- 1Department of Applied Biomedical Science, Faculty of Health Sciences, University of Malta, Msida, Malta
- 2Centre for Molecular Medicine and Biobanking, University of Malta, Msida, Malta
Osteoporosis is a multifactorial bone disease characterised by reduced bone mass and increased fracture risk. Family studies have made significant contribution in unravelling the genetics of osteoporosis. Yet, most of the underlying molecular and biological mechanisms remain unknown prompting the need for further studies. This review outlines the proper phenotyping and advanced genetic techniques in the form of high-throughput DNA sequencing used to identify genetic factors underlying monogenic osteoporosis in a family-based setting. The steps related to variant filtering prioritisation and curation are also described. From an evolutionary perspective, deleterious risk variants with higher penetrance tend to be rare as a result of negative selection. High-throughput sequencing (HTS) can identify rare variants with large effect sizes which are likely to be missed by candidate gene analysis or genome-wide association studies (GWAS) wherein common variants with small to moderate effect sizes are identified. We also describe the importance of replicating implicated genes, and possibly variants, identified following HTS to confirm their causality. Replication of the gene in other families, singletons or independent cohorts confirms that the shortlisted genes and/or variants are indeed causal. Furthermore, novel genes and/or variants implicated in monogenic osteoporosis require a thorough validation by means of in vitro and in vivo assessment. Therefore, analyses of families can continue to elucidate the genetic architecture of osteoporosis, paving the way for improved diagnostic and therapeutic strategies.
1 Introduction
Osteoporosis is a progressive skeletal disorder characterised by low bone mass and degradation of the microarchitecture of bone tissue that subsequently decrease bone strength and increase susceptibility to fragility fractures, such as those occurring from standing height or less (1, 2). Osteoporosis is considered a ‘silent disease’ until the first fracture occurs (3). The most sustained fractures are those of the hip, spine, wrist and humerus, resulting in increased morbidity, need for hospital care and institutionalisation. Moreover, spine and hip fractures can also result in death due to secondary complications (4). Consequently, osteoporosis is associated with a significant burden on the global economy and healthcare system with costs expected to increase due to a rise in the ageing population. Globally, osteoporosis is estimated to affect one in three women and one in five men over the age of 50 years (5). The prevalence of osteoporosis in the United States in the years 2017-2018 was approximately 12.6% among adults aged 50 years and over (6). In 2019, 32 million people in the EU27 + 2 (European Union countries, Switzerland, and the United Kingdom) were reported to have osteoporosis (7).
Osteoporosis may arise due to an imbalance in the bone remodelling equilibrium with a bone resorption rate that exceeds bone formation, failure to reach peak bone mass at the young adult stage, and/or due to physiological changes occurring in the body with advancing age such as oestrogen deficiency (6). All three scenarios are influenced by environmental (such as diet, smoking, alcohol intake and physical activity) and genetic factors (e.g., gender, low birth weight, early menopause, and low body mass index) (7). The risk exerted by these factors varies, with genetic factors imposing one of the strongest effects. Indeed, twin and family studies have shown that 50-85% of the changes in the bone mineral density (BMD) are genetically determined (8, 9). Genome-wide association studies (GWAS) have identified several gene variants as potential contributors of bone mass determination and fracture risk, each exerting modest effect sizes. In contrast, monogenic forms of osteoporosis are caused by a single gene variant that plays a significant role in skeletal development. Family studies harbouring multi-generation, affected relatives have aided in the identification of high-impact disease-causing genes and variants, some of which replicated at the population level. This brief review aims to provide an overview of the promising application of a family-based study approach to uncover the underlying genetic determinants of osteoporosis following high-throughput sequencing (HTS).
2 Family-based study design
2.1 Benefit of families in genetic study approaches
Family-based studies have successfully been used to identify genes underlying a variety of monogenic, highly penetrant disorders such as Duchenne muscular dystrophy (OMIM: 310200) (10, 11), Huntington disease (OMIM: 43100) (12), and cystic fibrosis (OMIM: 219700) (13). Generally, complex traits are differentiated from monogenic disorders in that: (i) they are more prevalent; (ii) do not demonstrate clean mendelian segregation patterns suggesting a polygenic cause whereby multiple gene variants (possibly involved in different signalling cascades) having different effect sizes collectively contribute to the phenotype; and (iii) the marginal effect of any single gene on a relevant clinical end point is likely to be small (14). The use of family studies having multiple affected members overcomes such issues. Identifying genes through family studies serves as a foundation in population-based research, addressing the various challenges inherent in analysing the genetic makeup of complex traits (15–18).
