- 1Department of Architecture & Planning, Malaviya National Institute of Technology Jaipur, Jaipur, India
- 2Department of Hydro and Renewable Energy, Indian Institute of Technology Roorkee, Roorkee, India
Net zero energy building (NZEB) is an efficient approach to boost the world’s sustainable development and climate mitigation. This paper provides a comprehensive review of multi-objective optimization (MOO)-based case studies of NZEB design. The critical analysis focuses on the “3Ps” criteria of sustainability, which encompass social, environmental, and economic aspects of protecting the people, planet, and profit. This study identifies the gaps in exploring case studies based on MOO techniques for decision-making regarding NZEB design in India and its requirements. Moreover, it demonstrates various passive design strategies, energy-efficient technologies, and renewable energy, and their impact on occupant comfort, energy model, and the building life cycle. It presents the global needs and requirements of NZEB, and definitions of NZEB in different countries and their policies. This review suggests a four-step methodological framework for NZEB design in India. It provides the potential and reliability of various algorithms, tools, and simulation engines to solve building problems. The framework presented here can aid designers in the decision-making of NZEB design, refurbishment, and renovation. Moreover, this study highlights the future research direction and potential challenges for implementing the proposed framework in India.
1 Introduction
The prime concerns for maintaining sustainability are increasing energy demand and global warming around the world due to rapid urbanization and climate change (Wilberforce et al., 2023). The building sector is the major contributor to global energy demand and GHG emissions (Zhang et al., 2018). The International Energy Agency (IEA) reported that buildings consumed 30% of the world’s total primary energy supply (TEPS) in 2015 (Xue and Liu, 2023; Ürge-Vorsatz et al., 2020). Building floor area may increase by 75% across the globe between 2020 and 2050, especially in developing countries, and up to 80% in countries like India and China. Therefore, the share of electricity may rise from 33% in 2020 to 50% and 66% in 2030 and 2050, respectively (Bano and Sehgal, 2019; Net Zero by 2050, 2021).
From 2005 to 2030, India’s built fabric will grow at a rate of 6.6% per year against the world growth rate of 5.2%. As per the IEA 2021 report, built area is expected to double over the next two decades (Sen et al., 2021; Gupta et al., 2023). The building of dwellings continuously grew due to India’s economic expansion. In 2017, total households were 272 million, which is predicted to increase to 328 and 386 million by 2027 and 2037, respectively (Sudhakar et al., 2019). Figure 1 shows the projected built area for residential buildings, both urban and rural. It demonstrates that in 2020, the residential built fabric was 17.7 billion m2, and it may increase three to four times by 2050 (India cooling action plan operationalizing space cooling recommendations and Ministry of Environment).
This has had a tremendous impact on electrical energy demand and the supply chain. The building sector accounts for 33% of total electricity generated in India, with residential buildings consuming 26%. This is projected to increase three to five times by 2031. The sector-wise electricity consumption in India in 2017 is shown in Figure 2 (Khosla and Janda, 2019). India generates the most energy through fossil fuels, which will ultimately contribute to GHG emissions. Meanwhile, India is the fourth largest carbon emitting country, in which the power sector accounts for half of all carbon emissions (Aggarwal et al., 2020; Jain and Rawal, 2022). Looking at the global emission scenario, India signed the Paris Agreement in 2015 and committed to reducing the country’s GHG emissions by 30% of its GDP by 2030. Furthermore, India set a target in COP26 of achieving net zero by 2070 (Kishore, 2022; Summary for Policymakers, 2018).
Therefore, transformations in the building sector are required, along with climate-responsive envelope design, energy-efficient appliances, bioclimatic assessment, efficient materials, efficient cooling and heating technologies, smart control and monitoring systems, and renewable energy (RE) (Cabeza and Chàfer, 2020; Saini et al., 2022). To address such building-related energy issues, the terms “net-zero energy building” (NZEB) or “zero energy building” describe buildings that produce as much energy as they consume over the course of a year. “Zero energy” means that the energy consumption of a building and the energy generated on-site through renewable energy sources like the sun and wind are the same (Moghaddasi et al., 2021a; Raj et al., 2021).
The concept of a net-zero building emphasizes the “3Ps” structure of sustainability—social (user satisfaction), environmental (energy efficiency, CO2 emissions), and economic (cost-effectiveness) —that encompass people, planet, and profit (Supplementary Figure S1) (Costa-Carrapiço et al., 2020; Slaper and Hall, 2011). There are usually four conventional design factors that lead to the formation of NZEB: passive design features, energy efficiency measures, renewable energy, and storage backup systems (Supplementary Figure S2) (Cabeza and Chàfer, 2020; Wu and Skye, 2021).
Several studies have reported that the hierarchy of NZEB focuses on building sufficiency, building efficiency, and renewable energy sources (RESs). Energy sufficiency emphasizes climate-adaptive design and the building of passive features to reduce energy demand. Energy efficiency includes efficient lighting, appliances, HVAC systems, heat storage systems, and building control systems that can reduce renewable energy demand. RESs include solar PV, wind turbines, solar collectors, and geothermal energy to fulfil a building’s operational demand. The biggest challenge is the simultaneous implementation of several design strategies and technologies without sacrificing a building’s social, environmental, and economic performance to build a net-zero or net-carbon structure. Therefore, research has used various building simulation tools and computational algorithmic optimization techniques for the early-stage design, refurbishment, and retrofit of high-performance and NZEB developments (Omrany et al., 2022; Sola et al., 2018). The intensive literature of the previous study identified algorithmic-based multi-objective optimization approaches as the most promising strategies for decision-making (DM) in high-performance or NZEB at the earlier design stage. Therefore, various MOO-based studies of various climatic zones were critically analysed; their comprehensive summaries in chronological order are listed in the Supplementary Table S1. Furthermore, despite this extensive research, a significant research gap was identified for net-zero energy residential building design in the Indian context, which is divided into two categories.
1. A deficiency of studies using algorithmic optimization techniques for the robust optimal solution of NZEB development that can satisfy several target performance objectives simultaneously while considering a wide range of design variables.
2. No framework is available for the development of NZEB that defines a hierarchy of performance objectives and their associated criteria for evaluation, design variables, optimization set-up, selection of a Pareto optimal solution after robustness assessment, and sensitivity analysis to investigate dominant design variables in the respective climatic zone.
The aim of this research is to identify several building performance objectives that encompass the social, environmental, and economic criteria of sustainability. Moreover, it aims to reveal the various factors and their assessment criteria for maintaining a healthy and comfortable indoor environment. It will also demonstrate the effectiveness of multi-objective optimization and its potential for decision-making in NZEB development with a wide range of design variables under various uncertain conditions. It illustrates the reliabilities and capabilities of various algorithms, tools, and simulation software after a critical comparative investigation. Finally, this research recommends a four-step framework based on MOO that demonstrates the hierarchy of NZEB design in India. This research contains the following subsequential sections: NZEB definitions and their policies, methodology, summary and discussion, the optimization framework for NZEB in India, a conclusion and future research directions, and potential challenges and suggestions for implementing the proposed NZEB design framework in India.
2 NZEB definitions and their policies
Many countries have set the target of achieving net-zero buildings and define their own definition of net-zero emission buildings (NZEB). Currently, more than 65 definitions and standards are available to define net-zero/zero-carbon buildings as per region-specific conditions. The concept of “zero-energy building” was proposed by Esbensen and Korsgaard from the University of Denmark in 1976 (Lin et al., 2020). After that, many zero-energy buildings were constructed in developed countries, and definitions of them were proposed by many researchers. Table 1 shows the definitions of NZEB available in different countries and their policies to achieve this goal.
