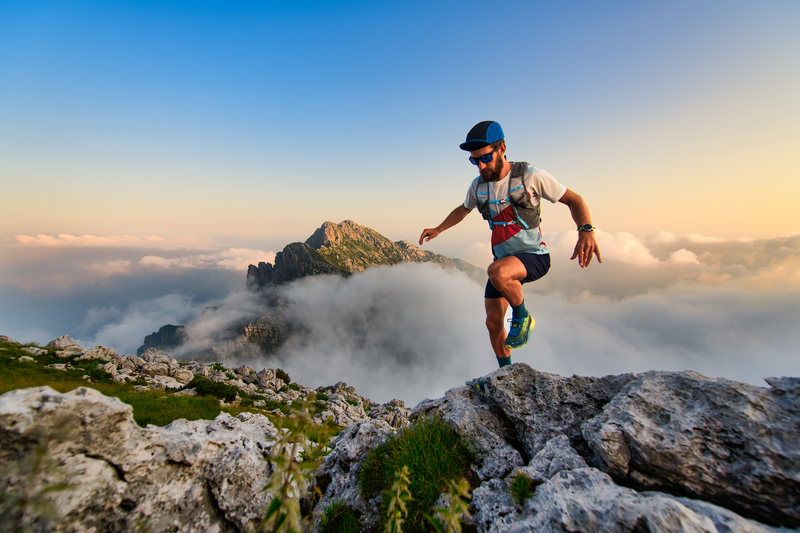
95% of researchers rate our articles as excellent or good
Learn more about the work of our research integrity team to safeguard the quality of each article we publish.
Find out more
ORIGINAL RESEARCH article
Front. Endocrinol. , 11 December 2024
Sec. Clinical Diabetes
Volume 15 - 2024 | https://doi.org/10.3389/fendo.2024.1459171
This article is part of the Research Topic Endocrine Complications of COVID-19: Short and Long View all 11 articles
Introduction: Post-COVID-19 syndrome (PCS) is a severe acute respiratory syndrome coronavirus 2 (SARS-CoV-2) infection-associated chronic condition characterized by long-term violations of physical and mental health. People with type 2 diabetes (T2D) are at high risk for severe COVID-19 and PCS.
Aim: The current study aimed to define the predictors of PCS development in people with T2D for further planning of preventive measures and improving patient outcomes.
Materials and methods: The data were collected through the national survey targeting persons with T2D concerning the history of COVID-19 course and signs and symptoms that developed during or after COVID-19 and continued for more than 12 weeks and were not explained by an alternative diagnosis. In total, 469 patients from different regions of Ukraine were enrolled in the study. Among them, 227 patients reported PCS development (main group), while 242 patients did not claim PCS symptoms (comparison group). Stepwise multivariate logistic regression and probabilistic neural network (PNN) models were used to select independent risk factors.
Results: Based on the survey data, 8 independent factors associated with the risk of PCS development in T2D patients were selected: newly diagnosed T2D (OR 4.86; 95% CI 2.55–9.28; p<0.001), female sex (OR 1.29; 95% CI 0.86–1.94; p=0.220), COVID-19 severity (OR 1.35 95% CI 1.05–1.70; p=0.018), myocardial infarction (OR 2.42 95% CI 1.26–4.64; p=0.002) and stroke (OR 3.68 95% CI 1.70–7.96; p=0.001) in anamnesis, HbA1c above 9.2% (OR 2.17 95% CI 1.37–3.43; p=0.001), and the use of insulin analogs (OR 2.28 95% CI 1.31–3.94; p=0.003) vs human insulin (OR 0.67 95% CI 0.39–1.15; p=0.146). Although obesity aggravated COVID-19 severity, it did not impact PCS development. In ROC analysis, the 8-factor multilayer perceptron (MLP) model exhibited better performance (AUC 0.808; 95% CІ 0.770–0.843), allowing the prediction of the risk of PCS development with a sensitivity of 71.4%, specificity of 76%, PPV of 73.6% and NPV of 73.9%.
Conclusions: Patients who were newly diagnosed with T2D, had HbA1c above 9.2%, had previous cardiovascular or cerebrovascular events, and had severe COVID-19 associated with mechanical lung ventilation were at high risk for PCS.
Approximately 95% of people with diabetes worldwide have type 2 diabetes (T2D). A 3% increase in age-standardized mortality rates from diabetes was recorded from 2000 to 2019 (1). T2D is a group of metabolic diseases caused by insulin resistance (IR) and altered insulin secretion by β-cells of the pancreas (2, 3).
The 2019 coronavirus disease (COVID-19) pandemic caused by severe acute respiratory syndrome coronavirus 2 (SARS-CoV-2) has become a global concern (4–6). T2D is one of the most common comorbidities in patients infected with the SARS-CoV-2 virus, with a relatively high incidence of severe COVID-19 (7, 8). T2D has a bidirectional relationship with COVID-19 (9). Poorly controlled, decompensated T2D exacerbates the severity of COVID-19 and leads to an increased risk of hospitalization and mortality (10, 11). Potential mechanisms contributing to enhanced susceptibility to SARS-CoV-2 infection and poorer prognosis in people with T2D include a proinflammatory state, weakened innate immune response, possibly elevated levels of angiotensin-converting enzyme 2 (ACE2), vascular dysfunction and a prothrombotic state (12–14). On the other hand, an extreme systemic immune response (“cytokine storm”), direct attack of pancreatic β-cells by SARS-CoV-2 by binding to ACE2, and an unbalanced immune response can, in turn, lead to glycemic profile disorders, uncontrolled hyperglycemia, and progression of IR in persons with T2D (15, 16).
COVID-19 combined with T2D enhances the risk of hospitalization and the need for mechanical ventilation, increasing the probability of post-COVID-19 syndrome development. Post-COVID-19 syndrome (PCS; long COVID-19, post-acute COVID-19, long-term effects of COVID-19) has become an emerging health problem in people recovering from COVID-19 infection (17–19). PCS condition occurs in individuals with a history of probable or confirmed SARS- CoV-2 infection, usually 3 months from the onset of COVID-19 with symptoms that last for at least 2 months and cannot be explained by an alternative diagnosis (20). Common symptoms include rapid fatigue, weakness, headaches, memory loss, distraction, depression, prolonged cough or shortness of breath, insomnia, heart palpitations, bone and joint aches, myalgias, gastrointestinal disorders, and insensitivity to smells and tastes (20). Symptoms may be new onset, following initial recovery from an acute COVID-19 episode or persist from the initial illness. Symptoms may also fluctuate or relapse over time (20).
