- Center for Management Studies of IST (CEG-IST), Instituto Superior Técnico, Lisbon University, Lisbon, Portugal
Commonly process industries supply chains (SCs) deal with chemical process based networks involving suppliers, producers, warehouses, retailers, and distributors. More recently, reverse logistics activities started to be also considered as part of such networks, being these responsible for incorporating into the forward chains non-conforming/end-of-life products through recovering processes. This supports the exploration of the waste-to-value concept within the SCs while addressing important environmental issues. The result of such behavior has increased the already complex management of such systems challenging the development of decision supporting tools that can help the related decision process. In this paper, we address this need and analyze the more relevant published works that contribute to this goal while identifying the classes of problems that have been solved and which are the new trends in the area. We finalize this article by drawing some conclusions where we discuss advances performed and recognized challenges for the future.
Introduction
Aggressive global competition has been forcing companies to become flexible, responsiveness, and efficient while guaranteeing costumers’ demands at minimum costs. In such context, supply chains (SCs) appear with a thrilling importance as they are the organizations systems responsible for delivering the products in the right location with the right quantity and at the right time. The management of such systems is, however, complex and such complexity increases with the number of entities, materials, and information involved. Conflictive objectives are often present and solutions trade-offs need to be found. The management of SCs – supply chain management (SCM) – was originally introduced by consultants in the early 1980s and in the 1990s an increasing number of academic and industry articles started to be published (Lambert and Cooper, 2000).
In this context and within the process industry, process SCs apart from facing the above identified challenges they additionally have to account for the intrinsic factors and technological characteristics of the process industries, which as stated by Shah (2005) are driven by a set of defies that are still actual:
• production of shorter product life-cycles so as to answer to the current market dynamics;
• desire to move from the product/process-oriented business to a service business, so as to add value to the final products and increasing costumers loyalty
• mass customization – produce “specialty” products at a minimum cost
• incorporate sustainability concerns in the day to day life, dealing adequately with environmental and social pressures while maintain the system economically profitable.
Such challenges imply an efficient SC integration needful a system wide approach where the different chain entities, aiming at a maximum service level, should form consistent systems where information and risks sharing should be pursued under an uncertain local and global setting. This is, however, a difficult to attain and the need of decision supporting tools that can help the different activities across SCs is then mandatory. Such activities are diverse and span across three main decision levels (see Figure 1).
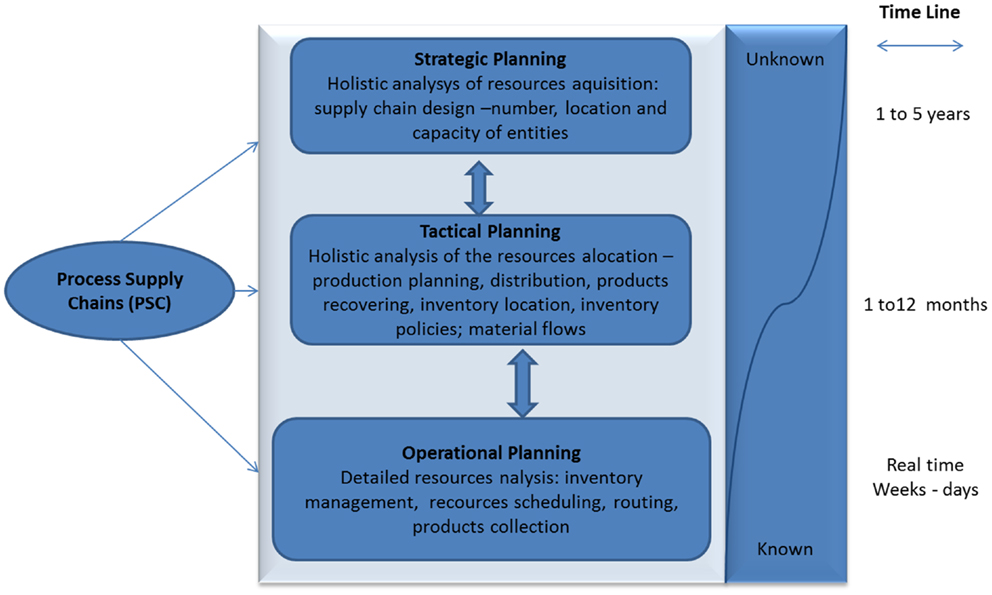
Figure 1. Supply chain decision levels (adapted from Shapiro, 2001).
• strategic planning – which deals with decisions that have a long-lasting effect, such as the network design – number, location, and capacity of the entities;
• tactical planning – includes resources planning, inventory policies definition, transportation strategies, material flows;
• operational planning – contemplate the day to day decisions where scheduling, routing, truck loading, and human resources allocation are to be defined.
The process systems engineering (PSE) has been playing an important role when addressing the above type of decisions within the process SCs. As stated by Stephanopoulos and Reklaitis (2012), on their very interesting paper on the PSE contributions, PSE has been addressing important process SC domains such as the location, capacity changes, flow magnitudes, allocation, inventories, and external links as well as more recently environmental, financial, product development, uncertainties, and marketing issues. On these issues, the readers are referred to recent articles that have been taken as basis to the present article and can be seen as complementary (see Table 1).
In the next sections, the relevant research on SCs addressing the above decision levels is reviewed and the role of PSE is analyzed. First, an overview of the recent progress achieved on the design and planning of SCs is made starting by analyzing the deterministic problems, followed by the treatment of stochastic problems and risk and sustainability topics. Subsequently, tactical and operational problems are assessed and the solution of real process SCs is emphasized. This article finalizes by identifying and discussing the main SCs challenges that will gush in coming years. A research agenda is defined aiming to create important trails to be pursued by the academic community working in the area in the coming years.
Supply Chain Network Design and Planning
The network design problem determines the physical configuration and infrastructure of the SCs. It includes decisions on the number, locations, and size of manufacturing plants, warehouses, and distribution centers and establishes the allocation of production and warehousing to retailers and costumers (see Figure 2). The typical planning horizon is of few years and the associated decisions are of last-long effects (see Figure 1). The main goal is to find the right balance between sourcing, production, inventory, and transportation costs so as to guarantee the defined service level leading to a maximum profit. Decisions taken at this level are not often revaluated and only occur when changes in the sourcing strategies, cost of running production plants, demand patterns, product mix, or production processes arises. If so, a retrofit problem is to be solved and the existent network is redesigned.
