- 1Jiangxi Medical College, Nanchang University, Nanchang, Jiangxi, China
- 2Jiangxi Provincial Geriatric Hospital, Jiangxi Provincial People’s Hospital, The First Affiliated Hospital of Nanchang Medical College, Nanchang, Jiangxi, China
- 3Jiangxi Cardiovascular Research Institute, Jiangxi Provincial People’s Hospital, The First Affiliated Hospital of Nanchang Medical College, Nanchang, Jiangxi, China
Objective: The Estimated Glucose Disposal Rate (eGDR) serves as a surrogate marker for insulin resistance, with numerous studies highlighting its significant prognostic value. This paper aims to analyze the impact of eGDR on cardiovascular and all-cause mortality across different glycemic metabolic statuses, including normal fasting glucose (NFG), prediabetes, and diabetes.
Methods: This study included 46,016 American adults who underwent health examinations as part of the National Health and Nutrition Examination Survey from 1999 to 2018. Multivariable Cox regression was employed to explore the relationships between eGDR and mortality rates under varying glycemic states. Additionally, Kaplan-Meier curves were used to compare the cumulative incidence of cardiovascular and all-cause mortality across different metabolic statuses. Finally, the predictive value of eGDR for mortality was assessed using receiver operating characteristic curves.
Results: During an average follow-up of 115 months, a total of 6,906 (15.01%) participants experienced all-cause mortality, with 1,798 (3.91%) deaths attributed to cardiovascular causes. Kaplan-Meier analysis revealed that higher eGDR levels were associated with gradually reduced mortality rates. After adjusting for confounders, elevated eGDR levels were protective against both cardiovascular and all-cause mortality; the protective effect was notably stronger for cardiovascular mortality [Cardiovascular mortality hazard ratio: 0.92; All-cause mortality hazard ratio: 0.94]. Further interaction tests indicated that glycemic status significantly modified the protective effect of eGDR (P-interaction<0.0001); specifically, high eGDR conferred stronger protection against cardiovascular and all-cause mortality in individuals with NFG and prediabetes compared to those with diabetes. Receiver operating characteristic analysis suggested that eGDR had superior predictive value for mortality in the NFG and prediabetic populations compared to the diabetic group.
Conclusion: eGDR is a straightforward surrogate for insulin resistance, acting as a protective factor against cardiovascular and all-cause mortality in American adults, with glycemic status modifying this protective effect. Specifically, high eGDR levels offer stronger protection in individuals with NFG and prediabetes compared to those with diabetes; moreover, eGDR appears to be more suitable for predicting mortality events in the NFG and prediabetic populations.
Background
Cardiovascular diseases (CVD) are a major health concern worldwide (1). According to the Global Burden of Disease study, the total incidence of CVD increased from 271 million cases in 1990 to 523 million cases in 2019, with associated cardiovascular deaths rising from 12.1 million in 1990 to 18.6 million in 2019 (1, 2). Despite intensified efforts in primary and secondary prevention and the widespread dissemination of knowledge about cardiovascular health, emphasizing the importance of lifestyle changes (3, 4), the global incidence of CVD continues to rise significantly (1). This suggests that there may be unrecognized residual cardiovascular risks (5). Current research largely agrees that adverse metabolic factors such as dyslipidemia (6, 7), hypertension (8), and abnormal glucose metabolism (9) are closely linked to the onset of CVD, with hypertension showing the strongest correlation (10).
Insulin resistance (IR) is a metabolic condition characterized by reduced responsiveness of target organs or tissues to insulin (11), leading to dysregulation of blood glucose (12) and affecting overall metabolic health. Extensive research has confirmed that IR increases the risk of stroke (12), CVD (11–13), and mortality (14–17); thus, understanding the characteristics and effects of IR is crucial for the prevention and treatment of metabolic diseases. Against this backdrop, several methods for assessing IR have been developed: (i) direct methods such as the hyperinsulinemic-euglycemic clamp technique and the insulin suppression test (18), which are invasive and costly, limiting their clinical application. (ii) Simple surrogate markers for IR, such as the Homeostatic Model Assessment for IR (HOMA-IR) (19), the triglyceride-glucose index (20), and the Estimated Glucose Disposal Rate (eGDR) (21). Previous studies predominantly used HOMA-IR to define IR; however, HOMA-IR is susceptible to interference from medications such as insulin, insulin sensitizers, and insulin secretagogues (22, 23). Furthermore, the newly developed triglyceride-glucose index, although it includes triglycerides (TG) (20), does not account for hypertension, which may weaken its effectiveness in assessing CVD risk. The eGDR is a new surrogate marker calculated based on obesity index waist circumference (WC), glycemic index glycated hemoglobin (HbA1c), and hypertension; studies suggest that eGDR has a similar accuracy to the hyperinsulinemic-euglycemic clamp technique in assessing IR status (24). Moreover, clinical evidence indicates that a lower eGDR is associated with an increased risk of mortality from various diseases (25–30); however, these studies primarily focus on individuals with diabetes or prediabetes, which could exaggerate or obscure the role of eGDR, necessitating further clarification of the impact of glycemic metabolic status on eGDR-related mortality. To address this issue, the present study aims to assess the impact of eGDR on cardiovascular and all-cause mortality among the US population across different glycemic metabolic statuses using follow-up data from the National Health and Nutrition Examination Survey (NHANES) 1999-2018.
Methods
Data source
The data used in this analysis is publicly available through the NHANES database. NHANES, conducted by the National Center for Health Statistics (NCHS) of the Centers for Disease Control and Prevention, is an extensive health survey that includes a broad range of health and nutritional information of the U.S. population. This study employed a complex, multistage, stratified sampling design and received approval from the NCHS Research Ethics Review Board. All NHANES participants provided informed consent. As this study utilized de-identified public data, it was exempt from Institutional Review Board approval.
For the exploration of the impact of eGDR on cardiovascular and all-cause mortality rates among the U.S. population, we included 59,364 participants registered at NHANES mobile examination centers from 1999 to 2018 with subsequent follow-up information, extracting demographic, clinical examination, laboratory data, and survey data. Participants were excluded if they were: (1) under the age of 20 (n=4,419); (2) missing baseline WC information (n=5,671) or baseline HbA1c (n=1,934); (3) uncertain hypertension status at baseline (n=10); (4) uncertain glycemic metabolic status (n=1,314). A total of 46,016 participants were finally included in the study related to eGDR. The inclusion and exclusion processes were detailed in Figure 1.
Data collection and processing
First, we extracted general demographic information from the NHANES database’s demographic and questionnaire modules, such as age, gender, race, poverty income ratio, educational level, disease information [hypertension, congestive heart failure, coronary heart disease (CHD)], disease treatment information (antihypertensive therapy, hypoglycemic therapy, lipid-lowering therapy) and smoking/drinking status, which were obtained using standardized questionnaires by trained survey personnel. Educational levels were categorized as Less than 9th grade, 9-11th grade, High School Grad/General Educational Development or Equivalent, and College graduate or above. Smoking and drinking status were divided into three categories: never, former, and current (31).
From the NHANES laboratory data module, we retrieved relevant biochemical metabolic markers, including total cholesterol (TC), TG, high-density lipoprotein cholesterol (HDL-C), low-density lipoprotein cholesterol (LDL-C), alanine aminotransferase (ALT), aspartate aminotransferase (AST), uric acid (UA), estimated glomerular filtration rate (eGFR), and fasting plasma glucose (FPG).
Important anthropometric measurements such as weight, height, WC, systolic blood pressure (SBP), and diastolic blood pressure (DBP) were extracted from the NHANES examination data module. All fasting biochemical tests and anthropometric measurements were conducted according to standardized procedures in mobile examination centers or corresponding laboratories. Hypertension diagnosis was based on the 2022 American clinical guidelines for hypertension, defined as an average SBP≥130 mmHg or DBP≥80 mmHg, or current use of antihypertensive medication (32).
Glycemic metabolic status was defined according to the American Diabetes Association guidelines as normal fasting glucose (NFG), prediabetes, and diabetes (33), where diabetes includes self-reported diagnosis, use of insulin or oral hypoglycemic agents, FPG≥126 mg/dL or HbA1c level≥6.5%; prediabetes is diagnosed based on self-reported prediabetes or FPG between 100 mg/dL and 125 mg/dL or HbA1c between 5.7% and 6.4%; NFG is defined as FPG <100 mg/dL or HbA1c <5.7%.