One of the consequences of lung damage in patients with SARS-CoV-2 infection, namely, pulmonary fibrosis, which can manifest as persistent shortness of breath requiring oxygen supplementation in the PCS period, is more common in people with poorly controlled diabetes (18). Not surprisingly, the bidirectional association between diabetes and PCS has been at the top of scientific discussions (21, 22). Some evidence suggests that diabetes may be a risk factor for the development of PCS (22, 23). Recent data also indicate that new-onset diabetes might be a complication of COVID-19 and represents the metabolic clinical phenotype of PCS (18, 24). However, the particular links between T2D and PCS are still under debate. Limited research exists on PCS incidence and prevalence in low- and middle-income countries.
The current study aimed to define the predictors of PCS development in people with T2D for further planning of preventive measures and improving patient outcomes.
The study protocol was approved by the Ethics Committee at Bogomolets National Medical University (protocol number: 171/2023) and was conducted according to the guidelines of the 1975 Declaration of Helsinki. Individuals with T2D were enrolled in the study during visits to endocrinologists at outpatient clinics. The purpose and methodology of the study were fully explained to the participants by the researchers, and all patients were asked to provide signed informed consent before data collection.
To gather data concerning the outcomes of COVID-19 in T2D persons, a questionnaire was developed. The following clinical and demographic data were collected: age, sex, anthropometric indicators, T2D duration and age at onset, T2D complications, history of COVID-19, COVID-19 severity and treatment, PCS symptoms, duration of PCS and hypoglycemic therapy. According to the WHO classification, COVID-19 was categorized as mild, moderate or severe. Mild COVID-19 was defined as respiratory symptoms without evidence of pneumonia or hypoxia, while moderate or severe infection required the presence of clinical and radiological evidence of pneumonia. In moderate cases, SpO2 ≥90% on room air, while one of the following was required to define severe cases: respiratory rate >30 breaths/min or SpO2 <90% on room air (25, 26). The data were collected and registered by a professional endocrinologist during the follow-up visits of patients to outpatient clinics. Medical data were also retrieved from the medical records of the participants.
The inclusion criteria were as follows: age over 18 years and the presence of T2D and COVID-19 confirmed by a positive RT−PCR test. The exclusion criteria included type 1 diabetes or secondary diabetes, autoimmune diseases, inflammatory diseases, other than T2D metabolic diseases and active malignancy. The data from 469 patients who suffered from COVID-19 infection were collected in different regions of Ukraine. According to the responses, patients were divided into 2 groups depending on the outcomes for up to 6 months after COVID-19 infection: the PCS group (main group, n=227) and patients who didn’t develop PCS (comparison group, n=242).
Body mass index (BMI) was calculated as body weight in kilograms divided by the square of the participant’s height in meters (weight/height2). The waist (narrowest diameter between the xiphoid process and iliac crest) circumference (WC) was also measured.
As obesity itself is an immunometabolic disorder, facilitating pro-inflammatory cytokines secretion, reducing insulin sensitivity (27) and modulating SARS-Cov2 retention (28) we provided a sub-analysis for assessing the effect of obesity on PCS development and COVID-19 severity. Patients were divided into two sub-groups including individuals with BMI<30kg/m2 (n=110) and patients with obesity (BMI≥30kg/m2, n=117).
In addition, cases of new-onset T2D were assessed separately. New-onset T2D in PCS group was defined when occur during or after acute COVID-19 phase within 3 months (n=43). From comparison group we included in sub analysis patients with onset of T2D before 3 months to COVID-19 (n=17).
Statistical analysis was performed using MedCalc® Statistical Software v. 22.026 (MedCalc Software Ltd., Ostend, Belgium; https://www.medcalc.org; 2024) and STATISTICA Neural Networks R.4.0 C (StatSoft. Inc. 1998-1999). To test the normality of the distribution, the Shapiro−Wilk test was used. Quantitative variables are presented as the median and interquartile range (Me, QI – QIII), and qualitative variables are presented as %. To estimate the difference in the incoming qualitative data, the χ2 test or Fisher’s exact test was used; for quantitative data, the Mann−Whitney test was used. Univariate logistic regression analysis was applied to assess variables associated with PCS development in patients with T2D.
Stepwise multivariate logistic regression and probabilistic neural network (PNN) models were used to select independent risk factors associated with PCS development. In the first stage, a minimal set of variables associated with PCS risk was selected. To select independent risk factors for multivariate logistic regression models, stepwise inclusion/exclusion of variables (stepwise with penter <0.1 та premove>0.2) was performed, and the genetic algorithm (GA) method of selection was used for the PNN models. For the PNN models, all patients were randomly (using a random number generator) divided into 3 sets: training (which was used to build the model and calculate weight coefficients of the neural network, n=369), test (used to prevent overtraining of the mathematical model, n=60) and verification (used to test the predictive ability of the mathematical model on new data for controlling model retraining, n=40) sets.
The diagnostic performance of the models was evaluated using receiver operating characteristic (ROC) curve analysis. The area under the ROC curve (AUC) and its 95% confidence interval (CI) were calculated. A p value < 0.05 was considered to indicate statistical significance in all tests. Optimal cutoff values were chosen to maximize the sum of sensitivity and specificity. Positive predictive values (PPVs) and negative predictive values (NPVs) were computed for these cutoff values (29).
The baseline clinical parameters, COVID-19 and T2D histories of the surveyed patients are presented in Table 1. Among the study subjects, the ages in the main group were 61 (54 – 67) and 60 (54 – 68) years (p=0.900), respectively. The main group comprised more patients over 60 years old (59% vs 53.7% in the comparison group), although these differences were not significant (p=0.264) (Table 1). There were 124 females (54.6%) in the PCS group, while the proportion of women was lower among patients with no PCS (115 out of 242, 47,5); however, sex differences were not significant between the groups (p=0.139). We also did not find differences in patient weight (p=0.994) or BMI (p=0.881) (Table 1).
By assessing the clinical phenotypes of PCS among T2D patients, we found that fatigue was the most often observed manifestation of PCS (59.5%). It was followed by muscle aches (49.3%), headache (44.1%), shortness of breath (39.2%), new or persistent cough (31.7%), loss or change of smell (31.3%), dyssomnia (28.8%), arrhythmia (23.3%), gastrointestinal disorders (19.8%), and depression (16.7%) (Figure 1A).
Figure 1. The distribution of PCS symptoms: (A) among persons with T2D (main group); (B) sub analysis depending on presence of obesity in patients among PCS group.
