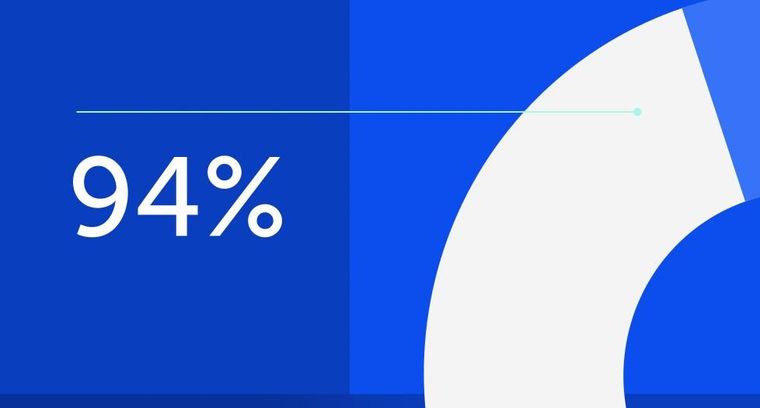
94% of researchers rate our articles as excellent or good
Learn more about the work of our research integrity team to safeguard the quality of each article we publish.
Find out more
ORIGINAL RESEARCH article
Front. Endocrinol., 25 February 2025
Sec. Reproduction
Volume 16 - 2025 | https://doi.org/10.3389/fendo.2025.1448530
This article is part of the Research TopicMale Reproduction and Oxidative Stress, Volume IIView all 14 articles
Background: Infertility affects 8-12% of couples globally, manifesting as a complex reproductive disorder with varied causes, negatively impacting emotional, physical, and social well-being. Inflammation is implicated in many diseases, including male and female infertility.
Methods: This study employed Mendelian randomization (MR) with two-sample, bidirectional, and mediation approaches to explore the relationship between circulating inflammatory proteins and infertility. Causal analysis was conducted using inverse variance-weighted (IVW) and MR-Egger regression, supplemented by enrichment analysis, protein-protein interaction (PPI) network exploration, and drug signature analysis.
Results: Our findings identified a significant positive correlation between C-X-C motif chemokine 6 (CXCL6) and male infertility, positioning CXCL6 as a potential therapeutic target or biomarker. No causal links were detected between circulating inflammatory proteins and female infertility post-FDR adjustment. Minor mediation effects were observed for metabolites such as androstenediol monosulfate, arachidonoylcholine, and serum phosphate to glycerol ratio. Cytokine-related pathways emerged as significant in both male and female infertility. Gene-drug interaction analysis highlighted the need for further investigation of pioglitazone in treating female infertility.
Conclusion: This study establishes a potentially causal relationship between CXCL6 and male infertility, suggesting its potential as a drug target or molecular biomarker. The integrative approach combining causal inference with molecular pathway and drug interaction analysis opens new avenues for understanding and treating infertility.
Infertility is a worldwide human health problem that affects approximately over 186 million individuals globally (1, 2). The causes of infertility vary, with female factors accounting for about 70% of infertility cases, with common causes including ovulatory disorders and fallopian tube damage (3). Male factors contribute to the remaining roughly 30% of infertility cases due to causes such as abnormal sperm count, morphology, or lifespan (4). Independent of etiology, infertility has a profound emotional, physical, and social impact on couples and results in a significant psychological burden (5). Thus, understanding the causes of infertility and exploring a possible causal link between risk factors and infertility are crucial for affected couples and healthcare professionals.
Inflammation is a physiologic host response to infection or injury. However, aberrant inflammatory responses result in tissue damage and are central to the pathogenesis of multiple diseases, including reproductive diseases (6). Inflammation can have significant effects on both male and female infertility. For example, untreated sexually transmitted diseases in females can lead to tubal factor infertility due to tubal inflammation, damage, and scarring (7). Mutations in TNFAIP3 have been linked to both infertility and central nervous system inflammation, highlighting the role of inflammation in female infertility (8). In males, inflammation can lead to leukocytospermia, due to the production of reactive oxygen free radicals, and chronic testicular inflammation, such as orchitis, is also known to cause male infertility (9, 10). The role of inflammation and oxidative stress in male infertility is well-documented, with mechanistic pathways linking causative factors of male reproductive tract inflammation, oxidative stress induction, and oxidant-sensitive cellular cascades leading to male infertility (11, 12). The presence of inflammatory states and the identification of semen markers of inflammation are crucial for the diagnostic-therapeutic management of male infertility (13). These results emphasize the critical role of inflammation in both male and female infertility, highlighting the need for further research and therapeutic interventions targeting inflammatory pathways to address infertility.
Inflammatory responses are orchestrated by a complex network of cells and inflammatory mediators, such as circulating inflammatory proteins (CIPs). For instance, high levels of CIPs, like growth-regulated oncogene-alpha (GROα) are related to increased risks of female infertility (14). CXCL6 plays a key role in recruiting neutrophils to facilitate non-specific immunity during the process of inflammation (15). Additionally, GCP-2/CXCL6 exhibits antibacterial activity and plays key roles in host defense of the male urogenital tract as well as during fertilization (16). Furthermore, CIPs are vulnerable to the direct effects of conventional small molecules or biologics, making them attractive potential drug targets (17). Therefore, the discovery of genetic determinants affecting CIP levels may offer important clues about the etiology of multiple diseases, such as infertility.
Randomized controlled trials (RCTs) are a gold standard means to test hypotheses at a population level (18, 19). However, RCTs require intensive human resources, cost, and time, and some interventional strategies are not suitable for RCT-dependent assessments. Recently, Mendelian randomization (MR) has gained attention as a complementary method of exploring the relationship between the treatment and outcome in patients, based on the frequency of single nucleotide polymorphisms (SNPs) as the instrumental variable (IV). This approach might avoid the confounding bias of conventional epidemiological investigations (20). Here, we performed a comprehensive two-sample MR analysis to determine the potential causal association between CIPs and infertility.
This epidemiologic MR study was carried out by adhering to the STROBE guidelines (21). The reliable implementation of MR is based on three major assumptions: (i) relevance (genetic variants are strongly linked to the exposure), (ii) independence (variants are not correlated with confounders), and (iii) absence of horizontal pleiotropy (variants affect the outcome only via the exposure) (22). Here, we utilized bidirectional and 2-sample MR to understand the pathological correlation between CIPs and infertility. Then we used significant positive CIPs to construct a phenome-wide association study (PheWAS) and phenotype scanning analysis. We also used MR to assess for a casual relationship between significant CIP and plasma metabolites to find potential mediators of infertility. A protein-protein interaction (PPI) map was then generated between the positive CIP and infertility, and we performed functional enrichment analysis and drug signature analysis with positive CIPs (Figure 1).