Typical network design problems involve large amounts of data including amongst others, locations of costumers, existing facilities, and suppliers; products to be produced and delivered, annual demands patterns by product and costumer; transportation modes; production processes and associated recipes; services levels and diverse involved costs: sourcing, production, warehousing, transportation, order processing, collecting, and reprocessing. The usage of such large amount of data implies the development of aggregation techniques prior to the use of models to solve the problem. Often such techniques are based on clustering techniques where aggregation by costumers and/or products is commonly explored. Costumers are aggregated within a geographical area (e.g., zip-code) and are often classified by their service level or frequency of delivery. Products aggregation can be made by distribution patterns or product type, exploring the stock keeping units (SKUs) concept. Although necessary, the aggregation techniques must be used in an adequate form as they have an important impact on the models effectiveness.
Once the data are collected and treated the next step within the network design problem is to optimize the logistics network. Several techniques can be used for this purpose as optimization, heuristics, or simulation, the latter being employed to evaluated predefined design alternatives. On this article, we focus on optimization techniques, as the treatment of all the other approaches will lead to a too long document.
Looking at the network design problem, it has been recognized that the inclusion of planning decisions appears as an important add on, as it creates value on the final solutions (see Figure 2). Designing the system while accounting for the usage of its main resources allows a more detailed description of the global system and results in more implementable and profitable solutions. It permits the definition of the inventory positioning and the planning of resources usage leading to the definition of appropriate SC decisions across the different SCs echelons. Through network planning, which combines network design, inventory position, and resource allocation, the companies can more efficiently and globally optimize the SCs performance matching adequately the supply and the demand.
To support such challenge, the use of optimization techniques has been quite explored. Optimization models may be of steady state or dynamic type and could deal with deterministic or stochastic problems. On the deterministic problems, the first problems addressed considered simple cases where only location–allocation situations were solved and where mainly distribution networks were considered. Such problem has, however, later on being extended and production–distribution problems started to be addressed. In 1997, Vidal and Goetschalckx (1997) on their SCs review concluded that the works published till then addressed quite simple problems and real cases have not being addressed. Issues as the treatment of international aspects, namely the modeling of different taxations on products; the inclusion of production processes in the networks, namely their capacity; the presence of uncertainty and the presence of diverse entities in the SC networks stood to be studied. Later on, Shah (2005) recognized that from 1997 up to 2005 an advance on disabling such limitations was accomplished. More complex networks have been addressed and the presence of uncertainties was underway. However, the author also stated that in the PSE community process capacity planning was commonly addressed independently of the network design problem. This was justified by the fact that in process industries, the production costs tend to be dominated and so the decoupling between production and distribution could be a satisfactory option due to the existent solution difficulties faced when solving complex problems. This fact has however changed over the years and as detailed by Papageorgiou (2009) and more recently by Barbosa-Póvoa (2012) SCs design and planning problems when solved simultaneously result in higher benefits. Additionally, the solution of both problems treated independently could lead to infeasible implementation solutions or even more costly solutions.
One pioneer work addressing simultaneously the design and planning of SCs was presented by Wilkinson et al. (1996). The authors using the resource-task network (RTN) representation developed a generic model where complex networks were studied. An aggregate model for the detailed problem was developed and the optimal design and planning of a real production and distribution system with 3 factories and 14 market warehouses with over a 100 products was solved. From that time onward, several works on the process SCs addressed the presence of production and distribution for simultaneous activities. This is the case of the works presented by Kallrath (2002), Tsiakis and Papageorgiou (2008), Jackson and Grossmann (2003), Naraharisetti et al. (2008), You and Grossmann (2008), Kallrath (2008), Lainez et al. (2009), and Naraharisetti and Karimi (2010). Different issues have been addressed such as facility location and relocation, production and distribution design and planning, supply contracts, and capital generation as well as investment and disinvestment.
More recently Liu et al. (2013) studied the production, distribution, and capacity planning of global SCs considering cost, responsiveness, and customer service level simultaneously. A multi-objective model was developed considering total cost, total flow time, and total lost sales as key objectives. The inclusion of activities details into the SC design and planning models has been also received recently some attention. Corsano et al. (2014), designed SCs with explicit consideration of the detailed plant performance of the embedded facilities, batch processes were incorporated. Coccola et al. (2013), considered the integration of production and distribution aspects into a multi-echelon SC where single stage parallel lines of multi-product batch plants together with multi-echelon, multi-product distribution networks were modeled. The model developed is able to solve complex problems providing detailed information on the scheduling coordination for the different SCs activities.
From the above works, it can be said that SCs characteristics and complexities have been fairly explored. However, these facts have increased the complexity of the related problems and consequently the difficulty of reaching a solution in reasonable computational time has increased accordingly. The need for more efficient solution approaches was identified. Such concern has been addressed in two quite recent articles (Shah and Ierapetritou, 2012; Copado-Mendez et al., 2013). Shah and Ierapetritou (2012) considered the problem of solving the complex problems of SCs and effectively deal with the associated increasing complexity by exploring the block angular structure of the constraints matrix. This was achieved by relaxing the inventory constraints between adjoining time periods using the augmented Lagrangian decomposition method. Copado-Mendez et al. (2013) proposed a novel solution method that combines the strengths of standard branch and cut techniques with the efficiency of large neighborhood search (LNS). The application of the developed approach is shown through the solution of two case-studies on energy applications: the design of SCs for bioethanol production and the strategic planning of hydrogen infrastructures for vehicle use.
The above analyzed works described essentially the study of deterministic problems nevertheless several authors have been also studied how to deal with uncertainty when designing and planning SCs. This aspect will be explored in the next section.
Design and Planning with Uncertainty and Risk
As represented in Figure 1, strategic and planning decisions involve a high level of uncertainty. Thus it has been for long recognized that accounting for uncertainty in the surrounding environment and associated data is important (Vidal and Goetschalckx (1997); Shah, 2005; Papageorgiou, 2009; Barbosa-Póvoa, 2012). This is even more important when knowing that today’s SCs act globally and consequently are more exposed to uncertainty and risks.
The scenario approach has dominated the works developed by the process system community, when addressing uncertainty. This ranges from a three scenario problem as in Liu and Sahinidis (1996) and Tsiakis et al. (2001) to multi-scenario approaches as in Guillen et al. (2006). Liu and Sahinidis (1996) studied the presence of uncertainty when dealing with long-range planning of process networks. Uncertainty in demands and prices was accounted for using a scenario approach. Applequist et al. (2000) introduced a risk premium as a measure of the return and risk of an investment relative to alternative financial investments. The right balance between expected value of investment performance and associated variance was obtained. Gupta and Maranas (2003) addressed the problem with demand uncertainty and explored the use of real options to model demand uncertainty. Also, Barbaro and Bagajewicz (2004) considered the presence of multi-objectives under an uncertain demand where objectives as profit, customer satisfaction, and financial risk were modeled. More recently, You et al. (2009) studied the mid-term planning of multi-product process industries SCs and explored risk management under demand and freight rates uncertainty. Risk measures were incorporated into a stochastic programing model allowing the establishment of trade-offs between cost and risk. Georgiadis et al. (2011) studied the design and planning of SCs and captured uncertainty in terms of a number of likely scenarios possible to materialize during the lifetime of the network to be designed. Klibi and Martel (2012), proposed a risk modeling approach for evaluating and designing SCs operating under uncertainty. Their approach provides the definition of robust and resilient networks that when used in conjunction with stochastic optimization approaches allows the resolution of complex problems leading to resilient SCs with superior mitigation risk capacities. Longinidis and Georgiadis (2013), integrated financial performance and credit solvency modeling within the design of SCs under economic uncertainty. A multi-objective mixed-integer non-linear programing (moMINLP) model was developed that measures financial performance through economic value added and credit solvency through a valid credit scoring model.
