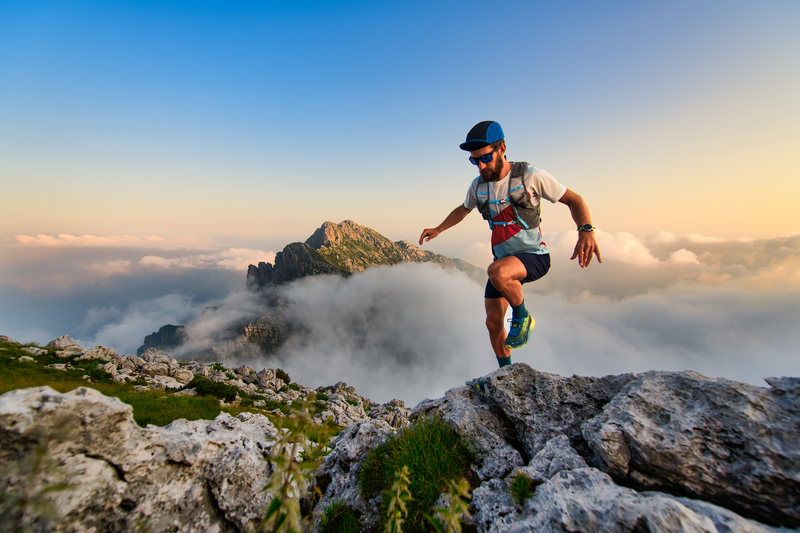
95% of researchers rate our articles as excellent or good
Learn more about the work of our research integrity team to safeguard the quality of each article we publish.
Find out more
ORIGINAL RESEARCH article
Front. Endocrinol. , 25 February 2025
Sec. Cardiovascular Endocrinology
Volume 16 - 2025 | https://doi.org/10.3389/fendo.2025.1532344
This article is part of the Research Topic Re-visiting Risk Factors for Cardiometabolic Diseases: Towards a New Epidemiological Frontier View all 25 articles
Objective: The body roundness index (BRI) and circadian syndrome (CircS) are considered new risk factors for cardiovascular disease (CVD), yet it remains uncertain whether elevated BRI is associated with CVD incidence in CircS patients. In this study, we investigated the association between BRI and CVD occurrence among CircS participants.
Methods: We conducted a retrospective cohort study involving 8,888 participants aged ≥45 years from the China Health and Retirement Longitudinal Study (CHARLS 2011-2020 wave). CircS was evaluated with a combination of the International Diabetes Federation (IDF) MetS, along with short sleep duration and depression. The threshold for CircS was established at ≥4. In the first phase, the receiver operating characteristic (ROC) curves were used to evaluate the accuracy of diagnosing CircS according to baseline BRI. During the 9-year follow-up, the associations between BRI and CVD incidence in CircS patients were explored by employing logistic regression, restricted cubic spline (RCS) analysis, and subgroup analysis.
Results: BRI demonstrated an independent association with CircS, and multivariable-adjusted restricted cubic spline analyses suggested “J-shaped” associations between BRI and risk of CircS. BRI demonstrated better diagnostic performance in diagnosing CircS compared to general obesity indices such as ABSI (AUC: 0.617), BMI (AUC: 0.746), and WC (AUC: 0.722), with an AUC of 0.760. After a 9-year follow-up, BRI was found to be independently associated with the occurrence of CVD in CircS patients, and the associations between incident CVD and the second, third, and fourth BRI quartiles were 1.30 (95% CI: 0.99~1.69), 1.32 (95% CI: 1.01~1.72), and 1.59 (95% CI: 1.21~2.08), respectively, relative to the first BRI quartile. Then, we assessed the relationship between other obesity indices and the CVD occurrence, and likewise observed a significant effect in the fourth quartile.
Conclusion: BRI was independently associated with CircS, outperforming obesity indices such as BMI and WC in identifying individuals with CircS. During the 9-year follow-up, elevated BRI levels was significantly associated with CVD incidence among CircS patients, especially in men. Thus, early identification of high-risk populations with CircS and elevated BRI levels may help promote healthy aging among middle-aged and elderly individuals.
Cardiovascular disease (CVD) remains a major worldwide public health concern and leading contributor to non-communicable disease-related mortality, imposing a growing economic strain on both individuals and society (1, 2). In 2020, CVD surpassed cancer and other diseases to rank first in both incidence and mortality rates among residents of China (3). Early intervention in controlling risk factors is essential for preventing its occurrence.
The concept of circadian syndrome (CircS) has emerged to describe the relationship between circadian disruptions and various diseases, including obesity, dyslipidemia, hypertension, type 2 diabetes, sleep disorders, depression and non-alcoholic fatty liver disease (4). As living conditions have generally increased in China, the prevalence of CircS is now approaching 40%. As such, in recent years there has been an increasing focus on CircS due to its link with CVD (5). Thus, identifying specific risk factors for CVD among CircS individuals may contribute to reducing the future burden of CVD.
Adipose tissue regulates lipid storage and energy balance while serving as a vital modulator of metabolic health, facilitating bidirectional communication with other organs, including the cardiovascular system (6). Adipose tissue can be classified into either visceral fat tissue (VAT) or subcutaneous fat tissue (SAT) based on its anatomical location. Previous studies have demonstrated a significant association between visceral obesity and elevated cardiometabolic risk (7–9). However, whether elevated visceral adiposity in CircS patients is associated with increased risk of CVD remains poorly understood.