Family studies offer several benefits for gene discovery as opposed to studies of unrelated individuals (Figure 1). This rests on the fact that family members are more likely to possess a homogenous and limited set of causative genes. Therefore, the statistical power for gene discovery is enhanced and findings can be replicated across other affected families. Compared to population studies, unrelated or related but unaffected relatives serve as good controls, most noticeably since they share common environmental influences and have a similar socioeconomic status with affected relatives. In addition, unaffected relatives share a good proportion of their genes, that can be used to control background genetic variation shifting the focus to potential causal variants (15, 18). Despite being unbiased, this approach requires correct and extensive pedigree information, along with good phenotyping and a distant relative or control to help in variant filtering.
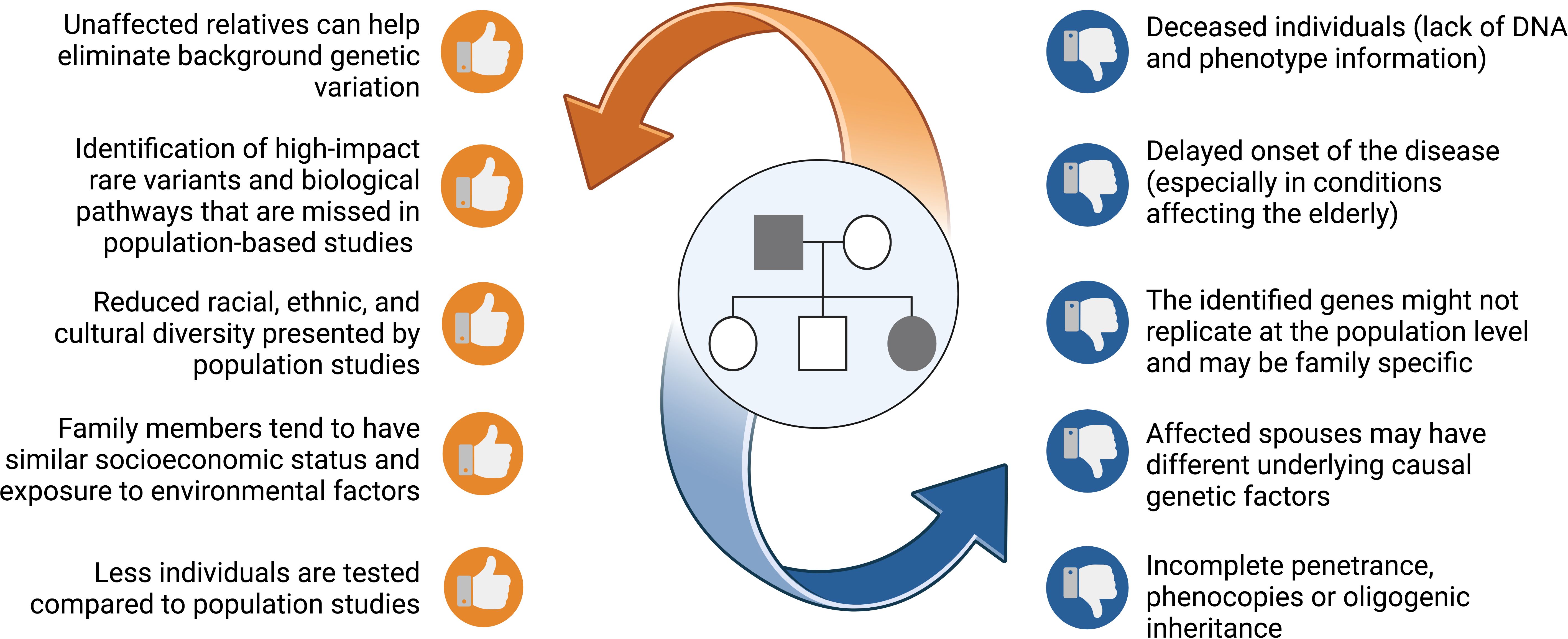
Figure 1. A list of benefits and risks that families offer when pursing genetic studies. Figure created using BioRender.