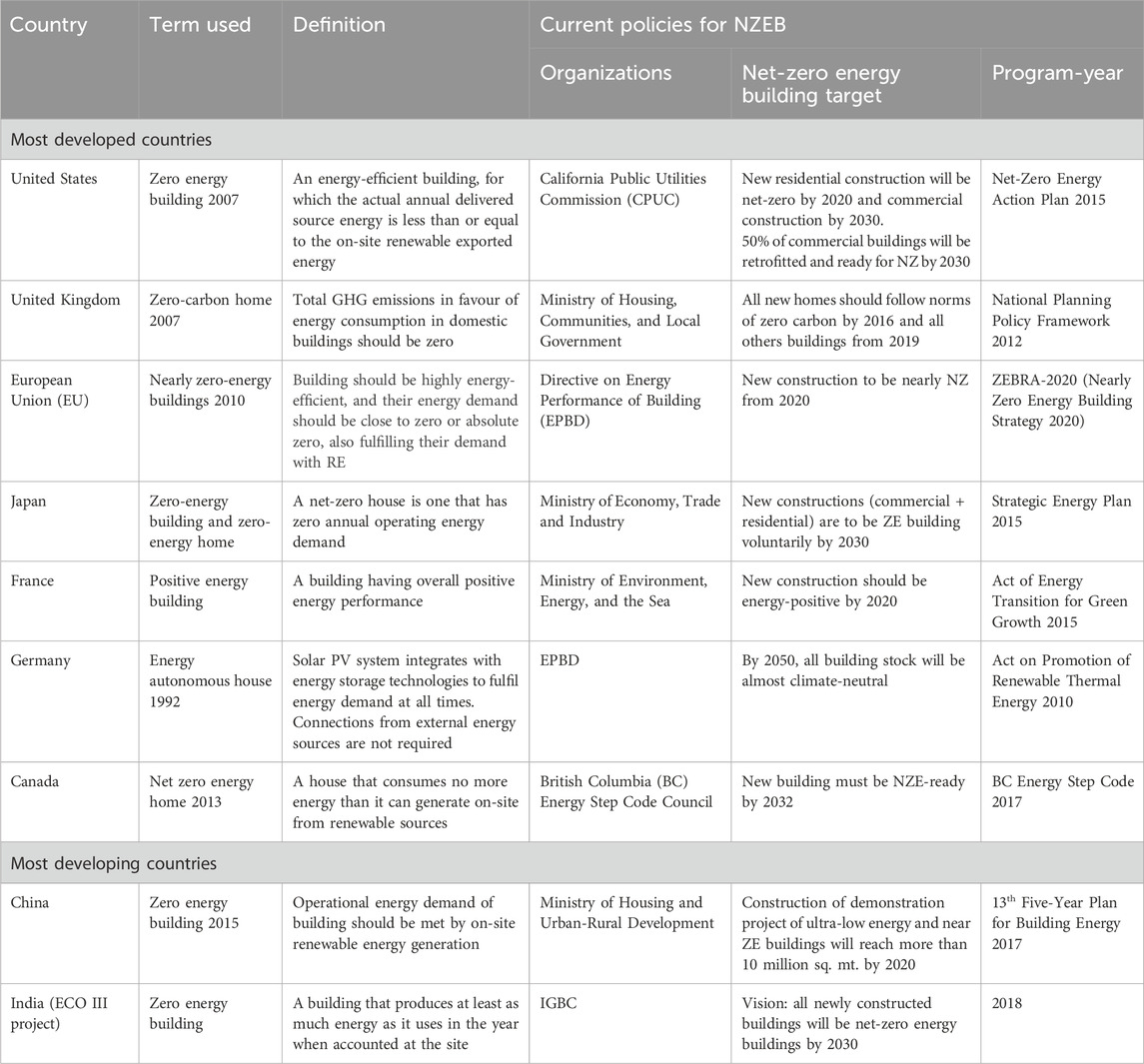
Table 1. Country-wise net-zero definition and policies (Feng et al., 2019; Hu and Qiu, 2019; Dalal-Kulkarni and Gokhale, 2011; Williams et al., 2016; Energy Step Code Council BC Energy Step Code, 2017; basic energy plan of Japan, 2014).
Torcellini and colleagues classified net zero building into four categories based on the balanced energy measurement process (Torcellini et al., 2006; Pless and Torcellini, 2010).
• Net-zero site energy: A building that meets its annual energy demand by renewable energy (RE). The generation of RE is at least equivalent to operational energy demand.
• Net-zero source energy: A building that generates RE as much as the amount of both operational demand and transmitted energy losses from the grid to the building. The energy losses are due to factors such as transmission, distribution, and primary fuel supply.
• Net-zero cost energy: A building that covers utility bills by selling the RE produced. It can also produce profits, but at the very least it should entirely cover the bills.
• Net zero-emission: A building that produces enough energy for consumption and compensates for the emissions produced by generating enough renewable energy.
2.1 Definition according to international organizations
According to the American Society of Heating, Refrigerating, and Air-Conditioning Engineers (ASHRAE), if energy crosses the building’s physical boundaries after fulfilling its annual energy demand with a renewable resources, then that building is labelled a NZEB (ASHRAE, 2008). The Federation of European Heating, Ventilation, and Air-Conditioning (REHVA) defines an NZEB as any building that fulfils its annual energy demand by on-site energy production, and excess energy is fed to the electricity grid to green credit (Voss, 2012). According to the European Directive on Energy Performance of Building (EPBD), any building having minimum energy requirements and whose annual demand is fulfilled by on-site or nearby RE resources is called a NZEB (Chiesa et al., 2019; Chaturvedi et al., 2024a). The US Department of Energy (DOE) defines a building having a minimum energy performance index (EPI) and 30%–40% of its energy demand fulfilled by RE technologies as a NZEB. If, in a residential building, the on-site generation of RE is higher than its consumption, that building is considered a passive net-zero house by the DOE (U.S. DOE, 2007). The International Energy Agency (IEA) states that any building fulfilling its energy requirements with RE resources and does not use any conventional energy sources such as fossil fuels is termed a NZEB. The concept of NZEB was introduced in India through the third phase of energy conservation and commercialization (ECO) (Kapoor et al., 2011). The motive was to enhance NZEB construction, increase energy production by renewable sources, and use efficient technologies and lighting systems in the building sector. The Indian Green Building Council (IGBC) launched the net-zero energy certification for commercial buildings in 2018. According to this, the ratio of actual energy performance to design energy performance should be a unity, and it should only be fulfilled by renewable energy resources (IGBC NetZero Energy Buildings, 2021; bureau of energy efficiency).
There are multiple criteria available to define “net zero”, such as the source of generation, variation in supply requirements and grid dependence, emission, and cost. Based on the available literature, Table 2 shows the NZEB definitions. The assessment criteria include on-site energy generation demands, the generation of RE within the building boundary (perimeter of the site) or building footprint (walls and roof). Off-site energy generation includes importing RE outside the building’s physical boundary or importing renewable sources to produce on-site electricity. Balanced demand is addressed, as is RE generation equivalent to the annual operational energy demand and transported energy losses from the energy grid to the building utilization process. Building design contains a building equipped with an advanced envelope design and efficient technology measures. Using RE for utilities and services in buildings will be considered as compensation for CO2 emissions (Wu and Skye, 2021; Harkouss et al., 2018a; Moghaddasi et al., 2021b).
Unfortunately, NZEB has been characterized by ambiguous and inconsistent calculation methods. This lack of homogeneity among NZEB definitions requires a recognized universal definition for it due to it becoming the main research stream across the world (Sartori et al., 2012). The lack of inherent consistency in an accepted definition of NZEB not only affects the creation of an unambiguous profile for it across the global community, hindering unified goal for global energy-efficient building policy, but also poses significant challenges to comparing various solutions in different contexts (Chaturvedi et al., 2024a; Lützkendorf and Frischknecht, 2020).
3 Methodology
Climate change has a significant impact on comfort, energy models, CO2 emissions, and the life cycle cost of buildings (Wang et al., 2023). According to the literature, the earth’s temperature is rising by 1.5 ℃ per decade due to GHG emissions. This has tremendous impacts on occupant behaviour, cooling and heating consumption patterns, and building life (The Future of Cooling, 2018; Khosla et al., 2019). Therefore, climate-adaptive high-performance or NZEB designs have become a principal research domain for reducing energy demand and limiting climate change. Its optimization has the potential to identify decision variables that can achieve objective functions and satisfy constraints. It provides the conceptual framework for developing and contrasting new design solutions for attaining Pareto solutions (Chaturvedi and Elangovan, 2023; Loonen et al., 2017).
In building design, two archetypes of optimization frameworks are single-objective optimization (SOO) and multi-objective optimization (MOO) (Hensen and Lamberts, 2011; Hamdy et al., 2013). In SOO, the DM process entails the performance objective of exploring the wide range of design variables. It is a time-consuming process to solve the design of large space, so it is not a feasible way to solve complex problems because of the trade-off between dealing with maximum user satisfaction, minimum energy demand, and life-cycle cost (Chaturvedi et al., 2023). Therefore, MOO plays a vital role in solving complex building-associated problems. It provides a set of mono solutions through the investigation of the wide range of design spaces without compromising the defined performance objectives (Bandyopadhyay and Pal, 2007; Escandón et al., 2019; Evins, 2013). Based on this concept, we identified relevant case studies (CS) based on inclusion and exclusion criteria. Figure 3 shows the methodological hierarchy adopted for this research. All the critically analysed case studies are systematically described in Supplementary Table S1 and are outlined in Table 3. All reviewed CS are critically investigated based on criteria which include optimization design variables and social, energy, environmental, and economic parameters. Optimization design variables were further categorized into the building envelope, energy-efficient systems, building control systems, and renewable energy source parameters. Social criteria are mapped as thermal comfort, visual comfort, and indoor air quality. Energy parameters are summarized as energy consumption, energy demand, and energy savings. Environmental parameters cover CO2 emissions, natural resource uses, and the life-cycle carbon footprint. The economic parameters include life-cycle cost. The principal hierarchy of the reviewed primary studies covers the publication year, objective functions, location, climate zone, building typology, design variables, simulation and optimization algorithms with algorithms, outcomes, and sustainability scopes (more detail listed in Supplementary Table S1). The characteristics of the primary case studies reviewed are discussed below.
• The reviewed case studies mainly focus on the triple bottom line of sustainability: social (people), environmental (planet), and economic (profit).
• A total of 18 reviewed primary studies covered uncertainty analysis in such areas as climate change, policy scenarios, life cycle, occupant behaviour, thermal and surface properties, and HVAC systems. These studies revealed the impact of uncertainty on building performance and energy models.
• CS mostly focused on social and environmental aspects to minimize the operational and embodied energy demand.