Magnetic resonance imaging (MRI) and computed tomography (CT) are widely regarded as the gold standards for assessing body fat and visceral fat tissue. However, their high costs prevent them from being used in routine clinical screening (10). The demand for a dependable and cost-effective measure of visceral adiposity has resulted in the creation of new indices that integrate anthropometric and biochemical evaluations: e.g., Thomas et al. introduced an innovative metric called the Body Roundness Index (BRI), which estimates body fat and visceral adipose tissue volume using waist circumference and height as inputs (11).
Recent research indicates a significant association between BRI and the risk of CVD (12–15). However, the relationship between BRI and CircS has yet to be explored, nor has the relationship between BRI and CVD in patients with CircS. To resolve this dearth in the literature, in the present study, we first investigate the relationship between BRI and CircS. Then, we evaluate the relationship between BRI and CVD among Chinese participants with CircS, focusing on middle-aged and elderly participants from the China Health and Retirement Longitudinal Study (CHARLS).
The China Health and Retirement Longitudinal Study (CHARLS), initiated in 2011, is a nationally representative cohort study that tracks individuals aged 45 and older from more than 20 provinces in China. Using a probability-proportional-to-size (PPS) sampling technique, the baseline survey involved over 17,000 participants from nearly 10,000 households in 450 villages and 150 counties. Participants were regularly followed up every two years through face-to-face interviews performed by the interviewer (using a computer to administer and record responses to survey questions). The follow-up survey waves were performed in 2013, 2015, 2018, and 2020. Conducted biennially by Peking University’s National School of Development, CHARLS adheres to strict ethical standards, including approval from Peking University’s Ethical Review Committee (IRB00001052-11015) and compliance with the Helsinki Declaration. The study provides critical insight into China’s aging population, with detailed methodological descriptions available in a prior publication (16).
The present study was conducted based on data from five waves (2011, 2013, 2015, 2018 and 2020) in CHARLS. The inclusion criteria for this study were: 1) individuals aged 45 years or older in CHARLS 2011; and 2) who had data regarding circadian syndrome status. The exclusion criteria included: 1) absence of data about cardiovascular disease status at follow-up; 2) lack of age information; 3) absence of sex information; 4) unavailability of height, weight, waist circumference (WC) or adiposity index data. Ultimately, we included 8,888 individuals (3,584 of whom had been diagnosed with CircS) from the 2011 cross-sectional study to determine the relationship between BRI and CircS. After 9 years of follow-up, 2,322 CircS participants were included in a longitudinal cohort study using data from 2011 to 2020 to further explore the relationship between BRI and CVD incidence, while 783 participants moved or were otherwise lost to follow up, and 479 participants were excluded because of CVD at baseline or missing data through 2020. Figure 1 illustrates the comprehensive selection procedure.
At baseline, trained interviewers collected data on characteristics by employing a predefined questionnaire. Socio-demographic variables included age, sex, education, marital status, and residence. Health-related factors included body mass index (BMI), smoking and drinking status (current, former or never), self-reported physician-diagnosed dyslipidemia, hypertension, diabetes or high blood glucose, cardiovascular disease and chronic kidney disease.
Physical examination data were also recorded, including WC, weight, height, systolic blood pressure (SBP), and diastolic blood pressure (DBP). Additionally, biochemical indicators were documented, involving fasting blood glucose, glycosylated hemoglobin, total cholesterol, triglycerides, high-density lipoprotein cholesterol, low-density lipoprotein cholesterol, and hypersensitive C-reactive protein levels. Participants were classified as “physically active” if they engaged in moderate activity for at least 30 minutes five times a week or vigorous activity for at least 20 minutes three times a week; the others were classified as “physically inactive” (17). Participants were given the instructions to fast 8–12 hours before blood sampling. Bioassays were conducted at national or local Centers for Disease Control that complied with standardized quality control standards, while blood samples were preserved at −70°C. Finally, night-time sleep duration and daytime napping were measured, and the Center for Epidemiologic Studies-Depression scale (CES-D) score was determined.
The CircS criteria, established and verified in CHARLS, encompassed abdominal obesity (WC ≥ 85 cm in men, and ≥ 80 cm in women), hypertension (SBP ≥ 130 mmHg and/or DBP ≥ 85 mmHg, or drug treatment for hypertension), hyperglycemia (≥100 mg/dl or drug treatment for elevated glucose), high triglycerides (≥150 mg/dl or drug treatment for high triglycerides), low HDL cholesterol (<40 mg/dl or drug treatment for low HDL cholesterol), depression and short sleep duration (<6 h/day) (5, 18, 19). CircS was diagnosed in participants who satisfied ≥ 4 of these criteria.
WC was obtained at the umbilicus level with participants in a standing position. Blood pressure was measured three times, using the SBP and DBP averages (5). Symptoms of depression were evaluated using the 10-item CES-D scale, with scores ≥10 indicating depressive symptoms (20). Total sleep duration, calculated by summing nap and nighttime sleep durations from a questionnaire, was considered short if it was less than 6 hours per day (19). CircS was assessed based on seven components—the 5 components used to define MetS as well as short sleep duration and depression (5, 19, 21).
Any values for WC, weight and height that were less than the 1% percentile cut-off were replaced with 1% percentile values to avoid the influence of outliers. Lacking uniform classification criteria, we divided the BRI into quartiles. We used the following formulas to measure the adiposity indices.
The CVD included heart disease and stroke. Similar to previous studies (18, 22), newly diagnosed CVD was assessed through responses to the following questionnaire item: “Have you been diagnosed with heart attack, coronary heart disease, angina, congestive heart failure, other heart problems, or stroke by a doctor?”
