015
Destek, M. A., and Aslan, A. (2017). Renewable and non-renewable energy consumption and economic growth in emerging economies: evidence from bootstrap panel causality. Renew. Energy 111, 757–763. doi: 10.1016/j.renene.2017.05.008
DiMase, D., Collier, Z. A., Heffner, K., and Linkov, I. (2015). Systems engineering framework for cyber physical security and resilience. Environ. Syst. Decisions 35, 291–300. doi: 10.1007/s10669-015-9540-y
Fang, Y., and Sansavini, G. (2017). Optimizing power system investments and resilience against attacks. Reliab. Eng. Syst. Saf. 159, 161–173. doi: 10.1016/j.ress.2016.10.028
He, P., Ng, T. S., and Su, B. (2017). Energy-economic recovery resilience with input-output linear programming models. Energy Econ. 68, 177–191. doi: 10.1016/j.eneco.2017.10.005
Hosseini, S., Barker, K., and Ramirez-Marquez, J. E. (2016). A review of definitions and measures of system resilience. Reliabil. Eng. Syst. Saf. 145, 47–61. doi: 10.1016/j.ress.2015.08.006
Ibrahim, M., Chen, J., and Kumar, R. (2016). “A resiliency measure for electrical power systems,” in 13th International Workshop on Discrete Event Systems (WODES) (Xi'an: IEEE), 385–390. doi: 10.1109/WODES.2016.749787
IEA (2020). Energy Ealances: Energy Projections for IEA Countries[EB/OL]. Available online at: https://doi.org/10.1787/data-00473-en (accessed october 15, 2018). doi: 10.1787/data-00473-en
Kharrazi, A., Sato, M., Yarime, M., Nakayama, H., Yu, Y., and Kraines, S. (2015). Examining the resilience of national energy systems: measurements of diversity in production-based and consumption-based electricity in the globalization of trade networks. Energy Policy 87, 455–464. doi: 10.1016/j.enpol.2015.09.019
Korhonen, J., and Snäkin, J. (2015). Quantifying the relationship of resilience and eco-efficiency in complex adaptive energy systems. Ecol. Econ. 120, 83–92. doi: 10.1016/j.ecolecon.2015.09.006
Mahadevan, R., and Asafu-Adjaye, J. (2007). Energy consumption, economic growth and prices: a reassessment using panel VECM for developed and developing countries. Energy Policy 35, 2481–2490. doi: 10.1016/j.enpol.2006.08.019
McLellan, B., Zhang, Q., Farzaneh, H., Utama, N. A., and Ishihara, K. N. (2012). Resilience, sustainability and risk management: a focus on energy. Challenges 3, 153–182. doi: 10.3390/challe3020153
Molyneaux, L., Brown, C., Wagner, L., and Foster, J. (2016). Measuring resilience in energy systems: Insights from a range of disciplines. Renew. Sustain. Energy Rev. 59, 1068–1079. doi: 10.1016/j.rser.2016.01.063
Molyneaux, L., Wagner, L., Froome, C., and Foster, J. (2012). Resilience and electricity systems: a comparative analysis. Energy Policy 47, 188–201. doi: 10.1016/j.enpol.2012.04.057
Mulyono, N. B. (2015). Mutual support in energy sector: toward energy resilience. Procedia Comput. Sci. 60, 1041–1050. doi: 10.1016/j.procs.2015.08.149
Nezamoddini, N., Mousavian, S., and Erol-Kantarci, M. (2017). A risk optimization model for enhanced power grid resilience against physical attacks. Electric Power Syst. Res. 143, 329–338. doi: 10.1016/j.epsr.2016.08.046
O'Brien, G. (2009). Vulnerability and resilience in the European energy system. Energy Environ. 20, 399–410. doi: 10.1260/095830509788066457
O'Brien, G., and Hope, A. (2010). Localism and energy: negotiating approaches to embedding resilience in energy systems. Energy Policy 38, 7550–7558. doi: 10.1016/j.enpol.2010.03.033
Ouyang, M., Dueñas-Osorio, L., and Min, X. (2012). A three-stage resilience analysis framework for urban infrastructure systems. Struct. Saf. 36–37, 23–31. doi: 10.1016/j.strusafe.2011.12.004
Rabbani, M., Bahadornia, S. M., and Torabi, S. A. (2015). Designing a resilient oil supply network with an intelligent solution algorithm. Uncertain Supply Chain Manage. 3, 289–310. doi: 10.5267/j.uscm.2015.3.001
Rose, A. (2007). Economic resilience to natural and man-made disasters: multidisciplinary origins and contextual dimensions. Environ. Hazards 7, 383–398. doi: 10.1016/j.envhaz.2007.10.001
Rose, A., Wei, D., and Paul, D. (2018). Economic consequences of and resilience to a disruption of petroleum trade: the role of seaports in US energy security. Energy Policy 115, 584–615. doi: 10.1016/j.enpol.2017.12.052
Sato, M., Kharrazi, A., Nakayama, H., Kraines, S., and Yarime, M. (2015). The resilience of embodied energy networks: a critical dimension for sustainable development goals (SDGs). Glob. Environ. Res. 19, 187–198.