The genome-wide pQTL (protein quantitative trait loci) mapping for CIP was obtained from studies by Zhao et al. (23). This dataset contained 91 CIP measured using the Olink Target Inflammation panel in 11 cohorts, with a total of 14,824 participants of European ancestry. The plasma metabolite data was obtained from a series of large GWASs by Chen et al. (24). This dataset included 1,091 metabolites and 309 metabolite ratios in 8,299 individuals from the Canadian Longitudinal Study on Aging (CLSA) cohort. We used publicly available GWAS summary datasets for male infertility from the FinnGen cohort (R9 release, total n = 73479; case =680, control = 72799) and female infertility data from the FinnGen cohort (R9 release, total n = 75450; case =6481, control = 68969). FinnGen is a large public-private collaboration that includes genomic data from 500,000 individuals of Finnish individuals who are over 18 years of age (25). These GWAS summary datasets and the GWAS summary datasets data for sensitivity analysis are listed in Table 1.
Exposure-associated independent SNPs (r2 = 0.001, ≥10000 kb) were selected according to their genome-wide (GW) significance (p<1.0×10−5) (26). The F-statistic (F-stat) of a phenotype is a measure of instrument strength (IS) and is related to the proportion of variance. An F-stat of at least 10 variables indicates a relatively lower risk of instrument bias (IB) in MR analysis (27). The number of SNPs for each CIP and the corresponding scale unit and F-stats are presented in Supplementary Table S1.
First, SNPs were harmonized to the exposure and outcome in an allele-specific manner to ensure proper alignment of effects. Wherever applicable, if any instrumental SNPs for the exposure were absent in the corresponding outcome dataset, a proxy was incorporated using GVs in the linkage disequilibrium (r2 > 0.8).
Initially, we employed an inverse variance-weighted (IVW) meta-analysis approach for MR analysis (28), in addition to the weighted median (29) and MR-Egger regression (MR-ER) approach. Multiple testing was conducted based on the false discovery rate (FDR) method and a pFDR<0.05 was deemed a significant CIP. P values <0.05 indicated a potential CIP.
The MR-ER method was further used to assess any potential impacts of the directional pleiotropy (DP) (30) and to perform the MR-pleiotropy residual sum and outlier (MR-PRESSO) method. A P value > 0.05 indicated no horizontal pleiotropy. Data heterogeneity was determined by Cochran’s Q test (31), with P > 0.05 indicating no heterogeneity Leave-one-out (LOO) analysis indicated the influence of a single SNP on overall estimates.
“TwoSampleMR v0.5.8,” “MRPRESSO v1.0”, and “MendelianRandomizaiton v0.9.0” packages in R v4.3.1 (source codes: https://github.com/studentyaoshi/MR) were applied for statistical analyses.
To detect potential RC, genetic instruments for infertility were selected in a similar strategy to CIPs selection from GWAS datasets for bidirectional MR analysis (32). The effect was estimated by MR-Egger, IVW, and weighted mode. A P<0.05 indicated statistical significance.
In mediation terms, a two-step MR was applied to distinguish between indirect and direct effects. Significant CIP-related genetic instruments (GIs) were used to assess the causal effects of plasma metabolites. GIs for plasma metabolites were then used to determine the effect of each plasma metabolite on possible CIP-related infertility. Notably, if significant CIP-related infertility was found to influence the mediator, we used the “product of coefficients” approach to evaluate the indirect effects of significant CIPs on infertility. The delta method was applied to calculate the standard error (SE) for an indirect effect.
To evaluate the horizontal pleiotropy of significant CIPs, a PheWAS was performed. We compiled a comparison SNP-set to serve as a control for PheWAS analyses. Four control SNPs were matched to the significant CIP-associated SNPs on: minor allele frequency (± 5%), surrounding gene density (± 50%), distance to the nearest gene (± 50%), and as a proxy for haplotype block size, the number of other SNPs in LD at R 2 ≥ 0.50 (± 50%) (33). GeneATLAS is a searchable dataset for 778 traits (118 quantitative, 660 binary) and associations with 9,113,133 genetic variants (genotyped or imputed) and can be queried for genetic or phenotypic data to assess genotype-phenotype associations (34). We queried GeneATLAS for trait associations with significant CIPs. Nominally significant SNP-trait associations (p < 0.01) were carried forward to trait-enrichment analyses, as previously described (35). PheWAS associations of the significant CIP SNPs with 778 phenotypic traits were compared to PheWAS results for the control SNP-set. We used Fisher’s exact tests to compare the frequency at which individual traits were associated with significant CIPs SNPs versus control SNPs to determine if traits were enriched for association with significant CIP risk variants. Fisher’s exact p-values for trait enrichment underwent FDR correction to adjust for multiple testing.
Phenotype scanning was carried out by searching the previous GWAS via a ‘phenoscanner’ to reveal the associations of an identified CIPs with other traits (36). A pleiotropic SNP was considered to possess: (i) a significant genome-wide association (P<5 × 10−8), (ii) a relationship with European descendants in GWAS, and (iii) associations with any known etiological risk factors, including protein, metabolic, and clinical traits. Additionally, the LD r2 among identified CIPs revealed their potential linkages with potential infertility drug targets.
The PPI network analysis (STRING v12; https://string-db.org/; minimum interaction score = 0.4) was used to detect any associations between positive CIPs.
Enrichr platform (https://maayanlab.cloud/Enrichr/), an independent web server for gene set enrichment analysis (37), was used to perform functional enrichment analysis on positive CIPs. Drug Signatures Database (DSigDB) was used to identify drug molecules that interacted with positive CIPs through the Enrichr platform.
All relevant data for this study are included in the article or uploaded as online supplemental information. Full GWAS summary statistics for the exposure and outcome data used in the manuscript can be found at https://www.ebi.ac.uk/gwas, https://gwas.mrcieu.ac.uk/, or https://r9.risteys.finngen.fi.
Two-sample MR and IVW analyses were performed to reveal any causal effects of CIPs on male infertility. After FDR adjustment, only C-X-C motif chemokine 6 (CXCL6) was found to have a positive relationship with male infertility (95% CI: 1.7564 to 6.2502, pFDR=0.01966) and considered a significant CIP. Another 6 CIPs were identified as having potentially significant relationships impacting male infertility, including 3 beneficial: Monocyte chemoattractant protein-1 (MCP1) (95% CI: 1.0956 to 1.7563, p=0.0066), Cystatin D (CST5) (95% CI: 1.0403 to 1.6323, p=0.0213), and Interleukin-2 (IL2) (95% CI: 1.0198 to 2.1619, p=0.0392) and 3 deleterious: Interleukin-1-alpha (IL1A) (95% CI: 0.4252 to 0.9275, p=0.0194), C-C motif chemokine 25 (CCL25) (95% CI: 0.7241 to 0.9867, p=0.0332), and TNF-related activation-induced cytokine (TRANCE) (95% CI: 0.6154 to 0.9888, p=0.0401). These results were further validated by LOO testing. Sensitivity analysis confirmed heterogeneity in the results without evidence of pleiotropy (Figure 2, Supplementary Table S2). MR-PRESSO analysis showed no horizontal pleiotropy and identified outliers (Supplementary Table S2), and bidirectional MR analyses did not identify significant causal relationships between male infertility and 7 positive CIPs (Supplementary Table S3).