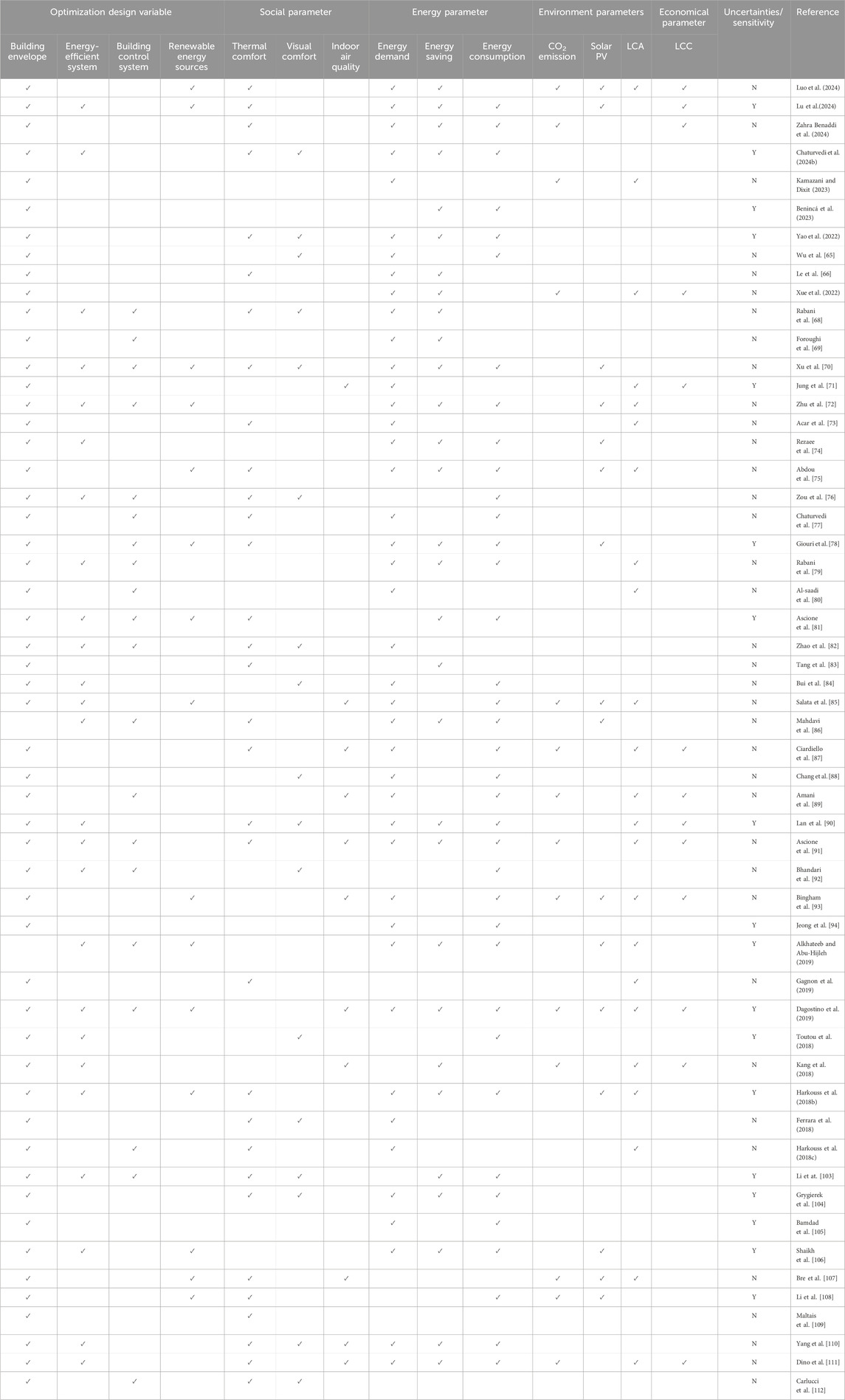
Table 3. Detailed objective functions and design variables addressed in the reviewed case studies, listed in chronological order.
4 Summary and discussion
Several studies of multi-objective optimization of NZEB/energy-efficient buildings are abbreviated based on the “3Ps” scale of sustainability. The adopted methodological structure for this research is classified as follows.
• Data collection: identify the relevant primary and secondary data and summarize it based on objective inclusion and exclusion criteria.
• Mapping criteria: identify base case studies related to characteristics of the published year, geographical context, climatic boundaries, optimization tools and techniques, derived methodology, decision variables, scientific qualities, and standards
• Assessment criteria: evaluation of a base case model addressing socioeconomic and environmental aspects.
• Identified gap: through extensive in-depth study, investigating the application of algorithmic multivariate optimization for NZEB development in the Indian context.
Figure 4 illustrates the maximum number of multivariate optimization techniques applied to residential buildings (58%), followed by commercial buildings (29%), educational buildings (7%), hotels (2%), and prototypes (3%). Computational optimization strategies for decision-making regarding NZEB are highly attentive to residential buildings due to the higher building footprint; these buildings have considerable energy-saving potential. Limited studies are also available on other building typologies.
All reviewed CS emphasized the triple bottom line of sustainability, followed by socio-economic and environmental aspects. Figure 5 shows that 85% of the reviewed primary studies covered the environmental scope, followed by social and economic aspects (64% and 51%, respectively). The extensive literature of case studies identified that most of the optimization employed building envelope parameters (96%), followed by energy efficiency systems including lighting and HVAC (45%), controlling and monitoring systems (36%), and renewable energy systems (27%). These design variables satisfied the objectives of minimizing energy demand, environmental impact, cost, and improving thermal and visual comfort and health productivity.
Moreover, sensitivity analyses were performed by the authors, and they observed which design variable was highly used in MOO as the decision matrix. The influence variables are listed in Figure 6: advanced envelope, floor plan, solar shading, insulation, WWR, blinds control system, advanced glazing, thermal mass, natural ventilation, HVAC system, lighting control, equipment, solar PV area, and tilt angle.
The advanced envelope comprises thickness, thermal conductivity, and the density of walls and roof. It is the most optimized and explored parameter undertaken by 97% of case studies for DM. HVAC parameters encompass air-conditioning type, size, schedule, thermal zoning, heat recovery systems, and radiant cooling. Lighting and control parameters comprise efficient lighting, advanced lighting control, skylights, and solar tubes. HVAC and lighting parameters taken for optimized decision-making in the defined case studies are 49% and 47%, respectively. Renewable energy systems and parameters such as photovoltaic systems, geothermal heat pumps, PV tilt angle and efficiency, and solar PV area are explored in 22% of the studies.
Different tools are used for computational optimization that work on static and dynamic simulation approaches. For example, the EnergyPlus optimization engine was used in 54% of studies, and TRNSYS, Design Builder, IDA IDC, and Rhino were used in 14%, 18%, 4%, and 10% of reviewed CS, respectively.
5 Optimization framework for NZEB in India
Previous studies have suggested various NZEB design frameworks for different regions of the world. Piderit et al. (2019) developed the NZEB framework based on building performance criteria suited to Chilean climatic conditions and an expert group discussion of the Chilean and European regions. This framework provides a dual approach for integrating thermal comfort, active systems, and internal loads and highlights the socio-economic feasibility of implementing NZEB in Chile. This framework’s adaptability is crucial for other regions due to varying technological and economic capabilities. Harkouss et al. (2018a) suggested a three-stage flowchart for decision-making in designing, optimizing, and categorizing NZEB. This framework often focuses on advanced simulation tools for optimization and ensuring high levels of precision and accuracy. However, this European framework does not involve the impact of uncertainties including weather and operations on building design and life-cycle performance. Hence, this framework cannot be adopted in other regions due to different economic and technological landscapes. Barber and Krarti (2022) provide a comprehensive framework aligned with an easy-to-use combined design and a control-oriented optimization toolset for building professionals to make informed decisions about net-zero design. This framework focuses on achieving net-zero building design by optimizing the integrated energy system and peak load management. Barber and Krarti (2022) do not explain the capabilities of building simulation tools and the robustness of the Pareto solution to enable net-zero design. Satola et al. (2021) presented a framework for net-zero carbon building design in the tropical climate of developing countries. They discussed the various regulatory options for implementing net-zero carbon emission standards into national and local policies. However, this study mainly pertains to operational energy demand and carbon emission during the entire life cycle of building and overlooks socioeconomic performance such as occupant comfort, IAQ, and life-cycle cost. Ascione et al. (2019) proposed a novel optimization paradigm using the Harlequin algorithm to consider the comfort, energy, and economic indicators of NZEB design for the Italian climate. Similarly, Lan et al. (2019) illustrated a holistic two-step optimization structure for DM of NZEB design for Singapore. Cabeza and Chàfer (2020) revealed various technological design options and strategies for carbon-neutral building design. Meanwhile, little research has focused on NZEB design regarding the “3Ps” criteria (social, environmental, and economical) of sustainability. In this study, through an extensive investigation of previous studies (Table 3 and Supplementary Table S1), a systematic framework is provided for NZEB development in India. This framework emphasizes the MOO technique to primarily focus on the “3Ps” criterion of sustainability. Moreover, it enables computing a large set of design variables including active, passive, and RE parameters, and helps provide the mono solution of NZEB design. Moreover, this framework discusses the various uncertainties in assessment for robust building design solutions. It contains four sequential steps (Figure 7). Step 1 shows the optimization framework, step 2 discusses the multi-criteria decision-making (MCDM) approach, step 3 demonstrates the robustness analysis, and step 4 reveals the grid connectivity to make the NZEB.
5.1 Optimization framework
The optimization framework consists of an optimization setup, objective functions, and design variables. It investigates the various building performance objectives and provides the Pareto optimal solutions by exploring the wide range of design variables using a computational algorithmic optimization approach. Multi-objective optimization processes require two types of input: building performance matrices (BPM) and decision variable matrices (DVM). BPMs include challenges such as thermal comfort, visual comfort, indoor air quality (IAQ), energy efficiency (operational and embodied), and life cycle cost, which can be investigated simultaneously due to their iterative relationships. DVMs include the typical building parameters and energy measures, which can be explored to identify the optimal combinations without sacrificing BPM (Fang and Cho, 2019; Ascione et al., 2015). The subsections of the optimization framework are discussed below.