Shahbaz, M., Sarwar, S., Chen, W., and Malik, M. N. (2017). Dynamics of electricity consumption, oil price and economic growth: global perspective. Energy Policy 108, 256–270. doi: 10.1016/j.enpol.2017.06.006
Sharifi, A., and Yamagata, Y. (2015). A conceptual framework for assessment of urban energy resilience. Energy Procedia 75, 2904–2909. doi: 10.1016/j.egypro.2015.07.586
Sharifi, A., and Yamagata, Y. (2016). Principles and criteria for assessing urban energy resilience: a literature review. Renew. Sustain. Energy Rev. 60, 1654–1677. doi: 10.1016/j.rser.2016.03.028
Skea, J. (2010). Valuing diversity in energy supply. Energy Policy 38, 3608–3621. doi: 10.1016/j.enpol.2010.02.038
Tang, C. F., Tan, B. W., and Ozturk, I. (2016). Energy consumption and economic growth in Vietnam. Renew. Sustain. Energy Rev. 54, 1506–1514. doi: 10.1016/j.rser.2015.10.083
Vehmas, J., Luukkanen, J., and Kaivo-oja, J. (2007). Linking analyses and environmental Kuznets curves for aggregated material flows in the EU. J. Clean. Prod. 15, 1662–1673. doi: 10.1016/j.jclepro.2006.08.010
Xie, W., Rose, A., Li, S., He, J., Li, N., and Ali, T. (2018). Dynamic economic resilience and economic recovery from disasters: a quantitative assessment. Risk Anal. 38, 1306–1318. doi: 10.1111/risa.12948
Keywords: energy resilience, economic recovery, post-disruption, inter-resilience, decoupling model
Citation: Wang Y, Gong Z and Pan W (2020) Promoting Economic Recovery From the Perspective of Energy-Economic Resilience: Model Construction and Case Study. Front. Energy Res. 8:212. doi: 10.3389/fenrg.2020.00212
Received: 24 March 2020; Accepted: 05 August 2020;
Published: 23 October 2020.
Edited by:
Xunpeng Shi, University of Technology Sydney, AustraliaReviewed by:
Bing Xue, Institute of Applied Ecology (CAS), ChinaCopyright © 2020 Wang, Gong and Pan. This is an open-access article distributed under the terms of the Creative Commons Attribution License (CC BY). The use, distribution or reproduction in other forums is permitted, provided the original author(s) and the copyright owner(s) are credited and that the original publication in this journal is cited, in accordance with accepted academic practice. No use, distribution or reproduction is permitted which does not comply with these terms.
*Correspondence: Zaijing Gong, Z29uZ3phaWppbmdAMTYzLmNvbQ==
Disclaimer: All claims expressed in this article are solely those of the authors and do not necessarily represent those of their affiliated organizations, or those of the publisher, the editors and the reviewers. Any product that may be evaluated in this article or claim that may be made by its manufacturer is not guaranteed or endorsed by the publisher.
Research integrity at Frontiers
Learn more about the work of our research integrity team to safeguard the quality of each article we publish.