The subjects who suffered from PCS reported a medical history of poor glycemic control during anamnesis and had higher values of HbA1c than did those in the comparison group (8.2% (7.2 - 10) vs 7.9% (7 – 9); p=0.005) (Table 1). Surprisingly, the mean duration of T2D was lower in the main group 10 (3–15) years as compared to the comparison group - 11 (7 – 16) years (p=0.015) (Table 1). This finding was explained by the increased incidence of newly diagnosed T2D during the COVID-19 pandemic, representing one of the clinical phenotypes of PCS. The number of patients with newly developed T2D was 43 out of 224 patients in the main group (18.9%), while only 17 individuals with newly diagnosed T2D were identified in the comparison group (7.0%; p<0.001) (Table 1). In sub analysis patient with new-onset T2D from PCS group characterized with more aggressive presentation as compared to matched patient from comparison group (7.0 (6.5 – 8.8) vs 7.8% (6.5 – 9.4); p=0.344). We did not find significant differences in the age of patients with new T2D between groups (57.0 (47.5 – 69.0) vs 58.0 (45.0 – 64.0) years, p=0.582). Surprisingly, patients with new-onset T2D in PCS group had lower weight (88.0 (80.0 – 96.0) vs 97.0 (85.0 – 109.0) kg; p=0.043) and BMI (28.4 (25.2 – 31.2) vs 31.5 (28.4 – 38.1) kg/m2; p=0.021) as compared to those with no PCS.
It is also worth noting that patients with PCS had a greater incidence of diabetic macrovascular complications: 13.7% and 11.9% of PCS patients reported myocardial infarction and stroke, respectively, during anamnesis (Table 1). In patients without PCS, a significantly lower prevalence of cardiovascular events was reported in their medical history: 7.9% for myocardial infarction (p=0.051) and 4.5% for stroke (p=0.004). The incidence of microvascular T2D complications did not differ significantly between the groups (Table 1).
PCS was associated with a greater rate of hospitalization and a more severe COVID-19 course. By comparing the COVID-19 course, we found that in the comparison group, most patients demonstrated mild (107; 44.2%) or moderate COVID-19 without hospitalization (91; 37.6%), and only a relatively small portion of the group had a moderate course of hospitalization (40; 16.5%) or suffered from severe COVID-19 (4; 1.7%) (Table 1). In contrast, approximately 40% of the main group reported severe (22; 9.7%) or moderate disease, with hospitalization (65; 28.6%) impacting further PCS development (p<0.001) (Table 1).
Importantly, PCS development was associated with a higher rate of noncompliance with antidiabetic medications: 7.9% of patients in the main group didn’t follow treatment recommendations, while the percentages were less than half in the comparison group (3.3%; p=0.042) (Table 1). We also found a difference in the rate of insulin and its analog administration between the observed groups: patients without PCS were more often administered human insulin than were those in the main group (24% vs 15.9%; p=0.029), where insulin analogs were used more often (23.3% vs 12.4%; p=0.002) (Table 1). In terms of the treatment of T2D, we did not find significant differences among the prescribed anti-diabetic drugs (ADDs) between the groups (Table 1).
There were also peculiarities related to COVID-19 treatment. Considering the increased rate of hospital admission and severe COVID-19 history, the PCS group reported a significantly increased rate of steroid prescription (42.7% vs 25.2%; p<0.001) and mechanical ventilation (7% vs 0.4%; p<0.001) due to the severity of COVID-19. At the same time, we did not find differences in the prescription of NSAIDs, antibiotics or O2 therapy between the groups (Table 1).
Obese people with T2D were more frequently diagnosed with moderate and severe forms of COVID-19 infection as compared to patients with BMI<30 (46.1% vs 30.0%, p=0.011; Table 2). Besides, glucocorticoid prescription was more frequent in obese patients with T2D (53.8% vs 30.9%; p<0.001; Table 2) during the COVID-19 course. The data representing clinical parameters, COVID-19 and T2D history in PCS group with respect to obesity are presented in Table 2. Important that T2D duration in normal/overweight patients was significantly shorter as compared to obese (8.5 (2.8 – 14.0) vs 11.0 (5.0 – 17.0) years, p=0.013). This finding can be explained by the higher occurrence of newly onset T2D in PCS group (Table 2). The profile of T2D complication and anti-diabetic treatment didn`t differ significantly between subgroups (Table 2). Moreover, there were no significant differences in PCS symptoms in T2D patients regarding obesity (Figure 1B).
Table 2. The sub analysis for distribution of clinical parameters, COVID-19 and T2D history depending on presence of obesity in patients of PCS group.
It is worth noting that patients with and without PCS did not differ in BMI (Table 1). Similarly, there were no differences in the incidence of obesity between PCS and comparison groups. The shares of people with obesity comprised 51.5% (117 of 227) among the PCS group being comparable with the value in the comparison group (116 of 242; 47.8%; p=0.435). Finally, among 233 T2D patients with comorbid obesity, about half (117; 50.2%) reported PCS symptoms while the rest – no, demonstrating no impact of obesity on PCS development in the observed cohort.
Thus, comorbid obesity aggravated COVID-19 severity but did not impact PCS development in patients with T2D.
Univariate logistic regression analysis revealed the following independent predictors of PCS development in patients with T2D: newly diagnosed T2D (p<0.001), poor glycemic control with an HbA1c above 9.2% (p<0.001), history of myocardial infarction (p=0.044) or stroke (p=0.005), treatment of T2D with insulin analogs (p=0.002), moderate-to-severe COVID-19 course (p<0.001), history of treating COVID-19 with glucocorticoids (p<0.001) and mechanical ventilation (p=0.005). In contrast, the use of human insulin (OR 0.598; 95% CI 0.377-0.950; p=0.029) had a protective effect on PCS development (Table 3).
To select the most informative risk factors, multifactorial logistic regression analysis was applied. As a result of the selection, the following 8 independent factors associated with the risk of PCS development in T2D patients were selected: newly diagnosed T2D (OR 4.86; 95% CI 2.55 – 9.28; p<0.001), female sex (OR 1.29; 95% CI 0.86 – 1.94; p=0.220), COVID-19 severity (OR 1.35 95% CI 1.05 – 1.70; p=0.018), presence of myocardial infarction (OR 2.42 95% CI 1.26 – 4.64; p=0.002) and stroke (OR 3.68 95% CI 1.70 – 7.96; p=0.001) in anamnesis, HbA1c above 9.2% (OR 2.17 95% CI 1.37 – 3.43; p=0.001), use of insulin analogs (OR 2.28 95% CI 1.31 – 3.94; p=0.003) vs human insulin (OR 0.67 95% CI 0.39 – 1.15; p=0.146), as specified in Table 4. The AUROC of the model was 0.74 (95% CI 0.697 - 0.779; p<0.001) (Figure 2A). This model demonstrated modest accuracy, as presented in Table 5.