Two comprehensive reviews have been published dealing with the treatment of uncertainty and risk in SCs (Peidro et al., 2009; Klibi and Martel, 2012). The need to further explore quantitative models that integrate different sources of uncertainty as well as new methods to model and solve uncertainty problems and the solution of real problems was recognized as future research areas.
Summarizing although some authors have dealt with uncertainty when designing and planning SCs and the stochastic approach could be classified as well-established, the application of such approaches to different uncertainty parameters is still to be done. The main treated uncertain parameter has been a demand and few works address other types of uncertainty in SCs. Furthermore, the risk modeling still remains as an area that requires further research attention. Risks can range from unknown–unknown to known–unknown and the first type of risks has been hardly addressed. Additionally, these aspects linked to the need of building resilient SCs has not yet been thoroughly analyzed by the academic community. Diverse strategies have been implemented by companies to deal with such events occurrence but deeper studies on how to measure SCs resilience are still to be studied so as to help managers to design and plan their SCs (see Table 2).
Designing and Planning Sustainable Conscious Supply Chains
The concept of sustainable SCs has recently gained a pronounced importance as companies are facing the challenge of dealing with sustainable issues caused by increased society awareness toward environmental and societal problems. As stated in Barbosa-Póvoa (2012), sustainable SCs can be described as operational structures that manage the raw materials and services from suppliers to costumers and back accounting for the explicit inclusion of social and environmental impacts. Within the process SCs, few works have been reported addressing such issues but the necessity to seriously explore them exists. On the one hand, because non-conform and/or end-of-life products are present in any chain and additionally process SCs are very sensitive when environmental and social concerns are at stake namely due to pollution and safety reasons (Papageorgiou, 2009; Barbosa-Póvoa, 2012; Nikolopoulou and Ierapetritou, 2012). As mentioned in Barbosa-Póvoa (2012), there are a number of forms to address sustainability into the SCs. More specifically, sustainability issues have been addressed by considering the design and planning of closed-loop SCs (Guide and Van Wassenhowe, 2002; Salema et al., 2010); by explicit model environmental issues into the SCs leading to the so-called green SCs (Srivastava, 2007) and more recently by adding the contribution of the social aspects (Carvalho and Barbosa-Póvoa, 2011).
Hugo and Pistikopoulos (2005) were one of the first to consider environmental concerns into the SCs design and planning. A mathematical formulation was developed, which was applied to a bulk chemicals SC. Duque et al. (2007), using the maximal state-task network (STN) proposed an optimization model for the design and operation of a recovery route for residual industrial products with uncertain demands. This work was extended in Duque et al. (2010) where the Eco-indicator 99 was used to quantify the environmental impacts of the global SC. Matos and Hall (2007) analyzed the integration of sustainable development concepts into the SCs and proposed a framework that guides the utilization of LCA methods into the SCs. Two case-studies were studied, oil sand refining and agriculture biotechnology. Bojarski et al. (2009) incorporated environmental impacts and regulations into the design and planning of SCs. SC activities impacts were calculated and the financial efforts that should be performed to reduce the existent environmental impacts were calculated. A carbon trade-scheme and a temporal distribution of environmental interventions were included. Guillen-Gosalbez and Grossmann (2009, 2010) studied the optimal design and planning of sustainable chemical SCs in the presence of uncertainty. The environmental impacts were calculated through the Eco-indicator 99. A bi-criterion stochastic non-convex mixed-integer non-linear program (MINLP) was developed where trade-offs between profit and environmental impacts were established. Pinto-Varela et al. (2011) also addressed the design and planning of SCs considering profit and environmental impacts calculation. A multi-objective model was developed and again possible trade-offs between both objectives considered could be achieved.
More recently, Zeballos et al. (2012) proposed a detailed model for a generic closed-loop SC where uncertainty in the quality and quantity of the return products was considered. The approach uses scenarios and a two-stage stochastic model was developed. Cardoso et al. (2013), also addressed closed-loop SCs under uncertainty. Activities as production, assembling, or disassembling are characterized in detail while accounting for the SC dynamics under an uncertain market environment. Finally, Zeballos et al. (2014) developed a multi-period, multi-product closed-loop SC design and planning problem where uncertain levels in the amount of raw materials and customer demands were studied. Several aspects of practical significance were considered, such as operational and environmental costs of different transportation modes, as well as capacity limits on production, distribution, and storage.
From the above works, it can be seen that there has been a clear attempt to addressed sustainability issues into the supply design and planning (see Table 2). However, there is still an open research problem where further details on the environmental and social issues need to be explored. The systems boundaries need to be clearly defined and the most important environmental and social components should be identified so as to accurately add them into the SCs modeling and optimization.
Table 2, presents in a summarized form the contribution of the above analyzed articles. As previously mentioned, several issues characterizing process SCs still need further study. These are related to the treatment of uncertainty, risk and resilience, sustainability (in particular social aspects); international issues; SC activities details and adequate solution methodologies.
Supply Chain Tactical and Operational Aspects
As represented in Figure 3 the SC planning can be started at a more strategic level spreading into operational levels where different decisions are to be taken. First, an upper level is important to match the supply and the demand. The development of aggregated plans is performed, which then are decomposed into more detailed plans at the lower levels. These are the master production and distribution plans – defined within periods of 3–1 months, where production and distribution activities are planned in more detail. Such master plans are then further decomposed and result into the operational plans, defined weekly or even daily, that help the definition of the day to day SC activities (e.g., resources; production; and distribution scheduling).
In this context and as mentioned previously, the SCs design is often coupled with the tactical decision level, which is here considered at the upper level. On the other hand, decisions at this tactical level should if possible be integrated with the operational levels. Such integration is not easy to perform but some works have tried to address such issue. Some of these relevant works are going to be next analyzed in a summarized form.