5.1.1 Optimization setup.
The optimization workflow hierarchy consists of a collection of tools, algorithms, and software. The software provides the user interface to formulate the MOO problem (Hong et al., 2018). Tools support tracking the problem coupled with an adequate optimization algorithm to provide the set of mono solutions. In building design and construction, two optimization search strategies are used: non-evolutionary (NEC) and evolutionary (EC) techniques. NEC techniques are primitive practices for the building sector in terms of decision-making because of several pitfalls and user input limitations. The EC technique is a sub-branch of computational intelligence inspired by natural evolution (human being as a biological organism; foraging and social behaviour of swarming and locating food, movement; pattern of an ant for searching food) (Hajela and Lin, 1992; Fancello et al., 2014; Cheung and Lee, 2012). However, several EC technique-based algorithms are accessible for expert-based optimization in the construction industry. EC generally integrates with dynamic energy simulation tools through an iterative stratagem. Previous studies have reported that various algorithms, such as deterministic, stochastic, and fuzzy logic, are available to solve the building associate problem. The stochastic algorithm is mostly used because probability and statistical methods randomly select the next calculation step in the expectation process. It has higher efficiency in solving complex problems (Mahmoud and Ahmed, 2015; Machairas et al., 2014). Some of the metaheuristic algorithms used by NZEB for decision-making are described below.
Genetic algorithms (GA) are the most famous evolutionary algorithm inspired by the Darwin’s theory of survival of the fittest. This concept was introduced in Holland around the 1960s and implemented in the 1980s by Schaffer to solve the machine learning problem. It is the most robust heuristic approach to solving multi-objective problems, working on the principle of population-based natural selection phenomena (Holland, 1992; Deb et al., 2002)
Non-dominated sorting genetic algorithm-II (NSGA-II) was introduced by Deb with a modified version of NSGA in the 2000s. It is the most reliable tool for building optimization. In this method, the initial population of size N is generated through the operations of selection, crossover, and mutation; in the second phase, the two parent populations and their offspring populations are combined, and fast non-dominated sorting is performed (Seshadri, 2006).
Multi-objective particle swarm optimization (MOPSO), proposed by Moore and Chapman, is characterized by a high computational process, excellent manoeuvrability, and convergence. The first population archive set is initialized, and the second non-dominated solutions are calculated and sorted in the archive. Thence, the velocity and position of the particles are updated and crowding is calculated. Finally, the non-dominated solution in the archive is updated (Bai, 2010).
Multi-objective differential evolution (MODE), introduced by Rainer Storn and Kenneth Price, has the inherent potential for randomly selecting the optimized population. The initial population is generated in a Gaussian distribution. Due to the uncertainties in input parameters, the outliers exceed the distribution, and then distance matrix relations are used for sorting the outliers and generating the Pareto solutions (Wang et al., 2014).
Artificial bee colony (ABC) works on the mechanism of an artificial neural network (ANN) and therefore enhances the convergence and computational process of optimization. Sometimes, disparity in input parameters and user bias mean that it is unable to meet the objective criteria and Pareto solution (Yan and Li, 2011; Pernodet et al., 2009).
Octopus is a multi-objective optimization parametric design algorithm that works on the evolutionary principle. It is used to design and model the space for rotational daylight distribution, solar heat gain, and reduced energy intensity with the Grasshopper plugin. It can automatically adjust variables, generate design iterations, trigger simulations, and toggle and record solutions (Dino and Üçoluk, 2017; Besbas et al., 2022; Sun et al., 2020).
5.1.2 Multi-objective optimization tools
Various building energy optimization tools (BEOTs) have been used in previous research. Table 4 shows the features of optimization tools used in building design. These BEOTs are classified into different categories based on their computational mechanisms (Bingham et al., 2019; Jeong et al., 2019) which are detailed below.
• Simulation-based generic optimization tools, in which the optimization algorithm is linked with the simulation engine. It provides the user interface for the external inputs to accomplish energy optimization. (e.g., Gen-Opt, Opt-E-Plus, Be-Opt, Design Builder Optimization module) (Abdou et al., 2021; Chaturvedi et al., 2020; Rabani et al., 2020; Salata et al., 2020; D’Agostino et al., 2019; Ferrara et al., 2018; Carlucci et al., 2015).
• Congenital optimization tools are used to perform energy simulation and data analysis. They have their own optimization engines through which users can choose any optimization algorithm. (e.g., JE Plus +EA, Grasshopper, MOBO, ENEROPT, MultiOpt) (Chaturvedi et al., 2024b; Kamazani and Dixit, 2023; Benincá et al., 2023; Xu et al., 2021; Rezaee et al., 2021; Zhao and Du, 2020; Lan et al., 2019; Bhandari and Sundaram, 2019; Bamdad et al., 2018)
• Customized tools can be used to optimize any objective function written in several programming languages, and they can be integrated with any energy simulation tool. (e.g., Fortran, C++, C, Visual Basic in Microsoft Excel) (Jung et al., 2021; Giouri et al., 2020; Bui et al., 2020; Kang et al., 2018).
A number of energy performance simulation engines available, such as Energy Plus, TRNSYS, eQuest, IDA-ICE ESP-r, BLAST, and HVAC-SIM, work on dynamic and static modelling approaches. Table 5 shows the available building simulation engines and their modelling features that support the optimization tools. The US Department of Energy developed EnergyPlus. It is an open-source platform, and users can provide text-based inputs and outputs coupled with external software such as “design-builder”. TRNSYS is the second most popular and user-friendly software. It was developed by the University of Wisconsin Madison (United States). It is a transient-based simulation tool and provides the flexibility to demonstrate different configurations. DOE-2 was developed in Lawrance Barkeley national laboratory in California and eQUEST was developed by energy-model.com San Francisco. IDA-ICE and some other graphical modelling tools such as Open Studio, SketchUp, and Revit, are also available to compute the optimization process.
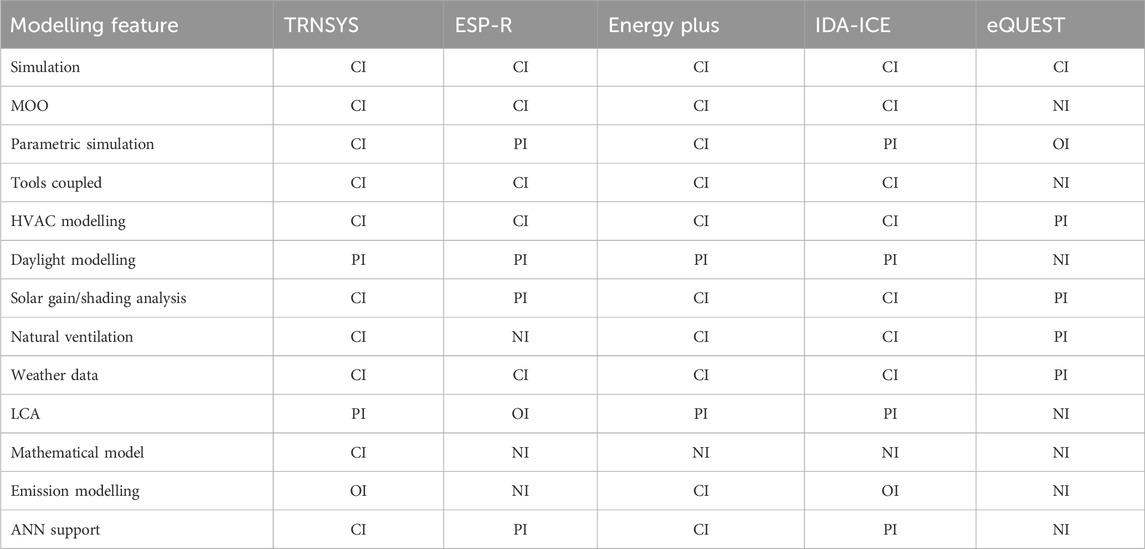
Table 5. List of building simulation software and their modelling features (CI, completely implemented; PI, partially implemented; OI, optionally implemented; NI, not implemented) (Harish and Kumar, 2016; Crawley et al., 2008).
5.1.3 Building performance objectives
NZEB is concerned with a number of building performance objective scenarios that are closely linked to social, environmental, and economic building behaviour. Social criteria are mapped as thermal comfort by natural ventilation, visual comfort through natural contact, and indoor air quality by maintaining an indoor environment. Environmental criteria encompass energy efficiency by installing efficient lighting, HVAC, and appliances and by reducing GHG emissions by adopting sustainable materials. Economic criteria focus on reducing the building life cycle cost (LCC) (Supplementary Figure S3).
We deeply analysed these case studies and identified various factors that affect the building performance objectives based on sustainability criteria (Figure 8. The dominance and impact of these parameters on building performance and energy modelling depend on climatic conditions.
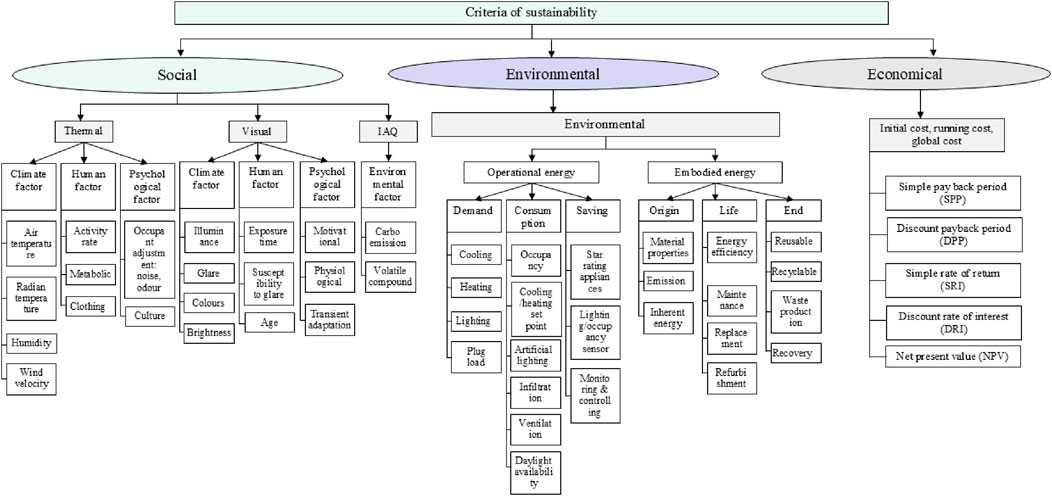
Figure 8. Identified parameters that affect the social, environmental, and economic criteria of sustainability.