Figure 2. ROC analysis for predicting PCS in patients with T2D. (A) logistic regression model; (B) MLP model; (C) pairwise comparison between models.
In the second stage, we built PNN models based on nonlinear relationships between variables and outcomes. We used a multilayer perceptron (MLP) with one hidden layer. The architecture of the hidden layer had 3 neurons with a logistic activation function. According to the ROC analysis, the AUC for the MLP model was 0.808 (95% CІ 0.770 - 0.843, p<0.001) (Figure 2B). The cutoff value for this model was chosen based on the Youden index (>0.490). When applying the optimal threshold, the following characteristics of the model were detected: sensitivity, 71.4% (95% CІ 65.0 - 77.2%); specificity, 76.0% (95% CІ 70.1 - 81.3%); PPV, 73.6% (95% CІ 68.7 - 78.0%); and NPV, 73.9% (95% CІ 69.5 - 77.9%) (Table 5). The forecasting results using neural networks were significantly better than those of the logistic model (p<0.001). The results of pairwise comparisons of the ROC curves are presented in Figure 2C. This indicates the presence of nonlinearity in the relationship between PCS risk and factor attributes that cannot be taken into account in a simple regression model.
Although high BMI and diabetes have been recognized as risk factors for developing severe COVID-19 and PCS, there are still no clearly articulated predictors of PCS development in T2D patients who restrict preventive measures for improving patient outcomes and quality of life (30). This study revealed the key risk factors associated with the risk of PCS development in T2D patients.
By applying various types of logistic regression analysis and PNN, we identified risk factors, including female sex, COVID-19 severity and corresponding mechanical ventilation experience, newly diagnosed during COVID-19 diabetes and an HbA1c higher than 9.2%, as well as myocardial infarction or stroke in medical history, as key risk factors for PCS prediction. Various studies of PCS prognosis have also revealed the prognostic role of different factors. Despite the variability of the results, the ability of core factors, including female sex and COVID-19 severity, to predict PCS has been underscored in different studies. Maglietta et al. in systematic review demonstrated the role of female sex and acute disease severity (31). Lemhöfer et al. reported that female sex, preexisting coagulation disorders and coronary artery disease were associated with a higher PCS rate (32). Similarly, in multivariate analysis, Zemni et al. showed that female sex, preexisting comorbidities, duration of acute COVID-19 illness, hospitalization, number of COVID-19 episodes and vaccination against SARS-CoV-2 are important in defining the risk of PCS development (33). These data obtained from the whole population analysis are consistent with our findings supporting the role of sex and acute viral infection severity. In this study, involving exclusively T2D patients, additional factors, including T2D severity and insulin treatment, were found to be essential for estimating the probability of PSC.
Diabetes is associated with a high risk of adverse outcomes of COVID-19 infection and PCS (30). On the other hand, the current study did not confirm that the presence of T2D was a risk factor for long-term symptoms of PCS (34). According to the obtained results, patients with and without T2D who recovered from COVID-19 at 7.2 (SD 0.6) months after hospital discharge had similar incidence rates of PCS symptoms (1.06, 95% CI 0.92-1.24; p=0.372) (34). Therefore, additional factors influencing the outcome of COVID-19 infection should be investigated.
Another challenge which is actively debated is assessment of preadmission use of different ADD for on COVID-19 adverse outcomes, mortality as well PCS development and severity. Recent studies revealed that pretreatment with metformin, GLP-1RA, and SGLT-2i was associated with a lower mortality rate, main adverse outcomes and hospitalization in patients with COVID-19 and T2D (35, 36). DPP-4i use was associated with a statistically significant increase in the risk of hospitalization, admission (35) to the ICU and mortality (36). Treatment with insulin is a risk factor for hospitalization and increased mortality (36, 37). The effects of sulfonylurea, thiazolidinedione, and alpha-glucosidase inhibitors on mortality are neutral (36). In contrast, the current study did not find a significant association between at-home ADD administration and mortality or adverse outcomes in patients with T2D admitted for COVID-19 (38). Recent data regarding the association between common T2D treatments and PCS development are scarce and limited to several reports on the protective effects of metformin (39–41). For instance, a recent multicenter, randomized, quadruple-blind, parallel-group, phase 3 trial demonstrated that outpatient treatment with metformin reduced the PCS incidence by approximately 41% (42). In our study, we noticed that insulin analogs significantly increased the risk of PCS development; in contrast, the use of human insulin had a protective effect. The other types of ADDs were neutral.
A close association between T2D and COVID-19 emerged early during the pandemic and is still active (43–45). A history of diabetes in subjects with acute SARS-CoV-2 infections was shown to worsen all outcomes and increase mortality (46). Although the respiratory system is the primary target of SARS-CoV-2, many other organs and cells can be affected by the virus, including the endothelium, cardiomyocytes, immune cells and β-cells of the pancreas. In fact, diabetes and SARS-CoV-2 infection share two essential commonalities - inflammatory pathway activation and multiorgan involvement in pathological processes (47, 48). This can result in manifestations of various severe pathologies, including acute cardiovascular dysfunction, digestive system disorders, neurological complications and metabolic disturbances.
Both severe COVID-19 and inefficient glucose control aggravated PCS development in T2D patients. However, there is a bidirectional interplay between COVID-19 and T2D (43), establishing a vicious cycle that facilitates the development of complications (49). SARS-CoV-2 infection and diabetes share two fundamental features: an inflammatory state and multiorgan involvement and damage (50). Notably, the close relationship between immunity and the endocrine system impacts immune cell functionality and the response to viruses. For instance, insulin can directly regulate immune cells, including T-lymphocytes, which are responsible for antiviral immunity (51). Both CD4+ and CD8+ T cells express insulin receptors, which are involved in facilitating glucose uptake and promoting glycolytic metabolism during T-cell activation (52). Moreover, an acute decrease in insulin levels impairs CD8+ T-cell responses to infection, whereas the injection of basal insulin increases the antiviral potential of these cells (53). Hyperglycemia directly undermines the key function of immune cells (54). High blood glucose is related to impaired cytotoxicity of CD8+ and NK cells, as well as abnormal cytokine production by CD4+ T cells, in patients with T2D following infection (55). In addition, HbA1c was shown to positively correlate with the course of infections induced by different pathogens, impacting both disease duration and severity (51). This mechanism could be related to the stimulatory effect of hyperglycemia on the replication of several pathogens (54), impeding the ability of the immune system to fight infectious agents. Thus, our findings showed that poor glycemic control or the use of insulin analogs instead of human insulin increased the risk of PCS development in T2D patients after COVID-19.