At the planning level, the readers are referred to the article by Shapiro (2004) where the author points out the importance of optimization planning decisions within the SCs. Some papers addressing these issues that have been published till 2004 are reviewed. Later on, Ryu and Pistikopoulos (2007) explored the SC planning and identified the distribution of multiple demands to multiple entities as a key issue. This is often a real problem faced by companies where the use of optimization tools is seen with a great value for supporting the final decision. Verderame and Floudas (2009) on the other hand considered the multi-site planning with production disaggregation where both production and shipment profiles along the chain were calculated. Amaro and Barbosa-Póvoa (2011) explored the different modeling aspects that should be accounted when dealing with the SC planning. Both forward and reverse flows are considered simultaneously. A discrete versus a continuous approach was compared concluding that the later one would allow the modeling of problem details at the expenses of higher computational times. Zamarripa et al. (2012) and Amaro and Barbosa-Póvoa (2008) extended the SC planning problem and studied cooperative and/or competitive scenarios.
When extending, the planning deterministic problem to deal with uncertainty different contributions appeared. In 2000, Gupta et al. (2000) considered a mid-term, multi-site SC planning under demand uncertainty to safeguard against inventory depletion at the production sites and excessive shortage at the customer demand. In 2003, Gupta and Maranas (2003) developed a bilevel decision-making framework where production decisions are made “here-and-now” prior to the resolution of uncertainty, while the SC decisions are postponed in a “wait-and-see” mode. Later on, Amaro and Barbosa-Póvoa (2009) studied uncertainty in demands and on products prices where partnerships collaboration was explored so as to deal with such uncertainties. Grossmann and co-workers have also addressed the SC planning under uncertainty (You et al., 2009, 2011a,b; You and Grossmann, 2013). Risk management, inventory management, and solution methods were respectively explored.
Additionally, the integration between tactical and operational levels has been also studied but in small number. Important works are identified namely the ones proposed by Guillen et al. (2006), Amaro and Barbosa-Póvoa (2008), and Shah and Ierapetritou (2012). Such works have shown that integrating different decision levels leads to better results that are translated into the maximization of SCs earnings. Shah and Ierapetritou (2012) further explored an efficient way to solve the resultant complex problem when integrating different decision levels.
Finally, addressing SC activities at the operational level results in important add-ons that enhance SC performance (Papageorgiou, 2009). Huang and Karimi (2006) focus on the modeling of transshipment operations of liquid chemical cargos and propose MILP models based on continuous-time representations. Al-Ameri et al. (2008) propose a rolling horizon approach for the ship-scheduling problem by combining aggregate and detailed MILP models. Amaro and Barbosa-Póvoa (2008) integrated operational decisions and transportation policies. Dondo et al. (2009) consider the operational level of multiple vehicle pickup and delivery problems with time windows commonly arising in multi-site systems. Dondo et al. (2011) studied the distribution problem considering vehicle routing and X-docking centers. Boschetto et al. (2012) considered the planning and scheduling of a set of complex distribution networks and have shown the difficulty involved when planning and scheduling levels are to be integrated. The advantage of such integration was also demonstrated.
Table 3 summarizes the contribution of the above analyzed articles in the area of SCs tactical and operational decision levels. From this, table is clear to see that works addressing multi-objective aspects as well as uncertainty, risk and resilience, sustainability, and international issues at the tactical and operational levels are scarce.
Process Supply Chains – Real Cases Solution
The solution of real SC cases within the process industries has been reported in the literature being the majority of the cases very interesting. In this section, some of these cases are analyzed, which are classified within four main areas: oil; pharmaceutical; gas/hydrogen; biomass/biofuel; agricultural products.
On the oil SCs, an important work was early presented by Neiro and Pinto (2004). An integrated mathematical framework was developed for the petroleum SC planning where a set of refineries, terminals, and pipeline networks was considered. The associated SC activities were modeled in detail. The model was applied to the solution of the Brazilian oil SC. More recently, Carneiro et al. (2010) also analyzed the strategic planning of part of the Brazilian oil SC, comprising six oil refineries. A two-stage stochastic model was developed with fixed recourse and incorporation of risk management. The conditional value-at-risk (CVaR) was adopted. From the same group Oliveira and Hamacher (2012) studied the problem of optimizing the investment planning process of a logistics infrastructure for the distribution of petroleum products. Uncertainty in demand was considered as well as specific operational issues as navigability of transportation systems, operational capacity of rotating tanks, and demurrage costs, among others. The methodology developed allowed to obtain solutions that are relatively close to the real optimal solution of the problem. Again the case-study focused on the Brazil SC but this time considering the distribution of petroleum products in Northern Brazil. Fernandes et al. (2014) also treated the oil SC but studied the downstream part where the distribution of products in Portugal was analyzed. The design and retrofit problems were studied and a detailed cost structure developed. The authors concluded that the actual distribution SC is not optimized and should be subject to a series of retrofit operations. Uni-entity networks and multi-entity networks with collaborative and/or individualistic operation were modeled. As main conclusion, the authors stated that a collaborative perspective could result in higher profits.
Hydrogen SCs were addressed by van den Heever and Grossmann (2003). These authors studied the optimization of a hydrogen supply network where the integration of production planning and reactive scheduling was modeled. A real SC formed by 5 plants, 4 inter-connected pipelines, and 20 customers was studied. Also, Almansoori and Shah (2006) addressed the design of hydrogen SCs where an UK base-case was solved. On the gas SCs, three works were identified: Hamedi et al. (2009) and You et al. (2011a,b) that studied the distribution planning problem. In the later apart from distribution problem also inventory planning was considered under an uncertain environment.
Bioenergy SCs were studied by Dunnett et al. (2007). The STN representation was used and a detailed modeling of specific activities as harvesting, drying, storage, and transportations were considered. Eksioglua et al. (2009) studied the logistical challenges with supplying biomass to a biorefinery. The model determines the number, size, and location of biorefineries needed to produce biofuel using the available biomass. The amount of biomass shipped, processed, and inventoried during a time period was calculated. A real case of the state of Mississippi in USA was solved identifying potential location for biorefineries, and giving insights on the factors that impact the delivery cost of c-ethanol. Kostin et al. (2011, 2012), addressed the optimal design and planning of a sugar SC in Argentina to produce ethanol and sugar. Production and storage capacity expansions associated with planning decisions are considered under several financial risk mitigation options. You and Wang (2011) addressed the optimal design and planning of biomass-to-liquids (BTL) SCs under economic and environmental criteria. A county-level case-study for the Iowa state in USA was solved. Finally, Tong et al. (2014a) presented an interesting study exploring the integration of a hydrocarbon biofuel SC with existing petroleum refineries. A multi-period, mixed-integer linear programing model is developed that provided the diverse conversion pathways, the technology to use, and the insertion point selections. Biomass seasonality, geographical diversity, biomass degradation, demand distribution, and government incentives were explored. This model simultaneously optimized the SC design, insertion point selection, and production planning. Pricing and quantity uncertainties were also considered by Tong et al. (2014b).