2.2 Successful use of family-based studies in bone diseases
Prior to the completion of the human genome project, highly penetrant variants with large effect sizes were identified using linkage analysis in families with monogenic bone disorders. This approach is based on the principle of identity-by-descent in combination with phenotypic information to identify the common gene loci shared amongst affected family members. The biological phenomenon of linkage analysis is that the closer the genetic marker is to the causative gene, the less likely it is to be separated by meiotic recombination between generations (19). Linkage analysis studies have identified a number of genes responsible for rare monogenic bone disorders including sclerosteosis (SOST; OMIM: 605740) (20), van Buchem disease (SOST; OMIM: 605740) (21), osteogenesis imperfecta (OI) including type I, II, III and IV (COL1A1; OMIM: 120150 and COL1A2; OMIM: 120160) (22, 23), osteoporosis-pseudoglioma syndrome (LRP5: OMIM: 603506) (24), and osteopetrosis (CLCN7: OMIM: 602727) (25). These genes have provided a better understanding of bone pathophysiology, some of which have paved the way for the development of targeted osteoanabolic therapy such as in the case of Romosozumab (26).
The cost effectiveness of HTS, coupled with its faster turnaround time, increased access, and wealth of data generated has led to a shift in the type of genetic testing undertaken, with omics technology favoured over classical linkage analysis and Sanger sequencing. HTS can be used to investigate genetic variation within exons (whole exome sequencing; WES), targeted genes (specific genes forming part of a panel that are known to contribute to disease) or the entire genome (whole genome sequencing; WGS). Short-read HTS has been instrumental in unravelling the genes underlying monogenic osteoporosis, reviewed in detail elsewhere (27–29). Independent family studies followed by WES have identified variants in the WNT1 (OMIM: 164820) involved in early-onset osteoporosis (EOOP) and OI type III (30, 31), and PLS3 (OMIM:30013) in four Dutch families with X-linked osteoporosis, with a specific PLS3 variant successfully replicating at the population level (32). Several other causal PLS3 mutations have since been reported in EOOP, including missense, nonsense and structural variants (SVs) (33–35). HTS has also identified two novel loss-of-function mutations in LRP5 (OMIM: 603506) that provided further knowledge on the role of this gene in canonical WNT signalling and its effect in the development of juvenile-onset primary osteoporosis (OMIM: 619884) (36). Besides osteoporosis, LRP5 variants have been implicated in high bone mass disorders and have also achieved genome-wide significance with hip and spine BMD (37–39). Other studies using a family-based approach combined with HTS have successfully identified genes with a clear-cut role in bone physiology including, SGMS2 (OMIM: 611574) (40), ARHGAP25 (OMIM: 610587) (41), WNT11 (OMIM: 603699) (42), RUNX1 (OMIM: 151385) (43), and FGFR2 (OMIM: 176943) (44). Besides osteoporosis, this study design has also been used to uncover genetic determinants in other diseases such as Alzheimer’s disease (45), Parkinson’s disease (46), diabetes mellitus (47–49) and cardiovascular disease (50, 51).
3 Gene discovery using family studies
The following steps are recommended to identify rare, high-risk DNA variants in individuals with suspected monogenic osteoporosis (Figure 2): recruitment of family members, rigorous deep phenotyping, genetic evaluation of HTS data including variant filtering and prioritisation, co-segregation of variants in the entire pedigree, and if possible, detection of the deleterious gene and/or variant in other families and/or population studies.
