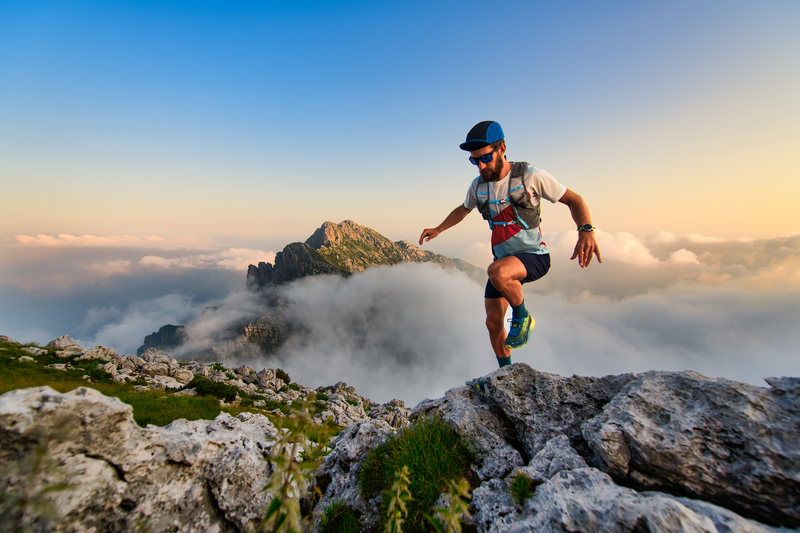
94% of researchers rate our articles as excellent or good
Learn more about the work of our research integrity team to safeguard the quality of each article we publish.
Find out more
ORIGINAL RESEARCH article
Front. Endocrinol. , 28 October 2024
Sec. Diabetes: Molecular Mechanisms
Volume 15 - 2024 | https://doi.org/10.3389/fendo.2024.1473329
Introduction: We investigated the genetic determinants of variation in the hemoglobin glycation index (HGI), an emerging biomarker for the risk of diabetes complications.
Methods: We conducted a genome-wide association study (GWAS) for HGI in the Action to Control Cardiovascular Risk in Diabetes (ACCORD) trial (N = 7,913) using linear regression and additive genotype encoding on variants with minor allele frequency greater than 3%. We conducted replication analyses of top findings in the Atherosclerosis Risk in Communities (ARIC) study with inverse variance-weighted meta-analysis. We followed up with stratified GWAS analyses by sex and self-reported race.
Results: In ACCORD, we identified single nucleotide polymorphisms (SNPs) associated with HGI, including a peak with the strongest association at the intergenic SNP rs73407935 (7q11.22) (P = 5.8e−10) with a local replication in ARIC. In black individuals, the variant rs10739419 on chromosome 9 in the Whirlin (WHRN) gene formally replicated (meta-P = 2.2e−9). The SNP-based heritability of HGI was 0.39 (P< 1e−10). HGI had significant sex-specific associations with SNPs in or near GALNT11 in women and HECW2 in men. Finally, in Hispanic participants, we observed genome-wide significant associations with variants near USF1 and NXNL2/SPIN1.
Discussion: Many HGI-associated SNPs were distinct from those associated with fasting plasma glucose or HbA1c, lending further support for HGI as a distinct biomarker of diabetes complications. The results of this first evaluation of the genetic etiology of HGI indicate that it is highly heritable and point to heterogeneity by sex and race.
Hemoglobin A1c (HbA1c) provides a more stable estimate of glycemic control over a 2- to 3-month period than point-estimate fasting plasma glucose (FPG). HbA1c is routinely used in diabetes diagnosis and treatment and is correlated with the risk of complications from diabetes. Collectively, HbA1c and FPG offer a nuanced view of an individual’s disease status. However, there is considerable heterogeneity in HbA1c, even among individuals with similar FPG or mean glucose levels. Because HbA1c is not a one-size-fits-all indicator of overall blood glucose control, the hemoglobin glycation index (HGI), which quantifies FPG‐independent biological variation in HbA1c in individuals with diabetes, may be important as an additional biomarker of clinical management/drug treatment outcomes. HGI is the difference between observed HbA1c and HbA1c predicted from other measures of glycemia (e.g., FPG) using linear regression. Studies of HGI, the glycation gap (1), and individuals with higher HbA1c values than those predicted by blood glucose reflect similar unexplained phenotypic variation in the temporal assessment of glycemic control predicted by a point estimate. Variation in HbA1c has been observed in individuals with and without diabetes and may be influenced by biological factors in addition to glucose, including erythrocyte lifespan, intracellular glucose concentration, intracellular or extracellular pH, deglycating enzyme activity, lipid peroxides, inorganic phosphates, hemoglobin oxygenation status, and inflammation (2–4). A recent landmark study in the Million Veterans Program highlighted that unexplained variation in HbA1c due to FPG contributes to increased disease progression at the time of diagnosis and increased risk of diabetes complications in men of African ancestry (5).
Previous studies have demonstrated that HGI can be used to identify individuals with type 2 diabetes at risk for multiple diabetes-related comorbidities and outcomes before the onset of other clinical symptoms (6). In a meta-analysis of 37,280 individuals with type 2 diabetes, those with high HGI had an increased risk of cardiovascular-related complications and death (7). HGI has been associated with the risk of retinopathy and nephropathy in individuals with type 1 diabetes and with the risk of coronary heart disease in individuals with type 2 diabetes (7) and those with normal glucose metabolism (8). As reviewed by Zhang et al. (7), in individuals with diabetes, high HGI calculated using either FPG or mean blood glucose (from patient meters or 1-day profile sets collected before and after meals) has been associated with a higher risk of hypertension, microvascular complications, hypoglycemia, and cardiovascular disease. In individuals without diabetes, high HGI has been associated with increased coronary artery calcification (9), carotid atherosclerosis (10), hepatic steatosis (11), kidney dysfunction (12), and inflammation and obesity (13, 14). Despite these associations, it has been suggested that because HGI is strongly associated with HbA1c, it should not be used as an independent predictor of microvascular complications (15). Studies have also reported increased HGI in black individuals and women (16). This evidence indicates that HGI reflects complexities in glucose control and the risk of chronic vascular disease in individuals both with and without diabetes.