Figure 2. MR analysis between CIPs and infertility. (A) The association between CIPs trait and male infertility. (B) The association between CIPs trait and female infertility. NSNP, number of single-nucleotide polymorphism. OR (95%CI): odds ratio (95% confidence interval). FDR, false discovery rate. A pFDR<0.05 was deemed a significant CIP, and Pval <0.05 indicated a potential CIP. Q_pval, p value of Cochran’s Q test. Egger_pval, p value of MR-Egger regression (MR-ER) method. Global_p, p value of MR-PRESSO.
After FDR adjustment, no CIPs were identified as having potential casual relationships with female infertility compared to non-FDR adjusted data, which identified 8 potentially significant CIPs. Of these, 3 had a beneficial impact on female infertility: Interleukin-5 (IL5) (95% CI: 1.0332 to 1.3101, p=0.0125), Oncostatin-M (OSM) (95% CI: 1.0242 to 1.2889, p=0.0179), and Artemin (ARTN) (95% CI: 1.0155 to 1.2518, p=0.0246), and 5 had a deleterious effect: Caspase 8 (CASP8) (95% CI: 0.7427 to 0.9517, p=0.0061), Leukemia inhibitory factor receptor (LIFR) (95% CI: 0.799 to 0.9798, p=0.0187), Interleukin-18 (IL18) (95% CI: 0.8182 to 0.9823, p=0.0192), Urokinase-type plasminogen activator (PLAU) (95% CI: 0.8273 to 0.9944,p=0.0376), and Interleukin-20 (IL20) (95% CI: 0.7768 to 0.9996, p=0.0493). Sensitivity analysis confirmed heterogeneity in the results without pleiotropy (Figure 2, Supplementary Table S2). MR-PRESSO analysis showed no horizontal pleiotropy and identified outliers (Supplementary Table S2). Bidirectional MR analyses also did not find evidence of significant causal relationships between female infertility and the 8 significant CIPs (Supplementary Table S3).
Given that CIPs may influence plasma metabolites, we used 1400 plasma metabolites from a prior study by Chen et al. (24) for mediation analysis.
First, IVs for CXCL6 were used to evaluate the potential causal effects of CXCL6 exposure on the plasma metabolites. Using IVW analysis, we found potential causal effects of CXCL6 on 57 of the 1400 tested plasma metabolites (Figure 3, Supplementary Table S4). Next, we assessed the potential associations of these plasma metabolites with male infertility. Sixty-one plasma metabolites were identified as having causal effects on male infertility via IVW analyses (Figure 3, Supplementary Table S4). Of the total 118 plasma metabolites associated with CXCL6 or male infertility, androstenediol (3beta,17beta) monosulfate (2) levels, sphingomyelin (d18:1/20:2, d18:2/20:1, d16:1/22:2) levels, sphingomyelin (d18:1/20:1, d18:2/20:0) levels, arachidonoylcholine levels, X-12822 levels, and phosphate to glycerol ratio appeared in both groups.
Figure 3. MR analysis for mediation analysis. (A) Positive results of IVW method for the association between CXCL6 and 1400 plasma metabolites. (B) Positive results of IVW method for the association between 1400 plasma metabolites and male infertility. The p-value represents significance. The smaller the p-value, the redder the color is, indicating a more significant result. Red color ID represents the GWAS code of potential mediators.
We estimated the indirect effect of CXCL6 on male infertility via 6 potential mediators (Table 2), however, the results were not significant. Androstenediol (3beta,17beta) monosulfate (2) levels, arachidonoylcholine (AA-CHOL) levels, and phosphate to glycerol ratio had 1.4%, 1.2%, and 1.85% mediation effect, respectively. While sphingomyelin (d18:1/20:2, d18:2/20:1, d16:1/22:2) levels, sphingomyelin (d18:1/20:1, d18:2/20:0) levels and X-12822 levels indicated suppressing effects instead of any mediating effects (38). Additionally, the bidirectional MR analyses didn’t find any evidence of significant causal relationships between 6 potential mediators and CXCL6 (Supplementary Table S5), suggesting that CXCL6 cannot serve as a mediator as well.
PheWAS results can be interpreted as the association of genetically determined protein expression with specific diseases or traits. We first identified 7 CXCL6-associated SNPs from previous MR analyses, 4 of which successfully passed clumping and matched to 4 control SNPs each (Supplementary Table S6). PheWAS analyses were then performed with the UK Biobank GeneATLAS database to test each CXCL6-associated variant and control variant for association with 778 traits. A total of 6 traits were more likely to be associated with CXCL6 SNPs than with control SNPs at statistical significance (p<0.05), including neutrophil count, lymphocyte percentage, neutrophil percentage, white blood cell (leukocyte) count, monocyte count, and basophil percentage (Supplementary Table S7).
In phenotype scanning, CXCL6 (rs12075, rs13148728, rs597808, rs74361503) expression was found to have associations with 138 other traits (Supplementary Table S8), 18 of which were related to disease. We then used MR to check the causal relationship between CXCL6 and 18 related diseases, but no causal relationship was identified (Supplementary Table S9). Collectively, these data suggest that CXCL6 may serve as a plasma molecular biomarker for male infertility.
GO analyses in male infertility identified potential CIPs chiefly involved in cytokine pathways with effects on cytokine activity (Figure 4), however in female infertility, potential CIPs had effects on cytokine receptor binding, serine protease inhibitor complex, or pro-inflammatory and profibrotic mediators (Figure 4).
Figure 4. Bar graphs of GO enrichment analysis and pathway enrichment analysis for potential causal CIPs. (A) Top 10 terms of male infertility in BP. (B) Top 10 terms of male infertility in MF. (C) WikiPathway of male infertility. (D) KEGG Pathway of male infertility. (E) Reactome Pathway of male infertility. (F) BioCarta Pathway of male infertility. (G) Top 10 terms of female infertility in BP. (H) Top 10 terms of female infertility in MF. (I) Top 10 terms of female infertility in CC. (J) WikiPathway of female infertility. (K) KEGG Pathway of female infertility. (L) Reactome Pathway of female infertility. (M) BioCarta Pathway of female infertility. BP, Biological Process; MF, Molecular Function; CC, Cellular Component.