All normally distributed continuous variables were reported as mean ± SD, and skewed continuous variables were presented as median and interquartile range. Categorical variables were presented as frequencies (%). Participants without CircS were compared to those with CircS based on the baseline characteristics. In addition, we categorized patients into quartiles according the baseline BRI levels in order to illustrate CircS patients’ baseline characteristics. We used Chi-square or Fisher’s exact (categorical variables), a one-way ANOVA test (normal distribution), or a Kruskal-Whallis H-test (skewed distribution) to test for differences among different groups.
We employed multivariate logistic regression models to evaluate the relationship between BRI and CircS diagnosis utilizing several multivariable models with various levels of adjustment. Three models were estimated: Model 1 was unadjusted; Model 2 adjusted for age and sex; Model 3 included the same adjustments as Model 2 with further adjustments for education level, residence, marital status, smoking, drinking, physical activity, blood pressure, HbA1c, history of hypertension, dyslipidemia, diabetes, cardiovascular disease and chronic kidney disease. We selected these confounders on the basis of previous research (12, 13), significant covariates in the univariate analysis, or their associations with the outcomes of interest or a change in effect estimate exceeding 10%. All variables included in the models met the criteria of tolerance (> 0.1) and had a variance inflation factor < 10. We assessed the diagnostic performance of BRI in determining CircS in comparison to adiposity indices employing ROC curve analyses, and utilized a restricted cubic spline to assess the dose-response relationship between BRI and CircS.
We employed multivariate logistic regression models to assess the relationship between CVD incidence among CircS patients and BRI, along with other adiposity indicators, after a 9-year follow-up. We estimated three models: Model 1 was unadjusted; Model 2 adjusted for age and sex; Model 3 included the same adjustments as Model 2 with further adjustments for education level, residence, marital status, smoking, drinking and physical activity. We selected these confounders on the basis of previous research (5, 18), significant covariates in the univariate analysis, or their associations with the outcomes of interest or a change in effect estimate exceeding 10%. All variables included in the models met the criteria of tolerance > 0.1 and variance inflation factor < 10. We conducted stratified analyses by sex and age, producing P values for interactions, and sensitivity analysis to assess the results’ robustness by excluding any participants who experienced CVD during the first 2 years. All analyses were conducted with R Statistical Software (Version 4.2.2, http://www.R-project.org, The R Foundation) and the Free Statistics analysis platform (Version 1.9). A two-sided P value < 0.05 was considered statistically significant.
Table 1 presents the characteristics of the participants according to CircS. After satisfying the above inclusion criteria, we analyzed a total of 8,888 participants at the baseline (Figure 1).
The mean and standard deviation for age in the study population was 59.4 ± 9.3 years, and 3,584 (40.3%) participants had been diagnosed with CircS at baseline. Individuals with CircS were more likely to be older, female, have lower literacy, be physically inactive, and have higher blood pressure, TC, TG, LDL-C, HbA1c, CRP and adiposity index scores (all P <0.05, Table 1).
As the BRI increased, there was a corresponding increase in the prevalence of CircS. Table 2 demonstrates the correlation between BRI and CircS. In the unadjusted model, for each additional SD increase in BRI, the risk of CircS increased by 1.76. In all of the adjusted models, BRI was independently associated with CircS, and the adjusted odds ratios were 2.31 (2.17~2.45), and 2.14 (2~2.28), respectively.
When assessed as quartiles, there was a significant association between BRI and CircS in the second, third, and fourth quartiles—even after adjusting for all confounding factors (adjusted OR: 2.72, 95% CI: 2.3~3.23; adjusted OR: 5.91, 95% CI: 5.01~6.97; adjusted OR: 8.68, 95% CI: 7.31~10.32, respectively). Additionally, multivariable-adjusted restricted cubic spline analyses suggested “J-shaped” associations between BRI and risk of CircS (Supplementary Figure 1; P for nonlinearity < 0.05).
We used ROC curve analysis to compare the diagnostic efficacy of BRI with other adiposity indices in detecting CircS (Supplementary Figure 2). In diagnosing CircS, the BRI demonstrated the highest AUC values (AUC: 0.760, 95% CI: 0.750 ~ 0.770), exceeding those of ABSI (AUC: 0.617, 95% CI: 0.606 ~ 0.629), CI (AUC: 0.649, 95% CI: 0.683 ~ 0.705), BMI (AUC: 0.722, 95% CI: 0.711 ~ 0.733) and WC (AUC: 0.746, 95% CI: 0.736 ~ 0.756). Table 3 presents the diagnostic performance of each anthropometric index in identifying CircS, encompassing sensitivity, specificity, and corresponding optimal cut-off values. BRI exhibited the highest Youden indices (0.415) for identifying CircS, with an optimal cut-off of 3.96.
Table 3. Cut-off between area under the curve, sensitivity, and specificity for adiposity indices to detect circadian syndrome.
Table 4 presents the characteristics of the participants with CircS according to the BRI quartiles (Q1: < 4.00, Q2: 4.00 ~ 4.82, Q3: 4.82 ~ 5.65, and Q4: > 5.65). Among these 2,322 participants with CircS, the prevalence of heart disease, stroke, and CVD was 10.3% (239/2,322), 22.4% (520/2,322) and 29.3% (680/2,322), respectively. Individuals with higher BRI were more likely to be older, female, have lower literacy, higher BMI, blood pressure, TC, LDL-C, and HbA1c, and have a higher likelihood of being diagnosed with hypertension (all P <0.05, Table 4).