HGI is a promising biomarker to determine the risk of diabetes comorbidities. Understanding the genetic etiology of this trait is an important goal and could provide insight into druggable targets and guide precision medicine approaches to treatment. While the genetics of HbA1c and other related traits have been extensively studied (17–21), to the authors’ knowledge, no studies have directly examined the genetics of HGI. To address this gap, we performed a genome-wide association study (GWAS) of HGI using data from the Action to Control Cardiovascular Risk in Diabetes (ACCORD) trial and replicated our findings using data from the Atherosclerosis Risk in Communities (ARIC) study, an independent cohort. In the spirit of discovery, we conducted stratified analyses by both genetic ancestry and biological sex.
We derived HGI by regressing FPG on HbA1c in ACCORD as previously reported (22). For the overall cohort and the 7,913 individuals genotyped in this study, the fitted line equates to: . We calculated the individual HGI from the residual (i.e., HbA1ci − ). For GWAS of HbA1c and FPG separately, and to calculate HGI, we used baseline values for HbA1c and FPG.
We performed genotyping using the HumanOmniExpressExome-8 v1.0 and Affymetrix Axiom Biobank1 arrays. Genotyping and imputation details are included in the Supplementary Material and have been previously described.
Details of the ACCORD genotyping can be found in the Supplementary Material. In brief, of the 10,250 ACCORD participants, 8,174 were genotyped across two platforms. Extensive QC, merging, and imputation were conducted and had been previously described (23, 24) and resulted in 8,054 participants with genetic data. After genotyping QC and phenotyping with the requisite FPG and HbA1c baseline values, 7,913 individuals were used in this GWAS. We conducted GWAS using ACCORD data to determine single nucleotide polymorphisms (SNPs) associated with baseline HGI using linear regression in six strata, namely, all participants, all men, all women, and white, black, and Hispanic individuals. To address population stratification, we conducted principal component analysis (PCA) using EIGENSTRAT v4.2 (25) and incorporated the principal components as model covariates. For each stratum, we repeated variable selection and PCA to address potential confounding. Some covariates were forced based on the results of previous studies and domain expertise, and others were based on backward selection using Bayesian information criteria. Supplementary Table 1 summarizes the selected covariates for each stratum, which include principal components, sex, years with type 2 diabetes, BMI, and other covariates. Supplementary Table 2 lists all the covariates considered for variable selection in ACCORD.
We used linear regression implemented in PLINK (26) (v1.9; www.cog-genomics.org/plink/1.9/) to test associations between phenotypes, covariates, and single common variants [minor allele frequency (MAF) > 3%]. We used additive encoding for genotyped variants, where is the number of minor alleles for the th individual. We implemented linear regression in the R programming language for imputed variants, where is the dosage score computed from the posterior probabilities for genotypes and . Samples were genotyped in two sets that were determined based on participant consent. For SNPs that were genotyped only in set 1 and imputed in set 2, we calculated association tests for each set separately and combined the results by meta-analysis using PLINK. SNPs with P<5e−8 were considered to have genome-wide significance, and those with P<5e−6 were considered to have suggestive significance. We report GWAS results for all participants, for all-male and all-female strata, and for white, black, and Hispanic individuals. We calculated SNP-based heritability with GCTA64 (27) (version 1.94.0; reml-grm and reml-alg 1 options), incorporating model covariates as described above.
Between 1987 and 1989, the ARIC prospective cohort study enrolled 15,792 adults aged 45 to 64 years from four U.S. communities (Forsyth County, NC; Jackson, MS; suburbs of Minneapolis, MN; and Washington County, MD) to examine the etiology and predictors of cardiovascular disease. The ARIC study design and methods are described in detail elsewhere (28). Notably, there are substantial differences in the ACCORD and ARIC cohorts in terms of the age of participants, mean HbA1c, and diabetes treatments, all of which have been associated with differences in HGI (see Table 1). This heterogeneity between cohorts minimizes potential associations due to bias. We downloaded the genome-wide autosomal SNP data available for 8,620 white ARIC participants from the database of Genotypes and Phenotypes (dbGaP). SNPs were genotyped using the Affymetrix 6.0 array and underwent previous sample quality control that included the removal of related pairs, duplicates, and sex misclassifications. We performed further preimputation quality control, additionally removing samples with a call rate<80% and SNPs with Hardy–Weinberg equilibrium (HWE) P<1e−6, missingness >10%, or MAF<3%. After filtering, 703,117 SNPs remained for genotype imputation. We conducted imputation from the All-ancestry panel of the 1000 Genome Phase III Integrated Release Version 5 using MiniMac software. After imputation, we removed SNPs with r2<0.30, MAF<1%, or HWE P<1 × 10−6 and retained 10,008,913 SNPs. Individuals selected for replication consisted of individuals with both FPG and HbA1c data (assays conducted at visit 5 follow-up ending in 2013), as well as genome-wide genotyping data (N = 3,741; 2,101 F/1,640 M; 3,160 EUR/581 AFR).