On the pharmaceutical SCs, several works have been appearing. Sousa et al. (2011) addressed the design and planning problems. Dynamic allocation/planning problems were developed, where different stages from production at primary and secondary sites to product distribution to markets were contemplated. Amaro and Barbosa-Póvoa (2008, 2009, 2011) looked into the planning and scheduling problems in pharmaceutical SCs. The introduction of reverse flows with recovering facilities, the presence of uncertainty, and the integration of planning and scheduling decisions were considered. A Portuguese SC with international operations and markets was studied in detail.
Finally, Bassett and Gardner (2010) considered the global SC at Dow AgroSciences and addressed the simultaneous optimization of the production and distribution. Later on, Bassett and Gardner (2013) explored the same agricultural chemicals SC and considered the tracing of products to their source for inventory valuation, taxation, and duty computation. The design of the underlying SC network was defined.
Table 4 summarizes the above analyzed works where the focus was on the solution of real case-studies. It can be seen that interesting cases have been studied but further areas of the process industries are still to be addressed. Furthermore, the works mainly address strategic and planning levels and few works have dealt with operational issues. Additionally, within the cases analyzed important aspects often present in real life as uncertainty and risk, multi-objective trade-offs, and sustainability issues have been insufficiently studied.
Recent Supply Chain Tendencies and a Future Research Agenda
This article aimed to provide an overview of the area of processes SCs. This has been driven by the requirements of the process industries for reducing costs, while satisfying clients’ needs, so as to remain competitive in a global marketplace. Some of the major addressed challenges from the strategic to the operational decision levels have been analyzed and real cases solutions were identified showing the importance of the problems addressed.
A common modeling/solution methodology was explored along this article, which focused on the development of optimization models. The significant research potential of such methodology on the solution of process SCs was shown proved by the number of models analyzed and their applicability to real case networks and operations. In this context, it is important to remark that a distinguishing feature of process industry SCs is that SC performance is very strongly affected by the flexibility and responsiveness of the production process (Shah, 2005). Consequently, the improved design and planning of existing processes as well as the effective design and planning of “new” SCs is to be pursued. Though numerous works have been reported in the area, further research needs to be performed, which should target methodologies to coordinate decision models and/or building holistic cross-functional models within SCs.
The PSE community has been playing an important role in such setting but still has much to do.
The PSE community has played and still has an important role to play in such setting. Exploring links to other research areas as Operations Research should be explored so as to build research foundations in addressing large-scale enterprise-wide modeling, optimization, and solution of complex systems as SCs (Stephanopoulos and Reklaitis, 2012). A number of novel type of SCs have been emerging and further ones will appear (Barbosa-Póvoa, 2012). The coordinated analysis of such systems is an open issue with vital defies. Shah (2005) in his review paper identified as examples of future process SCs diverse types of networks:
• hydrogen, and more generally, SCs to support fuel cells;
• water – to guarantee world needs of quality water;
• pharmaceutical SCs, that should support fast response therapeutics;
• energy – to guarantee world needs;
• life science products;
• biomass and biofuel;
• gas-to-value;
• waste-to-value and reverse production systems.
These systems are still valid and although some of them have already being gaining some attention by the academic and industrial communities, much is still to be done. Additionally, the well-known existing SCs still present a number of challenges that have to be trailed. In particular, the following topics are to be pursued establishing a future research agenda within process SCs:
– Detail SCs’ important characteristics – being SCs global networks, there are central aspects that should be considered. Amongst this are the modeling of: international taxes; transfer prices, duties as well as sharing of products amongst facilities in different locations allowing transformation along the network. The importance of considering process SCs traceability and perishability appear as also an important aspect to be explored so as to guarantee final product quality. Moreover, the additional complexity dimension created by the need of considering within process SCs the process synthesis and the design of production systems are aspects that need to be further researched. The models should then be more detailed.
– Individualist versus collaborative perspectives – the need to integrate coordination amongst SCs entities has been recognized for long. SCs involve inter-organizational levels but in the existent literature few studies address such issues. There is a need to develop models and implement strategies where all actors of the SC can act globally rather than locally. New incentive schemes, merit-based benefits, and new performance measures should be identified so as to enhance such integration.
– Addressing uncertainty. This is an area that has been increasingly gaining importance in the SC literature. The dynamics of the global environment mostly caused by market changing conditions need to be accounted in the models. Aspects related to price fluctuations; demands changes; suppliers availability; available transportation modes and amounts; production rates; amount and quality of returned products, are often subject to uncertainties and should be reflected in coherence.
– Risk management and resilience – developing strategies for managing SC risks and build SC resilience is at stake. One may consider using adequate risk measures and identify suitable performance targets for SC resilience. The analysis of the SCs dynamics should be dogged and new indicators characterizing such dynamics should be established.
– Include sustainability concerns by addressing environmental as well as socio-economical dimensions – these calls for new forms of looking into the problems, identifying appropriate forms to measure such dimensions while following a system’s thinking approach and a holistic treatment of such problems, implying a long-term engagement. Multi-objective approaches are required and the establishment of trade-offs should be explored. Sustainable SCs should help companies to become agile, adaptive, and aligned in balancing the people and the planet with profits.
– New performance measures – new objective functions rather than ordinary measures such as cost minimization and profit maximization should be explored. This clearly follows the two last topics where risk, resilience, and sustainability are to be incorporated into the SCs modeling. Hence, the development of multi-criteria and multi-objective problems, where conflicting objectives may be present, is required. More financial, non-financial, and decision-making level performance measures need to be accounted as well as tangibles that identify success, as service levels. Such measures ought to allow the identification of bottlenecks and waste, pursuing improvement and simplification
– Efficient solution methods – the consideration of the above identified research challenges will result in highly complex problems and thus an investment on efficient solution methods is vital. The process system engineering can have a particular important role on this challenge. Different type of solution methods such as rolling horizon; decomposition methods; heuristics-optimization models (math heuristics) amongst others have to be explored. The link of the PSC community to other research areas as operations research could help the development of such research line.
Apart from the above topics that are mainly focused on novel methods and tools, challenging emerging fields of application – as the ones above identified above midst others – should also be explored by considering their specific characteristics. Reaching out to novel types of SCs will avoid us to get trapped into marginal improvements of existing methods and tools. Furthermore and aiming to contribute to closing the gap between research and industry, the solution of real cases should continue to be followed where consistent co-operations between academia and industry should be fostered aiming to demonstrate the value of the methods developed in industrial settings.
To finalize, it is important to mention that the above covered topics represent the background and experience of the author and should not be considered an exhaustive set but just the identification of some important lines of research. A point to be raised is that some of the above challenges imply a long-term engagement and thus academics should follow such challenges without aiming at short-term successes while industrialists have to be patient and open-minded toward long-term research efforts that will originate from addressing such challenges. Looking ahead we believe that SCM and in particular process SCs will continue to be a fertile research area, supported by innovative process systems models, methodologies, and tools.