Mortality ascertainment
Mortality data were obtained from death certificate records in the National Death Index (provided by NCHS), updated until December 31, 2019. The primary outcomes considered in our study included CVD-specific mortality and all-cause mortality (34).
eGDR calculation formula
The formula for calculating eGDR is as follows: eGDR = 21.158 − (0.09*WC) − (3.407*hypertension) − (0.551*HbA1c), where WC is in centimeters (cm), hypertension is coded as no=0/yes=1, and HbA1c is in percentage (%) (21).
Statistical analysis
In this study, statistical analyses were performed using Empower(R) version 4.2 and R language version 4.2.1. Consistent with NHANES data analysis guidelines, all analyses accounted for sample weights (35). In the baseline characteristics description, the study population was divided into four groups according to eGDR quartiles. Continuous variables were presented as survey-weighted means [95% confidence interval (CI)] and categorical variables as unweighted frequencies (weighted percentages); group differences were assessed using survey-weighted linear regression and survey-weighted Chi-square tests.
Before examining the impact of eGDR on cardiovascular and all-cause mortality rates among individuals with different glycemic metabolic statuses, we calculated the variance inflation factor for all variables using multiple linear regression equations and excluded any variables with a variance inflation factor≥5 from subsequent analyses (Supplementary Tables S1, S2) (36). Following the STROBE guidelines, we evaluated three progressively adjusted mult-class logistic regression models to analyze the association between eGDR and prediabetes and diabetes; Additionally, we also constructed three multivariable Cox proportional hazards regression models to explore the effects of eGDR on cardiovascular and all-cause mortality among the U.S. population, testing the proportional hazards assumption using Schoenfeld residuals. Model I adjusted for key demographic indicators (age, gender, race), poverty-income ratio, and education; Model II further adjusted for simple anthropometric parameters and laboratory biochemical markers (height, BMI, SBP, DBP, ALT, AST, HDL-C, eGFR, HbA1c, UA); Model III further adjusted for lifestyle habits and medical history [drinking status, smoking status, congestive heart failure, CHD]; Model IV, as the final model, included additional adjustments for disease treatment information (antihypertensive therapy, hypoglycemic therapy, lipid-lowering therapy) (37, 38).
After establishing an independent association between eGDR and mortality, we further assessed the differences in the association between eGDR and mortality rates across different glycemic metabolic statuses based on Model IV. Additionally, Kaplan-Meier survival curves were plotted to illustrate the cumulative incidence of cardiovascular and all-cause mortality across different levels of eGDR and glycemic statuses, with statistical differences evaluated using the log-rank test.
To visually observe the association between eGDR and mortality rates, a four-knot restricted cubic spline (RCS) was constructed based on Model IV to evaluate the impact of eGDR on cardiovascular and all-cause mortality rates across different glycemic metabolic statuses. Upon detecting potential nonlinear associations, segmented Cox regression was applied using a recursive algorithm to calculate the optimal threshold values for eGDR.
Finally, receiver operating characteristic (ROC) curves were plotted to predict cardiovascular and all-cause mortality rates among different glycemic metabolic statuses based on eGDR, with the area under the curve (AUC) calculated accordingly. All P-values were two-tailed, with significance set at P < 0.05.
Results
Baseline characteristics
In this study, a total of 46,016 participants were included, comprising 23,162 males and 22,854 females, with an average age of 50 years. Baseline characteristics summarized by eGDR quartiles (Table 1, Q1: ≤5.21; Q2: 5.21-7.05; Q3: 7.05-9.71; Q4: ≥9.71) revealed that compared to participants in the lowest quartile (Q1), those in the highest quartile (Q4) tended to be younger, had lower BMI, more optimal heights and weights, better blood pressure levels, and biochemical markers closer to normal ranges (including HDL-C, LDL-C, TC, TG, ALT, AST, eGFR, HbA1c, FPG, UA). Additionally, compared to Q1, there was a gradual decrease in the proportion of male participants and an increase in female participants in Q4; those in Q4 also had higher educational levels, were more likely to smoke and drink, had a lower proportion of antihypertensive, hypoglycemic, and lipid-lowering therapies, and fewer instances of congestive heart failure and CHD.
eGDR association with prediabetes and diabetes
Based on cross-sectional survey data, we analyzed the association of eGDR with prediabetes and diabetes through multi-class logistic regression. The results of the study showed that after fully adjusting for confounders, eGDR was negatively associated with both prediabetes and diabetes (Supplementary Table S3); however, this negative association with diabetes was comparatively weaker.
Follow-up results
During an average follow-up of 115 months, 6,906 participants (15.01%) experienced all-cause mortality, of which 1,798 deaths (3.91%) were attributed to cardiovascular causes. Compared to the lowest eGDR quartile (Q1), higher eGDR quartiles showed a gradual decrease in the proportion of cardiovascular and all-cause mortality (Supplementary Table S4). Further stratification by glycemic metabolic status revealed that individuals with diabetes had higher proportions of cardiovascular and all-cause mortality compared to those with NFG and prediabetes (Supplementary Table S5).
Kaplan-Meier survival curves illustrated by eGDR quartiles (Figure 2) demonstrated that as eGDR quartiles increased, the risks of cardiovascular and all-cause mortality progressively decreased. When stratified by glycemic metabolic status, those with NFG and prediabetes exhibited relatively lower risks of cardiovascular and all-cause mortality compared to individuals with diabetes (Figure 3).
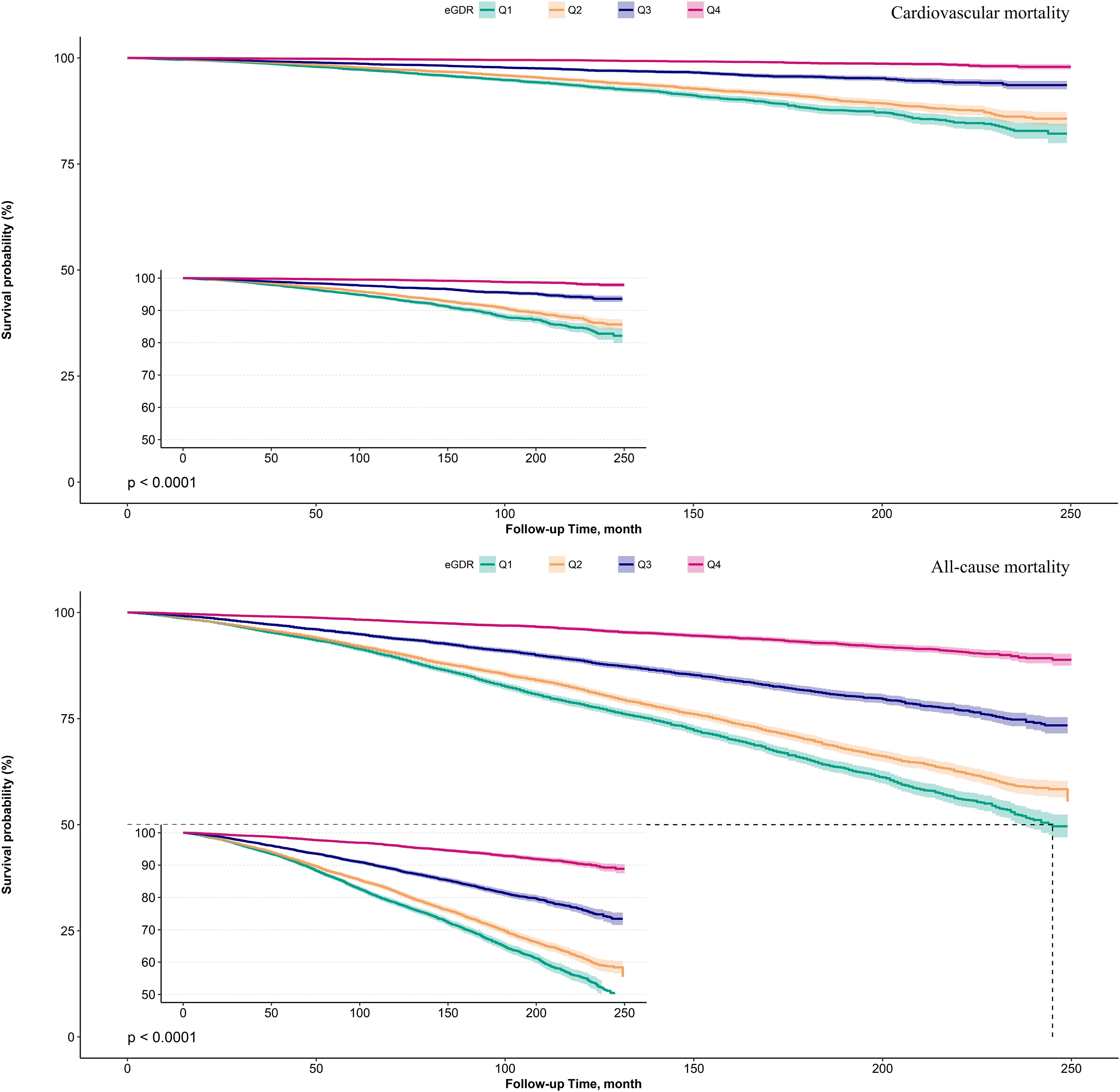
Figure 2. Survival curves showing cardiovascular mortality and all-cause mortality in the study population according to eGDR quartiles.