5.1.4 Design variables
Case studies that were investigated reveal that the DM for NZEB explored a wide range of design variables. These variables are categorized into four parts: passive parameters, energy efficiency measures, renewable energy sources, and building control systems (Table 6).
5.2 Multi-criteria decision making
Multi-optimization runs multiple input data sets in real time and provides the set of mono solutions. The MCDM technique is used to identify the optimal Pareto solution using a defined objective function with a trade-off. Therefore, various methods can be applied to solve the trade-off problem in MOO. The most common are aggregating and outranking methods. Aggregating methods include the weighted sum method, £-constraint method, weighted matric method, and analytical hierarchical process (AHP). In this category, the user assigns the weight to each objective function. Outranking works on the principles of concordance and discordance. It compresses the solutions into a binary outranking relationship and includes elimination and choice expressing reality (ELECTRE), preference ranking organization method for enrichment of evaluation (PROMETHEE), technique for order of preference by similarity to ideal solution (TOPSIS), and Benson’s method. Some of these are described as below:
• Weighted sum method, in which each objective function is mapped with its assigned weight. This strategy has the potential to solve convex problems with the desired Pareto solution. However, it cannot provide a well-distributed set of solutions due to the non-linear relationships between variables and their weights (Delgarm et al., 2016).
• £-constraint method has the potential to generate different Pareto solutions for convex and non-convex types of problems by changing the £ value. It depends on the minimum and maximum values of the individual parameters (Gossard et al., 2013).
• Weighted matrix method is applicable to both convex and non-convex problems. It has special characteristics for solving the conjunctive and reciprocal behaviours of objective functions (Nguyen et al., 2014; Mekhilef et al., 2011; Marler and Arora, 2010; Xu et al., 2020).
• Elimination and choice expressing the reality (ELECTRE-III) was introduced by Roy. It works on the accordance/discordance principle and compares solutions using binary outranking relationships (Marzouk, 2011).
5.3 Robustness/sensitivity analysis
Robustness analysis focuses on the robustness of the optimal Pareto solutions. Sensitivity analysis is used to identify the most influential design parameters affecting the building energy model and behaviour. Methods such as partial rank correlation coefficient (PRCC), standardized rank correlation coefficient (SRRC), and the Morris method are widely used for sensitivity analysis. Uncertainty analysis focuses on identifying and integrating uncertain variables such as environmental conditions, occupant behaviour, envelope, energy price, utility price, and life cycle costs that inherently show an impact on building life cycle performance. Research has found two types of uncertainty: aleatory and epistemic. Aleatory uncertainty, often known as “statistical uncertainty”, refers to the inherent uncertainty caused by probabilistic variability. This aleatory uncertainty is irreducible, and it is typically represented by a probability distribution. Epistemic uncertainty is caused by a lack of knowledge, such as a lack of comprehensive knowledge of the underlying processes, limited knowledge of the phenomena, or an inaccurate assessment of the related parameters and their behaviour. Two approaches are commonly used to analyse uncertainty: probabilistic and non-probabilistic. The probabilistic approach includes methods such as Monte Carlo, adaptive sequential, perturbation, eigenvector dimension reduction, active subspace, and polynomial chaos expansion. The non-probabilistic approach includes methods such as interval analysis, convexity, fuzzy theory, and possibility theory (Tian, 2013; Acar et al., 2021; Pang et al., 2020).
5.4 Net zero approach
This final step illustrates the category of net-zero energy buildings according to balance type grid connectivity.
• If a building’s annual generation is greater than its annual consumption, it falls under net-positive buildings.
• If annual generation is equal to a building’s annual operation energy demand, this is considered a balanced NZEB.
6 Conclusion and future research direction
6.1 Conclusion
This paper demonstrates the potential of a multivariate optimization approach for decision-making and the suitability and usability of the Pareto solution compared to exhaustive NZEB approaches. It identified and synthesized relevant primary case studies, and critically analysed and summarized them in textual and tabular format. This review has focused specifically on climate-adaptive net-zero buildings designed to reduce the global earth temperature, achieve net-zero emissions, and meet 100% of their energy demand with renewable energy by 2050. In several climatic regions of India, there are no guidelines and standards available for net-zero designs in any building typology. This review suggests a multi-objective optimization-based methodological framework for NZEB. The framework contains four hierarchical steps: optimization framework, multi-criteria decision-making, robustness assessment, and net zero approaches. This framework can help designers, stockholders, policymakers, and building industry experts in decision-making in the design, refurbishment, and renovation of high-performance and net-zero energy buildings. This paper summarizes the social, environmental, and economic criteria of building performance objectives and their trade-offs. Furthermore, it identifies and emphasizes passive design, HVAC systems, lighting, and renewable energy parameter accounting as decision variables and illustrates their impact on occupant comfort, building performance, and energy models. Moreover, it suggests that algorithm-coupled MOO can compute various building uncertainties and their impact on building performance objectives.
6.2 Future research direction
This review has certain limitations, and future research is needed. As discussed above, NZEB design is a complex structure that focuses on the integration and optimization of various design and operational strategies. India has a diverse range of climatic boundaries, so it is crucial to optimize uncertain parameters such as weather, occupants, and operations to satisfy building performance objectives. Future research should thus focus on the development of a more robust framework and their experimental validation with actual scenarios to improve reliability.
The proposed framework in this study employed existing algorithms to solve the architectural problem and DM of NZEB design. However, optimizing the large set of design variables, control parameters, and advanced energy systems in real-time, along with evaluating on-site RE generation and storage while reducing grid dependency, is crucial. Hence, a novel hybrid nature-inspired or physics-based metaheuristic optimization algorithm is needed that enables informed decisions for NZEB design. Moreover, the design of an advanced computational package for predictive analytics and the real-time optimization of building energy systems could be beneficial for future NZEB research.
Policy and regulatory frameworks are essential for adopting and implementing NZEB in India. Therefore, an advanced framework along with a user-friendly graphical user interface (GUI) to integrate energy codes and standards is essential to facilitate the architects, designers, and policymakers of NZEB development.
7 Potential challenge and suggestion
In addition to suggesting the NZEB design framework in India, the authors critically analyse the potential challenges of implementing the proposed net-zero framework and highlight the corresponding solutions to address them.
7.1 Financial challenges
The high upfront cost of high-performance building materials, advanced lighting, HVAC systems, and the installation of on-site RE generation and storage systems has a substantial impact on occupants and stakeholders. Additionally, the adoption of the proposed framework for NZEB design in realistic scenarios becomes challenging and expensive due to the longer payback period, high investment costs, poor economy, and market. Thus, government subsidies and incentives can help compensate for the financial burden. Developing cost-effective strategies such as additional floor-to-area ratio (FAR) not only encourages stockholders but also reduces operational cost and energy savings, thus contributing to achieving India’s net zero target.
7.2 Technical challenges
Technical challenges include the lack of advanced building material inventory, efficient technology, and the integration of hybrid energy sources. Additionally, the absence of advanced building simulation tools to perform multivariate optimization, accurate and comprehensive information regarding building energy models, and expertise and specialized skills further complicate the implementation. Thus, enforcing research in the development of advanced optimization computational packages and innovative cutting-edge research in net zero building solutions will ensure the implementation of the NZEB framework. Moreover, conducting a training program and hands-on experience in advanced building tools for architects and designers to build technical proficiency will ensure the integral adoption of NZEB practices.
7.3 Social and institutional challenges
Significant challenges in the widespread adoption of the NZEB framework rise from the deficiency in unified benchmarking criteria aligned with net-zero energy codes and standards, the absence of policies, guidelines, and regulations for NZEB design construction, and the lack of awareness and understanding about net zero among the stakeholders and occupants. Thus, a comprehensive regulatory mechanism, a stringent building energy code, and ensuring their practical implementation and enforcement can lead NZEB development.
7.4 Operational challenges
Ensuring the performance and sustainability of NZEBs in their entire lifespan requires effective monitoring and continuous upgrading of evaluation mechanisms, which are often lacking. Therefore, implementing an advanced monitoring and real-time performance evaluation system for natural resource consumption, generation, and GHG emission can provide valuable data for continuous optimization.
Author contributions
PC: writing–original draft and writing–review and editing. NK: writing–review and editing and writing–original draft. RL: writing–review and editing and writing–original draft.
Funding
The authors declare that no financial support was received for the research, authorship, and/or publication of this article.
Conflict of interest
The authors declare that the research was conducted in the absence of any commercial or financial relationships that could be construed as a potential conflict of interest.
Publisher’s note
All claims expressed in this article are solely those of the authors and do not necessarily represent those of their affiliated organizations, or those of the publisher, the editors, and the reviewers. Any product that may be evaluated in this article, or claim that may be made by its manufacturer, is not guaranteed or endorsed by the publisher.