We also found that a history of myocardial infarction or stroke can significantly increase the risk of PCS. This finding is consistent with previous findings demonstrating the impact of coronary artery disease on increasing the rate of PCS. Similarly, cardiovascular comorbidities and cerebrovascular events were also shown to enhance the probability of PCS (56). Both myocardial infarction and stroke have common mechanisms in their pathogenesis based on the compromised regulation of the blood clotting system (platelet aggregation and coagulation cascades), endothelial dysfunction, mild long-term inflammation and oxidative stress (57). Importantly, most of these pathophysiological processes interact with theories about the mechanisms underlying PCS development. In addition to persisting viral reservoirs and sustained inflammation, with autoimmune components, dysfunction of the endothelium and corresponding alterations in blood clotting have been underscored in PCS pathogenesis (58, 59). Notably, endothelial dysfunction, inflammation and blood clotting are closely related to myocardial infarction and stroke. These mechanisms are also main players in diabetic progression and complication development. Therefore, patients who experience myocardial infarction and stroke are at high risk for PCS and should be considered for preventing PCS complications. Thus, patients who experience severe COVID-19, especially those on mechanical ventilation, are at greater risk for long-term PCS, which can affect both their physical and mental health.
Finally, we found that patients with T2D manifested during acute COVID-19 infections more frequently observed in normal/overweight persons and characterized with more aggressive presentation as compared to matched patient with onset of T2D before COVID-19. This finding addresses recent scientific discussions of a new specific type of diabetes. It’s still debatable if this phenomenon represents abrupt onset of classical type 1 and type 2 diabetes or a new type of diabetes? Preliminary studies have provided evidence that β-cell infection may be involved in COVID-19 pathogenesis or, alternatively, that pancreatic infection may impact β cells by changing their local microenvironment. The precise underlying mechanisms are not clearly defined, the existing research studies suggests that the pathogenesis of new-onset diabetes due to COVID-19 might be linked to direct viral effects on pancreatic islets as well as systemic inflammatory responses that disrupt glucose metabolism (60). SARSCoV-2 uses ACE2 to enter human cells and TMPRSS2 for ‘priming’ (61). Both proteins are highly expressed in gastrointestinal epithelial cells, pancreatic ductal, acinar and islet cells (62). SARS-CoV-2 is also able to cause diffuse severe endotheliitis of the submucosal vessels in several anatomical sites, and these changes, in turn, cause diffuse microischemic disease (63). Similar ischemic damage could occur in the pancreas due to expression of ACE2 isoform in pancreatic microvasculature (64). Infected pancreatic islets demonstrated reduced glucose-stimulated insulin secretion, fewer insulin granules (65, 66) and characterized with increased islet-cell apoptosis hat may be due to the viral spike protein (60). Müller et al. also suggested that infected cells may lose their hormone content via de-differentiation (65). Data from current studies at least partly could give the background for more aggressive presentation of T2D obtained by our results.
The study was based on questionnaire results that can impact the accuracy of the data and details concerning treatment regimens. When recording the COVID-19 history, there were no data documenting the test systems used for the diagnostics, viral load and vaccination against SARS-CoV-2.
This study revealed several risk factors facilitating PCS development in T2D patients in Ukraine. We found that patients who were newly diagnosed with T2D, had an HbA1c above 9.2%, had previous cardiovascular or cerebrovascular events, and had severe COVID-19 associated with mechanical lung ventilation were at high risk for PCS development. The developed predictive PNN model allows us to assess the probability of PCS in T2D patients and identify high-risk groups for tailoring their treatment during viral infection.
New-onset T2D which occur during or after acute COVID-19 phase more frequently observed in normal/overweight persons and characterized with more aggressive presentation as compared to matched patient with onset of T2D before COVID-19.
The raw data supporting the conclusions of this article will be made available by the authors, without undue reservation.
The studies involving humans were approved by Bogomolets National Medical University. The studies were conducted in accordance with the local legislation and institutional requirements. The participants provided their written informed consent to participate in this study.
AM: Conceptualization, Data curation, Formal analysis, Validation, Writing – original draft. VY: Investigation, Methodology, Writing – original draft. SZ: Formal analysis, Methodology, Writing – review & editing. YI: Investigation, Methodology, Writing – original draft. VG: Data curation, Formal analysis, Methodology, Writing – review & editing. ZS: Investigation, Methodology, Writing – review & editing. TF: Formal analysis, Methodology, Writing – review & editing. OS: Conceptualization, Data curation, Methodology, Project administration, Supervision, Writing – original draft. NK: Conceptualization, Formal analysis, Funding acquisition, Investigation, Project administration, Supervision, Writing – original draft.
The author(s) declare that financial support was received for the research, authorship, and/or publication of this article. The work resulting in this publication was funded by the National Research Foundation of Ukraine under grant agreement No. 2022.01/0089 “The optimization of medical care to patients with type 2 diabetes during the war and postwar periods”.
The authors declare that the research was conducted in the absence of any commercial or financial relationships that could be construed as potential conflicts of interest.
The author(s) declared that they were an editorial board member of Frontiers, at the time of submission. This had no impact on the peer review process and the final decision.
All claims expressed in this article are solely those of the authors and do not necessarily represent those of their affiliated organizations, or those of the publisher, the editors and the reviewers. Any product that may be evaluated in this article, or claim that may be made by its manufacturer, is not guaranteed or endorsed by the publisher.