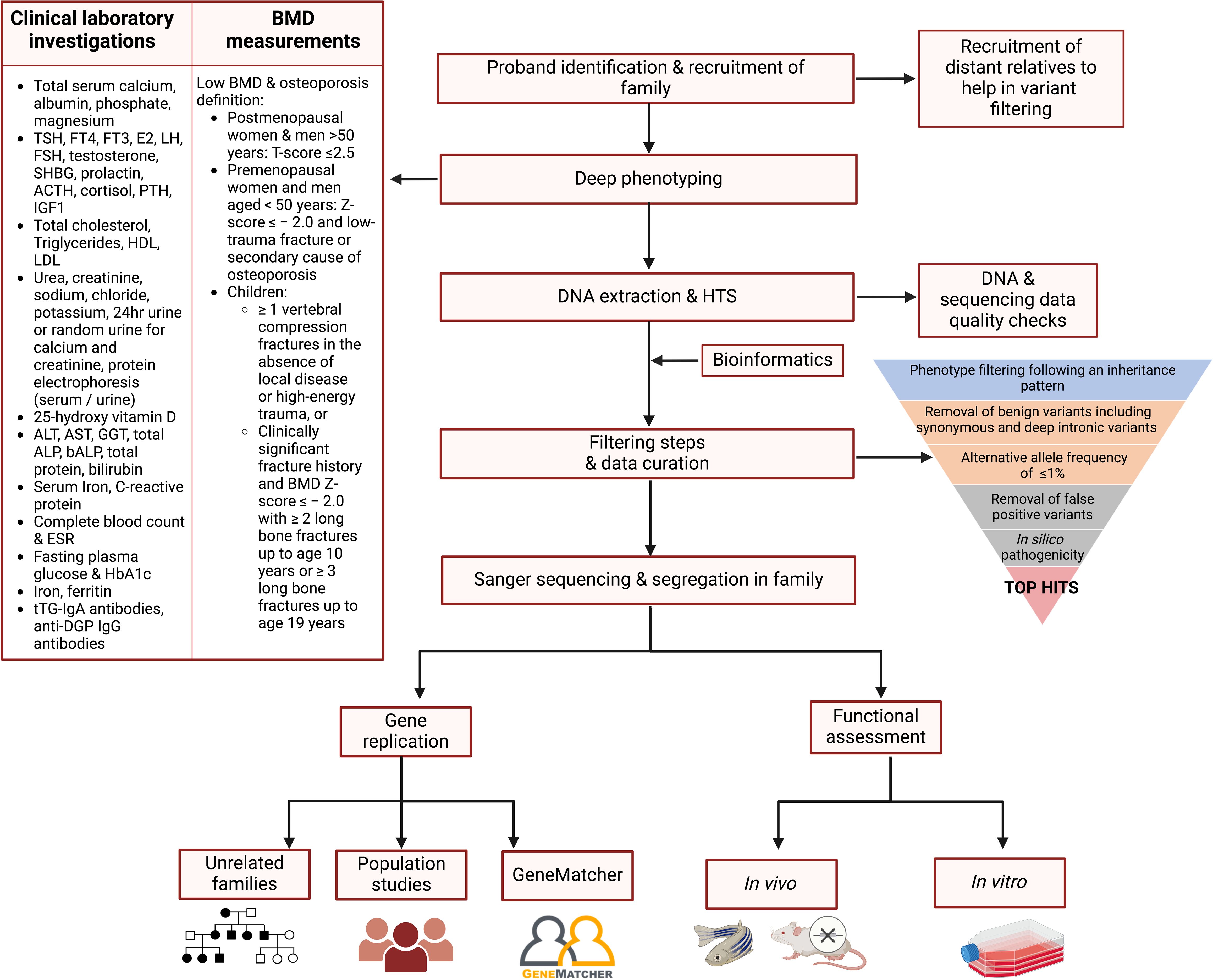
Figure 2. Proposed flowchart for identifying the genetic variants contributing to osteoporosis in a family study. Affected proband and relatives are recruited and subjected to deep phenotyping followed by genetic evaluation using HTS. A stepwise filtering scheme is applied with the aim of filtering out common, benign, and low-penetrance variants that are unlikely to be causal, retaining high-impact variants possibly residing in genes having a role in bone biology. Segregation of the variants in the entire pedigree is confirmed by Sanger sequencing. The shortlisted variants are tested in other independent families and/or population studies, and functionally validated using cells or animal models to confirm their role in bone metabolism and osteoporosis pathogenesis. ACTH, adrenocorticotropic hormone; ALP, alkaline phosphatase; ALT, alanine transaminase; AST, aspartate transaminase; DGP, deamidated gliadin peptide; E2, oestradiol; ESR, erythrocyte sedimentation rate; FSH, follicle-stimulating hormone; FT3, free T3; FT4, free T4; GGT, gamma-glutamyltransferase; HbA1c, glycosylated haemoglobin; HDL, high-density lipoprotein; IGF1, insulin-like growth factor 1; LH, luteinising hormone; LDL, low-density lipoprotein; PTH, parathyroid hormone; SHBG, sex hormone-binding globulin; TSH, thyroid-stimulating hormone; tTG, tissue transglutaminase; 25-OH Vitamin D, 25-hydroxy vitamin D. Figure created using BioRender.