We assessed discovery variants from the ACCORD data with P<5e−6 for the overall cohort (N = 7,913) and strata for white and black participants (n = 5,085 and n = 1,378, respectively) from the ARIC data (Supplementary Table 3). ARIC performed association tests for each discovery variant using linear regression with an additive genetic model, adjusting for age, sex, BMI, trial clinic center, and the first 11 principal components. We analyzed summary statistics from the discovery (ACCORD) and replication (ARIC) cohorts (Supplementary Table 3) and conducted an inverse-variance-weighted meta-analysis using METAL (https://genome.sph.umich.edu/wiki/METAL_Documentation).
In total, we tested for replication of discovery variants with P<5e−6 for the three strata looked up in ARIC (all individuals, white individuals, and black individuals). The number of independent loci in each stratum was 24, 9, and 15, respectively. We considered a discovery finding to be formally replicated when a genome-wide significant (P< 5e−8) sentinel discovery variant was also found in ARIC with the same direction of effect and a replication P<0.05/number of independent loci, or when any discovery variant at suggestive significance (5e−8< P< 5e−6) achieved genome-wide significance after meta-analysis of discovery and ARIC. We defined local replication when any variant in discovery with P<5e−6 was found in ARIC with the same direction of effect and with replication P<0.05/number of independent loci.
All annotations were mapped to the GRCh37 assembly. We developed data visualizations using R version 4.10 and LocusZoom (http://locuszoom.org/). We identified expression quantitative trait loci (eQTL) variants using FIVEx (https://fivex.sph.umich.edu/). We assessed genetic colocalization with the eQTL signal using LocusFocus (https://locusfocus.research.sickkids.ca/). LocusFocus utilizes a simple sum method to identify the colocalization of genetic variation signal and expression (via GTEx) in tissue by gene combinations (29). We examined characterized genes within 150 kbp of the lead SNP and tissues related to the pancreas, vessel, muscle, and brain (n = 19). The Bonferroni-corrected P-values for genetic colocalization were as follows: rs73407935 chr7:67680375, 15 gene/tissues (combinations passed stage 1 and were tested using a simple sum statistic) within 150 kbp, adjusted P = 0.0033 (0.05/15).
We defined loci associated with HGI as non-overlapping genomic regions separated by r2<0.05 from (suggestive) association signals with P<5e−6 (Table 2). Complete results for all strata (P< 5e−6) can be found in Supplementary Table 6, and all variants for all GWAS strata are available through the LocusZoom hyperlinks in Supplementary Table 4.
The SNP-based heritability (h2) was 0.39 (P< 1e−10) for the combined ACCORD cohort (N = 7,913), 0.52 (P< 1e−10) for male participants (n = 4,769), and 0.51 (P = 4.64e−7) for female participants (n = 3,111), indicating that a substantial portion of the variability in HGI is due to underlying genetic factors. This high degree of heritability is common in complex genetic traits and supports the rationale for conducting a GWAS.
In the ACCORD discovery, 24 independent loci were associated with HGI at genome-wide suggestive significance (Table 2). In the GWAS of HGI for all participants, the lead association with HGI was variation in the intergenic variant rs73407935 (LOC105375341, 7q11.22; P = 5.82e−10) on chromosome 7 (Figures 1A, B). The genetic dosage of the T allele was associated with increased HGI (Figure 1C). For replication in ARIC, we selected variants with suggestive genome-wide significance of P<5e−6 (see Supplementary Table 3 for details). While the sentinel variant rs73407935 was not formally replicated in ARIC (Figures 2A, B; same direction of effect, P = 0.11), we did have local replication with rs73397205 in LD with the sentinel variant (R2 = 0.46; Figures 2A, C; discovery P = 1.3e−06, replication P = 0.001) after correcting for 24 independent lookup loci in discovery. Furthermore, this variant reached genome-wide significance after meta-analysis (Supplementary Table 5).
Figure 1. GWAS of HGI in ACCORD. (A) Manhattan plot for HGI for all subjects (n = 7,913). Variants with minor allele frequency >3% are ordered by chromosome (X-axis), and –log10 (P-values) are plotted (Y-axis). P-values are adjusted for baseline age, sex, BMI, treatment arm, principal components 1–3, number of years since diabetes diagnosis, and history of albuminuria. Dark dotted line: P = 5e−8; light dotted line P = 5e−6. (B) LocusZoom plot of the lead variant (rs73407935). (C) The allelic plot of the lead variant (rs73407935) showing HGI (Y-axis) against genotype (X-axis). A red dot represents the mean HGI response by genotype. The number of participants for each genotype is listed above the X-axis.
Figure 2. Replication in ARIC. (A) LocusZoom plot of the lead variant (rs73407935) with the formally replicated variant (rs73397205—R2 = 0.46) annotated for all subjects. (B) Forest plot of the lead variant for discovery (ACCORD), replication (ARIC), and inverse-variance-weighted meta-analysis (META) estimates and 95% confidence intervals [coded allele (CA) = T]. (C) Forest plot of the variant in linkage disequilibrium with the lead SNP that formally replicated with P<0.0021 (0.05/24 unique non-correlated genomic regions examined in ARIC). Each copy of the coded allele (CA = A) results in an increase in HGI of approximately 0.15 for this variant. (D) LocusZoom plot of the variant rs10739419, which replicated in ARIC for black participants with meta-P reaching genome-wide significance. (E) Forest plot of rs10739419 (CA = T).