1. Ong KL, Stafford LK, McLaughlin SA, Boyko EJ, Vollset SE, Smith AE, et al. Global, regional, and national burden of diabetes from 1990 to 2021, with projections of prevalence to 2050: a systematic analysis for the Global Burden of Disease Study 2021. Lancet (London England). (2023) 402:203–34. doi: 10.1016/S0140-6736(23)01301-6
2. Yousri NA, Suhre K, Yassin E, Al-Shakaki A, Robay A, Elshafei M, et al. Metabolic and metabo-clinical signatures of type 2 diabetes, obesity, retinopathy, and dyslipidemia. Diabetes. (2022) 71:184–205. doi: 10.2337/db21-0490
3. Marushchak M, Kozak K, Krynytska I. Comorbid overweight/obesity and chronic pancreatitis exacerbate the dyslipidemia progression in type 2 diabetic patients. Endocrine Regulations. (2022) 56:168–77. doi: 10.2478/enr-2022-0018
4. Sharma A, Tiwari S, Deb MK, Marty JL. Severe acute respiratory syndrome coronavirus-2 (SARS-CoV-2): a global pandemic and treatment strategies. Int J antimicrobial Agents. (2020) 56:106054. doi: 10.1016/J.IJANTIMICAG.2020.106054
5. Sulaieva O, Dudin O, Botsun P, Pischanska S, Karpachova V, Omelianenko I, et al. The detrimental consequences of two consecutive disasters impacting cytopathology in Ukraine: COVID followed by the war. Cytopathology: Off J Br Soc Clin Cytology. (2023) 34:442–9. doi: 10.1111/CYT.13236
6. Buchynskyi M, Kamyshna I, Lyubomirskaya K, Moshynets O, Kobyliak N, Oksenych V, et al. Efficacy of interferon alpha for the treatment of hospitalized patients with COVID-19: A meta-analysis. Front Immunol. (2023) 14:1069894. doi: 10.3389/FIMMU.2023.1069894
7. Abumayyaleh M, Núñez Gil IJ, Viana-LLamas MC, Raposeiras Roubin S, Romero R, Alfonso-Rodríguez E, et al. Post-COVID-19 syndrome and diabetes mellitus: a propensity-matched analysis of the International HOPE-II COVID-19 Registry. Front Endocrinol. (2023) 14:1167087. doi: 10.3389/FENDO.2023.1167087
8. Petakh P, Kobyliak N, Kamyshnyi A. Gut microbiota in patients with COVID-19 and type 2 diabetes: A culture-based method. Front Cell infection Microbiol. (2023) 13:1142578. doi: 10.3389/FCIMB.2023.1142578
9. Cao H, Baranova A, Wei X, Wang C, Zhang F. Bidirectional causal associations between type 2 diabetes and COVID-19. J Med Virol. (2023) 95:e28100. doi: 10.1002/JMV.28100
10. Pranata R, Henrina J, Raffaello WM, Lawrensia S, Huang I. Diabetes and COVID-19: The past, the present, and the future. Metabolism: Clin Exp. (2021) 121:154814. doi: 10.1016/J.METABOL.2021.154814
11. Nazari P, Pozzilli P. Type 2 diabetes and Covid-19: Lessons learnt, unanswered questions and hints for the future. Diabetes Res Clin Pract. (2023) 204:110896. doi: 10.1016/J.DIABRES.2023.110896
12. Rajpal A, Rahimi L, Ismail-Beigi F. Factors leading to high morbidity and mortality of COVID-19 in patients with type 2 diabetes. J Diabetes. (2020) 12:895–908. doi: 10.1111/1753-0407.13085
13. Yin Y, Rohli KE, Shen P, Lu H, Liu Y, Dou Q, et al. The epidemiology, pathophysiological mechanisms, and management toward COVID-19 patients with Type 2 diabetes: A systematic review. Primary Care Diabetes. (2021) 15:899–909. doi: 10.1016/J.PCD.2021.08.014
14. Wu KCH, He Q, Bennett AN, Li J, Chan KHK. Shared genetic mechanism between type 2 diabetes and COVID-19 using pathway-based association analysis. Front Genet. (2022) 13:1063519. doi: 10.3389/FGENE.2022.1063519
15. Khunti K, Prato SD, Mathieu C, Kahn SE, Gabbay RA, Buse JB. COVID-19, hyperglycemia, and new-onset diabetes. Diabetes Care. (2021) 44:2645–55. doi: 10.2337/DC21-1318
16. Kim SH, Arora I, Hsia DS, Knowler WC, LeBlanc E, Mylonakis E, et al. New-onset diabetes after COVID-19. J Clin Endocrinol Metab. (2023) 108:E1164–74. doi: 10.1210/CLINEM/DGAD284
17. Mittal J, Ghosh A, Bhatt SP, Anoop S, Ansari IA, Misra A. High prevalence of post COVID-19 fatigue in patients with type 2 diabetes: A case-control study. Diabetes Metab syndrome. (2021) 15:102302. doi: 10.1016/j.dsx.2021.102302
18. Raveendran AV, Misra A. Post COVID-19 syndrome (“Long COVID”) and diabetes: challenges in diagnosis and management. Diabetes Metab syndrome. (2021) 15:102235. doi: 10.1016/J.DSX.2021.102235
19. Matviichuk A, Yerokhovych V, Ilkiv Y, Krasnienkov D, Korcheva V, Gurbych O, et al. HbA1c and leukocyte mtDNA levels as major factors associated with post-COVID-19 syndrome in type 2 diabetes patients. Sci Rep. (2024) 14:25533. doi: 10.1038/S41598-024-77496-2
20. Post COVID-19 condition . Available online at: https://www.who.int/teams/health-care-readiness/post-covid-19-condition (Accessed November 13, 2024).
21. Xie Y, Al-Aly Z. Risks and burdens of incident diabetes in long COVID: a cohort study. Lancet Diabetes Endocrinol. (2022) 10:311–21. doi: 10.1016/S2213-8587(22)00044-4
22. Harding JL, Oviedo SA, Ali MK, Ofotokun I, Gander JC, Patel SA, et al. The bidirectional association between diabetes and long-COVID-19 - A systematic review. Diabetes Res Clin Pract. (2023) 195:110202. doi: 10.1016/J.DIABRES.2022.110202
23. Kirbiš S, Sobotkiewicz N, Schaubach BA, Završnik J, Kokol P, Završnik M, et al. The effects of diabetes and being overweight on patients with post-COVID-19 syndrome. Infect Dis Rep. (2023) 15:747–57. doi: 10.3390/IDR15060067
24. Lai H, Yang M, Sun M, Pan B, Wang Q, Wang J, et al. Risk of incident diabetes after COVID-19 infection: A systematic review and meta-analysis. Metabolism: Clin Exp. (2022) 137:155330. doi: 10.1016/J.METABOL.2022.155330
25. Clinical management of COVID-19 . Available online at: https://www.who.int/teams/health-care-readiness/covid-19 (Accessed March 27, 2024).