The pathways with the strongest implications for potential CIPs were captured among four global bases, including WikiPathway, KEGG, Reactome, and BioCarta. These results identified pro-inflammatory and profibrotic mediators and cytokine-cytokine receptor interaction as the strongest pathways in male and female infertility in WikiPathway and KEGG, respectively. Inflammatory Bowel Disease Signaling also appeared in 4 pathway results (Figure 4).
We generated a PPI network of potential CIPs in male and female infertility (Figure 5). Of the potential CIPs, IL2 and IL1A had the highest combined score (0.997) in male infertility, and OSM and LIFR had the highest combined score (0.999) in female infertility (Supplementary Table S10).
Figure 5. Protein-protein interaction network among the potential causal CIPs. (A) Relationship between the suggestive causal CIPs (P < 0.05) in male infertility. (B) Relationship between the suggestive causal CIPs (P < 0.05) in female infertility.
We then used Enrichr to analyze the positive or negative relationships between potential CIPs infertility and identify drugs that may modulate these CIPs using the DSigDB database (Tables 3, 4). For positively related CIPs indicated that manganese and pioglitazone are the top drug candidates for male and female infertility, respectively. With respect to negatively related CIPs, leupeptin and histamine emerged as the top drug candidates for treating male and female infertility, respectively.
Infertility is a complex problem affecting both males and females through varied processes. Male infertility etiologies include genetic mutations (39), autoimmune responses (40), oxidative stress (41), and inflammatory indicators (42). Female infertility has also been linked to oxidative stress (43) and inflammatory mechanisms (44). Here, we use two-sample Mendelian Randomization to investigate infertility-associated CIPs and plasma metabolites linked to infertility. We identified CXCL6 as a promising therapeutic target and possible plasma biomarker for male infertility. Using the infertility-associated CIPs, we also explored potential infertility mechanisms using enrichment analyses, Protein-Protein Interaction (PPI), and Gene-drug interaction studies. We found some potential drugs like leupeptin or pioglitazone which are already used for some diseases, thereby advancing the understanding of both male and female infertility.
CXCL6 is a chemokine involved in neutrophil trafficking and activation that has been implicated in the pathogenesis of several diseases and has been shown to promote cancer cell metastasis, fibrosis, and inflammation (45–47). Moreover, studies have suggested that CXCL6 can be regulated by specific cell signaling pathways, such as the HIF-1α pathway in hepatocellular carcinoma and the IL4 pathway through the JAK-STAT mechanism in atopic dermatitis (48, 49). The diverse roles of CXCL6 in various diseases and its involvement in cellular processes and the tumor microenvironment make it a promising drug target for therapeutic intervention in multiple pathological conditions. Our findings align with existing research identifying CXCL6 as a potential plasma biomarker in many conditions (50–52). Significantly, our phenotype analysis and PheWAS results indicate that CXCL6 is not associated with other diseases. This lack of association with additional conditions enhances its suitability as a specific plasma biomarker for male infertility, offering a more targeted and potentially accurate diagnostic tool in this context. Our findings propose that CXCL6 not only warrants in-depth study but also presents potential as both a drug target and a plasma molecular biomarker for male infertility.
In our study, we analyzed potentially causal links between 1,400 plasma metabolites and male infertility using the Inverse Variance Weighted (IVW) methodology. This approach led to the identification of 66 metabolites positively correlated with male infertility. Notably, we observed that levels of androstenediol (3beta,17beta) monosulfate (2), AA-CHOL, and the phosphate-to-glycerol ratio were associated with both CXCL6 and male infertility. Androstenediol (3beta,17beta) monosulfate (2) is a metabolite involved in various physiological functions. Matsui et al. (53) noted its role in testosterone sulfate metabolism in rats, while other studies have identified it as a precursor in testosterone synthesis from DHEA (54). Additionally, the anabolic steroid 19-nor-4-androstenediol-3 beta and one of its derivatives 17 beta-diol (3 beta,19-NA), have been hypothesized to increase muscle and bone mass with minimal prostate stimulation (55). AA-CHOL acts as an endogenous modulator of acetylcholine signaling, found in atherosclerotic plaques and the arterial intima (56), and is known to regulate cholinesterases (57). Acetylcholine is involved in penile erection and relaxation as well as the central control of ejaculation. Acetylcholinesterase is found to plays role in the pathogenesis of male infertility via modulating inflammatory pathways (58). These data suggested that AA-CHOL might affect male fertility via modulating acetylcholine and acetylcholinesterase activities. Furthermore, phosphate is crucial for male fertility and affects sperm motility and function, as well as semen liquefaction (59, 60). However, its role in correcting infertility is unclear, as simple dietary supplementation cannot reverse infertility in mice (61). Glycerol plays a critical role in lipid metabolism and sperm motility but has both beneficial and harmful effects on sperm health and viability (62–64). Exactly how the phosphate-to-glycerol ratio affects male infertility merits further research.
Our enrichment analysis underscored the importance of cytokine-related pathways in infertility, aligning with existing research that implicates a deleterious role of cytokines in both male and female infertility. Cytokines are signaling molecules that play crucial roles in testicular function and male infertility. Cytokines like interleukin 1β (IL-1β), IL-6, IL-10, IL-18, tumor necrosis factor α (TNF-α), interferon g (IFN-g), and transforming growth factor β1 (TGF-β1) are notably elevated in idiopathically infertile males and those with varicocele (65, 66). Attia et al. (67) also highlighted the significant role of pro-inflammatory cytokines and microRNAs in male infertility. Cytokines in the male gonad primarily originate from testicular macrophages, with Leydig and Sertoli cells also contributing. Additionally, cytokines, such as IL2 and IL1A had the highest combined score in the PPI network associated with male infertility. IL-2 plays a crucial role in T-cell mediated immune responses. IL-2 levels in seminal plasma are found to be related to sperm count, motility, and morphology, and may be a potential marker in male infertility (68). IL-2 concentrations are closely associated with Testosterone hormone levels in COVID-19 infertile patients (69). IL-1 is primarily secreted by macrophages and monocytes in reaction to foreign antigens and inflammatory stimuli. A previous study has demonstrated that IL1A is involved in testicular paracrine/autocrine regulation, potentially influencing spermatogenesis, spermiogenesis, and male fertility (70). In female infertility, it has been shown that cytokine profiles are differentially expressed in the peritoneal fluid of patients with and without endometriosis being evaluated for infertility (71). Notably, the levels of CXCL6 are influenced by other cytokines and reproductive hormones, further supporting its function in both normal and abnormal human reproduction (72). A deeper understanding of cytokines’ roles in infertility could lead to the development of novel therapeutic approaches for those facing conception challenges.