As the first GWAS of HGI, we also provided LocusZoom plots of genome-wide suggestive hits for variants in or near N-acylsphingosine amidohydrolase 1 (ASAH1), leucine-rich repeat containing 4 (LRRC4), NUT family member 2B (NUTM2B; formerly FAM222B), c (SHLD1), and collagen type iv alpha 2 chain (COL4A2) (Supplementary Figures 1A–G). Meta-analysis with ARIC for 178 overlapping variants with P<5e−6 demonstrated a consistent direction of effect in 150 variants (Supplementary Table 5). The two most significant variants in FAM222B were also strong eQTLs—rs7217547 and rs12943388 (Supplementary Figures 1C–E). As these variants are highly significant eQTLs associated with the regulation of expression of multiple genes in pancreatic and brain tissues and immune cell populations, we conducted a formal expression colocalization test with the LocusFocus online web tool (see the Methods section for details), which revealed that the FAM222B discovery locus was a strong eQTL after Bonferroni correction for 15 gene/tissue combinations. A strong colocalization was observed between genetic variation in the FAM222B locus and the expression of FAM222B in cardiac tissue; FLOT2 in aortic tissue; PROCA1 in pancreatic, cardiac, and brain tissue; TLCD1 in cardiac and arterial tissue; and TRAF4 in arterial tissue (Figure 3).
Figure 3. Genetic colocalization with gene expression—FAM222B discovery signal. Heatmap of 19 tissues (brain, heart, vessels, glands, and pancreas) with nine nearby genes tested for genetic correlation with GTEx data. In total, 15 tissue/gene combinations were selected for stage 2 colocalization. A family-wise Bonferroni-adjusted P-value of –log10(0.05/15) >2.477 was used to determine significance.
Because of the need to treat sex as a biological variable, and to further conduct valuable science in underrepresented populations, we performed stratified GWAS by self-reported race for white, black, and Hispanic participants and by sex for the overall analysis. Supplementary Figure 2 displays the stacked Manhattan plot for HGI in combined/male/female strata with sex-specific associations for HGI. In women of all self-reported races, HGI was associated with genome-wide significant variants in polypeptide N-acetylgalactosaminyltransferase 11 (GALNT11; rs76030055; P = 4.99e−9). In men, HGI was associated with genome-wide significant variants in HECT-type E3 ubiquitin transferase (HECW2; rs16849034; P = 1.5e−8). These peaks were sex-specific, with minimal associations observed in the opposite sex. Supplementary Table 4 provides links to interrogatable Manhattan plots for each stratum.
We were unable to find replication cohorts with available data stratified by sex or Hispanic ethnicity. However, we were able to test for replication in ARIC for the self-identified white and black strata. In black participants (ACCORD: N = 1,378, ARIC: N = 581), the variant rs10739419 on chromosome 9 in the Whirlin (WHRN) gene was formally replicated (Figures 2D, E) in ARIC (meta-P = 2.20e−9), with each T allele associated with a 0.40 increase in HGI value. In white participants, despite a strong discovery association with HGI in COL4A2 (rs9521792; P = 5.17e−8; Supplementary Figure 1H) and a consistent direction of effect for all overlapping variants between ARIC and ACCORD, no variants met our criteria for replication in ARIC (Supplementary Table 5).
Hispanics are a largely understudied population in human genetics. In the ACCORD cohort, 544 individuals with genotyping data self-identified as Hispanic. We conducted GWAS on these participants. Two discovery loci had genome-wide significant associations with the following sentinel SNPs: rs2516837 (P = 1.48e−9) located on chromosome 1 in the USF1 gene and rs141006133 (P = 6.92e−9) located upstream of the nucleoredoxin-like 2 (NXNL2) gene on chromosome 9 (Supplementary Figures 1I–K). All variants for each stratum with P<5e−6 are reported in Supplementary Table 6.
Variants in any stratum at P<5e−6 (Supplementary Table 6) were examined for published results in the Human Genome Research Institute GWAS Catalog (30) (downloaded 6.7.2024). The reported traits with HGI-associated variants included height, educational attainment, otosclerosis, platelet count and crit, and alkaline phosphatase (Supplementary Table 7).
We also investigated the reported traits of HGI-associated genes for genes previously associated with type 2 diabetes. Eight genes associated with HGI were previously associated with type 2 diabetes in the GWAS catalog—ASAH1, CPED1, CPNE4, JMY, PTPRD, TMEM132D, UBASH3A, and UNC13C. A complete GWAS trait list of HGI-associated genes can be found in Supplementary Table 8.
Finally, for the sentinel variants in Table 2, we retrieved all variants with LD >0.60 from the GWAS catalog. These results, displayed in Supplementary Table 9, highlight numerous blood cell morphometric traits in addition to HbA1c and glomerular filtration rate. Taken together, these results demonstrate that HGI is a heritable trait and that genetic associations with HGI are very different than those with FPG and HbA1c. Consistent with recent publications highlighting the association of HGI with T2D complications and comorbidities, these data suggest the clinical utility of HGI in the management of T2D.
HGI has been associated with the severity of comorbid conditions in individuals with and without type 2 diabetes. In patients with type 2 diabetes, we mapped genetic variants associated with HGI with potential utility in clinical and precision medicine settings. For example, rs9515223 is a cis-eQTL in the COL4A2-AS1 RNA gene that has been associated with cerebral small vessel disease and decreased expression of COL4A2 and RAB20 in immune cells. This is an interesting discovery as brain atrophy and cerebrovascular disease are associated with type 2 diabetes (31). Additionally, rs6816425 is a strong cis-eQTL for increased expression of CXCL11 in the pancreas, which has been implicated in autoimmune disease pathogenesis, including diabetes, and is associated with insulin resistance in obese individuals (32, 33). Of particular interest are variants in and near genes associated with extracellular matrix function and immune function. Genes associated with extracellular matrix function are of interest because influences on collagen synthesis (e.g., variation in lysyl oxidase activity or vitamin C metabolism) represent a potential mechanistic link to the slow loss of vascular integrity and chronic vascular disease, which are associated with diabetes and high HbA1c. Genes associated with immune function are of interest because high HGI has been associated with increased levels of C-reactive protein (10, 13, 34, 35) and fibrinogen (10), which are suggestive of low-grade inflammation and altered systemic redox homeostasis.