3.1 Recruitment of family members
Maximising the inclusion of both affected and unaffected individuals in multigenerational pedigrees enhances the likelihood of identifying causal variants. Affected family members should be directly related by blood (at least one affected relative in each generation), with a minimal number of affected spouses. A thorough clinical history for all recruited individuals is crucial and should involve data concerning demographic and lifestyle factors such as physical activity, calcium and Vitamin D intake, alcohol consumption, smoking habits, age at menopause and medication use. A comprehensive fracture history should comprise of all fractures sustained from childhood and specify the age at which each fracture occurred, the site of fracture and the mechanism of fracture (low, moderate, or high-impact trauma). Family history of bone disease, osteoporosis and low-trauma fractures is also essential, irrespective of age.
3.2 Phenotyping of family members
Precise differentiation between affected and unaffected relatives is crucial, as incorrect phenotyping can hinder the identification of causal gene variants. Laboratory analyses should include relevant biochemical parameters of coexisting diseases that may affect bone homeostasis (52–54). Furthermore, anthropometric measurements should also be recorded using validated equipment which is calibrated according to WHO guidelines (55). Dual-energy X-ray absorptiometry (DXA) remains the gold standard for the non-invasive measurement of BMD in all age groups, including children and young adults thanks to its worldwide availability, precision, reproducibility, and availability of normative data (54). BMD measurements are expressed in T-scores and Z-scores based on the WHO classification and the International Society for Clinical Densitometry (ISCD) for ease of interpretation (56). An affected status may be defined by a T-score of ≤-2.5 in the case of postmenopausal women and men over the age of 50; and a Z-score below -2.0 accompanied by a low-trauma fracture history in the case of premenopausal women and men younger than 50 years (55). In the case of EOOP, an affected status in children and young adults is further defined in (29).
3.3 Genetic analysis and bioinformatics
Genomic DNA is typically isolated from peripheral blood leukocytes using ethylenediaminetetraacetic acid (EDTA) or citrated blood tubes or harvested buffy coat layers depending on blood volumes available. Where possible, aliquots of whole blood (with or without stabilisers), serum and plasma samples should be banked (at -80°C) for potential future omics studies (i.e., transcriptomics, metabolomics, proteomics). If phlebotomy is not possible, genomic DNA can be obtained from saliva or buccal swabs. High-quality genomic DNA is essential for accurate and comprehensive short-read sequencing, whereas high-molecular weight DNA is required for long-read sequencing (57). HTS should be carried out on the most genetically informative relatives based on the structure of the pedigree. WGS is becoming an attractive alternative to WES due to its broader coverage and decreasing costs. Unlike WES, WGS does not require enrichment and capture steps, leading to a more uniform coverage and improved detection of variants (58). If no significant variants are identified in the extended coding regions, non-coding variants can be further scrutinised. Before analysing variants, sequencing data must undergo quality control to avoid losing statistical power, minimising false positives and negative results (59). FastQC can be utilised to perform quality checks on raw HTS data providing modular analyses including pre-base analysis of sequencing reads with the aim of pinpointing sequencing artefacts that may affect downstream analyses (60). Subsequently, clean raw reads are aligned to the latest version of the human reference genome, such as UCSC Genome (61) or NCBI RefSeq (62). The most commonly used aligners for short read sequencing data are Burrows-Wheeler Aligner (63), MOSAIK (64), and Bowtie (65). The aligned reads are run through Picard tools to flag any duplicate reads arising from enrichment bias during sequencing. Tools such as FastQC (60) and fastp (66) can be used for duplicate removal. The following step is variant calling whereby aligned reads are compared to the reference genome and any nucleotide variations are identified. SNV (single nucleotide variants) and InDel (Insertions or Deletions) calling can be performed using specific tools such as Genome Analysis Tool Kit HaplotypeCaller (GATK-HC) (67), Samtools mpileup (68), DeepVariant (69), and varScan (70). Calling of SVs can be done by running the BAM files in either LUMPY (71) or Manta (72), and in the case of copy number variations also using CNVnator (73) or CNVkit (74). VEP (Variant Effect Predictor) (75) and SnpEff (76) are commonly employed to annotate variants based on the genomic location, and to predict the functional effect on a gene. Additionally, variant calling tools incorporate information related to: (i) alternative allele frequency from public databases such as the 1000 Genomes Project (77), the Single Nucleotide Polymorphism Database (dbSNP) (78), and Genome Aggregation Database (gnomAD) (79); (ii) conservation scores according to PhyloP (80) and the Genomic Evolutionary Rate Profiling (GERP) score (81); and (iii) in silico predictions of variant pathogenicity according to Polymorphism Phenotyping (PolyPhen-2) (82), Sorting Intolerant From Tolerant (SIFT) (83), The Likelihood Ratio Test (LRT) (84), Variant Effect Scoring tool (VEST3) (85), MutationTaster (86), MutationAssessor (87), MetaLR (88), Functional Analysis through Hidden Markov Models (FATHMM) (89), and Meta-analytic support vector machine (MetaSVM) (90), amongst others.