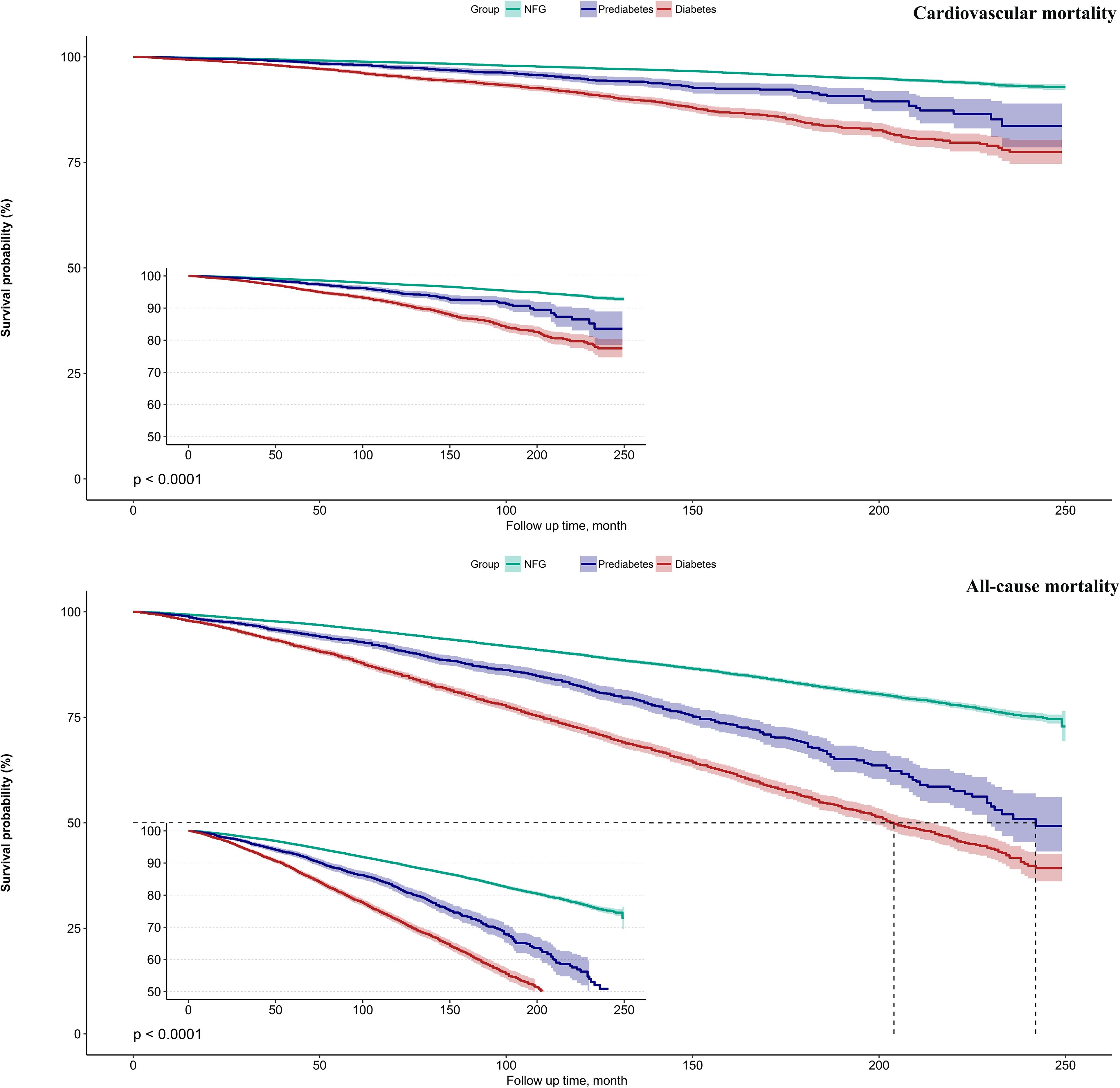
Figure 3. Survival curves showing cardiovascular mortality and all-cause mortality in the study population according to glycemic metabolic status.
Independent association between eGDR and mortality
Table 2 presents the associations between baseline eGDR and cardiovascular and all-cause mortality across three progressively adjusted Cox regression models. A consistent negative correlation was observed between eGDR and both cardiovascular and all-cause mortality across Models I-IV. In the fully adjusted model (Model IV), each unit increase in eGDR was associated with a 8% reduction in cardiovascular mortality risk [hazard ratio (HR): 0.92; 95%CI: 0.92, 0.93] and a 6% reduction in all-cause mortality risk (HR: 0.94; 95%CI: 0.94, 0.94). When eGDR was categorized, the negative correlation with cardiovascular and all-cause mortality progressively decreased from the lowest to the highest eGDR quartiles [Cardiovascular mortality: Q2 (HR: 1.01; 95%CI: 1.00, 1.01), Q3 (HR: 0.99; 95%CI: 0.98, 0.99), Q4 (HR: 0.78; 95%CI: 0.78, 0.78), P-trend <0.0001; All-cause mortality: Q2 (HR: 0.96; 95%CI: 0.96, 0.96), Q3 (HR: 1.00; 95%CI: 1.00, 1.00), Q4 (HR: 0.87; 95%CI: 0.87, 0.87), P-trend <0.0001].
Modifying effect of glycemic metabolic status
The modifying effect of glycemic metabolic status on the association between eGDR and cardiovascular and all-cause mortality was assessed. Table 3 shows that glycemic metabolic status significantly modified the protective effects of high eGDR against cardiovascular and all-cause mortality risks (P-interaction<0.0001); specifically, high eGDR provided stronger protection against cardiovascular and all-cause mortality risks in individuals with NFG and prediabetes compared to those with diabetes.
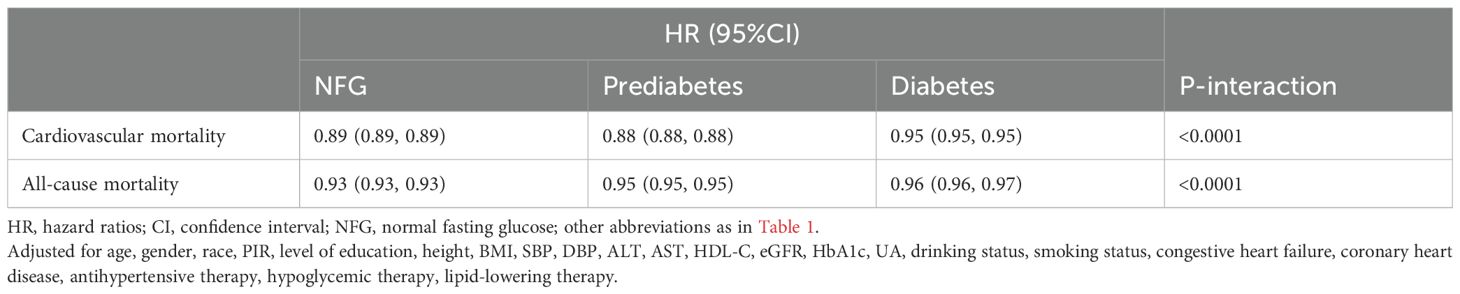
Table 3. Multivariable Cox regression analysis of the modifying effect of glycemic metabolic status on the association of eGDR-related cardiovascular mortality and all-cause mortality.