Supplementary material
The Supplementary Material for this article can be found online at: https://www.frontiersin.org/articles/10.3389/fenef.2024.1430647/full#supplementary-material
References
Abdou, N., el Mghouchi, Y., Hamdaoui, S., el Asri, N., and Mouqallid, M. (2021). Multi-objective optimization of passive energy efficiency measures for net-zero energy building in Morocco. Build. Environ. 204, 108141. doi:10.1016/j.buildenv.2021.108141
Acar, E., Bayrak, G., Jung, Y., Lee, I., Ramu, P., and Ravichandran, S. S. (2021). Modeling, analysis, and optimization under uncertainties: a review. Struct. Multidiscip. Optim. 64 (5), 2909–2945. doi:10.1007/s00158-021-03026-7
Aggarwal, V., Meena, C. S., Kumar, A., Alam, T., Kumar, A., Ghosh, A., et al. (2020). Potential and future prospects of geothermal energy in space conditioning of buildings: India and worldwide review. Sustainability 12 (20), 1–19. doi:10.3390/su12208428
Alkhateeb, E., and Abu-Hijleh, B. (2019). Potential for retrofitting a federal building in the UAE to net zero electricity building (nZEB). Heliyon 5 (6), e01971. doi:10.1016/j.heliyon.2019.e01971
Ascione, F., Bianco, N., De Masi, R. F., Mauro, G. M., and Vanoli, G. P. (2015). Design of the building envelope: a novel multi-objective approach for the optimization of energy performance and thermal comfort. Sustainability 7 (8), 10809–10836. doi:10.3390/su70810809
Ascione, F., Bianco, N., Mauro, G. M., and Vanoli, G. P. (2019). A new comprehensive framework for the multi-objective optimization of building energy design: Harlequin. Appl. Energy 241, 331–361. doi:10.1016/j.apenergy.2019.03.028
ASHRAE (2008). ASHRAE vision 2020 producing net zero energy buildings. Atlanta: American Society of Heating, Refrigerating, and Air-Conditioning Engineers.
Bureau of energy efficiency impact of energy efficiency Measures a report on. Available at: www.beeindia.gov.
Bai, Q. (2010). Analysis of particle swarm optimization algorithm. Comput. Inf. Sci. 3 (1), 180. doi:10.5539/cis.v3n1p180
Bamdad, K., Cholette, M. E., Guan, L., and Bell, J. (2018). Building energy optimisation under uncertainty using ACOMV algorithm. Energy Build. 167, 322–333. doi:10.1016/j.enbuild.2018.02.053
Bandyopadhyay, S., and Pal, S. K. (2007). Classification and learning using genetic algorithms: applications in bioinformatics and web intelligence. Springer Science and Business Media.
Bano, F., and Sehgal, V. (2019). Finding the gaps and methodology of passive features of building envelope optimization and its requirement for office buildings in India. Therm. Sci. Eng. Prog. 9, 66–93. doi:10.1016/j.tsep.2018.11.004
Barber, K. A., and Krarti, M. (2022). A review of optimization based tools for design and control of building energy systems. Renew. Sustain. Energy Rev. 160, 112359. doi:10.1016/j.rser.2022.112359
basic energy plan of Japan (2014). Available at: http://www.meti.go.jp/english/press/2014/0411_02.html October 1, 2018).
Benincá, L., Crespo Sánchez, E., Passuello, A., Karini Leitzke, R., Grala da Cunha, E., and Maria González Barroso, J. (2023). Multi-objective optimization of the solar orientation of two residential multifamily buildings in south Brazil. Energy Build. 285, 112838. doi:10.1016/j.enbuild.2023.112838
Besbas, S., Nocera, F., Zemmouri, N., Khadraoui, M. A., and Besbas, A. (2022). Parametric-based multi-objective optimization workflow: daylight and energy performance study of hospital building in Algeria. Sustainability 14 (19), 12652. doi:10.3390/su141912652
Bhandari, N., and Sundaram, A. M. (2019). “Optimization of windows for daylighting and energy consumption for south facade in office building in hot and dry climate of India,” in Smart innovation, systems and technologies (Springer Science and Business Media Deutschland GmbH), 307–320.
Bingham, R. D., Agelin-Chaab, M., and Rosen, M. A. (2019). Whole building optimization of a residential home with PV and battery storage in the Bahamas. Renew. Energy 132, 1088–1103. doi:10.1016/j.renene.2018.08.034
Bui, D. K., Nguyen, T. N., Ghazlan, A., Ngo, N. T., and Ngo, T. D. (2020). Enhancing building energy efficiency by adaptive façade: a computational optimization approach. Appl. Energy, 265. doi:10.1016/j.apenergy.2020.114797
bureau of energy efficiency NZEB. Available at: https://nzeb.in/.
Cabeza, L. F., and Chàfer, M. (2020). Technological options and strategies towards zero energy buildings contributing to climate change mitigation: a systematic review. Energy Build. 219, 110009. doi:10.1016/j.enbuild.2020.110009
Carlucci, S., Cattarin, G., Causone, F., and Pagliano, L. (2015). Multi-objective optimization of a nearly zero-energy building based on thermal and visual discomfort minimization using a non-dominated sorting genetic algorithm (NSGA-II). Energy Build. 104, 378–394. doi:10.1016/j.enbuild.2015.06.064
Chaturvedi, P. K., Kumar, N., and Lamba, R. (2023). “Multi-objective optimization-based methodological framework for net zero energy building design in India. Artif. Intell. Blockchain, Comput. Secur. Volume 1 1, 187–194. doi:10.1201/9781003393580-29
Chaturvedi, P. K., Kumar, N., and Lamba, R. (2024a). Finding the gaps in design strategies and technological advancements for net-zero energy buildings development in India. Energy and Environ., 0958305X241256039. doi:10.1177/0958305x241256039
Chaturvedi, P. K., Kumar, N., and Lamba, R. (2024b). Multi-objective optimization for visual, thermal, and cooling energy performance of building envelope design in the composite climate of Jaipur (India). Energy and Environ., 0958305X241228513. doi:10.1177/0958305x241228513
Chaturvedi, S., and Elangovan, R. (2023). Grey Wolf algorithmic framework for building energy optimization in India's Köppen-Geiger climatic zones. Adv. Build. Energy Res. 17 (3), 277–302. doi:10.1080/17512549.2023.2184422
Chaturvedi, S., Rajasekar, E., and Natarajan, S. (2020). Multi-objective building design optimization under operational uncertainties using the NSGA II algorithm. Buildings 10 (5), 88. doi:10.3390/buildings10050088
Cheung, C. M., and Lee, M. K. (2012). What drives consumers to spread electronic word of mouth in online consumer-opinion platforms. Decis. support Syst. 53 (1), 218–225. doi:10.1016/j.dss.2012.01.015
Chiesa, G., Acquaviva, A., Grosso, M., Bottaccioli, L., Floridia, M., Pristeri, E., et al. (2019). Parametric optimization of window-to-wall ratio for passive buildings adopting a scripting methodology to dynamic-energy simulation. Sustainability 11 (11), 3078. doi:10.3390/su11113078
Costa-Carrapiço, I., Raslan, R., and González, J. N. (2020). A systematic review of genetic algorithm-based multi-objective optimisation for building retrofitting strategies towards energy efficiency. Energy Build. 210, 109690. doi:10.1016/j.enbuild.2019.109690
Crawley, D. B., Hand, J. W., Kummert, M., and Griffith, B. T. (2008). Contrasting the capabilities of building energy performance simulation programs. Build. Environ. 43 (4), 661–673. doi:10.1016/j.buildenv.2006.10.027
D’Agostino, D., Parker, D., and Melià, P. (2019). Environmental and economic implications of energy efficiency in new residential buildings: a multi-criteria selection approach. Energy Strategy Rev. 26, 100412. doi:10.1016/j.esr.2019.100412
Dalal-Kulkarni, A. P., and Gokhale, V. Applicability of net zero energy building (nzeb) concept in the residential sector in India. 2011.
Deb, K., Pratap, A., Agarwal, S., and Meyarivan, T. A. (2002). A fast and elitist multiobjective genetic algorithm: NSGA-II. IEEE Trans. Evol. Comput. 6 (2), 182–197. doi:10.1109/4235.996017
Delgarm, N., Sajadi, B., Kowsary, F., and Delgarm, S. (2016). Multi-objective optimization of the building energy performance: a simulation-based approach by means of particle swarm optimization (PSO). Appl. energy 170, 293–303. doi:10.1016/j.apenergy.2016.02.141
Dino, I. G., and Üçoluk, G. (2017). Multiobjective design optimization of building space layout, energy, and daylighting performance. J. Comput. Civ. Eng. 31 (5), 04017025. doi:10.1061/(asce)cp.1943-5487.0000669
Energy Step Code Council BC Energy Step Code (2017). 2010 on the energy performance of buildings (recast). Official J. Eur. Union. Available at: https://energystepcode.ca/.