The associations between BRI and different CVD components are shown in Table 5. In all adjusted models, for each additional SD increase, BRI was independently associated with CVD occurrence, and the adjusted odds ratios were 1.16 (1.06~1.28), and 1.14 (1.04~1.26), respectively. When assessed as quartiles, the associations between incident CVD and the second, third, and fourth BRI quartiles were 1.30 (95% CI: 0.99~1.69), 1.32 (95% CI: 1.01~1.72), and 1.59 (95% CI: 1.21~2.08), respectively, relative to the first BRI quartile. However, only the fourth BRI quartile was significantly associated with the incidence of heart disease and stroke, with ORs of 1.09 (95% CI: 0.98~1.21) and 1.18 (95% CI: 1.03~1.36). To evaluate whether there was a dose-response relationship between BRI and CVD incidence in CircS patients, we used a smoothing function analysis. Adjusted smoothed plots suggested a straightforward linear relationship between BRI and CVD incidence (Supplementary Figure 3, P for non-linearity = 0.635). As the BRI level increases, the risk of CVD shows an upward trend.
We also explore the relationship between other obesity indices and CVD occurrence (Supplementary Table 1). After adjusting for confounding factors, compared with the first quantile, the fourth quartiles of BMI, WC, and CVAI were significantly associated with the occurrence of CVD (OR: 1.54, 95% CI: 1.18~2; OR: 1.72, 95% CI: 1.33~2.22; OR: 1.34, 95% CI: 1.03~1.73, respectively). To evaluate whether there was a dose-response relationship between obesity indices and CVD incidence in CircS patients, we used a smoothing function analysis. After adjusting for potential confounding factors, we observed a linear relationship between BMI, CVAI and CVD (Supplementary Figures 3, 4; P for non-linearity >0.05). Additionally, the risk of CVD incidence was linearly associated with BMI and CVAI (Supplementary Figures 4, 5), and followed a J -shaped curve with respect to WC (Supplementary Figure 6).
Table 6 shows results stratified by sex, and age group. We found similar associations between BRI and CVD in most of the subgroup analyses. In subgroup analysis, the third and fourth BRI quartiles were significantly associated with higher CVD incidence in men (adjusted OR: 1.72, 95% CI: 1.12~2.64; adjusted OR: 2.59, 95% CI: 1.55~4.33, respectively). However, we found similar positive associations among women, with no significant differences. Furthermore, the significant associations between the fourth BRI quartiles and CVD incidence in the middle-aged and elderly adults were 1.48 (95% CI: 1.02~2.14) and 1.83 (95% CI: 1.23~2.72). Figure 2 illustrates a forest plot of values after adjusting for confounding factors for sex and age.
Furthermore, a sensitivity analysis showed results similar to the primary analysis. When excluding those participants with less than 2 years of follow-up, we observed results similar to the association between BRI and CVD incidence in CircS patients, as shown in Supplementary Table 2. The associations between incident CVD and the fourth BRI quartiles were 1.59 (95% CI: 1.21~2.1), relative to the first BRI quartile.
In this study, we investigated the relationship between CircS, BRI, and CVD among middle-aged and elderly individuals. We found that BRI was independently associated with CircS, outperforming obesity indices such as BMI and WC in identifying individuals with CircS. During the 9-year follow-up, we discovered a significant association between elevated BRI and CVD incidence, especially among men with CircS.
This is the first study to reveal an independent association between BRI and CircS. In this study, the prevalence of CircS was 40.3%, consistent with previous research findings (5). CircS, based on metabolic syndrome and associated with circadian rhythm disturbances, represented a new CVD risk cluster (4). The other two components—reduced sleep duration and depression—were also linked to CVD (23, 24). Additionally, as a new anthropometric index, BRI provided a better prediction of body fat and visceral adipose tissue volume (11). Some research has indicated that BRI could serve as a fat indicator to determine the presence of hyperuricemia (25, 26), arterial stiffness (27–29), CVD (30, 31), diabetes (25, 26, 32, 33), dyslipidemia (34, 35), and hypertension (36, 37). An extensive meta-analysis revealed that BRI demonstrated strong predictive ability for metabolic syndrome across various nationalities and races, outperforming traditional indices like BMI, WHR, ABSI, and BAI in predicting metabolic syndrome (38). Recent studies have also shown an association between BRI levels and depressive symptoms (39, 40). Additionally, a meta-analysis of prospective cohort studies revealed that compared to normal sleep duration, short sleep duration (defined in most studies as less than 5 or 6 hours per day) was linked to a 38% absolute increase in the incidence of obesity (41). Further evidence has suggested that insufficient sleep and circadian rhythm disruption contributes to poor metabolic health and obesity by altering various components of energy metabolism and behavior (42). In addition, obese individuals frequently report issues with sleep duration and quality (43), which may be linked to behavioral factors such as poor diet and lack of physical exercise among this population (42). Our study revealed that BRI, when compared to obesity indices such as BMI and WC, exhibits a higher AUC and a stronger overall discriminative capacity, showing exceptional performance in CircS diagnosis among middle-aged and elderly Chinese individuals. This indicates that BRI, as a quantitative indicator of visceral fat, is a more reliable marker for CircS than general obesity indices.