Predictive value of eGDR in different glycemic metabolic states
To further assess whether glycemic metabolic status influenced the predictive value of eGDR for cardiovascular and all-cause mortality, ROC curves were plotted, and AUC along with corresponding 95% CIs, optimal thresholds, sensitivity, and specificity were calculated (Table 4). The comparison of AUCs across different glycemic metabolic statuses revealed that eGDR had a higher predictive value for cardiovascular and all-cause mortality in individuals with NFG and prediabetes compared to those with diabetes (Figure 4); however, it should still be noted that in predicting death, the AUC of eGDR was less than 0.7 in all glycemic status groups.
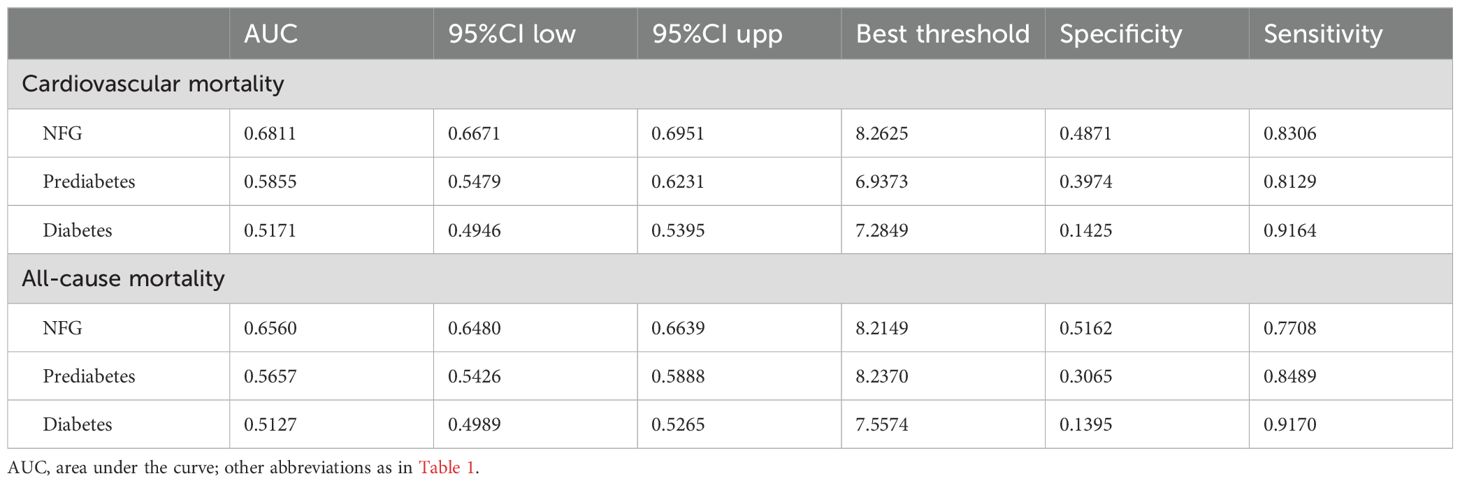
Table 4. ROC analysis of the predictive value of eGDR for cardiovascular mortality and all-cause mortality under different glucose metabolism status.
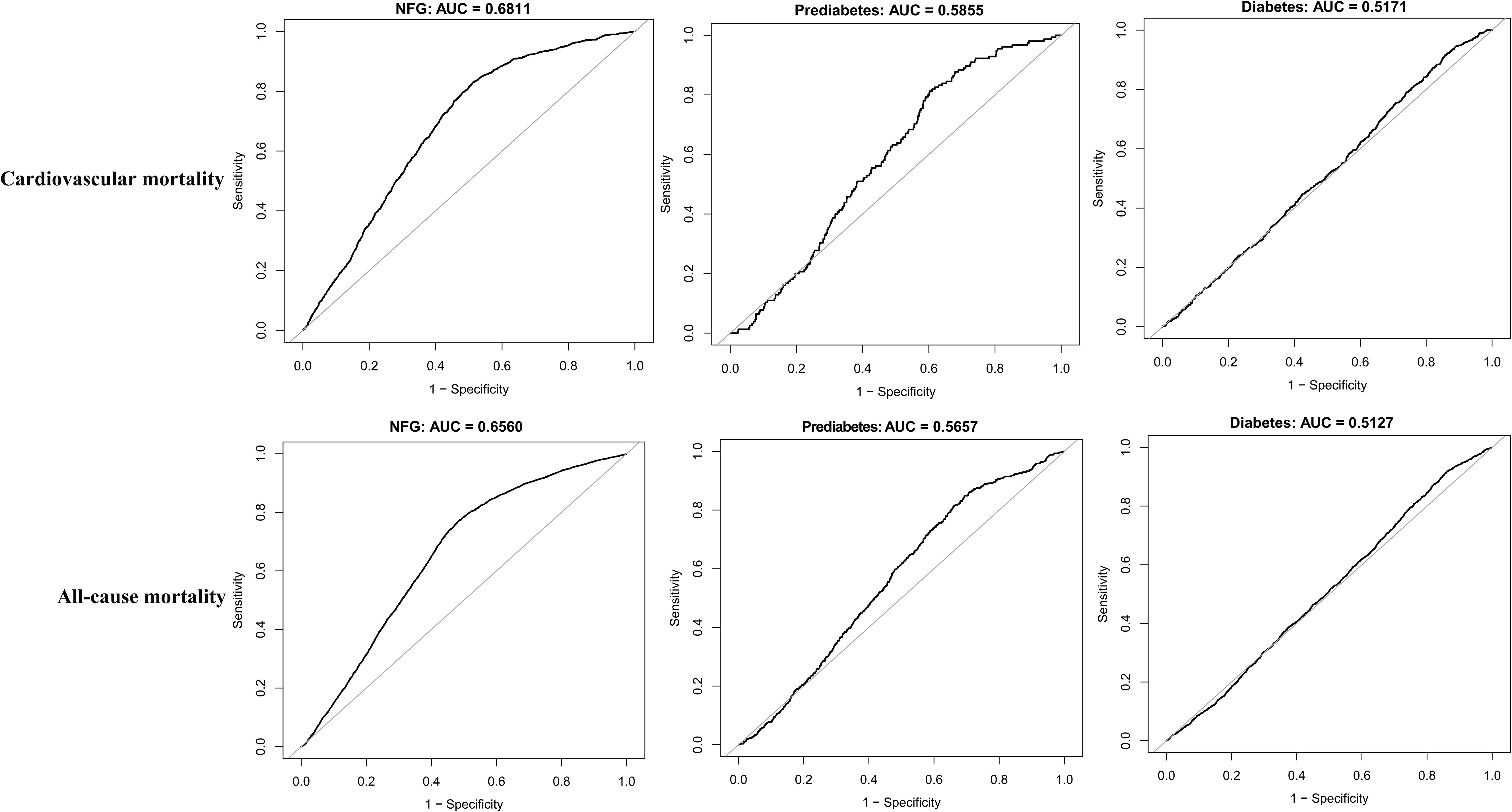
Figure 4. ROC curve analyzes the predictive value of eGDR for cardiovascular mortality and all-cause mortality under different glycemic metabolic statuses.
Dose-response relationship between eGDR and mortality
The dose-response relationship between eGDR and mortality was further analyzed using RCS. Results indicated a nonlinear association between eGDR and both cardiovascular and all-cause mortality in the NFG population; a similar nonlinear association was also observed for all-cause mortality in individuals with diabetes (Figure 5, P for nonlinearity<0.05). Segmental Cox regression identified eGDR thresholds for all-cause and cardiovascular mortality in the NFG population as 5.19 and 4.45, respectively, and 4.65 for all-cause mortality in the diabetes group (Table 5).