The replication of significant findings is necessary to minimize false discovery in GWAS but is potentially problematic, as few substantially sized cohorts measure both HbA1c and FPG. Excitingly, across all ACCORD participants, the lead discovery locus was replicated in ARIC. This variant is an uncharacterized lncRNA. Furthermore, the discovery variant in the WHRN gene was replicated in black ARIC participants. Variants in WHRN have been associated with Usher syndrome and non-autoimmune adolescent diabetes (36). Variants in SHLD1 have been associated with multiple traits that include waist-to-hip ratio and apolipoprotein B levels (37, 38). Variants in FAM222B have been associated with increased hemoglobin and mean corpuscular hemoglobin (39, 40), along with decreased red blood cell density and erythrocyte count (39, 41).
GWAS trait lookups of all discovery variants from Supplementary Table 6 are shown in Supplementary Table 7. Discovery variants associated with HGI have also been associated with blood protein measurements, body height, platelet crit and count, and alkaline phosphatase measurements (40, 42–45). A lookup of all discovery genes in the associated GWAS catalog (Supplementary Table 8) revealed overwhelming associations with blood cell morphometry and quantification traits. We examined all discovery loci in Table 2 that were listed in the GWAS catalog. This revealed that blood cell morphometry and quantification were overwhelmingly associated with HGI-associated variants (Supplementary Table 9). A recent publication highlighted an increase in RBC turnover commensurate with a decrease in HbA1c relative to glucose levels, and an increase in the incidence of diabetes complications (diabetic retinopathy) in individuals with a variant in the G6PD gene (5). Including HGI as an additional clinical biomarker would have flagged these individuals for possible glucose management long before indication by aberrant HbA1c measurements, potentially preventing or delaying complications from T2D. Furthermore, we found a strong colocalization signal between HGI variation and expression of PROCA1 in brain, vascular, and pancreatic tissues. Variants in and near PROCA1 have been associated with cholesterol levels (46) and with reticulocyte count (39), further implicating blood cell counts and morphometric traits in the severity of T2D comorbidities.
In summary, we found sex- and self-reported race differences in association with HGI and achieved local replication in ARIC for the lead locus (rs73397205; CHR7:67736280) in all individuals and a variant in the WHRN gene in black individuals. This is striking considering the substantial differences between the ACCORD and ARIC cohorts in terms of participants’ age, BMI, and measures of glucose control, all of which are associated with HGI.
It is critical for research to focus on understudied populations, and we have done so here, although smaller sample sizes resulted in reduced statistical power. Nevertheless, we found striking associations in individuals of Hispanic ancestry. Although we were unable to find a replication cohort for the Hispanic strata in our discovery cohort, we did uncover unique genome-wide significant findings. Genetic mapping of Hispanic individuals in a cohort of this size (n = 544) is uncommon, as Hispanic patients are underrepresented in diabetes research, making the results even more important. We discovered genome-wide significant findings in USF1 and NXNL2. NXNL2 is involved in the sensory perception of smell and sight and is associated with neuroblastoma (47), and USF1 is a known lipogenic transcription factor (48, 49). Accordingly, follow-up in a cohort of Hispanic ethnicity is warranted.
In the GWAS catalog, HGI-associated variants were largely non-overlapping with FPG and HbA1c associations, two long-established measures of glucose control, indicating that HGI is a distinct, complex genetic trait. Our GWAS results indicate that HGI, like FPG and HbA1c, is highly heritable, with similar heritability to HbA1c (40%–50%). We also found several unique associations in the stratified analyses that indicate heterogeneity by sex and race. For example, we found a genome-wide significant association in women between HGI and GALNT11. GALNT11 plays a role in kidney function deterioration (50), and HGI has been associated with an increased risk of kidney dysfunction (12).
To the best of the authors’ knowledge, this is the first direct genetic study of HGI. We both discovered and replicated a variant locus in a previously characterized lncRNA. Despite substantial differences between ACCORD and ARIC, this locus was replicated in ARIC. Furthermore, we conducted genetic mapping of HGI stratified by self-reported race and sex. Given its emerging clinical importance, the discovery and validation of genetic variants associated with HGI may improve our understanding of its etiology, identify predictive biomarkers, and aid in the identification of increased comorbidities and the discovery of druggable targets.
Publicly available datasets were analyzed in this study. These data can be found here: https://biolincc.nhlbi.nih.gov/studies/accord/, https://biolincc.nhlbi.nih.gov/studies/aric/.
The institutional review board or ethics committee at each ACCORD center approved the ACCORD study protocol prior to data collection.
JSH: Formal analysis, Project administration, Supervision, Visualization, Writing – original draft, Writing – review & editing. JB: Funding acquisition, Writing – review & editing. FA: Formal analysis, Writing – review & editing. VE: Formal analysis, Visualization, Writing – review & editing. JBB: Conceptualization, Funding acquisition, Writing – review & editing. JH: Methodology, Writing – review & editing. AD: Funding acquisition, Writing – review & editing. JM: Funding acquisition, Writing – review & editing. VF: Conceptualization, Writing – review & editing. MS: Formal analysis, Writing – review & editing. CL: Formal analysis, Writing – review & editing. SL: Formal analysis, Writing – review & editing. TK: Writing – review & editing. DR: Writing – review & editing. AM-R: Conceptualization, Funding acquisition, Methodology, Supervision, Writing – original draft, Writing – review & editing.