Our research revealed a significant correlation between elevated BRI levels and CVD incidence in CircS patients. Compared to the first quartile, individuals in the third and fourth quartiles of BRI have a higher risk of CVD occurrence. This suggests that even when considering the circadian rhythm’s predictive role in CVD occurrence, the effect of abdominal obesity should not be ignored. Previous studies have indicated that visceral adipose tissue is an important predictor of cardiovascular risk. BRI has been shown to improve the ability to predict body fat and visceral adipose tissue, thereby better reflecting the body’s health status (11). It has also been found that BRI has a U-shaped relationship with all-cause mortality and cardiovascular mortality (15). This could be due to the significant association between BRI and insulin resistance (38), which leads to imbalances in glucose and lipid metabolism, oxidative stress, inflammation, and vascular endothelial cell damage (44). Additionally, we discovered that among CircS patients, other obesity indices, such as BMI, are significantly associated with CVD occurrence only in the fourth quartile, with no statistical significance observed in the second or third quartiles. This could be due to the closer relationship between abdominal obesity and disruptions in circadian rhythms (45, 46), as well as cardiovascular disease, compared to general obesity. Moreover, circadian rhythm disturbances could disrupt lipid and glucose metabolism, gut microbiota, and the neuroendocrine regulation of appetite. Sleep deprivation exacerbated by circadian rhythm disruptions may also lead to unhealthy eating habits, resulting in metabolic disorders and ultimately obesity, especially abdominal obesity (42).
Of note, we found that men with CircS who had elevated BRI levels have a higher risk of developing CVD. In this study, the proportion of females in the CircS group was higher, and among CircS patients, the proportion of females increased with higher BRI levels. However, existing evidence on differences in circadian rhythm disturbances and obesity between the sexes is limited and sometimes conflicting (47), calling for more rigorous evidence exploring their sex-specificity. It has been established that men with CircS have a higher risk of CVD than women (5, 21). In this study, we considered that this may be related to the cardioprotective effect of estrogen under circadian disruption. It has been found that circadian disruption has a significant sex-dependent effect on glucose and energy metabolism (48), leading to abnormal levels of the satiety hormone leptin, the respiratory quotient and the hunger hormone ghrelin (49), with disturbances in these processes being risk factors for obesity and CVD. Male gonadal hormones exacerbate these metabolic effects, while female gonadal hormones can mitigate them (50). However, further evidence needed to investigate the potential mechanisms of sex hormones in abdominal obesity and CVD under circadian disruption remains lacking.
Our study has several advantages in terms of design and strategy. Firstly, we employed a comprehensive dataset from a nationally representative epidemiological survey, characterized by a large sample size and a prolonged follow-up period. This robust dataset allowed us to generalize our findings to the broader middle-aged and elderly population in China. Additionally, this is the first study to investigate the association between BRI and CVD occurrence in CircS patients, and the results were stratified to explore the effects of sex and age on the outcomes. The results indicated that CircS and BRI should be incorporated into the early warning and prevention of cardiovascular disease in middle-aged and elderly individuals.
Our observational study also has several limitations. Firstly, even though we adjusted for many covariates, unmeasured factors may still have biased the results. Additionally, CVD diagnosis relied on self-reported physician diagnoses rather than medical records, which may have introduced some deviation. However, self-reported CVD has been shown to be reliable in ascertaining non-fatal events (51, 52). Additionally, other large-scale studies have demonstrated notable agreement between self-reported CVD and medical records (53). Therefore, we interpreted our findings with caution and recommend further prospective studies of larger populations to improve the accuracy and reliability of the results.
In our study, BRI was independently associated with CircS, outperforming obesity indices such as BMI and WC in identifying individuals with CircS. During the 9-year follow-up, elevated BRI levels was significantly associated with CVD incidence in CircS patients, especially among men. These observations have important implications for current CVD management strategies, indicating that BRI can be an excellent tool for screening CircS. Thus, early identification of high-risk populations with CircS and elevated BRI levels may help promote healthy aging in middle-aged and elderly individuals.
The datasets presented in this study can be found in online repositories. The names of the repository/repositories and accession number(s) can be found below: China Health and Retirement Longitudinal Study (CHARLS)(http://charls.pku.edu.cn/).
The studies involving humans were approved by The Ethics Review Committee at the Peking University School of Medicine. The studies were conducted in accordance with the local legislation and institutional requirements. The participants provided their written informed consent to participate in this study.
FZ: Conceptualization, Data curation, Formal Analysis, Writing – original draft. WS: Data curation, Methodology, Software, Writing – original draft. JW: Data curation, Methodology, Writing – original draft. HC: Data curation, Formal Analysis, Writing – original draft. WX: Formal Analysis, Methodology, Writing – original draft. TL: Writing – review & editing. WJ: Writing – review & editing. XC: Funding acquisition, Supervision, Writing – review & editing. WL: Funding acquisition, Supervision, Writing – review & editing.
The author(s) declare that financial support was received for the research, authorship, and/or publication of this article. This work was supported by the Guangzhou Basic and Applied Basic Research Foundation (2024A03J0030), the Research Project of Guangdong Provincial Bureau of Traditional Chinese Medicine (20242023), the 17th Guangdong Provincial Hospital of Chinese Medicine Outstanding Talent Program (BJ2022YL10) and the Guangdong Provincial Hospital of Chinese Medicine Special Fund for Clinical Research (YN10101910).
We would like to thank all of the participants and investigators who participated in the China Health and Retirement Longitudinal Study (CHARLS).
The authors declare that the research was conducted in the absence of any commercial or financial relationships that could be construed as a potential conflict of interest.
The author(s) declare that no Generative AI was used in the creation of this manuscript.
All claims expressed in this article are solely those of the authors and do not necessarily represent those of their affiliated organizations, or those of the publisher, the editors and the reviewers. Any product that may be evaluated in this article, or claim that may be made by its manufacturer, is not guaranteed or endorsed by the publisher.
The Supplementary Material for this article can be found online at: https://www.frontiersin.org/articles/10.3389/fendo.2025.1532344/full#supplementary-material.