26. Hegazy MA, Lithy RM, Abdel-Hamid HM, Wahba M, Ashoush OA, Hegazy MT, et al. COVID-19 disease outcomes: does gastrointestinal burden play a role? Clin Exp Gastroenterol. (2021) 14:199–207. doi: 10.2147/CEG.S297428
27. Nance SA, Muir L, Lumeng C. Adipose tissue macrophages: Regulators of adipose tissue immunometabolism during obesity. Mol Metab. (2022) 66:101642. doi: 10.1016/J.MOLMET.2022.101642
28. El-Sayed Moustafa JS, Jackson AU, Brotman SM, Guan L, Villicaña S, Roberts AL, et al. ACE2 expression in adipose tissue is associated with cardio-metabolic risk factors and cell type composition-implications for COVID-19. Int J Obes (2005). (2022) 46:1478–86. doi: 10.1038/S41366-022-01136-W
29. Mykhalchyshyn G, Kobyliak N, Bodnar P. Diagnostic accuracy of acyl-ghrelin and it association with non-alcoholic fatty liver disease in type 2 diabetic patients. J Diabetes Metab Disord. (2015) 14:44. doi: 10.1186/s40200-015-0170-1
30. D’Souza F, Buzzetti R, Pozzilli P. Diabetes, COVID-19, and questions unsolved. Diabetes/metabolism Res Rev. (2023) 39:e3666. doi: 10.1002/DMRR.3666
31. Maglietta G, Diodati F, Puntoni M, Lazzarelli S, Marcomini B, Patrizi L, et al. Prognostic factors for post-COVID-19 syndrome: A systematic review and meta-analysis. J Clin Med. (2022) 11:1541. doi: 10.3390/JCM11061541
32. Lemhöfer C, Bahmer T, Baumbach P, Besteher B, Boekel A, Finke K, et al. Variations and predictors of post-COVID syndrome severity in patients attending a post-COVID outpatient clinic. J Clin Med. (2023) 12:4013. doi: 10.3390/JCM12124013
33. Zemni I, Gara A, Bennasrallah C, Ezzar S, Kacem M, Chokri R, et al. Incidence and risk factors of post COVID-19 syndrome: a Tunisian cohort study. BMC Infect Dis. (2024) 24:461. doi: 10.1186/S12879-023-08949-8
34. Fernóandez-De-las-Penas C, Guijarro C, Torres-Macho J, Velasco-Arribas M, Plaza-Canteli S, Hernóandez-Barrera V, et al. Diabetes and the risk of long-term post-COVID symptoms. Diabetes. (2021) 70:2917–21. doi: 10.2337/DB21-0329
35. Nassar M, Abosheaishaa H, Singh AK, Misra A, Bloomgarden Z. Noninsulin-based antihyperglycemic medications in patients with diabetes and COVID-19: A systematic review and meta-analysis. J Diabetes. (2023) 15:86–96. doi: 10.1111/1753-0407.13359
36. Nguyen NN, Ho DS, Nguyen HS, Ho DKN, Li HY, Lin CY, et al. Preadmission use of antidiabetic medications and mortality among patients with COVID-19 having type 2 diabetes: A meta-analysis. Metabolism: Clin Exp. (2022) 131:155196. doi: 10.1016/J.METABOL.2022.155196
37. Dimnjaković J, Buble T, Ivanko P, Pristaš I, Brborović O, Brborović H. Association of anti-diabetic drugs and COVID-19 outcomes in patients with diabetes mellitus type 2 and cardiomyopathy. Sci Rep. (2024) 14:7227. doi: 10.1038/S41598-024-57871-9
38. Pérez-Belmonte LM, Torres-Peña JD, López-Carmona MD, Ayala-Gutiérrez MM, Fuentes-Jiménez F, Huerta LJ, et al. Mortality and other adverse outcomes in patients with type 2 diabetes mellitus admitted for COVID-19 in association with glucose-lowering drugs: a nationwide cohort study. BMC Med. (2020) 18:359. doi: 10.1186/S12916-020-01832-2
39. MCCarthy MW. Metformin as a potential treatment for COVID-19. Expert Opin pharmacotherapy. (2023) 24:1199–203. doi: 10.1080/14656566.2023.2215385
40. Wise J. Covid-19: Metformin reduces the risk of developing long term symptoms by 40%, study finds. BMJ (Clinical Res ed). (2023) 381:p1306. doi: 10.1136/BMJ.P1306
41. COVID-19 update: Metformin to prevent long COVID? Med letter Drugs Ther. (2023) 65:87–8. doi: 10.58347/TML.2023.1677E
42. Bramante CT, Buse JB, Liebovitz DM, Nicklas JM, Puskarich MA, Cohen K, et al. Outpatient treatment of COVID-19 and incidence of post-COVID-19 condition over 10 months (COVID-OUT): a multicentre, randomised, quadruple-blind, parallel-group, phase 3 trial. Lancet Infect Dis. (2023) 23:1119–29. doi: 10.1016/S1473-3099(23)00299-2
43. Lima-Martínez MM, Carrera Boada C, Madera-Silva MD, Marín W, Contreras M. COVID-19 and diabetes: A bidirectional relationship. Clinica e investigacion en arteriosclerosis: publicacion oficial la Sociedad Espanola Arterioscler. (2021) 33:151–7. doi: 10.1016/J.ARTERI.2020.10.001
44. Heald AH, Williams R, Jenkins DA, Stewart S, Bakerly ND, Mccay K, et al. The prevalence of long COVID in people with diabetes mellitus-evidence from a UK cohort. EClinicalMedicine. (2024) 71:102607. doi: 10.1016/J.ECLINM.2024.102607
45. Wu J, Zhang J, Sun X, Wang L, Xu Y, Zhang Y, et al. Influence of diabetes mellitus on the severity and fatality of SARS-CoV-2 (COVID-19) infection. Diabetes Obes Metab. (2020) 22:1907–14. doi: 10.1111/DOM.14105
46. Steenblock C, Hassanein M, Khan EG, Yaman M, Kamel M, Barbir M, et al. Diabetes and COVID-19: short- and long-term consequences. Hormone Metab Res = Hormon- und Stoffwechselforschung = Hormones metabolisme. (2022) 54:503–9. doi: 10.1055/A-1878-9566
47. Primorac D, Vrdoljak K, Brlek P, Pavelić E, Molnar V, Matišić V, et al. Adaptive immune responses and immunity to SARS-coV-2. Front Immunol. (2022) 13:848582. doi: 10.3389/FIMMU.2022.848582
48. Fleming N, Sacks LJ, Pham CT, Neoh SL, Ekinci EI. An overview of COVID-19 in people with diabetes: Pathophysiology and considerations in the inpatient setting. Diabetic medicine: J Br Diabetic Assoc. (2021) 38:e14509. doi: 10.1111/DME.14509