Our gene-drug interaction analysis for positively related CIPs indicated that manganese and pioglitazone are the top drug candidates for male and female infertility, respectively. Manganese is necessary for metabolism, growth, and reproduction (73), but excess levels have been linked to male infertility (74) via mechanisms that include increased spontaneous abortion rates in partners, impotence, and infertility (75). Pioglitazone already shows potential in treating female infertility, particularly in polycystic ovary syndrome (PCOS). Nagao et al. (76) found that pioglitazone can suppress excessive ovarian follicule development in mice, suggesting a mechanism explaining its impact on reproductive health. With respect to negatively related CIPs, leupeptin and histamine emerged as the top drug candidates for treating male and female infertility, respectively. Leupeptin is a protease inhibitor used in the treatment of AIDS, hepatitis, pancreatitis, and cancer (77) with high antiviral activity against influenza and coronavirus, notably binding strongly with the TMPRSS2 protease in COVID-19 (78). Leupeptin also acts as an autophagy-blocking agent, which is implicated in its mechanism of action in the treatment of HIV-1-associated neurological disorders (79), yet a role for it in the treatment of male infertility is unknown. Histamine has a regulatory effect on the female reproductive system and is known to influence the electrophysiology of the human oviduct, affecting fertility (80, 81). Together, these two molecules merit further study in the treatment of female infertility.
Limitations of this study included the analysis of disease-causing effects identified in different studies, and inconsistent measurements across studies might have introduced biases in results. Additionally, only one target CXCL6 passed our initial FDR correction, so we performed other analyses on potential associated targets. Moreover, the CXCL6 detection results was not validated in clinical male infertility patients, and further studies are required to confirm the diagnostic value of CXCL6 and its practical applicability as a biomarker in male infertility. Furthermore, the study population and datasets used here primarily comprise patients of European ancestry, which may limit applicability with respect to other racial and ethnic groups. Therefore, caution is needed when generalizing our results to more diverse global populations. Further research in ethnically diverse cohorts is essential to validate our results and to assess whether similar genetic associations hold true across different racial and ethnic groups. Lastly, we did not analyze the correlation between CXCL6 and ART outcomes. Moreover studies are necessary to explore this relationship and its potential role in reproductive success.
Using several methods of genetic analyses and Mendelian randomization, we found that circulating CXCL6 may be causally associated with male infertility and have applications as a potential drug target or plasma molecular biomarker in male infertility. Cytokine-related pathways were also highly associated with the development and progression of infertility, and gene-drug interaction analysis suggested pioglitazone may help treat female infertility. Further studies are warranted to explore the potential of CXCL6 as a biomarker for early screening and diagnosis of male infertility, evaluate its role in therapeutic development, and assess the clinical feasibility of related drugs such as pioglitazone in improving fertility outcomes of patients struggling with infertility.
The original contributions presented in the study are included in the article/Supplementary Material. Further inquiries can be directed to the corresponding author.
This study used only publicly available, deidentified summary statistics, and specific ethical review and informed consent had been obtained in all the original studies. The studies were conducted in accordance with the local legislation and institutional requirements. The participants provided their written informed consent to participate in this study.
PC: Conceptualization, Data curation, Formal Analysis, Investigation, Methodology, Software, Validation, Visualization, Writing – original draft, Writing – review & editing. SN: Conceptualization, Methodology, Supervision, Visualization, Writing – original draft, Writing – review & editing. LO: Conceptualization, Supervision, Writing – review & editing.
The author(s) declare that no financial support was received for the research, authorship, and/or publication of this article.
The authors declare that the research was conducted in the absence of any commercial or financial relationships that could be construed as a potential conflict of interest.
All claims expressed in this article are solely those of the authors and do not necessarily represent those of their affiliated organizations, or those of the publisher, the editors and the reviewers. Any product that may be evaluated in this article, or claim that may be made by its manufacturer, is not guaranteed or endorsed by the publisher.
The Supplementary Material for this article can be found online at: https://www.frontiersin.org/articles/10.3389/fendo.2025.1448530/full#supplementary-material
1. Agarwal A, Baskaran S, Parekh N, Cho CL, Henkel R, Vij S, et al. Male infertility. Lancet. (2021) 397(10271):319–33. doi: 10.1016/S0140-6736(20)32667-2
2. Inhorn MC, Patrizio P. Infertility around the globe: new thinking on gender, reproductive technologies and global movements in the 21st century. Hum Reprod Update. (2015) 21(4):411–26. doi: 10.1093/humupd/dmv016
3. Al-Mahmood. AAS, Al-Ajeely IMZ. Epidemiology of female infertility among reproductive age women in tikrit city. Indian J Public Health Res Dev. (2020) 11(9):252–7. doi: 10.37506/ijphrd.v11i9.11017
4. Shrook AS, Al-Sabbah A.H.A.E.A.A.M. The most influential factors for a successful pregnancy after in vitro fertilization by model of probit regression. Ann Trop Med Public Health. (2020) 23(14):24. doi: 10.36295/asro.2020.231424