Escandón, R., Ascione, F., Bianco, N., Mauro, G. M., Suárez, R., and Sendra, J. J. (2019). Thermal comfort prediction in a building category: artificial neural network generation from calibrated models for a social housing stock in southern Europe. Appl. Therm. Eng. 150, 492–505. doi:10.1016/j.applthermaleng.2019.01.013
Evins, R. (2013). A review of computational optimisation methods applied to sustainable building design. Renew. Sustain. energy Rev. 22, 230–245. doi:10.1016/j.rser.2013.02.004
Fancello, G., Carta, M., and Fadda, P. (2014). A decision support system based on Electre III for safety analysis in a suburban road network. Transp. Res. Procedia 3, 175–184. doi:10.1016/j.trpro.2014.10.103
Fang, Y., and Cho, S. (2019). Design optimization of building geometry and fenestration for daylighting and energy performance. Sol. Energy 191, 7–18. doi:10.1016/j.solener.2019.08.039
Feng, W., Zhang, Q., Ji, H., Wang, R., Zhou, N., Ye, Q., et al. (2019). A review of net zero energy buildings in hot and humid climates: experience learned from 34 case study buildings. Renew. Sustain. Energy Rev., 114. doi:10.1016/j.rser.2019.109303
Ferrara, M., Sirombo, E., and Fabrizio, E. (2018). Automated optimization for the integrated design process: the energy, thermal and visual comfort nexus. Energy Build. 168, 413–427. doi:10.1016/j.enbuild.2018.03.039
Gagnon, R., Gosselin, L., and Armand Decker, S. (2019). Performance of a sequential versus holistic building design approach using multi-objective optimization. J. Build. Eng., 26. doi:10.1016/j.jobe.2019.100883
Giouri, E. D., Tenpierik, M., and Turrin, M. (2020). Zero energy potential of a high-rise office building in a Mediterranean climate: using multi-objective optimization to understand the impact of design decisions towards zero-energy high-rise buildings. Energy Build. 209, 109666. doi:10.1016/j.enbuild.2019.109666
Gossard, D., Lartigue, B., and Thellier, F. (2013). Multi-objective optimization of a building envelope for thermal performance using genetic algorithms and artificial neural network. Energy Build. 67, 253–260. doi:10.1016/j.enbuild.2013.08.026
Gupta, G., Mathur, S., Mathur, J., and Nayak, B. K. (2023). Comparison of energy-efficiency benchmarking methodologies for residential buildings. Energy Build. 285, 112920. doi:10.1016/j.enbuild.2023.112920
Hajela, P., and Lin, C. Y. (1992). Genetic search strategies in multicriterion optimal design. Struct. Optim. 4 (2), 99–107. doi:10.1007/bf01759923
Hamdy, M., Hasan, A., and Siren, K. (2013). A multi-stage optimization method for cost-optimal and nearly-zero-energy building solutions in line with the EPBD-recast 2010. Energy Build. 56, 189–203. doi:10.1016/j.enbuild.2012.08.023
Harish, V. S., and Kumar, A. (2016). A review on modeling and simulation of building energy systems. Renew. Sustain. energy Rev. 56, 1272–1292. doi:10.1016/j.rser.2015.12.040
Harkouss, F., Fardoun, F., and Biwole, P. H. (2018a). Optimization approaches and climates investigations in NZEB—a review. Build. Simul. 11 (5), 923–952. doi:10.1007/s12273-018-0448-6
Harkouss, F., Fardoun, F., and Biwole, P. H. (2018b). Multi-objective optimization methodology for net zero energy buildings. J. Build. Eng. 16, 57–71. doi:10.1016/j.jobe.2017.12.003
Harkouss, F., Fardoun, F., and Biwole, P. H. (2018c). Passive design optimization of low energy buildings in different climates. Energy 165, 591–613. doi:10.1016/j.energy.2018.09.019
Holland, J. H. (1992). control, and artificial intelligence. MIT press.Adaptation in natural and artificial systems: an introductory analysis with applications to biology.
Hong, T., Langevin, J., and Sun, K. (2018). Building simulation: ten challenges. Build. Simul. 11 (5), 871–898. doi:10.1007/s12273-018-0444-x
Hu, M., and Qiu, Y. (2019). A comparison of building energy codes and policies in the USA, Germany, and China: progress toward the net-zero building goal in three countries. Clean Technol. Environ. Policy 21 (2), 291–305. doi:10.1007/s10098-018-1636-x
IGBC NetZero Energy Buildings (2021). Igbc. Available at: https://igbc.in/igbc/redirectHtml.htm?redVal=showNetZeroEnergyBuildingsNosignin.
India cooling action plan operationalizing space cooling recommendations Ministry of Environment. New Delhi, India: Forest and Climate Change Government of India.
Jain, M., and Rawal, R. (2022). Emissions from a net-zero building in India: life cycle assessment. Build. Cities 3 (1), 398. doi:10.5334/bc.194Available at: https://journal-buildingscities.org/article/10.5334/bc.194/.
Jeong, K., Hong, T., Kim, J., and Cho, K. (2019). Development of a multi-objective optimization model for determining the optimal CO2 emissions reduction strategies for a multi-family housing complex. Renew. Sustain. Energy Rev. 110, 118–131. doi:10.1016/j.rser.2019.04.068
Jung, Y., Heo, Y., and Lee, H. (2021). Multi-objective optimization of the multi-story residential building with passive design strategy in South Korea. Build. Environ., 203. doi:10.1016/j.buildenv.2021.108061
Kamazani, M. A., and Dixit, M. K. (2023). Multi-objective optimization of embodied and operational energy and carbon emission of a building envelope. J. Clean. Prod. 428, 139510. doi:10.1016/j.jclepro.2023.139510
Kang, S., Yong, S. gil, Kim, J., Jeon, H., Cho, H., and Koo, J. (2018). Automated processes of estimating the heating and cooling load for building envelope design optimization. Build. Simul. 11 (2), 219–233. doi:10.1007/s12273-017-0389-5
Kapoor, R., Deshmukh, A., and Lal, S. Strategy roadmap for net zero energy buildings in India. 2011.
Khosla, R., and Janda, K. B. (2019). India’s building stock: towards energy and climate change solutions. Build. Res. and Inf. 47 (1), 1–7. doi:10.1080/09613218.2019.1522482
Khosla, R., Sircar, N., and Bhardwaj, A. (2019). Energy demand transitions and climate mitigation in low-income urban households in India. Environ. Res. Lett. 14 (9), 095008. doi:10.1088/1748-9326/ab3760
Kishore, N. (2022). Impact of climate change on future bioclimatic potential and residential building thermal and energy performance in India. Indoor Built Environ. 31 (2), 329–354. doi:10.1177/1420326x21993919
Lan, L., Wood, K. L., and Yuen, C. (2019). A holistic design approach for residential net-zero energy buildings: a case study in Singapore. Sustain. Cities Soc., 50. doi:10.1016/j.scs.2019.101672
Lin, Y., Mojtabai, R., Goes, F. S., Zandi, P. P., and Li, C. Q. 2020. Trends in prescriptions of lithium and other medications for patients with bipolar disorder in office-based practices in the United States, J. Affect. Disord. 276, 883, 889. doi:10.1016/j.jad.2020.07.063
Loonen, R. C., Favoino, F., Hensen, J. L., and Overend, M. (2017). Review of current status, requirements and opportunities for building performance simulation of adaptive facades. J. Build. Perform. Simul. 10 (2), 205–223. doi:10.1080/19401493.2016.1152303
Lu, M., Sun, Y., and Ma, Z. (2024). Multi-objective design optimization of multiple energy systems in net/nearly zero energy buildings under uncertainty correlations. Appl. Energy 370, 123620. doi:10.1016/j.apenergy.2024.123620
Luo, X., Zhang, Y., Lu, J., and Ge, J. (2024). Multi-objective optimization of the office park building envelope with the goal of nearly zero energy consumption. J. Build. Eng. 84, 108552. doi:10.1016/j.jobe.2024.108552
Lützkendorf, T., and Frischknecht, R. (2020). (Net-) zero-emission buildings: a typology of terms and definitions. Build. Cities 1 (1), 662–675. doi:10.5334/bc.66
Machairas, V., Tsangrassoulis, A., and Axarli, K. (2014). Algorithms for optimization of building design: a review. Renew. Sustain. energy Rev. 31, 101–112. doi:10.1016/j.rser.2013.11.036
Mahmoud, T., and Ahmed, B. S. (2015). An efficient strategy for covering array construction with fuzzy logic-based adaptive swarm optimization for software testing use. Expert Syst. Appl. 42 (22), 8753–8765. doi:10.1016/j.eswa.2015.07.029
Marler, R. T., and Arora, J. S. (2010). The weighted sum method for multi-objective optimization: new insights. Struct. Multidiscip. Optim. 41 (6), 853–862. doi:10.1007/s00158-009-0460-7
Marzouk, M. M. (2011). ELECTRE III model for value engineering applications. Automation Constr. 20 (5), 596–600. doi:10.1016/j.autcon.2010.11.026
Mekhilef, S., Saidur, R., and Safari, A. (2011). A review on solar energy use in industries. Renew. Sustain. energy Rev. 15 (4), 1777–1790. doi:10.1016/j.rser.2010.12.018
Moghaddasi, H., Culp, C., and Vanegas, J. (2021a). Net zero energy communities: integrated power system, building and transport sectors. Energies 14 (21), 7065. doi:10.3390/en14217065
Moghaddasi, H., Culp, C., Vanegas, J., and Ehsani, M. (2021b) Net zero energy buildings: variations, clarifications, and requirements in response to the paris agreement. Energies, 14, 3760, doi:10.3390/en14133760
Net Zero by 2050 A roadmap for the global energy sector net zero by 2050 interactive iea.li/nzeroadmap net zero by 2050 data iea.li/nzedata. 2021.