Conflict of Interest Statement
The author declares that the research was conducted in the absence of any commercial or financial relationships that could be construed as a potential conflict of interest.
Acknowledgments
The authors gratefully acknowledge financial support from Fundação para a Ciência e Tecnologia (FCT), project EXPL/EMS-GIN/1930/2013.
References
Al-Ameri, T. A., Shah, N., and Papageorgiou, L. G. (2008). Optimization of vendor managed inventory systems in a rolling horizon framework. Comput. Ind. Eng. 54, 1019–1047. doi:10.1016/j.cie.2007.12.003
Almansoori, A., and Shah, N. (2006). Design and operation of a future hydrogen supply chain – snapshot model. Chem. Eng. Res. Des. 84, 423–438. doi:10.1205/cherd.05193
Amaro, A. C., and Barbosa-Póvoa, A. P. (2009). The effect of uncertainty on the optimal closed-loop supply chain planning under different partnerships structure. Comput. Chem. Eng. 33, 2144–2158. doi:10.1016/j.compchemeng.2009.06.003
Amaro, A. C., and Barbosa-Póvoa, A. P. (2011). Supply chains planning with reverse flows: optimal alternative time formulations. Ind. Eng. Chem. Res. 50, 5005–5022. doi:10.1021/ie101416q
Amaro, A. C. S., and Barbosa-Póvoa, A. P. (2008). Planning and scheduling of industrial supply chains with reverse flows: a real pharmaceutical case study. Comput. Chem. Eng. 32, 2606–2625. doi:10.1016/j.compchemeng.2008.03.006
Applequist, G. E., Pekny, J. F., and Reklaitis, G. V. (2000). Risk and uncertainty in managing chemical manufacturing supply chains. Comput. Chem. Eng. 24, 2211–2222. doi:10.1016/S0098-1354(00)00585-8
Barbaro, A., and Bagajewicz, M. J. (2004). Managing financial risk in planning under uncertainty. AIChE J. 50, 963–989. doi:10.1002/aic.10095
Barbosa-Póvoa, A. P. (2012). Progresses and challenges in process industry supply chains optimization. Curr. Opin. Chem. Eng. 1, 446–452. doi:10.1016/j.coche.2012.09.006
Bassett, M., and Gardner, L. (2013). Designing optimal global supply chains at Dow AgroSciences. Ann. Oper. Res. 203, 187–216. doi:10.1007/s10479-010-0802-2
Bassett, M., and Gardner, L. (2010). Optimizing the design of global supply chains at Dow AgroSciences. Comput. Chem. Eng. 34, 254–265. doi:10.1016/j.compchemeng.2009.08.002
Bojarski, A. D., Laínez, J. M., Espuña, A., and Puigjaner, L. (2009). Incorporating environmental impacts and regulations in a holistic supply chains modelling: an LCA approach. Comput. Chem. Eng. 2009, 1747–1759. doi:10.1016/j.compchemeng.2009.04.009
Boschetto, S. N., Magatao, L., Polli, H. L., Flávio Neves, F. Jr., Arruda, L., Relvas, S., et al. (2012). Planning and sequencing product distribution in a real-world pipeline network: an MILP decomposition approach. Ind. Eng. Chem. Res. 51, 4591–4609. doi:10.1021/ie2010252
Cardoso, S., Barbosa-Póvoa, A., and Relvas, S. (2013). Design and planning of supply chains with integration of reverse logistics activities under demand uncertainty. Eur. J. Oper. Res. 226, 436–451. doi:10.1016/j.ejor.2012.11.035
Carneiro, M. C., Ribas, G. P., and Hamacher, S. (2010). Risk management in the oil supply chain: a CVaR approach. Ind. Eng. Chem. Res. 49, 3286–3294. doi:10.1021/ie901265n
Carvalho, A., and Barbosa-Póvoa, A. P. (2011). “Social indicators for supply chain analysis,” in EuROma – European Operation Management Conference, 2011 (Cambridge).
Coccola, M. E., Zamarripa, M., Mendez, C. A., and Espuña, A. (2013). Toward integrated production and distribution management in multi-echelon supply chains. Comput. Chem. Eng. 57, 78–94. doi:10.1016/j.compchemeng.2013.01.004
Copado-Mendez, P. J., Blum, C., Guillen-Gosalbez, G., and Jiménez, L. (2013). Large neighbourhood search applied to the efficient solution of spatially explicit strategic supply chain management problems. Comput. Chem. Eng. 49, 114–126. doi:10.1016/j.compchemeng.2012.09.006
Corsano, G., Guillen-Gosalbez, G., and Montagna, J. M. (2014). Computational methods for the simultaneous strategic planning of supply chains and batch chemical manufacturing sites. Comput. Chem. Eng. 60, 154–171. doi:10.1016/j.compchemeng.2013.09.001
Dondo, R., Mendez, C. A., and Cerda, J. (2009). Managing distribution in supply chain networks. Ind. Eng. Chem. Res 48, 9961–9978. doi:10.1021/ie900792s
Dondo, R., Mendez, C. A., and Cerda, J. (2011). The multi-echelon vehicle routing problem with cross docking in supply chain management. Comput. Chem. Eng. 35, 3002–3024. doi:10.1016/j.compchemeng.2011.03.028
Dunnett, A., Adjiman, C., and Shah, N. (2007). Biomass to heat supply chains applications of process optimization. Process Saf. Environ. Protect. 85, 419–429. doi:10.1205/psep07022
Duque, J., Barbosa-Póvoa, A. P., and Novais, A. Q. (2007). Synthesis and optimisation of the recovery route for residual products with uncertain product demands. Comput. Oper. Res. 34, 1463–1490. doi:10.1016/j.cor.2005.06.008
Duque, J., Barbosa-Póvoa, A. P., and Novais, A. Q. (2010). Design and planning of sustainable industrial networks: application to a recovery network of residual products. Ind. Eng. Chem. Res. 49, 4230–4248. doi:10.1021/ie900940h
Eksioglua, S. A., Ambarish, A., Liam, E., and Sumesh, A. (2009). Analyzing the design and management of biomass-to-biorefinery supply chain. Comput. Ind. Eng. 57, 1342–1352. doi:10.1016/j.biortech.2013.09.120
Fernandes, L., Relvas, S., and Barbosa-Póvoa, A. P. (2014). Strategic network design of downstream petroleum supply chains: single versus multi-entity participation. Chem. Eng. Sci. 91, 1557–1587.