5. Lotti F, Maggi M. Sexual dysfunction and male infertility. Nat Rev Urol. (2018) 15(5):287–307. doi: 10.1038/nrurol.2018.20
6. Panigrahy D, Gilligan MM, Serhan CN, Kashfi K. Resolution of inflammation: An organizing principle in biology and medicine. Pharmacol Ther. (2021) 227:107879. doi: 10.1016/j.pharmthera.2021.107879
7. Tsevat DG, Wiesenfeld HC, Parks C, Peipert JF. Sexually transmitted diseases and infertility. Am J Obstet Gynecol. (2017) 216(1):1–9. doi: 10.1016/j.ajog.2016.08.008
8. Zammit NW, McDowell J, Warren J, Muskovic W, Gamble J, Shi YC, et al. TNFAIP3 reduction-of-function drives female infertility and CNS inflammation. Front Immunol. (2022) 13:811525. doi: 10.3389/fimmu.2022.811525
9. Jung JH, Kim MH, Kim J, Baik SK, Koh SB, Park HJ, et al. Treatment of leukocytospermia in male infertility: A systematic review. World J Mens Health. (2016) 34(3):165–72. doi: 10.5534/wjmh.2016.34.3.165
10. Schuppe HC, Meinhardt A, Allam JP, Bergmann M, Weidner W, Haidl G. Chronic orchitis: a neglected cause of male infertility? Andrologia. (2008) 40(2):84–91. doi: 10.1111/j.1439-0272.2008.00837.x
11. Dutta S, Sengupta P, Slama P, Roychoudhury S. Oxidative stress, testicular inflammatory pathways, and male reproduction. Int J Mol Sci. (2021) 22(18):10043. doi: 10.3390/ijms221810043
12. Hussain T, Kandeel M, Metwally E, Murtaza G, Kalhoro DH, Yin Y, et al. Unraveling the harmful effect of oxidative stress on male fertility: A mechanistic insight. Front Endocrinol (Lausanne). (2023) 14:1070692. doi: 10.3389/fendo.2023.1070692
13. Signorini C, Moretti E, Noto D, Micheli L, Ponchia R, Collodel G. Fatty acid oxidation and pro-resolving lipid mediators are related to male infertility. Antioxid (Basel). (2022) 11(1):107. doi: 10.3390/antiox11010107
14. Lin Y, Wang G, Li Y, Yang H, Zhao Y, Liu J, et al. Circulating inflammatory cytokines and female reproductive diseases: A mendelian randomization analysis. J Clin Endocrinol Metab. (2023) 108(12):3154–64. doi: 10.1210/clinem/dgad376
15. Dai CL, Yang HX, Liu QP, Rahman K, Zhang H. CXCL6: A potential therapeutic target for inflammation and cancer. Clin Exp Med. (2023) 23(8):4413–27. doi: 10.1007/s10238-023-01152-8
16. Collin M, Linge HM, Bjartell A, Giwercman A, Malm J, Egesten A. Constitutive expression of the antibacterial CXC chemokine GCP-2/CXCL6 by epithelial cells of the male reproductive tract. J Reprod Immunol. (2008) 79(1):37–43. doi: 10.1016/j.jri.2008.08.003
17. Folkersen L, Gustafsson S, Wang Q, Hansen DH, Hedman Å K, Schork A, et al. Genomic and drug target evaluation of 90 cardiovascular proteins in 30,931 individuals. Nat Metab. (2020) 2(10):1135–48. doi: 10.1038/s42255-020-00287-2
18. Hoffmann TJ, Choquet H, Yin J, Banda Y, Kvale MN, Glymour M, et al. A large multiethnic genome-wide association study of adult body mass index identifies novel loci. Genetics. (2018) 210(2):499–515. doi: 10.1534/genetics.118.301479
19. Stanley K. Design of randomized controlled trials. Circulation. (2007) 115(9):1164–9. doi: 10.1161/CIRCULATIONAHA.105.594945
20. Sekula P, Del Greco MF, Pattaro C, Kottgen A. Mendelian randomization as an approach to assess causality using observational data. J Am Soc Nephrol. (2016) 27(11):3253–65. doi: 10.1681/ASN.2016010098
21. Skrivankova VW, Richmond RC, Woolf BAR, Yarmolinsky J, Davies NM, Swanson SA, et al. Strengthening the reporting of observational studies in epidemiology using mendelian randomization: the STROBE-MR statement. JAMA. (2021) 326(16):1614–21. doi: 10.1001/jama.2021.18236
22. Burgess S, Butterworth A, Malarstig A, Thompson SG. Use of Mendelian randomisation to assess potential benefit of clinical intervention. BMJ. (2012) 345:e7325. doi: 10.1136/bmj.e7325
23. Zhao JH, Stacey D, Eriksson N, Macdonald-Dunlop E, Hedman AK, Kalnapenkis A, et al. Genetics of circulating inflammatory proteins identifies drivers of immune-mediated disease risk and therapeutic targets. Nat Immunol. (2023) 24(9):1540–51. doi: 10.1038/s41590-023-01588-w
24. Chen Y, Lu T, Pettersson-Kymmer U, Stewart ID, Butler-Laporte G, Nakanishi T, et al. Genomic atlas of the plasma metabolome prioritizes metabolites implicated in human diseases. Nat Genet. (2023) 55(1):44–53. doi: 10.1038/s41588-022-01270-1
25. Kurki MI, Karjalainen J, Palta P, Sipilä TP, Kristiansson K, Donner KM, et al. FinnGen provides genetic insights from a well-phenotyped isolated population. Nature. (2023) 613(7944):508–18. doi: 10.1038/s41586-022-05473-8
26. Larsson SC, Burgess S, Michaelsson K. Association of genetic variants related to serum calcium levels with coronary artery disease and myocardial infarction. JAMA. (2017) 318(4):371–80. doi: 10.1001/jama.2017.8981
27. Palmer TM, Lawlor DA, Harbord RM, Sheehan NA, Tobias JH, Timpson NJ, et al. Using multiple genetic variants as instrumental variables for modifiable risk factors. Stat Methods Med Res. (2012) 21(3):223–42. doi: 10.1177/0962280210394459
28. Burgess S, Butterworth A, Thompson SG. Mendelian randomization analysis with multiple genetic variants using summarized data. Genet Epidemiol. (2013) 37(7):658–65. doi: 10.1002/gepi.2013.37.issue-7
29. Bowden J, Davey Smith G, Haycock PC, Burgess S. Consistent estimation in mendelian randomization with some invalid instruments using a weighted median estimator. Genet Epidemiol. (2016) 40(4):304–14. doi: 10.1002/gepi.2016.40.issue-4
30. Burgess S, Thompson SG. Interpreting findings from Mendelian randomization using the MR-Egger method. Eur J Epidemiol. (2017) 32(5):377–89. doi: 10.1007/s10654-017-0255-x
31. Kulinskaya E, Dollinger MB. An accurate test for homogeneity of odds ratios based on Cochran’s Q-statistic. BMC Med Res Methodol. (2015) 15:49. doi: 10.1186/s12874-015-0034-x
32. Davey Smith G, Hemani G. Mendelian randomization: genetic anchors for causal inference in epidemiological studies. Hum Mol Genet. (2014) 23(R1):R89–98. doi: 10.1093/hmg/ddu328
33. Pers TH, Timshel P, Hirschhorn JN. SNPsnap: a Web-based tool for identification and annotation of matched SNPs. Bioinformatics. (2015) 31(33):418–20. doi: 10.1093/bioinformatics/btu655