1. Diseases GBD, Injuries C. Global burden of 369 diseases and injuries in 204 countries and territories, 1990-2019: A systematic analysis for the global burden of disease study 2019. Lancet. (2020) 396:1204–22. doi: 10.1016/S0140-6736(20)30925-9
2. Shaw LJ, Goyal A, Mehta C, Xie J, Phillips L, Kelkar A, et al. 10-year resource utilization and costs for cardiovascular care. J Am Coll Cardiol. (2018) 71:1078–89. doi: 10.1016/j.jacc.2017.12.064
3. Wang Z, Ma L, Liu M, Fan J, Hu S, Writing Committee of the Report on Cardiovascular H, et al. Summary of the 2022 report on cardiovascular health and diseases in China. Chin Med J (Engl). (2023) 136:2899–908. doi: 10.1097/CM9.0000000000002927
4. Zimmet P, Alberti K, Stern N, Bilu C, El-Osta A, Einat H, et al. The circadian syndrome: is the metabolic syndrome and much more! J Intern Med. (2019) 286:181–91. doi: 10.1111/joim.12924
5. Shi Z, Tuomilehto J, Kronfeld-Schor N, Alberti GK, Stern N, El-Osta A, et al. The circadian syndrome predicts cardiovascular disease better than metabolic syndrome in chinese adults. J Intern Med. (2021) 289:851–60. doi: 10.1111/joim.13204
6. Polkinghorne MD, West HW, Antoniades C. Adipose tissue in cardiovascular disease: from basic science to clinical translation. Annu Rev Physiol. (2024) 86:175–98. doi: 10.1146/annurev-physiol-042222-021346
7. Koenen M, Hill MA, Cohen P, Sowers JR. Obesity, adipose tissue and vascular dysfunction. Circ Res. (2021) 128:951–68. doi: 10.1161/CIRCRESAHA.121.318093
8. Van’t Klooster CC, Ridker PM, Hjortnaes J, van der Graaf Y, Asselbergs FW, Westerink J, et al. The relation between systemic inflammation and incident cancer in patients with stable cardiovascular disease: A cohort study. Eur Heart J. (2019) 40:3901–9. doi: 10.1093/eurheartj/ehz587
9. Koskinas KC, Van Craenenbroeck EM, Antoniades C, Bluher M, Gorter TM, Hanssen H, et al. Obesity and cardiovascular disease: an esc clinical consensus statement. Eur J Prev Cardiol. (2024) 1–37. doi: 10.1093/eurjpc/zwae279
10. Ceniccola GD, Castro MG, Piovacari SMF, Horie LM, Correa FG, Barrere APN, et al. Current technologies in body composition assessment: advantages and disadvantages. Nutrition. (2019) 62:25–31. doi: 10.1016/j.nut.2018.11.028
11. Thomas DM, Bredlau C, Bosy-Westphal A, Mueller M, Shen W, Gallagher D, et al. Relationships between body roundness with body fat and visceral adipose tissue emerging from a new geometrical model. Obes (Silver Spring). (2013) 21:2264–71. doi: 10.1002/oby.20408
12. Ding J, Chen X, Shi Z, Bai K, Shi S. Association of body roundness index and its trajectories with all-cause and cardiovascular mortality among a chinese middle-aged and older population: A retrospective cohort study. Front Public Health. (2023) 11:1107158. doi: 10.3389/fpubh.2023.1107158
13. Zhang S, Han S, Zheng L, Sun Y, Sun Z. Associations of trajectories in body roundness index with incident cardiovascular disease: A prospective cohort study in rural China. Front Nutr. (2024) 11:1291093. doi: 10.3389/fnut.2024.1291093
14. Nkwana MR, Monyeki KD, Lebelo SL. Body roundness index, a body shape index, conicity index, and their association with nutritional status and cardiovascular risk factors in South African rural young adults. Int J Environ Res Public Health. (2021) 18:281. doi: 10.3390/ijerph18010281
15. Zhou D, Liu X, Huang Y, Feng Y. A nonlinear association between body roundness index and all-cause mortality and cardiovascular mortality in general population. Public Health Nutr. (2022) 25:3008–15. doi: 10.1017/S1368980022001768
16. Zhao Y, Hu Y, Smith JP, Strauss J, Yang G. Cohort profile: the China health and retirement longitudinal study (Charls). Int J Epidemiol. (2014) 43:61–8. doi: 10.1093/ije/dys203
17. Wang S, Chen R, Liu Q, Shu Z, Zhan S, Li L. Prevalence, awareness and treatment of chronic kidney disease among middle-aged and elderly: the China health and retirement longitudinal study. Nephrol (Carlton). (2015) 20:474–84. doi: 10.1111/nep.12449
18. Hu X, Nie Z, Ou Y, Lin L, Qian Z, Vaughn MG, et al. Long-term exposure to ambient air pollution, circadian syndrome and cardiovascular disease: A nationwide study in China. Sci Total Environ. (2023) 868:161696. doi: 10.1016/j.scitotenv.2023.161696
19. Ran J, Tao C, Zhang S, Chen Q, Yang P, Hu Y, et al. Circadian syndrome is associated with the development of chronic kidney disease and rapid decline in kidney function in middle-aged and elder adults: A China nationwide cohort study. J Nutr Health Aging. (2024) 28:100011. doi: 10.1016/j.jnha.2023.100011
20. Andresen EM, Malmgren JA, Carter WB, Patrick DL. Screening for depression in well older adults: evaluation of a short form of the ces-D (Center for epidemiologic studies depression scale). Am J Prev Med. (1994) 10:77–84. doi: 10.1016/S0749-3797(18)30622-6