Georgiadis, M. C., Tsiaki, P., Longinidis, P., and Sofioglou, M. K. (2011). Optimal design of supply chain networks under uncertain transient demand variations. Omega 39, 254–272. doi:10.1016/j.omega.2010.07.002
Grossmann, I. E. (2012). Advances in mathematical programming models for enterprise-wide optimization. Comput. Chem. Eng. 47, 2–18. doi:10.1016/j.compchemeng.2012.06.038
Guide, D. R., and Van Wassenhowe, L. N. (2002). The reverse supply chain. Harv. Bus. Rev. 80, 25–26.
Guillen, G., Badell, M., Espuña, A., and Puigjaner, L. (2006). Simultaneous optimization of process operations and financial decisions to enhance the integrated planning/scheduling of chemical supply chains. Comput. Chem. Eng. 30, 421–436.
Guillen-Gosalbez, G., and Grossmann, I. E. (2009). Optimal design and planning of sustainable chemical supply chains under uncertainty. AIChE J. 55, 99–121. doi:10.1016/j.biortech.2011.11.090
Guillen-Gosalbez, G., and Grossmann, I. E. (2010). A global optimization strategy for the environmentally conscious design of chemical supply chains under uncertainty in the damage assessment model. Comput. Chem. Eng. 34, 42–58. doi:10.1016/j.compchemeng.2009.09.003
Gupta, A., and Maranas, C. D. (2003). Managing demand uncertainty in supply chain planning. Comput. Chem. Eng. 27, 1219–1227.
Gupta, A., Maranas, C. D., and McDonald, C. M. (2000). Mid-term supply chain planning under demand uncertainty: customer demand satisfaction and inventory management. Comput. Chem. Eng. 24, 2613–2621. doi:10.1016/S0098-1354(00)00617-7
Hamedi, M., Farahani, R. Z., Husseini, M. M., and Gholam, R. E. (2009). A distribution planning model for natural gas supply chain: a case study. Energy Policy 37, 3708–3708. doi:10.1016/j.enpol.2008.10.030
Huang, C., and Karimi, I. A. (2006). Scheduling trans-shipment operations in maritime chemical transportation. Ind. Eng. Chem. Res. 45, 1955–1973. doi:10.1021/ie060379j
Hugo, A., and Pistikopoulos, E. N. (2005). Environmentally conscious long-range planning and design of supply chain networks. J. Clean. Prod. 13, 1471–1491. doi:10.1016/j.jclepro.2005.04.011
Jackson, J. R., and Grossmann, I. E. (2003). Temporal decomposition scheme for nonlinear multisite production planning and distribution models. Ind. Eng. Chem. Res. 42, 3045–3055. doi:10.1021/ie030070p
Kallrath, J. (2002). Combined strategic and operational planning – an MILP success story in chemical industry. OR Spectrum 24, 315–341. doi:10.1007/s00291-002-0102-6
Kallrath, J. (2008). “Combining strategic design and operative planning in the process industry,” in Supply Chain Optimization: Part I, eds L. G. Papageorgiou and M. C. Georgiadis (Weinheim: Wiley-VCH), 219–243.
Klibi, W., and Martel, A. (2012). Modeling approaches for the design of resilient supply networks under disruptions. Int. J. Prod. Econ. 135, 882–898. doi:10.1016/j.ijpe.2011.10.028
Kostin, A. M., Guillén-Gosálbez, G., Mele, F. D., Bagajewiczc, M. J., and Jiménez, L. (2011). A novel rolling horizon strategy for the strategic planning of supply chains. Application to the sugar cane industry of Argentina. Comput. Chem. Eng. 35, 2540–2563. doi:10.1016/j.compchemeng.2011.04.006
Kostin, A. M., Guillén-Gosálbez, G., Mele, F. D., Bagajewiczc, M. J., and Jiménez, L. (2012). Design and planning of infrastructures for bioethanol and sugar production under demand uncertainty. Chem. Eng. Res. Des. 90, 359–376. doi:10.1016/j.cherd.2011.07.013
Lainez, J. M., Kopanos, G., Espunã, A., and Puigjaner, L. (2009). Flexible design-planning of supply chain networks. AIChE J. 55, 1736–1753. doi:10.1002/aic.11942
Lainez, J. M., Schaefer, E., and Reklaitis, G. V. (2012). Challenges and opportunities in enterprise-wide optimization in the pharmaceutical industry. Comput. Chem. Eng. 47, 19–28.
Lambert, D. M., and Cooper, M. (2000). Issues in supply chain management. Ind. Market. Manag. 29, 65–83.
Liu, M. L., and Sahinidis, N. V. (1996). Optimization in process planning under uncertainty. Ind. Eng. Chem. Res. 35, 4154–4165. doi:10.1021/ie9504516
Liu, S., Sawik, T., and Papageorgiou, L. G. (2013). Multiobjective optimisation of production, distribution and capacity planning of global supply chains in the process industry. Omega 41, 369–382. doi:10.1016/j.omega.2012.03.007
Longinidis, P., and Georgiadis, M. C. (2013). Managing the trade-offs between financial performance and credit solvency in the optimal design of supply chain networks under economic uncertainty. Comput. Chem. Eng. 48, 264–279.
Matos, S., and Hall, J. (2007). Integrating sustainable development in the supply chain: the case of life cycle assessment in oil and gas and agricultural biotechnology. J. Oper. Manag. 25, 1083–1102. doi:10.1016/j.jom.2007.01.013
Naraharisetti, P. K., and Karimi, I. A. (2010). Supply chain redesign and new process introduction in multipurpose plants. Chem. Eng. Sci. 65, 2596–2607. doi:10.1016/j.ces.2009.12.036
Naraharisetti, P. K., Karimi, I. A., and Srinivasan, R. (2008). “Chemical supply chain redesign,” in Supply Chain Optimization: Part I, eds L. G. Papageorgiou and M. C. Georgiadis (Weinheim: Wiley-VCH), 245–300.
Neiro, S. M. S., and Pinto, J. M. (2004). A general modeling framework for the operational planning of petroleum supply chains. Comput. Chem. Eng. 28, 871–896.
Nikolopoulou, A., and Ierapetritou, M. G. (2012). Optimal design of sustainable chemical processes and supply chains: a review. Comput. Chem. Eng. 44, 94–103.
Oliveira, F., and Hamacher, S. (2012). Optimization of the petroleum product supply chain under uncertainty: a case study in Northern Brazil. Ind. Eng. Chem. Res. 51, 4279–4287. doi:10.1021/ie2013339
Papageorgiou, L. G. (2009). Supply chain optimisation for the process industries: advances and opportunities. Comput. Chem. Eng. 33, 1931–1938.
Peidro, D., Mula, J., Poler, R., and Lario, F. C. (2009). Quantitative models for supply chain planning under uncertainty: a review. Int. J. Adv. Manuf. Technol. 43, 400–420. doi:10.1007/s00170-008-1715-y
Pinto-Varela, T., Barbosa-Póvoa, A. P., and Novais, A. Q. (2011). Bi-objective optimization approach to the design and planning of supply chains: economic versus environmental performances. Comput. Chem. Eng. 35, 1454–1468.