34. Canela-Xandri O, Rawlik K, Tenesa A. An atlas of genetic associations in UK Biobank. Nat Genet. (2018) 50:1593–9. doi: 10.1038/s41588-018-0248-z
35. Semmes EC, Vijayakrishnan J, Zhang C, Hurst JH, Houlston RS, Walsh KM. Leveraging genome and phenome-wide association studies to investigate genetic risk of acute lymphoblastic leukemia. Cancer Epidemiol Biomarkers Prev. (2020) 29(8):1606–14. doi: 10.1158/1055-9965.EPI-20-0113
36. Kamat MA, Blackshaw JA, Young R, Surendran P, Burgess S, Danesh J, et al. PhenoScanner V2: an expanded tool for searching human genotype-phenotype associations. Bioinformatics. (2019) 35(22):4851–3. doi: 10.1093/bioinformatics/btz469
37. Kuleshov MV, Jones MR, Rouillard AD, Fernandez NF, Duan Q, Wang Z, et al. Enrichr: a comprehensive gene set enrichment analysis web server 2016 update. Nucleic Acids Res. (2016) 44(W1):W90–7. doi: 10.1093/nar/gkw377
38. MacKinnon DP, Krull JL, Lockwood CM. Equivalence of the mediation, confounding and suppression effect. Prev Sci. (2000) 1(4):173–81. doi: 10.1023/A:1026595011371
39. Liu K, Zhao R, Shen M, Ye J, Li X, Huang Y, et al. Role of genetic mutations in folate-related enzyme genes on Male Infertility. Sci Rep. (2015) 5:15548. doi: 10.1038/srep15548
40. Chereshnev VA, Pichugova SV, Beikin YB, Chereshneva MV, Iukhta AI, Stroev YI, et al. Pathogenesis of autoimmune male infertility: juxtacrine, paracrine, and endocrine dysregulation. Pathophysiology. (2021) 28(4):471–88. doi: 10.3390/pathophysiology28040030
41. Zhang QF, Wang S, Zhang H, Liu QL, Wei Y, Deng W, et al. Effects of alpha-lipoic acid on sperm quality in patients with varicocele-related male infertility: study protocol for a randomized controlled clinical trial. Trials. (2022) 23(1):1002. doi: 10.1186/s13063-022-06951-0
42. Bary A--, Elnilly D, khedr NA, Alamiri WM. Evaluation of seminal plasma interleukin 17 in infertile males. Hum Androl. (2021) 11(11):1–5. doi: 10.21608/ha.2021.79815.1076
43. Agarwal A, Aponte-Mellado A, Premkumar BJ, Shaman A, Gupta S. The effects of oxidative stress on female reproduction: a review. Reprod Biol Endocrinol. (2012) 10:49. doi: 10.1186/1477-7827-10-49
44. Huang Y, Hu C, Ye H, Luo R, Fu X, Li X, et al. Inflamm-aging: A new mechanism affecting premature ovarian insufficiency. J Immunol Res 2019. (2019) p:8069898. doi: 10.1155/2019/8069898
45. Liu Y, Li D, Chen Y, Liu Y, Lin Y, Huang X, et al. Integrated bioinformatics analysis for conducting a prognostic model and identifying immunotherapeutic targets in gastric cancer. BMC Bioinf. (2023) 24(1):191. doi: 10.1186/s12859-023-05312-1
46. Li X, Lee EJ, Gawel DR, Lilja S, Schafer S, Zhang H, et al. Meta-analysis of expression profiling data indicates need for combinatorial biomarkers in pediatric ulcerative colitis. J Immunol Res 2020. (2020) p:8279619. doi: 10.1155/2020/8279619
47. Lin D, Zhao W, Yang J, Wang H, Zhang H. Integrative analysis of biomarkers and mechanisms in adamantinomatous craniopharyngioma. Front Genet. (2022) 13:830793. doi: 10.3389/fgene.2022.830793
48. Tian H, Huang P, Zhao Z, Tang W, Xia J. HIF-1alpha plays a role in the chemotactic migration of hepatocarcinoma cells through the modulation of CXCL6 expression. Cell Physiol Biochem. (2014) 34(5):1536–46. doi: 10.1159/000366357
49. Subramanian I, Singh VK, Jere A. Elucidating mechanistic insights into drug action for atopic dermatitis: a systems biology approach. BMC Dermatol. (2018) 18(1):3. doi: 10.1186/s12895-018-0070-4
50. Julian DR, Kazakoff MA, Patel A, Jaynes J, Willis MS, Yates CC. Chemokine-based therapeutics for the treatment of inflammatory and fibrotic convergent pathways in COVID-19. Curr Pathobiol Rep. (2021) 9(4):93–105. doi: 10.1007/s40139-021-00226-0
51. Sun MY, Wang SJ, Li XQ, Shen YL, Lu JR, Tian XH, et al. CXCL6 promotes renal interstitial fibrosis in diabetic nephropathy by activating JAK/STAT3 signaling pathway. Front Pharmacol. (2019) 10:224. doi: 10.3389/fphar.2019.00224
52. Aversa J, Song M, Shimazu T, Inoue M, Charvat H, Yamaji T, et al. Prediagnostic circulating inflammation biomarkers and esophageal squamous cell carcinoma: A case-cohort study in Japan. Int J Cancer. (2020) 147(3):686–91. doi: 10.1002/ijc.v147.3
53. Matsui M, Kinuyama Y, Hakozaki M. Metabolism of testosterone sulfate in the rat: analysis of biliary metabolites. Steroids. (1975) 25(5):637–48. doi: 10.1016/0039-128X(75)90018-5
54. Vehof J, Hysi PG, Hammond CJ. A metabolome-wide study of dry eye disease reveals serum androgens as biomarkers. Ophthalmology. (2017) 124(4):505–11. doi: 10.1016/j.ophtha.2016.12.011
55. Page ST, Marck BT, Tolliver JM, Matsumoto AM. Tissue selectivity of the anabolic steroid, 19-nor-4-androstenediol-3beta,17beta-diol in male Sprague Dawley rats: selective stimulation of muscle mass and bone mineral density relative to prostate mass. Endocrinology. (2008) 149(4):1987–93. doi: 10.1210/en.2007-0956
56. Akimov MG, Kudryavtsev DS, Kryukova EV, Fomina-Ageeva EV, Zakharov SS, Gretskaya NM, et al. Arachidonoylcholine and other unsaturated long-chain acylcholines are endogenous modulators of the acetylcholine signaling system. Biomolecules. (2020) 10(2):283. doi: 10.3390/biom10020283
57. Gok M, Cicek C, Bodur E. Butyrylcholinesterase in lipid metabolism: A new outlook. J Neurochem. (2023) 168(4):381–5. doi: 10.1111/jnc.v168.4
58. Sadia K, Leonel Javeres MN, Tahir F, Shah STA, Habib R, Muneer Z, et al. Acetylcholinesterase, pro-inflammatory cytokines, and association of ACHE SNP rs 17228602 with male infertility. PloS One. (2023) 18(4):e0282579. doi: 10.1371/journal.pone.0282579
59. Dobrakowski M, Kaletka Z, Machon-Grecka A, Kasperczyk S, Horak S, Birkner E, et al. The role of oxidative stress, selected metals, and parameters of the immune system in male fertility. Oxid Med Cell Longev. (2018) 2018:6249536. doi: 10.1155/2018/6249536
60. Park YJ, Pang MG. Mitochondrial functionality in male fertility: from spermatogenesis to fertilization. Antioxid (Basel). (2021) 10(1):98. doi: 10.3390/antiox10010098