21. Shi Z, Tuomilehto J, Kronfeld-Schor N, Alberti G, Stern N, El-Osta A, et al. The circadian syndrome is a significant and stronger predictor for cardiovascular disease than the metabolic syndrome-the nhanes survey during 2005-2016. Nutrients. (2022) 14:5317. doi: 10.3390/nu14245317
22. Harshfield EL, Pennells L, Schwartz JE, Willeit P, Kaptoge S, Bell S, et al. Association between depressive symptoms and incident cardiovascular diseases. JAMA. (2020) 324:2396–405. doi: 10.1001/jama.2020.23068
23. Hall MH, Brindle RC, Buysse DJ. Sleep and cardiovascular disease: emerging opportunities for psychology. Am Psychol. (2018) 73:994–1006. doi: 10.1037/amp0000362
24. Hare DL, Toukhsati SR, Johansson P, Jaarsma T. Depression and cardiovascular disease: A clinical review. Eur Heart J. (2014) 35:1365–72. doi: 10.1093/eurheartj/eht462
25. Zhang N, Chang Y, Guo X, Chen Y, Ye N, Sun Y. A body shape index and body roundness index: two new body indices for detecting association between obesity and hyperuricemia in rural area of China. Eur J Intern Med. (2016) 29:32–6. doi: 10.1016/j.ejim.2016.01.019
26. Tian S, Zhang X, Xu Y, Dong H. Feasibility of body roundness index for identifying a clustering of cardiometabolic abnormalities compared to bmi, waist circumference and other anthropometric indices: the China health and nutrition survey, 2008 to 2009. Med (Baltimore). (2016) 95:e4642. doi: 10.1097/MD.0000000000004642
27. Haraguchi N, Koyama T, Kuriyama N, Ozaki E, Matsui D, Watanabe I, et al. Assessment of anthropometric indices other than bmi to evaluate arterial stiffness. Hypertens Res. (2019) 42:1599–605. doi: 10.1038/s41440-019-0264-0
28. Gomez-Sanchez L, Garcia-Ortiz L, Patino-Alonso MC, Recio-Rodriguez JI, Rigo F, Marti R, et al. Adiposity measures and arterial stiffness in primary care: the mark prospective observational study. BMJ Open. (2017) 7:e016422. doi: 10.1136/bmjopen-2017-016422
29. Zhang J, Fang L, Qiu L, Huang L, Zhu W, Yu Y. Comparison of the ability to identify arterial stiffness between two new anthropometric indices and classical obesity indices in chinese adults. Atherosclerosis. (2017) 263:263–71. doi: 10.1016/j.atherosclerosis.2017.06.031
30. Chung W, Park JH, Chung HS, Yu JM, Kim DS, Moon S. Utility of the Z-score of log-transformed a body shape index (Lbsiz) in the assessment for sarcopenic obesity and cardiovascular disease risk in the United States. Sci Rep. (2019) 9:9292. doi: 10.1038/s41598-019-45717-8
31. Maessen MF, Eijsvogels TM, Verheggen RJ, Hopman MT, Verbeek AL, de Vegt F. Entering a new era of body indices: the feasibility of a body shape index and body roundness index to identify cardiovascular health status. PloS One. (2014) 9:e107212. doi: 10.1371/journal.pone.0107212
32. Zhao Q, Zhang K, Li Y, Zhen Q, Shi J, Yu Y, et al. Capacity of a body shape index and body roundness index to identify diabetes mellitus in han chinese people in northeast China: A cross-sectional study. Diabetes Med. (2018) 35:1580–7. doi: 10.1111/dme.13787
33. Yang J, Wang F, Wang J, Han X, Hu H, Yu C, et al. Using different anthropometric indices to assess prediction ability of type 2 diabetes in elderly population: A 5 year prospective study. BMC Geriatr. (2018) 18:218. doi: 10.1186/s12877-018-0912-2
34. Zaid M, Ameer F, Munir R, Rashid R, Farooq N, Hasnain S, et al. Anthropometric and metabolic indices in assessment of type and severity of dyslipidemia. J Physiol Anthropol. (2017) 36:19. doi: 10.1186/s40101-017-0134-x
35. Zhang K, Zhao Q, Li Y, Zhen Q, Yu Y, Tao Y, et al. Feasibility of anthropometric indices to identify dyslipidemia among adults in jilin province: A cross-sectional study. Lipids Health Dis. (2018) 17:16. doi: 10.1186/s12944-017-0648-6
36. Chang Y, Guo X, Guo L, Li Z, Li Y, Sun Y. The feasibility of two new anthropometric indices to identify hypertension in rural China: A cross-sectional study. Med (Baltimore). (2016) 95:e5301. doi: 10.1097/MD.0000000000005301
37. Choi JR, Ahn SV, Kim JY, Koh SB, Choi EH, Lee GY, et al. Comparison of various anthropometric indices for the identification of a predictor of incident hypertension: the arirang study. J Hum Hypertens. (2018) 32:294–300. doi: 10.1038/s41371-018-0043-4
38. Rico-Martin S, Calderon-Garcia JF, Sanchez-Rey P, Franco-Antonio C, Martinez Alvarez M, Sanchez-Munoz-Torrero JF. Effectiveness of body roundness index in predicting metabolic syndrome: A systematic review and meta-analysis. Obes Rev. (2020) 21:e13023. doi: 10.1111/obr.13023
39. Wang Y, Zhang X, Li Y, Gui J, Mei Y, Yang X, et al. Predicting depressive symptom by cardiometabolic indicators in mid-aged and older adults in China: A population-based cross-sectional study. Front Psychiatry. (2023) 14:1153316. doi: 10.3389/fpsyt.2023.1153316