Nguyen, A. T., Reiter, S., and Rigo, P. (2014). A review on simulation-based optimization methods applied to building performance analysis. Appl. Energy 113, 1043–1058. doi:10.1016/j.apenergy.2013.08.061
Omrany, H., Chang, R., Soebarto, V., Zhang, Y., Ghaffarianhoseini, A., and Zuo, J. (2022). A bibliometric review of net zero energy building research 1995–2022. Energy Build. 262, 111996. doi:10.1016/j.enbuild.2022.111996
Pang, Z., O'Neill, Z., Li, Y., and Niu, F. (2020). The role of sensitivity analysis in the building performance analysis: a critical review. Energy Build. 209, 109659. doi:10.1016/j.enbuild.2019.109659
Pernodet, F., Lahmidi, H., and Michel, P. (2009). Use of genetic algorithms for multicriteria optimization of building refurbishment. In11th Int. IBPSA Conf., 27–30.
Piderit, M. B., Vivanco, F., Van Moeseke, G., and Attia, S. (2019). Net zero buildings—a framework for an integrated policy in Chile. Sustainability 11 (5), 1494. doi:10.3390/su11051494
Pless, S., and Torcellini, P. (2010). Net-zero energy buildings: a classification system based on renewable energy suppy options. Available at: http://www.osti.gov/bridge.
Rabani, M., Bayera Madessa, H., Mohseni, O., and Nord, N. (2020). Minimizing delivered energy and life cycle cost using Graphical script: an office building retrofitting case. Appl. Energy, 268. doi:10.1016/j.apenergy.2020.114929
Raj, B. P., Meena, C. S., Agarwal, N., Saini, L., Hussain Khahro, S., Subramaniam, U., et al. (2021). A review on numerical approach to achieve building energy efficiency for energy, economy and environment (3E) benefit. Energies 14 (15), 4487. doi:10.3390/en14154487
Rezaee, R., Vakilinezhad, R., and Haymaker, J. (2021). Parametric framework for a feasibility study of zero-energy residential buildings for the design stage. J. Build. Eng., 35. doi:10.1016/j.jobe.2020.101960
Saini, L., Meena, C. S., Raj, B. P., Agarwal, N., and Kumar, A. (2022). Net Zero Energy Consumption building in India: an overview and initiative toward sustainable future. Int. J. Green Energy 19 (5), 544–561. doi:10.1080/15435075.2021.1948417
Salata, F., Ciancio, V., Dell’Olmo, J., Golasi, I., Palusci, O., and Coppi, M. (2020). Effects of local conditions on the multi-variable and multi-objective energy optimization of residential buildings using genetic algorithms. Appl. Energy 260, 114289. doi:10.1016/j.apenergy.2019.114289
Sartori, I., Napolitano, A., and Voss, K. (2012). Net zero energy buildings: a consistent definition framework. Energy Build. 48, 220–232. doi:10.1016/j.enbuild.2012.01.032
Satola, D., Balouktsi, M., Lützkendorf, T., Wiberg, A. H., and Gustavsen, A. (2021). How to define (net) zero greenhouse gas emissions buildings: the results of an international survey as part of IEA EBC annex 72. Build. Environ. 192, 107619. doi:10.1016/j.buildenv.2021.107619
Sen, R., Bhattacharya, S. P., and Chattopadhyay, S. (2021). Are low-income mass housing envelops energy efficient and comfortable? A multi-objective evaluation in warm-humid climate. Energy Build. 245, 111055. doi:10.1016/j.enbuild.2021.111055
Slaper, T. F., and Hall, T. J. (2011). The triple bottom line: what is it and how does it work. Indiana Bus. Rev. 86 (1), 4–8.
Sola, A., Corchero, C., Salom, J., and Sanmarti, M. (2018). Simulation tools to build urban-scale energy models: a review. Energies 11 (12), 3269. doi:10.3390/en11123269
Sudhakar, K., Winderl, M., and Priya, S. S. (2019). Net-zero building designs in hot and humid climates: a state-of-art. Case Stud. Therm. Eng. 13, 100400. doi:10.1016/j.csite.2019.100400
Summary for Policymakers (2018). IPCC. Geneva, Switzerland: World Meteorological Organization, 1–24. Available at: https://www.ipcc.ch/sr15/chapter/spm/.
Sun, C., Liu, Q., and Han, Y. (2020). Many-objective optimization design of a public building for energy, daylighting and cost performance improvement. Appl. Sci. 10 (7), 2435. doi:10.3390/app10072435
The Future of Cooling (2018). The future of cooling opportunities for Energy- Efficient air conditioning. Organization for Economic Co-operation and Development. Int. Energy Agency, 1–92. doi:10.1787/9789264301993-en
Tian, W. (2013). A review of sensitivity analysis methods in building energy analysis. Renew. Sustain. energy Rev. 20, 411–419. doi:10.1016/j.rser.2012.12.014
Torcellini, P., Pless, S., Deru, M., and Crawley, D. (2006). Zero energy buildings: a critical look at the definition. United State: Preprint. Available at: http://www.osti.gov/bridge
Toutou, A., Fikry, M., and Mohamed, W. (2018). The parametric based optimization framework daylighting and energy performance in residential buildings in hot arid zone. Alexandria Eng. J. 57 (4), 3595–3608. doi:10.1016/j.aej.2018.04.006
Ürge-Vorsatz, D., Khosla, R., Bernhardt, R., Chan, Y. C., Vérez, D., Hu, S., et al. Annual review of environment and resources advances toward a net-zero global building sector. 2020. doi:10.1146/annurev-environ-012420-
Wang, B., Xia, X., and Zhang, J. (2014). A multi-objective optimization model for the life-cycle cost analysis and retrofitting planning of buildings. Energy Build. 77, 227–235. doi:10.1016/j.enbuild.2014.03.025
Wang, Y., Jiang, Z., Li, L., Qi, Y., Sun, J., and Jiang, Z. (2023). A bibliometric and content review of carbon emission analysis for building construction. Buildings 13 (1), 205. doi:10.3390/buildings13010205
Wilberforce, T., Olabi, A. G., Sayed, E. T., Elsaid, K., Maghrabie, H. M., and Abdelkareem, M. A. (2023). A review on zero energy buildings–Pros and cons. Energy Built Environ. 4 (1), 25–38. doi:10.1016/j.enbenv.2021.06.002
Williams, J., Mitchell, R., Raicic, V., Vellei, M., Mustard, G., Wismayer, A., et al. (2016). Less is more: a review of low energy standards and the urgent need for an international universal zero energy standard. J. Build. Eng. 6, 65–74. doi:10.1016/j.jobe.2016.02.007
Wu, W., and Skye, H. M. (2021). Residential net-zero energy buildings: review and perspective. Renew. Sustain. Energy Rev. 142, 110859. doi:10.1016/j.rser.2021.110859
Xu, Y., Yan, C., Liu, H., Wang, J., Yang, Z., and Jiang, Y. (2020). Smart energy systems: a critical review on design and operation optimization. Sustain. Cities Soc. 62, 102369. doi:10.1016/j.scs.2020.102369
Xu, Y., Zhang, G., Yan, C., Wang, G., Jiang, Y., and Zhao, K. (2021). A two-stage multi-objective optimization method for envelope and energy generation systems of primary and secondary school teaching buildings in China. Build. Environ., 204. doi:10.1016/j.buildenv.2021.108142
Xue, Q., Wang, Z., and Chen, Q. (2022). Multi-objective optimization of building design for life cycle cost and CO2 emissions: a case study of a low-energy residential building in a severe cold climate. Build. Simul. 15 (1), 83–98. doi:10.1007/s12273-021-0796-5
Xue, Y., and Liu, W. (2023). A study on the optimization of atrium daylight and energy performance through skylight and shading design in commercial buildings in cold zones. Buildings 13 (1), 228. doi:10.3390/buildings13010228
Yan, G., and Li, C. (2011). An effective refinement artificial bee colony optimization algorithm based on chaotic search and application for pid control tuning. J. Comput. Inf. Syst. 7 (9), 3309–3316.
Yao, S., Jiang, Z., Yuan, J., Wang, Z., and Huang, L. (2022). Multi-objective optimization of transparent building envelope of rural residences in cold climate zone, China. Case Stud. Therm. Eng. 34, 102052. doi:10.1016/j.csite.2022.102052
Zahra Benaddi, F., Boukhattem, L., and Cesar Tabares-Velasco, P. (2024). Multi-objective optimization of building envelope components based on economic, environmental, and thermal comfort criteria. Energy Build. 305, 113909. doi:10.1016/j.enbuild.2024.113909
Zhang, Y., Bai, X., Mills, F. P., and Pezzey, J. C. (2018). Rethinking the role of occupant behavior in building energy performance: a review. Energy Build. 172, 279–294. doi:10.1016/j.enbuild.2018.05.017
Keywords: net zero energy building, multi-objective optimization, climate change, sustainability, building energy simulation
Citation: Chaturvedi PK, Kumar N and Lamba R (2024) Identification of climate adaptive multivariate optimization methodologies for the design of NZEB in India: a holistic review. Front. Energy Effic. 2:1430647. doi: 10.3389/fenef.2024.1430647
Received: 10 May 2024; Accepted: 23 August 2024;
Published: 13 September 2024.
Edited by:
Lurui Fang, Xi’an Jiaotong-Liverpool University, ChinaReviewed by:
Shahryar Habibi, University of Ferrara, ItalyLuca Evangelisti, Roma Tre University, Italy
Hasim Altan, Prince Mohammad bin Fahd University, Saudi Arabia
Copyright © 2024 Chaturvedi, Kumar and Lamba. This is an open-access article distributed under the terms of the Creative Commons Attribution License (CC BY). The use, distribution or reproduction in other forums is permitted, provided the original author(s) and the copyright owner(s) are credited and that the original publication in this journal is cited, in accordance with accepted academic practice. No use, distribution or reproduction is permitted which does not comply with these terms.
*Correspondence: Pushpendra Kr. Chaturvedi, pkchaturvedi8561@gmail.com