49. Fedotkina O, Sulaieva O, Ozgumus T, Cherviakova L, Khalimon N, Svietleisha T, et al. Novel reclassification of adult diabetes is useful to distinguish stages of β-cell function linked to the risk of vascular complications: the DOLCE study from northern Ukraine. Front Genet. (2021) 12:637945. doi: 10.3389/FGENE.2021.637945
50. Feldman EL, Savelieff MG, Hayek SS, Pennathur S, Kretzler M, Pop-Busui R. COVID-19 and diabetes: A collision and collusion of two diseases. Diabetes. (2020) 69:2549–65. doi: 10.2337/DBI20-0032
51. Turk Wensveen T, Gašparini D, Rahelić D, Wensveen FM. Type 2 diabetes and viral infection; cause and effect of disease. Diabetes Res Clin Pract. (2021) 172:108637. doi: 10.1016/J.DIABRES.2020.108637
52. Tsai S, Clemente-Casares X, Zhou AC, Lei H, Ahn JJ, Chan YT, et al. Insulin receptor-mediated stimulation boosts T cell immunity during inflammation and infection. Cell Metab. (2018) 28:922–934.e4. doi: 10.1016/J.CMET.2018.08.003
53. Šestan M, Marinović S, Kavazović I, Cekinović Đ, Wueest S, Turk Wensveen T, et al. Virus-induced interferon-γ Causes insulin resistance in skeletal muscle and derails glycemic control in obesity. Immunity. (2018) 49:164–177.e6. doi: 10.1016/J.IMMUNI.2018.05.005
54. Geerlings SE, Hoepelman AI. Immune dysfunction in patients with diabetes mellitus (DM). FEMS Immunol Med Microbiol. (1999) 26:259–65. doi: 10.1111/J.1574-695X.1999.TB01397.X
55. Kumar NP, Sridhar R, Nair D, Banurekha VV, Nutman TB, Babu S. Type 2 diabetes mellitus is associated with altered CD8(+) T and natural killer cell function in pulmonary tuberculosis. Immunology. (2015) 144:677–86. doi: 10.1111/IMM.12421
56. Vigili de Kreutzenberg S. Long COVID-19 and diabetes mellitus: a short review. Metab Target Organ Damage. (2023) 3:4. doi: 10.20517/MTOD.2022.30
57. Li J, Zhou Y, Ma J, Zhang Q, Shao J, Liang S, et al. The long-term health outcomes, pathophysiological mechanisms and multidisciplinary management of long COVID. Signal transduction targeted Ther. (2023) 8:416. doi: 10.1038/S41392-023-01640-Z
58. Mehandru S, Merad M. Pathological sequelae of long-haul COVID. Nat Immunol. (2022) 23:194–202. doi: 10.1038/S41590-021-01104-Y
59. Sherif ZA, Gomez CR, Connors TJ, Henrich TJ, Reeves WB. Pathogenic mechanisms of post-acute sequelae of SARS-CoV-2 infection (PASC). eLife. (2023) 12:e86002. doi: 10.7554/ELIFE.86002
60. Wu CT, Lidsky PV, Xiao Y, Lee IT, Cheng R, Nakayama T, et al. SARS-CoV-2 infects human pancreatic β cells and elicits β cell impairment. Cell Metab. (2021) 33:1565–1576.e5. doi: 10.1016/J.CMET.2021.05.013
61. de-Madaria E, Capurso G. COVID-19 and acute pancreatitis: examining the causality. Nat Rev Gastroenterol Hepatol. (2021) 18:3–4. doi: 10.1038/S41575-020-00389-Y
62. Hamming I, Timens W, Bulthuis MLC, Lely AT, Navis GJ, van Goor H. Tissue distribution of ACE2 protein, the functional receptor for SARS coronavirus. A first step in understanding SARS pathogenesis. J Pathol. (2004) 203:631–7. doi: 10.1002/PATH.1570
63. Pons S, Fodil S, Azoulay E, Zafrani L. The vascular endothelium: the cornerstone of organ dysfunction in severe SARS-CoV-2 infection. Crit Care (London England). (2020) 24:353. doi: 10.1186/S13054-020-03062-7
64. Fignani D, Licata G, Brusco N, Nigi L, Grieco GE, Marselli L, et al. SARS-coV-2 receptor angiotensin I-converting enzyme type 2 (ACE2) is expressed in human pancreatic β-cells and in the human pancreas microvasculature. Front Endocrinol. (2020) 11:596898. doi: 10.3389/FENDO.2020.596898
65. Müller JA, Groß R, Conzelmann C, Krüger J, Merle U, Steinhart J, et al. SARS-CoV-2 infects and replicates in cells of the human endocrine and exocrine pancreas. Nat Metab. (2021) 3:149–65. doi: 10.1038/S42255-021-00347-1
Keywords: post-COVID-19 Syndrome, long COVID-19, COVID-19 infection, SARS-CoV-2, type 2 diabetes
Citation: Matviichuk A, Yerokhovych V, Zemskov S, Ilkiv Y, Gurianov V, Shaienko Z, Falalyeyeva T, Sulaieva O and Kobyliak N (2024) Unveiling risk factors for post-COVID-19 syndrome development in people with type 2 diabetes. Front. Endocrinol. 15:1459171. doi: 10.3389/fendo.2024.1459171
Received: 03 July 2024; Accepted: 27 November 2024;
Published: 11 December 2024.
Edited by:
Kamyar Asadipooya, University of Kentucky, United StatesReviewed by:
Charlotte Steenblock, Technical University Dresden, GermanyCopyright © 2024 Matviichuk, Yerokhovych, Zemskov, Ilkiv, Gurianov, Shaienko, Falalyeyeva, Sulaieva and Kobyliak. This is an open-access article distributed under the terms of the Creative Commons Attribution License (CC BY). The use, distribution or reproduction in other forums is permitted, provided the original author(s) and the copyright owner(s) are credited and that the original publication in this journal is cited, in accordance with accepted academic practice. No use, distribution or reproduction is permitted which does not comply with these terms.
*Correspondence: Nazarii Kobyliak, bmF6YXJpaWtvYnlsaWFrQGdtYWlsLmNvbQ==
Disclaimer: All claims expressed in this article are solely those of the authors and do not necessarily represent those of their affiliated organizations, or those of the publisher, the editors and the reviewers. Any product that may be evaluated in this article or claim that may be made by its manufacturer is not guaranteed or endorsed by the publisher.
Research integrity at Frontiers
Learn more about the work of our research integrity team to safeguard the quality of each article we publish.