Ryu, J. H., and Pistikopoulos, E. N. (2007). Multiperiod planning of enterprise-wide supply chains using an operation policy. Ind. Eng. Chem. Res. 4, 8058–8065. doi:10.1021/ie070508b
Salema, M., Barbosa-Póvoa, A. P., and Novais, A. (2010). Simultaneous design and planning of supply chains with reverse flows: a generic modelling formulation. Eur. J. Oper. Res. 203, 336–349. doi:10.1016/j.ejor.2009.08.002
Shah, N. (2005). Process industry supply chains: advances and challenges. Comput. Chem. Eng. 29, 1225–1235. doi:10.1016/j.ejps.2012.06.009
Shah, N. K., and Ierapetritou, M. G. (2012). Integrated production planning and scheduling optimization of multisite, multiproduct process industry. Comput. Chem. Eng. 37, 214–226.
Shapiro, J. F. (2004). Challenges of strategic supply chain planning and modeling. Comput. Chem. Eng. 28, 855–861.
Sousa, R. T., Liu, S., Papageorgiou, L. G., and Shah, N. (2011). Global supply chain planning for pharmaceuticals. Chem. Eng. Res. Des. 89, 2396–2409. doi:10.1016/j.cherd.2011.04.005
Srivastava, S. K. (2007). Green supply-chain management: a state-of-the-art literature review. Int. J. Manag. Rev. 9, 53–80. doi:10.1111/j.1468-2370.2007.00202.x
Stephanopoulos, G., and Reklaitis, G. V. (2012). Process systems engineering: from Solvay to modern bio- and nanotechnology: a history of development, successes and prospects for the future. Chem. Eng. Sci. 66, 4272–4306. doi:10.1016/j.ces.2011.05.049
Tong, K., Gleeson, M.-J., Rong, G., and You, F. (2014a). Optimal design of advanced drop-in hydrocarbon biofuel supply chain integrating with existing petroleum refineries under uncertainty. Biomass Bioenergy 60, 108–120. doi:10.1016/j.biombioe.2013.10.023
Tong, K., Gong, J., Yue, D., and You, F. (2014b). Stochastic programming approach to optimal design and operations of integrated hydrocarbon biofuel and petroleum supply chains. ACS Sustain. Chem. Eng. 2, 49–61. doi:10.1021/sc400267t
Tsiakis, P., and Papageorgiou, L. G. (2008). Optimal production allocation and distribution supply chain networks. Int. J. Prod. Econ. 111, 468–483. doi:10.1016/j.ijpe.2007.02.035
Tsiakis, P., Shah, N., and Pantelides, C. C. (2001). Design of multi-echelon supply chain networks under demand uncertainty. Ind. Eng. Chem. Res. 40, 3585–3604. doi:10.1021/ie0100030
van den Heever, S. A., and Grossmann, I. E. (2003). A strategy for the integration of production planning and reactive scheduling in the optimization of a hydrogen supply network. Comput. Chem. Eng. 27, 1813–1839. doi:10.1016/S0098-1354(03)00158-3
Verderame, P. M., and Floudas, C. A. (2009). Operational planning framework for multisite production and distribution networks. Comput. Chem. Eng. 33, 1036–1050.
Vidal, C. J., and Goetschalckx, M. (1997). Strategic production-distribution models: a critical review with emphasis on global supply chain models. Eur. J. Oper. Res. 98, 1–18. doi:10.1016/S0377-2217(97)80080-X
Wilkinson, S. J., Cortier, A., Shah, N., and Pantelides, C. C. (1996). Integrated production and distribution scheduling on a Europe-wide basis. Comput. Chem. Eng. 20, S1275–S1280. doi:10.1016/0098-1354(96)00220-7
You, F., and Grossmann, I. E. (2008). “Optimal design and operational planning of responsive process supply chains,” in Supply Chain Optimization: Part I, eds L. G. Papageorgiou and M. C. Georgiadis (Weinheim: Wiley-VCH), 107–134.
You, F., Grossmann, I. E. (2013). Multicut benders decomposition algorithm for process supply chain planning under uncertainty. Ann. Oper. Res. 210 191–211. doi:10.1007/s10479-011-0974-4
You, F., Pinto, J. M., Capon, E., Grossmann, I. E., Arora, N., and Mega, L. (2011a). Optimal distribution-inventory planning of industrial gases. I. Fast computational strategies for large-scale problems. Ind. Eng. Chem. Res. 50, 2910–2927. doi:10.1021/ie101758u
You, F., Pinto, J. M., Capon, E., Grossmann, I. E., Arora, N., and Mega, L. (2011b). Optimal distribution-inventory planning of industrial gases. II. MINLP models and algorithms for stochastic cases. Ind. Eng. Chem. Res. 50, 2928–2945. doi:10.1021/ie101758u
You, F., and Wang, B. (2011). Life cycle optimization of biomass-to-liquid supply chains with distributed-centralized processing networks. Ind. Eng. Chem. Res. 50, 10102–10127. doi:10.1021/ie200850t
You, F., Wassick, J. M., and Grossmann, I. E. (2009). Risk management for a global supply chain planning under uncertainty: models and algorithms. AIChE J. 55, 931–946. doi:10.1002/aic.11721
Zamarripa, M. A., Aguirre, A. M., Mendez, C. A., and Espuña, A. (2012). Improving supply chain planning in a competitive environment. Comput. Chem. Eng. 42, 178–188.
Keywords: supply chains, process operations, design, planning, optimization, uncertainty, risk, sustainability
Citation: Barbosa-Póvoa AP (2014) Process supply chains management – where are we? Where to go next? Front. Energy Res. 2:23. doi: 10.3389/fenrg.2014.00023
Received: 15 April 2014; Accepted: 09 June 2014;
Published online: 23 June 2014.
Edited by:
Gurkan Sin, Technical University of Denmark, DenmarkReviewed by:
Thomas Alan Adams, McMaster University, CanadaFengqi You, Northwestern University, USA
Carlos Alberto Mendez, Universidad Nacional del Litoral, Argentina
Copyright: © 2014 Barbosa-Póvoa. This is an open-access article distributed under the terms of the Creative Commons Attribution License (CC BY). The use, distribution or reproduction in other forums is permitted, provided the original author(s) or licensor are credited and that the original publication in this journal is cited, in accordance with accepted academic practice. No use, distribution or reproduction is permitted which does not comply with these terms.
*Correspondence: Ana Paula Barbosa-Póvoa, Center for Management Studies of IST (CEG-IST), Instituto Superior Técnico, DEG, 1049-001 Lisbon, Portugal e-mail: apovoa@tecnico.ulisboa.pt