The author(s) declare that financial support was received for the research, authorship, and/or publication of this article. This research was supported in part by the Intramural Research Program of the National Institute of Environmental Health Sciences.
We thank Hannah Collins Cakar for assistance with manuscript preparation.
The authors declare that the research was conducted in the absence of any commercial or financial relationships that could be construed as a potential conflict of interest.
All claims expressed in this article are solely those of the authors and do not necessarily represent those of their affiliated organizations, or those of the publisher, the editors and the reviewers. Any product that may be evaluated in this article, or claim that may be made by its manufacturer, is not guaranteed or endorsed by the publisher.
The Supplementary Material for this article can be found online at: https://www.frontiersin.org/articles/10.3389/fendo.2024.1473329/full#supplementary-material.
1. Cohen RM, Holmes YR, Chenier TC, Joiner CH. Discordance between HbA1c and fructosamine: evidence for a glycosylation gap and its relation to diabetic nephropathy. Diabetes Care. (2003) 26:163–7. doi: 10.2337/diacare.26.1.163
2. Khera PK, Joiner CH, Carruthers A, Lindsell CJ, Smith EP, Franco RS, et al. Evidence for interindividual heterogeneity in the glucose gradient across the human red blood cell membrane and its relationship to hemoglobin glycation. Diabetes. (2008) 57:2445–52. doi: 10.2337/db07-1820
3. Kunika K, Itakura M, Yamashita K. Inorganic phosphate accelerates hemoglobin A1c synthesis. Life Sci. (1989) 45:623–30. doi: 10.1016/0024-3205(89)90048-9
4. Hempe JM, Hsia DS. Variation in the hemoglobin glycation index. J Diabetes Complications. (2022) 36:108223. doi: 10.1016/j.jdiacomp.2022.108223
5. Breeyear JH, Hellwege JN, Schroeder PH, House JS, Poisner HM, Mitchell SL, et al. Adaptive selection at G6PD and disparities in diabetes complications. Nat Med. (2024) 30(9):2480–8. doi: 10.1038/s41591-024-03089-1
6. Kim MK, Jeong JS, Yun JS, Kwon HS, Baek KH, Song KH, et al. Hemoglobin glycation index predicts cardiovascular disease in people with type 2 diabetes mellitus: A 10-year longitudinal cohort study. J Diabetes Complications. (2018) 32:906–10. doi: 10.1016/j.jdiacomp.2018.08.007
7. Zhang L, Wang M, Zhang R, Zhong Y, Fan H, Wang M, et al. Hemoglobin glycation index in relationship to the risk of cardiovascular complication in patients with type 2 diabetes: A systematic review and meta-analysis. J Diabetes Complications. (2020) 34:107673. doi: 10.1016/j.jdiacomp.2020.107673
8. Lee B, Heo YJ, Lee YA, Lee J, Kim JH, Lee SY, et al. Association between hemoglobin glycation index and cardiometabolic risk factors in Korean pediatric nondiabetic population. Ann Pediatr Endocrinol Metab. (2018) 23:196–203. doi: 10.6065/apem.2018.23.4.196
9. Nagayama D, Watanabe Y, Yamaguchi T, Saiki A, Shirai K, Tatsuno I. High hemoglobin glycation index is associated with increased systemic arterial stiffness independent of hyperglycemia in real-world Japanese population: a cross-sectional study. Diabetes Vasc Dis Res. (2020) 17:1479164120958625. doi: 10.1177/1479164120958625
10. Marini MA, Fiorentino TV, Succurro E, Pedace E, Andreozzi F, Sciacqua A, et al. Association between hemoglobin glycation index with insulin resistance and carotid atherosclerosis in non-diabetic individuals. PloS One. (2017) 12:e0175547. doi: 10.1371/journal.pone.0175547
11. Fiorentino T, Marini M, Succurro E, Andreozzi F, Sciacqua A, Hribal M, et al. Association between hemoglobin glycation index and hepatic steatosis in non-diabetic individuals. Diabetes Res Clin Practice. (2017) 134:53–61. doi: 10.1016/j.diabres.2017.09.017
12. Fiorentino TV, Marini MA, Succurro E, Sciacqua A, Andreozzi F, Perticone F, et al. Elevated hemoglobin glycation index identify non-diabetic individuals at increased risk of kidney dysfunction. Oncotarget. (2017) 8:79576–86. doi: 10.18632/oncotarget.18572
13. Liu S, Hempe JM, McCarter RJ, Li S, Fonseca VA. Association between inflammation and biological variation in hemoglobin A1c in U. S. non-diabetic adults. J Clin Endocrinol Metab. (2015) 100:2364–71. doi: 10.1210/jc.2014-4454
14. Lyu L, Yu J, Liu Y, He S, Zhao Y, Qi M, et al. High Hemoglobin glycation index is associated with telomere attrition independent of HbA1c, mediating by TNFα. J Clin Endocrinol Metab. (2022) 107:462–73. doi: 10.1210/clinem/dgab703
15. Lachin JM, Genuth S, Nathan DM, Rutledge BN. The hemoglobin glycation index is not an independent predictor of the risk of microvascular complications in the Diabetes Control and Complications Trial. Diabetes. (2007) 56:1913–21. doi: 10.2337/db07-0028