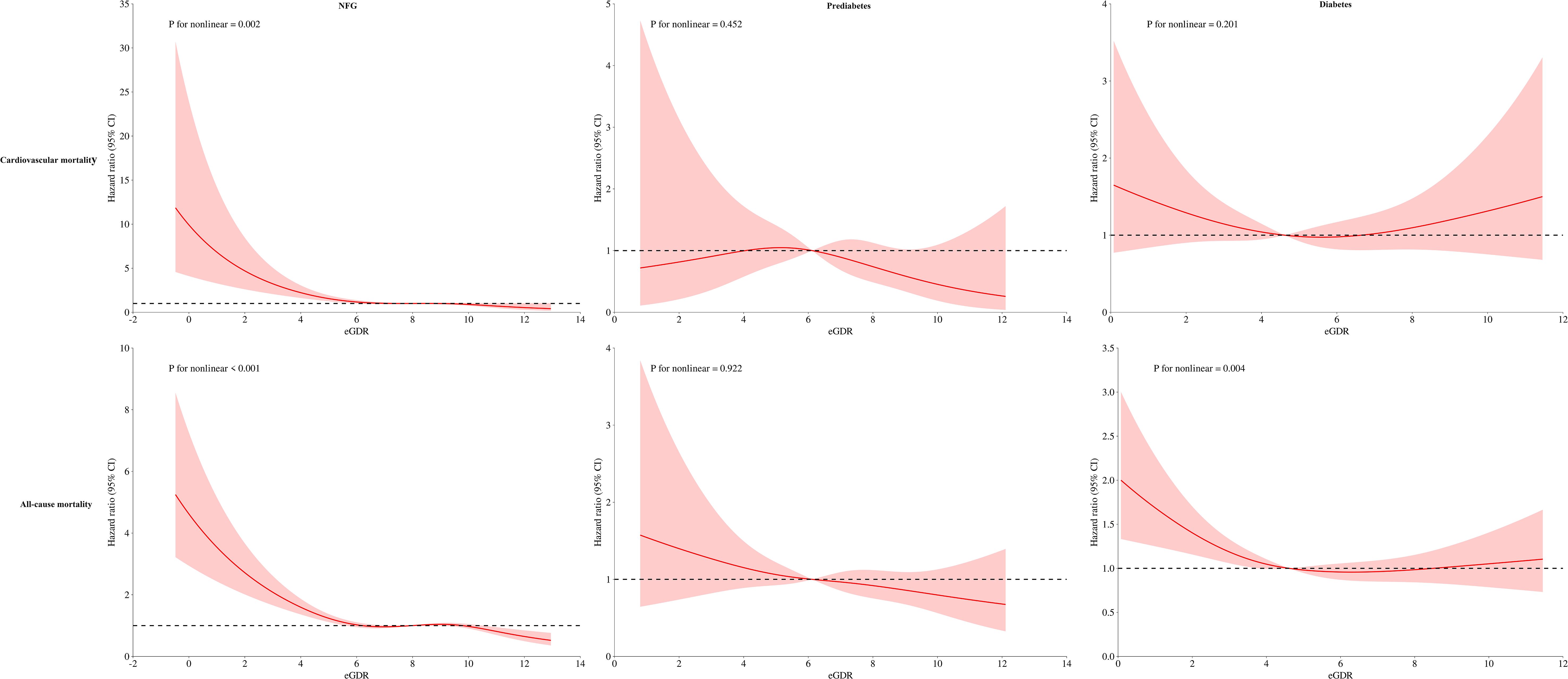
Figure 5. Dose-response relationship curves between eGDR and cardiovascular mortality and all-cause mortality under different glycemic metabolic statuses.
Discussion
Our study is one of the first to evaluate the impact of eGDR on cardiovascular and all-cause mortality risks across different glycemic metabolic statuses. The key findings can be summarized as follows: (1) Higher eGDR serves as a protective factor against both cardiovascular and all-cause mortality, with glycemic metabolic status significantly modulating this protective effect. Specifically, high eGDR offers stronger protective benefits against mortality risks in individuals with NFG and prediabetes compared to those with diabetes. (2) RCS analysis revealed a nonlinear relationship between eGDR and both cardiovascular and all-cause mortality in the NFG population; a similar nonlinear association was also noted in diabetic patients. (3) eGDR demonstrates greater accuracy in predicting cardiovascular and all-cause mortality in individuals with NFG and prediabetes than in those with diabetes.
IR is characterized by a decreased sensitivity and responsiveness to insulin, leading to impaired glucose utilization and subsequent metabolic abnormalities such as hyperglycemia (11). Numerous studies have established a close association between IR and the onset of cardiovascular diseases and strokes (11–13), as well as influencing the prognosis of various diseases (14–17). Our findings reinforce the notion that the surrogate marker for IR, eGDR, is closely linked to long-term cardiovascular and all-cause mortality. The biological mechanisms underlying the association between eGDR and mortality risks are not entirely clear, but insights can be gleaned from the adverse outcomes associated with IR: (1) Chronic IR can lead to a cascade of metabolic dysfunctions that cause damage to multiple organ systems, increasing the risks of cardiovascular and all-cause mortality (39); (2) The oxidative stress and inflammatory responses induced by IR can further elevate these risks (40); (3) IR can also lead to neuroendocrine dysregulation, such as the overactivation of the RAAS and sympathetic nervous systems, thereby increasing cardiac workload and impacting cardiovascular mortality risk (41).
As a surrogate for IR, eGDR integrates indices related to glucose, blood pressure, and obesity, providing a comprehensive assessment of IR (21). Previous research has demonstrated that low eGDR is associated with diabetic complications such as peripheral neuropathy (42), retinopathy (43), and progression of kidney diseases (44). Recent studies have further linked low eGDR with an increased risk of stroke and adverse cardiovascular and all-cause mortality events. For example, Zabala et al. found that a low eGDR significantly increased the risk of stroke and mortality in type 2 diabetes (25). Similar findings have been reported in populations with type 1 diabetes (T1DM), where low eGDR was associated with increased mortality (27), as well as in studies by Kong, Penno, and Olson (26, 28, 29). Our results corroborate these earlier findings, supporting the notion that low eGDR is a significant risk factor for cardiovascular and all-cause mortality.
In recent years, the focus of research has gradually shifted toward the impact of glycemic metabolic status. However, clinical understanding of different glycemic metabolic states remains limited due to a scarcity of studies (45, 46). From the perspective of time change trends, blood glucose metabolism status can be roughly divided into two categories: short-term changes and long-term status. In terms of short-term changes, the stress hyperglycemia ratio (SHR) may be a good parameter to study. Related studies have also reported some SHR-related research results (47, 48); For example, Lai et al. examined the association between SHR and adverse chronic kidney disease outcomes in adults with diabetes in the United States. They found that either too low or too high SHR levels were significantly associated with adverse renal outcomes in the diabetic population (47). A similar finding was reported in a recent study by Tian et al., who reported a U-shaped association of SHR with mortality in patients with cardiogenic shock (48). Additionally, hyperglycemia itself can have adverse effects on the body, it can be debilitating in older people (49), and can affect normal fertility in women of childbearing age (50). This may be related to the inflammatory reaction or hormonal changes secondary to hyperglycemia, which in turn affects the thickness of the endometrium and causes infertility (50), and even affects the therapeutic effect of intrauterine insemination (51). For the long-term status of blood glucose metabolism, this requires comprehensive assessment and diagnosis based on relatively comprehensive blood glucose measurement information to determine the classification of the blood glucose status of the study population: the main categories are normoglycemic state, prediabetic state and diabetic state. In general, studies on glucose metabolism status that have been completed in the past have focused on metabolism-related diseases, such as the impact of glycemic control on carotid atherosclerosis plaque (52, 53) and CHD (54, 55). Furthermore, Wu et al. found that glycemic metabolic status could modulate the influence of atherosclerotic indices on CHD (56), while Zhang et al. discovered that the SHR was significantly related to the risk and predicted severity of multi-vessel CHD, especially in individuals with prediabetes and diabetes (57). These findings underscore the potential clinical significance of incorporating glycemic metabolic status into practice.