61. Ichikawa S, Austin AM, Gray AK, Allen MR, Econs MJ. Dietary phosphate restriction normalizes biochemical and skeletal abnormalities in a murine model of tumoral calcinosis. Endocrinology. (2011) 152(12):4504–13. doi: 10.1210/en.2011-1137
62. Crisostomo L, Alves MG, Calamita G, Sousa M, Oliveira PF. Glycerol and testicular activity: the good, the bad and the ugly. Mol Hum Reprod. (2017) 23(11):725–37. doi: 10.1093/molehr/gax049
63. Morita M, Fujinoki M, Okuno M. K+-independent initiation of motility in chum salmon sperm treated with an organic alcohol, glycerol. J Exp Biol. (2005) 208(Pt 23):4549–56. doi: 10.1242/jeb.01921
64. Parrilla I, del Olmo D, Caballero I, Tarantini T, Cuello C, Gil MA, et al. The effect of glycerol concentrations on the post-thaw in vitro characteristics of cryopreserved sex-sorted boar spermatozoa. Reprod Domest Anim. (2012) 47(6):965–74. doi: 10.1111/j.1439-0531.2012.02000.x
65. Havrylyuk A, Chopyak V, Boyko Y, Kril I, Kurpisz M. Cytokines in the blood and semen of infertile patients. Cent Eur J Immunol. (2015) 40(3):337–44. doi: 10.5114/ceji.2015.54596
66. Fang Y, Su Y, Xu J, Hu Z, Zhao K, Liu C, et al. Varicocele-mediated male infertility: from the perspective of testicular immunity and inflammation. Front Immunol. (2021) 12:729539. doi: 10.3389/fimmu.2021.729539
67. Attia H, Finocchi F, Orciani M, Mehdi M, Zidi Jrah I, Lazzarini R, et al. Pro-inflammatory cytokines and microRNAs in male infertility. Mol Biol Rep. (2021) 48(8):5935–42. doi: 10.1007/s11033-021-06593-6
68. Paradisi R, Capelli M, Mandini M, Bellavia E, Focacci M, Flamigni C. Interleukin-2 in seminal plasma of fertile and infertile men. Arch Androl. (1995) 35(1):35–41. doi: 10.3109/01485019508987851
69. Hammood SA, Younus AS, Al-Fatlawey ES, ALkaabi ZS. Assessment of concentrations of interleukin IL-1β, interleukin 2, interleukin 15 and level of Testosterone hormone in COVID-19 infertile patients. Multidiscip Sci J. (2024) 6(8):2024144–2024144. doi: 10.31893/multiscience.2024144
70. Huleihel M, Lunenfeld E. Involvement of intratesticular IL-1 system in the regulation of Sertoli cell functions. Mol Cell Endocrinol. (2002) 187(1):125–32. doi: 10.1016/S0303-7207(01)00690-6
71. Jorgensen H, Hill AS, Beste MT, Kumar MP, Chiswick E, Fedorcsak P, et al. Peritoneal fluid cytokines related to endometriosis in patients evaluated for infertility. Fertil Steril. (2017) 107(5):1191–1199.e2. doi: 10.1016/j.fertnstert.2017.03.013
72. Ullah A, Wang MJ, Wang YX, Shen B. CXC chemokines influence immune surveillance in immunological disorders: Polycystic ovary syndrome and endometriosis. Biochim Biophys Acta Mol Basis Dis. (2023) 1869(5):166704. doi: 10.1016/j.bbadis.2023.166704
73. Wu C, Woo JG, Zhang N. Association between urinary manganese and blood pressure: Results from National Health and Nutrition Examination Survey (NHANES), 2011-2014. PloS One. (2017) 12(11):e0188145. doi: 10.1371/journal.pone.0188145
74. Frisbie SH, Mitchell EJ, Sarkar B. Urgent need to reevaluate the latest World Health Organization guidelines for toxic inorganic substances in drinking water. Environ Health. (2015) 14:63. doi: 10.1186/s12940-015-0050-7
75. Chao HH, Zhang Y, Dong PY, Gurunathan S, Zhang XF. Comprehensive review on the positive and negative effects of various important regulators on male spermatogenesis and fertility. Front Nutr. (2022) 9:1063510. doi: 10.3389/fnut.2022.1063510
76. Nagao S, Baba T, Fujibe Y, Adachi S, Ikeda K, Morishita M, et al. Pioglitazone suppresses excessive follicular development in murine preantral follicles. J Ovarian Res. (2019) 12(1):82. doi: 10.1186/s13048-019-0556-7
77. Singh R, Kumar M, Mittal A, Mehta PK. Microbial metabolites in nutrition, healthcare and agriculture. Biotech. (2017) 7(1):15. doi: 10.1007/s13205-016-0586-4
78. Ramakrishnan J, Kandasamy S, Iruthayaraj A, Magudeeswaran S, Chinnasamy K, Poomani K. Strong binding of leupeptin with TMPRSS2 protease may be an alternative to camostat and nafamostat for SARS-coV-2 repurposed drug: evaluation from molecular docking and molecular dynamics simulations. Appl Biochem Biotechnol. (2021) 193(6):1909–23. doi: 10.1007/s12010-020-03475-8
79. Ojha CR, Lapierre J, Rodriguez M, Dever SM, Zadeh MA, DeMarino C, et al. Interplay between autophagy, exosomes and HIV-1 associated neurological disorders: new insights for diagnosis and therapeutic applications. Viruses. (2017) 9(7):176. doi: 10.3390/v9070176
80. Zhang F, Xiong DH, Wang W, Shen H, Xiao P, Yang F, et al. HDC gene polymorphisms are associated with age at natural menopause in Caucasian women. Biochem Biophys Res Commun. (2006) 348(4):1378–82. doi: 10.1016/j.bbrc.2006.08.008
Keywords: infertility, Mendelian randomization, drug target, molecular biomarker, CXCL6
Citation: Chen P, Ni S and Ou-Yang L (2025) Causal inference of inflammatory proteins in infertility: a Mendelian randomization study. Front. Endocrinol. 16:1448530. doi: 10.3389/fendo.2025.1448530
Received: 13 June 2024; Accepted: 05 February 2025;
Published: 25 February 2025.
Edited by:
Weibin Bai, Jinan University, ChinaReviewed by:
Zhengrui Li, Shanghai Jiao Tong University, ChinaCopyright © 2025 Chen, Ni and Ou-Yang. This is an open-access article distributed under the terms of the Creative Commons Attribution License (CC BY). The use, distribution or reproduction in other forums is permitted, provided the original author(s) and the copyright owner(s) are credited and that the original publication in this journal is cited, in accordance with accepted academic practice. No use, distribution or reproduction is permitted which does not comply with these terms.
*Correspondence: Sha Ni, bnNzamNtdUAxNjMuY29t
Disclaimer: All claims expressed in this article are solely those of the authors and do not necessarily represent those of their affiliated organizations, or those of the publisher, the editors and the reviewers. Any product that may be evaluated in this article or claim that may be made by its manufacturer is not guaranteed or endorsed by the publisher.
Research integrity at Frontiers
Learn more about the work of our research integrity team to safeguard the quality of each article we publish.