40. Zhang L, Yin J, Sun H, Dong W, Liu Z, Yang J, et al. The relationship between body roundness index and depression: A cross-sectional study using data from the national health and nutrition examination survey (Nhanes) 2011-2018. J Affect Disord. (2024) 361:17–23. doi: 10.1016/j.jad.2024.05.153
41. Chaput JP, Dutil C, Featherstone R, Ross R, Giangregorio L, Saunders TJ, et al. Sleep duration and health in adults: an overview of systematic reviews. Appl Physiol Nutr Metab. (2020) 45:S218–S31. doi: 10.1139/apnm-2020-0034
42. Chaput JP, McHill AW, Cox RC, Broussard JL, Dutil C, da Costa BGG, et al. The role of insufficient sleep and circadian misalignment in obesity. Nat Rev Endocrinol. (2023) 19:82–97. doi: 10.1038/s41574-022-00747-7
43. Jean-Louis G, Williams NJ, Sarpong D, Pandey A, Youngstedt S, Zizi F, et al. Associations between inadequate sleep and obesity in the us adult population: analysis of the national health interview survey (1977-2009). BMC Public Health. (2014) 14:290. doi: 10.1186/1471-2458-14-290
44. Witteles RM, Fowler MB. Insulin-resistant cardiomyopathy clinical evidence, mechanisms, and treatment options. J Am Coll Cardiol. (2008) 51:93–102. doi: 10.1016/j.jacc.2007.10.021
45. Chen JD, Lin YC, Hsiao ST. Obesity and high blood pressure of 12-hour night shift female clean-room workers. Chronobiol Int. (2010) 27:334–44. doi: 10.3109/07420520903502242
46. Macagnan J, Pattussi MP, Canuto R, Henn RL, Fassa AG, Olinto MT. Impact of nightshift work on overweight and abdominal obesity among workers of a poultry processing plant in southern Brazil. Chronobiol Int. (2012) 29:336–43. doi: 10.3109/07420528.2011.653851
47. Smith KL, Danyluk AB, Munir SS, Covassin N. Shift work and obesity risk-are there sex differences? Curr Diabetes Rep. (2022) 22:341–52. doi: 10.1007/s11892-022-01474-z
48. Zhu L, Zou F, Yang Y, Xu P, Saito K, Othrell Hinton A Jr., et al. Estrogens prevent metabolic dysfunctions induced by circadian disruptions in female mice. Endocrinology. (2015) 156:2114–23. doi: 10.1210/en.2014-1922
49. Qian J, Morris CJ, Caputo R, Wang W, Garaulet M, Scheer F. Sex differences in the circadian misalignment effects on energy regulation. Proc Natl Acad Sci U.S.A. (2019) 116:23806–12. doi: 10.1073/pnas.1914003116
50. In Het Panhuis W, Schonke M, Siebeler R, Banen D, Pronk ACM, Streefland TCM, et al. Circadian disruption impairs glucose homeostasis in male but not in female mice and is dependent on gonadal sex hormones. FASEB J. (2023) 37:e22772. doi: 10.1096/fj.202201586R
51. Engstad T, Bonaa KH, Viitanen M. Validity of self-reported stroke: the tromso study. Stroke. (2000) 31:1602–7. doi: 10.1161/01.str.31.7.1602
52. Barr EL, Tonkin AM, Welborn TA, Shaw JE. Validity of self-reported cardiovascular disease events in comparison to medical record adjudication and a statewide hospital morbidity database: the ausdiab study. Intern Med J. (2009) 39:49–53. doi: 10.1111/j.1445-5994.2008.01864.x
Keywords: cardiovascular disease, circadian syndrome, body roundness index, obesity, CHARLS
Citation: Zhang F, Shi W, Wen J, Cao H, Xu W, Lan T, Jiang W, Chen X and Lu W (2025) Elevated body roundness index increases the risk of cardiovascular disease in Chinese patients with circadian syndrome. Front. Endocrinol. 16:1532344. doi: 10.3389/fendo.2025.1532344
Received: 21 November 2024; Accepted: 04 February 2025;
Published: 25 February 2025.
Edited by:
Yashendra Sethi, PearResearch, IndiaReviewed by:
Duong Dinh Le, Hue University of Medicine and Pharmacy, VietnamCopyright © 2025 Zhang, Shi, Wen, Cao, Xu, Lan, Jiang, Chen and Lu. This is an open-access article distributed under the terms of the Creative Commons Attribution License (CC BY). The use, distribution or reproduction in other forums is permitted, provided the original author(s) and the copyright owner(s) are credited and that the original publication in this journal is cited, in accordance with accepted academic practice. No use, distribution or reproduction is permitted which does not comply with these terms.
*Correspondence: Xiankun Chen, eGlhbmt1bi5jaGVuQGd6dWNtLmVkdS5jbg==; Weihui Lu, d2VpaHVpLmx1QGd6dWNtLmVkdS5jbg==
Disclaimer: All claims expressed in this article are solely those of the authors and do not necessarily represent those of their affiliated organizations, or those of the publisher, the editors and the reviewers. Any product that may be evaluated in this article or claim that may be made by its manufacturer is not guaranteed or endorsed by the publisher.
Research integrity at Frontiers
Learn more about the work of our research integrity team to safeguard the quality of each article we publish.