Our study further explores the association between eGDR and mortality risks across different glycemic statuses, finding a more pronounced negative correlation between eGDR and mortality risks in NFG and prediabetes compared to diabetes. This suggests that, at the same high level of eGDR, the mortality risk reduction benefits are relatively smaller for diabetic patients. This may be due to the higher complication risks associated with diabetes, which increase cardiovascular and overall mortality risks (58–60). Additionally, diabetes, as a strong exposure risk factor, increases the risks of cardiovascular and all-cause mortality (9, 61). Furthermore, it should be noted that in the current study, we also found that eGDR was negatively correlated with both prediabetes and diabetes risk; in contrast, high levels of eGDR may have a weaker protective effect on diabetes. In view of this finding, we consider that the weak protective effect of high eGDR on diabetes may further persist into the poor prognosis of diabetic patients. Based on the above correlation analysis, the current study also assesses the predictive value of eGDR for these risks, showing more accurate predictions in NFG and prediabetes than in diabetes. The integration of correlation analysis and ROC findings suggests that high eGDR provides enhanced protection and predictive value for populations with preferred glycemic metabolism.
Further RCS analysis revealed interesting results: eGDR demonstrated a nonlinear, L-shaped relationship with mortality risks in the NFG (for cardiovascular and all-cause mortality) and diabetic (for all-cause mortality) populations. The RCS model indicated that as eGDR levels incrementally increase, the risks of cardiovascular and all-cause mortality progressively decreased, stabilizing once eGDR surpassed a certain threshold. Similar L-shaped relationships were previously reported in a study on a Swiss T1DM population by Nyström et al., where the threshold for eGDR was identified to be between 8-10, beyond which all-cause mortality risks stabilized (27). Although the threshold values from Nyström et al.’s study slightly differ from ours, likely due to population and disease type differences, the overall trends align with our findings, underscoring the nuanced interactions between eGDR levels and health outcomes.
The findings of the current study have implications for daily clinical practice. Given the NHANES sampling and weighting methods, the findings are highly applicable to the general population. From the perspective of eGDR development background, eGDR is obtained through a comprehensive assessment of hypertension, HbA1c and obesity. It is easy and convenient to obtain and can quickly understand the IR status of the population. Therefore, the clinical significance of the current study is to offer a simple surrogate for IR to predict poor prognosis across various glycemic statuses. In addition, the evidence from the current study can also provide useful research materials for subsequent further studies. Considering the limited value of eGDR for mortality prediction in the population of all glycemic states in the current study, we suggest incorporating eGDR as an add-on in future studies to develop more accurate prediction models.
Strengths and limitations
There are several strengths of the current study worth mentioning: (1) This study is the first to explore the effects of eGDR on cardiovascular and all-cause mortality risks across different glycemic metabolic statuses. (2) The NHANES national database, designed through a complex multistage probability sampling and controlling for potential confounders in multivariable models, lends reliability to the findings.
This article still has some shortcomings: (1) This study was conducted solely within the U.S. population, and further research is needed to determine whether the results can be generalized to other populations. (2) The endpoint events were obtained from NCHS, and there might be a delay in the recording of some mortality cases, potentially leading to an underestimation of actual mortality risks. (3) This was not a predefined analysis; such observational studies are susceptible to potential biases and confounding factors. (4) Some data were inevitably missing from the surveys, leading to the exclusion of some samples from the analysis, which could introduce selection bias. Furthermore, to ensure a viable sample size for analysis, variables with a high level of missing data such as TG, LDL-C, and FPG (over 50%) were excluded from the multivariable models [see Supplementary Table S6 for missing information], which may introduce some selection bias. However, the larger sample size used in the analyses helps to reinforce and support the study results.
Conclusion
In the U.S. adult population, high eGDR acts as a protective factor against cardiovascular and all-cause mortality, with glycemic metabolic status significantly modifying this protective effect. Specifically, high eGDR provides stronger protection for individuals with NFG and prediabetes. It is also noteworthy that eGDR appears to be more applicable for predicting mortality in populations with NFG and prediabetes, suggesting its potential utility in clinical settings for these groups. Future research should aim to explore these relationships in diverse populations and settings to validate and extend these findings.
Data availability statement
The raw data supporting the conclusions of this article will be made available by the authors, without undue reservation.
Ethics statement
The studies involving humans were approved by NCHS Research Ethics Review Board. The studies were conducted in accordance with the local legislation and institutional requirements. The participants provided their written informed consent to participate in this study.
Author contributions
SH: Investigation, Software, Writing – original draft. CW: Investigation, Writing – original draft. XH: Investigation, Writing – original draft. GJ: Investigation, Writing – review & editing. ZL: Investigation, Writing – review & editing. KJ: Investigation, Writing – review & editing. GX: Formal analysis, Investigation, Validation, Writing – review & editing. GS: Conceptualization, Formal analysis, Methodology, Project administration, Supervision, Validation, Writing – review & editing. YZ: Conceptualization, Formal analysis, Investigation, Methodology, Project administration, Software, Supervision, Validation, Writing – review & editing.
Funding
The author(s) declare financial support was received for the research, authorship, and/or publication of this article. This work was supported by the Natural Science Foundation of Jiangxi Province (No. 20232BAB216004).
Acknowledgments
We would like to thank the NHANES project team for their great efforts throughout the project.
Conflict of interest
The authors declare that the research was conducted in the absence of any commercial or financial relationships that could be construed as a potential conflict of interest.
Publisher’s note
All claims expressed in this article are solely those of the authors and do not necessarily represent those of their affiliated organizations, or those of the publisher, the editors and the reviewers. Any product that may be evaluated in this article, or claim that may be made by its manufacturer, is not guaranteed or endorsed by the publisher.
Supplementary material
The Supplementary Material for this article can be found online at: https://www.frontiersin.org/articles/10.3389/fendo.2024.1494820/full#supplementary-material
References
1. Roth GA, Mensah GA, Johnson CO, Addolorato G, Ammirati E, Baddour LM, et al. Global burden of cardiovascular diseases and risk factors, 1990–2019: update from the GBD 2019 study. J Am Coll Cardiol. (2020) 76:2982–3021. doi: 10.1016/j.jacc.2020.11.010
2. GBD 2019 Risk Factors Collaborators. Global burden of 87 risk factors in 204 countries and territories, 1990-2019: a systematic analysis for the Global Burden of Disease Study 2019. Lancet. (2020) 396:1223–49. doi: 10.1016/S0140-6736(20)30752-2
3. Weir HK, Anderson RN, Coleman King SM, Soman A, Thompson TD, Hong Y, et al. Heart disease and cancer deaths - trends and projections in the United States, 1969-2020. Prev Chronic Dis. (2016) 13:E157. doi: 10.5888/pcd13.160211