16. Hsia DS, Rasouli N, Pittas AG, Lary CW, Peters A, Lewis MR, et al. Implications of the hemoglobin glycation index on the diagnosis of prediabetes and diabetes. J Clin Endocrinol Metab. (2020) 105:e130–8. doi: 10.1210/clinem/dgaa029
17. Hachiya T, Komaki S, Hasegawa Y, Ohmomo H, Tanno K, Hozawa A, et al. Genome-wide meta-analysis in Japanese populations identifies novel variants at the TMC6-TMC8 and SIX3-SIX2 loci associated with HbA1c. Sci Rep. (2017) 7:16147. doi: 10.1038/s41598-017-16493-0
18. Snieder H, Sawtell PA, Ross L, Walker J, Spector TD, Leslie RD. HbA1c levels are genetically determined even in type 1 diabetes: evidence from healthy and diabetic twins. Diabetes. (2001) 50:2858–63. doi: 10.2337/diabetes.50.12.2858
19. Hivert MF, Christophi CA, Jablonski KA, Edelstein SL, Kahn SE, Golden SH, et al. Genetic ancestry markers and difference in A1c between African American and white in the Diabetes Prevention Program. J Clin Endocrinol Metab. (2019) 104:328–36.doi: 10.1210/jc.2018-01416
20. An P, Miljkovic I, Thyagarajan B, Kraja AT, Daw EW, Pankow JS, et al. Genome-wide association study identifies common loci influencing circulating glycated hemoglobin (HbA) levels in non-diabetic subjects: The Long Life Family Study (LLFS). Metabolism. (2013) 63:461–8.doi: 10.1016/j.metabol.2013.11.018
21. Wheeler E, Leong A, Liu CT, Hivert MF, Strawbridge RJ, Podmore C, et al. Impact of common genetic determinants of Hemoglobin A1c on type 2 diabetes risk and diagnosis in ancestrally diverse populations: A transethnic genome-wide meta-analysis. PloS Med. (2017) 14:e1002383. doi: 10.1371/journal.pmed.1002383
22. Hempe JM, Liu S, Myers L, McCarter RJ, Buse JB, Fonseca V. The hemoglobin glycation index identifies subpopulations with harms or benefits from intensive treatment in the ACCORD trial. Diabetes Care. (2015) 38:1067–74. doi: 10.2337/dc14-1844
23. Irvin MR, Rotroff DM, Aslibekyan S, Zhi D, Hidalgo B, Motsinger-Reif A, et al. A genome-wide study of lipid response to fenofibrate in Caucasians: a combined analysis of the GOLDN and ACCORD studies. Pharmacogenet Genomics. (2016) 26:324–33. doi: 10.1097/FPC.0000000000000219
24. Marvel SW, Rotroff DM, Wagner MJ, Buse JB, Havener TM, McLeod HL, et al. Common and rare genetic markers of lipid variation in subjects with type 2 diabetes from the ACCORD clinical trial. PeerJ. (2017) 5:e3187. doi: 10.7717/peerj.3187
25. Price AL, Patterson NJ, Plenge RM, Weinblatt ME, Shadick NA, Reich D. Principal components analysis corrects for stratification in genome-wide association studies. Nat Genet. (2006) 38:904–9. doi: 10.1038/ng1847
26. Weeks JP. plink: an R package for linking mixed-format tests using IRT-based methods. J Stat Software. (2010) 35:1–33. doi: 10.18637/jss.v035.i12
27. Yang J, Lee SH, Goddard ME, Visscher PM. GCTA: a tool for genome-wide complex trait analysis. Am J Hum Genet. (2011) 88:76–82. doi: 10.1016/j.ajhg.2010.11.011
28. The Atherosclerosis Risk in Communities (ARIC) Study: design and objectives. The ARIC investigators. Am J Epidemiol. (1989) 129:687–702. doi: 10.1093/oxfordjournals.aje.a115184
29. Panjwani N, Wang F, Mastromatteo S, Bao A, Wang C, He G, et al. LocusFocus: Web-based colocalization for the annotation and functional follow-up of GWAS. PloS Comput Biol. (2020) 16:e1008336. doi: 10.1371/journal.pcbi.1008336
30. Buniello A, MacArthur JAL, Cerezo M, Harris LW, Hayhurst J, Malangone C, et al. The NHGRI-EBI GWAS Catalog of published genome-wide association studies, targeted arrays and summary statistics 2019. Nucleic Acids Res. (2019) 47:D1005–d12. doi: 10.1093/nar/gky1120
31. Moran C, Phan TG, Chen J, Blizzard L, Beare R, Venn A, et al. Brain atrophy in type 2 diabetes: regional distribution and influence on cognition. Diabetes Care. (2013) 36:4036–42. doi: 10.2337/dc13-0143
32. Fallahi P, Ferrari SM, Ragusa F, Ruffilli I, Elia G, Paparo SR, et al. Th1 chemokines in autoimmune endocrine disorders. Diabetes Care. (2013) 36(12):4036–42. doi: 10.1210/clinem/dgz289
33. Moreno B, Hueso L, Ortega R, Benito E, Martínez-Hervas S, Peiro M, et al. Association of chemokines IP-10/CXCL10 and I-TAC/CXCL11 with insulin resistance and enhance leukocyte-endothelial arrest in obesity. Microvasc Res. (2022) 139:104254. doi: 10.1016/j.mvr.2021.104254
34. Ahn CH, Min SH, Lee DW, Oh TJ, Kim KM, Moon JH, et al. Hemoglobin glycation index is associated with cardiovascular diseases in people with impaired glucose metabolism. J Clin Endocrinol Metab. (2017) 102:2905–13. doi: 10.1210/jc.2017-00191
35. Hempe JM, Yang S, Liu S, Hsia DS. Standardizing the haemoglobin glycation index. Endocrinol Diabetes Metab. (2021) 4:e00299. doi: 10.1002/edm2.v4.4