4. Xu J, Murphy SL, Kochanek KD, Arias E. Mortality in the United States, 2015. NCHS Data Brief. (2016) 267):1–8.
5. Chisholm-Burns MA, Kim Lee J, Spivey CA, Slack M, Herrier RN, Hall-Lipsy E, et al. US pharmacists' effect as team members on patient care: systematic review and meta-analyses. Med Care. (2010) 48:923–33. doi: 10.1097/MLR.0b013e3181e57962
6. Powell-Wiley TM, Poirier P, Burke LE, Després JP, Gordon-Larsen P, Lavie CJ, et al. Obesity and cardiovascular disease: A scientific statement from the american heart association. Circulation. (2021) 143:e984–e1010. doi: 10.1161/CIR.0000000000000973
7. Grundy SM, Stone NJ, Bailey AL, Beam C, Birtcher KK, Blumenthal RS, et al. 2018 AHA/ACC/AACVPR/AAPA/ABC/ACPM/ADA/AGS/APhA/ASPC/NLA/PCNA guideline on the management of blood cholesterol: A report of the american college of cardiology/american heart association task force on clinical practice guidelines. Circulation. (2019) 139:e1082–143. doi: 10.1161/CIR.0000000000000625
8. Whelton PK, Carey RM, Aronow WS, Casey DE Jr, Collins KJ, Dennison Himmelfarb C, et al. 2017 ACC/AHA/AAPA/ABC/ACPM/AGS/APhA/ASH/ASPC/NMA/PCNA guideline for the prevention, detection, evaluation, and management of high blood pressure in adults: A report of the american college of cardiology/american heart association task force on clinical practice guidelines. Circulation. (2018) 138:e484–594. doi: 10.1161/CIR.0000000000000596
9. Joseph JJ, Deedwania P, Acharya T, Aguilar D, Bhatt DL, Chyun DA, et al. Comprehensive management of cardiovascular risk factors for adults with type 2 diabetes: A scientific statement from the american heart association. Circulation. (2022) 145:e722–59. doi: 10.1161/CIR.0000000000001040
10. Yusuf S, Joseph P, Rangarajan S, Islam S, Mente A, Hystad P, et al. Modifiable risk factors, cardiovascular disease, and mortality in 155 722 individuals from 21 high-income, middle-income, and low-income countries (PURE): a prospective cohort study. Lancet. (2020) 395:795–808. doi: 10.1016/S0140-6736(19)32008-2
11. Zhang Z, Zhao L, Lu Y, Meng X, Zhou X. Association between non-insulin-based insulin resistance indices and cardiovascular events in patients undergoing percutaneous coronary intervention: a retrospective study. Cardiovasc Diabetol. (2023) 22:161. doi: 10.1186/s12933-023-01898-1
12. Zhou M, Li H, Wang Y, Pan Y, Wang Y. Causal effect of insulin resistance on small vessel stroke and Alzheimer's disease: A Mendelian randomization analysis. Eur J Neurol. (2022) 29:698–706. doi: 10.1111/ene.15190
13. Ormazabal V, Nair S, Elfeky O, Aguayo C, Salomon C, Zuñiga FA. Association between insulin resistance and the development of cardiovascular disease. Cardiovasc Diabetol. (2018) 17:122. doi: 10.1186/s12933-018-0762-4
14. Ausk KJ, Boyko EJ, Ioannou GN. Insulin resistance predicts mortality in nondiabetic individuals in the U. S Diabetes Care. (2010) 33:1179–85. doi: 10.2337/dc09-2110
15. Pan K, Chlebowski RT, Mortimer JE, Gunther MJ, Rohan T, Vitolins MZ, et al. Insulin resistance and breast cancer incidence and mortality in postmenopausal women in the Women's Health Initiative. Cancer. (2020) 126:3638–47. doi: 10.1002/cncr.33002
16. de Boer IH, Katz R, Chonchol MB, Fried LF, Ix JH, Kestenbaum B, et al. Insulin resistance, cystatin C, and mortality among older adults. Diabetes Care. (2012) 35:1355–60. doi: 10.2337/dc11-1657
17. Lee JH, Jeon S, Joung B, Lee HS, Kwon YJ. Associations of homeostatic model assessment for insulin resistance trajectories with cardiovascular disease incidence and mortality. Arterioscler Thromb Vasc Biol. (2023) 43:1719–28. doi: 10.1161/ATVBAHA.123.319200
18. DeFronzo RA, Tobin JD, Andres R. Glucose clamp technique: a method for quantifying insulin secretion and resistance. Am J Physiol. (1979) 237:E214–23. doi: 10.1152/ajpendo.1979.237.3.E214
19. Matthews DR, Hosker JP, Rudenski AS, Naylor BA, Treacher DF, Turner RC. Homeostasis model assessment: insulin resistance and beta-cell function from fasting plasma glucose and insulin concentrations in man. Diabetologia. (1985) 28:412–9. doi: 10.1007/BF00280883
20. Alizargar J, Bai CH, Hsieh NC, Wu SV. Use of the triglyceride-glucose index (TyG) in cardiovascular disease patients. Cardiovasc Diabetol. (2020) 19:8. doi: 10.1186/s12933-019-0982-2
21. Williams KV, Erbey JR, Becker D, Arslanian S, Orchard TJ. Can clinical factors estimate insulin resistance in type 1 diabetes? Diabetes. (2000) 49:626–32. doi: 10.2337/diabetes.49.4.626
22. Kozawa J, Iwahashi H, Okita K, Okauchi Y, Imagawa A, Shimomura I. Insulin tolerance test predicts the effectiveness of insulin sensitizers in Japanese type 2 diabetic patients. Diabetes Ther. (2010) 1:121–30. doi: 10.1007/s13300-010-0011-7
23. van der Aa MP, Elst MA, van de Garde EM, van Mil EG, Knibbe CA, van der Vorst MM. Long-term treatment with metformin in obese, insulin-resistant adolescents: results of a randomized double-blinded placebo-controlled trial. Nutr Diabetes. (2016) 6:e228. doi: 10.1038/nutd.2016.37
24. Liu C, Liu X, Ma X, Cheng Y, Sun Y, Zhang D, et al. Predictive worth of estimated glucose disposal rate: evaluation in patients with non-ST-segment elevation acute coronary syndrome and non-diabetic patients after percutaneous coronary intervention. Diabetol Metab Syndr. (2022) 14:145. doi: 10.1186/s13098-022-00915-9
25. Zabala A, Darsalia V, Lind M, Svensson AM, Franzén S, Eliasson B, et al. Estimated glucose disposal rate and risk of stroke and mortality in type 2 diabetes: a nationwide cohort study. Cardiovasc Diabetol. (2021) 20:202. doi: 10.1186/s12933-021-01394-4
26. Kong X, Wang W. Estimated glucose disposal rate and risk of cardiovascular disease and mortality in U.S. adults with prediabetes: a nationwide cross-sectional and prospective cohort study. Acta Diabetol. (2024) 61:1413–21. doi: 10.1007/s00592-024-02305-1
27. Nyström T, Holzmann MJ, Eliasson B, Svensson AM, Sartipy U. Estimated glucose disposal rate predicts mortality in adults with type 1 diabetes. Diabetes Obes Metab. (2018) 20:556–63. doi: 10.1111/dom.13110
28. Penno G, Solini A, Orsi E, Bonora E, Fondelli C, Trevisan R, et al. Insulin resistance, diabetic kidney disease, and all-cause mortality in individuals with type 2 diabetes: a prospective cohort study. BMC Med. (2021) 19:66. doi: 10.1186/s12916-021-01936-3
29. Olson JC, Erbey JR, Williams KV, Becker DJ, Edmundowicz D, Kelsey SF, et al. Subclinical atherosclerosis and estimated glucose disposal rate as predictors of mortality in type 1 diabetes. Ann Epidemiol. (2002) 12:331–7. doi: 10.1016/S1047-2797(01)00269-1
30. Garofolo M, Gualdani E, Scarale MG, Bianchi C, Aragona M, Campi F, et al. Insulin resistance and risk of major vascular events and all-cause mortality in type 1 diabetes: a 10-year follow-up study. Diabetes Care. (2020) 43:e139–41. doi: 10.2337/dc20-0433
31. Leaks K, Norden-Krichmar T, Brody JP. Predicting moderate drinking behaviors in National Health and Nutrition Examination Survey participants using biochemical and demographical factors with machine learning. Alcohol. (2023) 113:1–10. doi: 10.1016/j.alcohol.2023.07.005
32. Whelton PK, Carey RM, Mancia G, Kreutz R, Bundy JD, Williams B. Harmonization of the american college of cardiology/american heart association and european society of cardiology/european society of hypertension blood pressure/hypertension guidelines: comparisons, reflections, and recommendations. Circulation. (2022) 146:868–77. doi: 10.1161/CIRCULATIONAHA.121.054602
33. American Diabetes Association. 2. Classification and diagnosis of diabetes: standards of medical care in diabetes-2018. Diabetes Care. (2018) 41:S13–27. doi: 10.2337/dc18-S002
34. International Statistical Classification of Diseases and Related Health Problems. 5th ed. Geneva, Switzerland: World Health Organization (2016). 10th revision.
35. NHANES Survey Methods and Analytic Guidelines. Available online at: https://wwwn.cdc.gov/nchs/nhanes/analyticguidelines.aspx (Accessed 9 June 2024).