36. Al Mutair AN, Brusgaard K, Bin-Abbas B, Hussain K, Felimban N, Al Shaikh A, et al. Heterogeneity in phenotype of usher-congenital hyperinsulinism syndrome: hearing loss, retinitis pigmentosa, and hyperinsulinemic hypoglycemia ranging from severe to mild with conversion to diabetes. Diabetes Care. (2013) 36:557–61. doi: 10.2337/dc12-1174
37. Sinnott-Armstrong N, Tanigawa Y, Amar D, Mars N, Benner C, Aguirre M, et al. Genetics of 35 blood and urine biomarkers in the UK Biobank. Nat Genet. (2021) 53:185–94. doi: 10.1038/s41588-020-00757-z
38. Christakoudi S, Evangelou E, Riboli E, Tsilidis KK. GWAS of allometric body-shape indices in UK Biobank identifies loci suggesting associations with morphogenesis, organogenesis, adrenal cell renewal and cancer. Sci Rep. (2021) 11:10688. doi: 10.1038/s41598-021-89176-6
39. Vuckovic D, Bao EL, Akbari P, Lareau CA, Mousas A, Jiang T, et al. The polygenic and monogenic basis of blood traits and diseases. Cell. (2020) 182:1214–31.e11. doi: 10.1016/j.cell.2020.08.008
40. Astle WJ, Elding H, Jiang T, Allen D, Ruklisa D, Mann AL, et al. The allelic landscape of human blood cell trait variation and links to common complex disease. Cell. (2016) 167:1415–29.e19. doi: 10.1016/j.cell.2016.10.042
41. Chen MH, Raffield LM, Mousas A, Sakaue S, Huffman JE, Moscati A, et al. Trans-ethnic and ancestry-specific blood-cell genetics in 746,667 individuals from 5 global populations. Cell. (2020) 182:1198–213.e14. doi: 10.1016/j.cell.2020.06.045
42. Gudjonsson A, Gudmundsdottir V, Axelsson GT, Gudmundsson EF, Jonsson BG, Launer LJ, et al. A genome-wide association study of serum proteins reveals shared loci with common diseases. Nat Commun. (2022) 13:480. doi: 10.1038/s41467-021-27850-z
43. Kichaev G, Bhatia G, Loh PR, Gazal S, Burch K, Freund MK, et al. Leveraging polygenic functional enrichment to improve GWAS power. Am J Hum Genet. (2019) 104:65–75. doi: 10.1016/j.ajhg.2018.11.008
44. Sakaue S, Kanai M, Tanigawa Y, Karjalainen J, Kurki M, Koshiba S, et al. A cross-population atlas of genetic associations for 220 human phenotypes. Nat Genet. (2021) 53:1415–24. doi: 10.1038/s41588-021-00931-x
45. Chen VL, Du X, Chen Y, Kuppa A, Handelman SK, Vohnoutka RB, et al. Genome-wide association study of serum liver enzymes implicates diverse metabolic and liver pathology. Nat Commun. (2021) 12:816. doi: 10.1038/s41467-020-20870-1
46. Graham SE, Clarke SL, Wu KH, Kanoni S, Zajac GJM, Ramdas S, et al. The power of genetic diversity in genome-wide association studies of lipids. Nature. (2021) 600:675–9. doi: 10.1038/s41586-021-04064-3
47. Barr EK, Applebaum MA. Genetic predisposition to neuroblastoma. Children (Basel). (2018) 5(9):119. doi: 10.3390/children5090119
48. Wang Y, Viscarra J, Kim SJ, Sul HS. Transcriptional regulation of hepatic lipogenesis. Nat Rev Mol Cell Biol. (2015) 16:678–89. doi: 10.1038/nrm4074
49. Viscarra J, Sul HS. Epigenetic regulation of hepatic lipogenesis: role in hepatosteatosis and diabetes. Diabetes. (2020) 69:525–31. doi: 10.2337/dbi18-0032
Keywords: hemoglobin glycation index, HGI, HbA1c, ACCORD, ARIC, GWAS, genome-wide association study
Citation: House JS, Breeyear JH, Akhtari FS, Evans V, Buse JB, Hempe J, Doria A, Mychaleckyi JC, Fonseca V, Shi M, Li C, Liu S, Kelly TN, Rotroff D and Motsinger-Reif AA (2024) A genome-wide association study identifies genetic determinants of hemoglobin glycation index with implications across sex and ethnicity. Front. Endocrinol. 15:1473329. doi: 10.3389/fendo.2024.1473329
Received: 30 July 2024; Accepted: 03 October 2024;
Published: 28 October 2024.
Edited by:
Vincent Poitout, Montreal University, CanadaCopyright © 2024 House, Breeyear, Akhtari, Evans, Buse, Hempe, Doria, Mychaleckyi, Fonseca, Shi, Li, Liu, Kelly, Rotroff and Motsinger-Reif. This is an open-access article distributed under the terms of the Creative Commons Attribution License (CC BY). The use, distribution or reproduction in other forums is permitted, provided the original author(s) and the copyright owner(s) are credited and that the original publication in this journal is cited, in accordance with accepted academic practice. No use, distribution or reproduction is permitted which does not comply with these terms.
*Correspondence: Alison A. Motsinger-Reif, YWxpc29uLm1vdHNpbmdlci1yZWlmQG5paC5nb3Y=
Disclaimer: All claims expressed in this article are solely those of the authors and do not necessarily represent those of their affiliated organizations, or those of the publisher, the editors and the reviewers. Any product that may be evaluated in this article or claim that may be made by its manufacturer is not guaranteed or endorsed by the publisher.
Research integrity at Frontiers
Learn more about the work of our research integrity team to safeguard the quality of each article we publish.