36. Kim JH. Multicollinearity and misleading statistical results. Korean J Anesthesiol. (2019) 72:558–69. doi: 10.4097/kja.19087
37. Tang B, Li X, Wang Y, Sjölander A, Johnell K, Thambisetty M, et al. Longitudinal associations between use of antihypertensive, antidiabetic, and lipid-lowering medications and biological aging. Geroscience. (2023) 45:2065–78. doi: 10.1007/s11357-023-00784-8
38. Santulli G, Visco V, Varzideh F, Guerra G, Kansakar U, Gasperi M, et al. Prediabetes increases the risk of frailty in prefrail older adults with hypertension: beneficial effects of metformin. Hypertension. (2024) 81:1637–43. doi: 10.1161/HYPERTENSIONAHA.124.23087
39. Chen W, Wang X, Chen J, You C, Ma L, Zhang W, et al. Household air pollution, adherence to a healthy lifestyle, and risk of cardiometabolic multimorbidity: results from the China health and retirement longitudinal study. Sci Total Environ. (2023) 855:158896. doi: 10.1016/j.scitotenv.2022.158896
40. Mastrototaro L, Roden M. Insulin resistance and insulin sensitizing agents. Metabolism. (2021) 125:154892. doi: 10.1016/j.metabol.2021.154892
41. Jia G, Whaley-Connell A, Sowers JR. Diabetic cardiomyopathy: a hyperglycaemia- and insulin-resistance-induced heart disease. Diabetologia. (2018) 61:21–8. doi: 10.1007/s00125-017-4390-4
42. Zhang Y, Sun W, Zhang Q, Bai Y, Ji L, Zheng H, et al. Estimated glucose disposal rate predicts the risk of diabetic peripheral neuropathy in type 2 diabetes: A 5-year follow-up study. J Diabetes. (2024) 16:e13482. doi: 10.1111/1753-0407.13482
43. Linn W, Persson M, Rathsman B, Ludvigsson J, Lind M, Andersson Franko M, et al. Estimated glucose disposal rate is associated with retinopathy and kidney disease in young people with type 1 diabetes: a nationwide observational study. Cardiovasc Diabetol. (2023) 22:61. doi: 10.1186/s12933-023-01791-x
44. Peng J, Li A, Yin L, Yang Q, Pan J, Yi B. Estimated glucose disposal rate predicts renal progression in type 2 diabetes mellitus: A retrospective cohort study. J Endocr Soc. (2023) 7:bvad069. doi: 10.1210/jendso/bvad069
45. Rooney MR, Fang M, Ogurtsova K, Ozkan B, Echouffo-Tcheugui JB, Boyko EJ, et al. Global prevalence of prediabetes. Diabetes Care. (2023) 46:1388–94. doi: 10.2337/dc22-2376
46. Cui J, Sun J, Wang W, Yasmeen N, Ke M, Xin H, et al. Triglycerides and total cholesterol concentrations in association with IFG/IGT in Chinese adults in Qingdao, China. BMC Public Health. (2018) 18:444. doi: 10.1186/s12889-018-5286-z
47. Lai W, Meng Y, Zhou Y, Zhang T, Zhang B, Huang Z, et al. Association of stress hyperglycemia ratio with presence and severity of chronic kidney disease among US adults with diabetes mellitus. Front Endocrinol (Lausanne). (2024) 15:1446390. doi: 10.3389/fendo.2024.1446390
48. Tian J, Zhou T, Liu Z, Dong Y, Xu H. Stress hyperglycemia is associated with poor prognosis in critically ill patients with cardiogenic shock. Front Endocrinol (Lausanne). (2024) 15:1446714. doi: 10.3389/fendo.2024.1446714
49. Mone P, De Gennaro S, Frullone S, Marro A, Santulli G. Hyperglycemia drives the transition from pre-frailty to frailty: The Monteforte study. Eur J Intern Med. (2023) 111:135–7. doi: 10.1016/j.ejim.2023.01.006
50. Huniadi A, Zaha IA, Naghi P, Stefan L, Sachelarie L, Bodog A, et al. Autologous platelet-rich plasma (PRP) efficacy on endometrial thickness and infertility: A single-centre experience from Romania. Med (Kaunas). (2023) 59:1532. doi: 10.3390/medicina59091532
51. Huniadi A, Bimbo-Szuhai E, Botea M, Zaha I, Beiusanu C, Pallag A, et al. Fertility predictors in intrauterine insemination (IUI). J Pers Med. (2023) 13:395. doi: 10.3390/jpm13030395
52. Yang T, Zheng H, Pan G, Guo R, Liu F, Liu S, et al. Relationship between the circulating N-terminal pro B-type natriuretic peptide and the risk of carotid artery plaque in different glucose metabolic states in patients with coronary heart disease: a CSCD-TCM plus study in China. Cardiovasc Diabetol. (2023) 22:299. doi: 10.1186/s12933-023-02015-y
53. Li Z, He Y, Wang S, Li L, Yang R, Liu Y, et al. Association between triglyceride glucose index and carotid artery plaque in different glucose metabolic states in patients with coronary heart disease: a RCSCD-TCM study in China. Cardiovasc Diabetol. (2022) 21:38. doi: 10.1186/s12933-022-01470-3
54. Su J, Li Z, Huang M, Wang Y, Yang T, Ma M, et al. Triglyceride glucose index for the detection of the severity of coronary artery disease in different glucose metabolic states in patients with coronary heart disease: a RCSCD-TCM study in China. Cardiovasc Diabetol. (2022) 21:96. doi: 10.1186/s12933-022-01523-7
55. Zhuang Y, Qiu L, Han D, Qiao Z, Wang F, Jiang Q, et al. The association between triglyceride-glucose index and related parameters and risk of cardiovascular disease in American adults under different glucose metabolic states. Diabetol Metab Syndr. (2024) 16:102. doi: 10.1186/s13098-024-01340-w
56. Wu X, Qiu W, Yang H, Chen YJ, Liu J, Zhao G. Associations of the triglyceride-glucose index and atherogenic index of plasma with the severity of new-onset coronary artery disease in different glucose metabolic states. Cardiovasc Diabetol. (2024) 23:76. doi: 10.1186/s12933-024-02163-9
57. Zhang Y, Song H, Bai J, Xiu J, Wu G, Zhang L, et al. Association between the stress hyperglycemia ratio and severity of coronary artery disease under different glucose metabolic states. Cardiovasc Diabetol. (2023) 22:29. doi: 10.1186/s12933-023-01759-x
58. Dal Canto E, Ceriello A, Rydén L, Ferrini M, Hansen TB, Schnell O, et al. Diabetes as a cardiovascular risk factor: an overview of global trends of macro and micro vascular complications. Eur J Prev Cardiol. (2019) 26:25–32. doi: 10.1177/2047487319878371
59. Zhang P, Guo D, Ceriello A, Rydén L, Ferrini MW, Liu W, et al. Association of serum 25-hydroxyvitamin D with cardiovascular outcomes and all-cause mortality in individuals with prediabetes and diabetes: results from the UK biobank prospective cohort study. Diabetes Care. (2022) 45:1219–29. doi: 10.2337/dc21-2193
60. Harding JL, Pavkov ME, Magliano DJ, Shaw JE, Gregg EW. Global trends in diabetes complications: a review of current evidence. Diabetologia. (2019) 62:3–16. doi: 10.1007/s00125-018-4711-2
Keywords: insulin resistance, estimated glucose disposal rate, cardiovascular mortality, eGDR, all-cause mortality
Citation: He S, Wang C, Huang X, Jian G, Lu Z, Jiang K, Xie G, Sheng G and Zou Y (2025) Analyzing the impact of glycemic metabolic status on cardiovascular mortality and all-cause mortality related to the estimated glucose disposal rate: a nationwide cohort study. Front. Endocrinol. 15:1494820. doi: 10.3389/fendo.2024.1494820
Received: 11 September 2024; Accepted: 31 December 2024;
Published: 21 January 2025.
Edited by:
Changting Xiao, University of Saskatchewan, CanadaReviewed by:
Cosmin Mihai Vesa, University of Oradea, RomaniaFrancesco Spione, Montevergine Clinic, Italy
Copyright © 2025 He, Wang, Huang, Jian, Lu, Jiang, Xie, Sheng and Zou. This is an open-access article distributed under the terms of the Creative Commons Attribution License (CC BY). The use, distribution or reproduction in other forums is permitted, provided the original author(s) and the copyright owner(s) are credited and that the original publication in this journal is cited, in accordance with accepted academic practice. No use, distribution or reproduction is permitted which does not comply with these terms.
*Correspondence: Guotai Sheng, dGdzMjAwNTA5QDE2My5jb20=; Yang Zou, anh5eHl6eUAxNjMuY29t
†These authors have contributed equally to this work and share first authorship