3.4 Variant filtering, prioritisation and curation
Based on a thorough literature review, the following filtering pipeline is recommended to narrow down the extensive list of gene variants, leaving a shortlist of potentially causal variants as described below.
i. Phenotype filtering following an inheritance pattern as observed for the studied family can help remove the shared benign genetic variants shifting the focus on causal gene variants.
ii. Removal of low impact variants including synonymous, as well as deep intronic and intergenic variants, in favour of nonsense (stopgain) and stoploss variants, missense, frameshift (including those in splicing regions or resulting in start/stop loss), and variants affecting splice sites (within 20 nucleotides upstream or downstream of exon-intron boundaries).
iii. Retaining of variants with an observed alternative allele frequency (AAF) of ≤1% in all population-based allele frequency databases, particularly gnomAD for SNVs, InDels and more recently also SVs, assuming the presence of rare and penetrant variants.
iv. Removal of recurrent false positive variants (also known as ‘frequent hitters’) resulting from assembly misalignment, variants falling in highly polymorphic areas and mislabelled variants due to misleading reference genome data (91, 92).
v. Prioritisation of variants based on in silico pathogenicity, Combined Annotation–Dependent Depletion (CADD) score (93), conservation scores, and classification by the American College of Medical Genetics and Genomics/Association for Molecular Pathology (ACMG/AMP) guidelines (94). Various in silico tools, particularly those for coding variants, exhibit different thresholds and cut-offs for variant scoring and have variable concordance with each other. Consequently, it is advised to utilise multiple in silico tools concurrently for variant interpretation, and a scoring criterion to ensure consistency. Variants predicted to be ‘deleterious’/’pathogenic’/’damaging’ by most of the tools will be given priority over variants predicted to be ‘benign’/’neutral’/’tolerated’ (95). VarSome (96) should also be used to further assess the impact of variants on protein structure and function. This tool is based on an accurate analysis of HTS data from numerous databases such as MetaRNN (97), DANN SNVs (98), UniProt (99), dbscSNV (100), gnomAD and ClinVar (101). Moreover, VarSome automatically performs the classification of genetic variants according to the ACMG/AMP guidelines.
vi. To further curate the variant list, those residing in genes involved directly or indirectly in bone physiology or expressed in bone tissue can be prioritised. Some examples of resources and online databases which can be utilised include: Mouse Genome Informatics (http://www.informatics.jax.org) (102), the Musculoskeletal Knowledge Portal (http://mskkp.org/) (103), International Mouse Phenotyping Consortium (https://www.mousephenotype.org/) (104), Gene Ontology (http://www.geneontology.org) (105), Online Mendelian Inheritance in Man (http://omim.org) (106), HumanBase network (https://hb.flatironinstitute.org/) (107), Kyoto Encyclopaedia of Genes and Genomes (KEGG) Pathway (available at http://www.genome.jp/kegg/) (108), and QIAGEN’s Ingenuity Pathway Analysis® (109).
3.5 Confirmation of shortlisted variants and co-segregation studies
All remaining shortlisted variants should be analysed in the IGV software (Integrative Genomics Viewer, Broad Institute and the Regents of the University of California, USA) (110) for the distinction between true variants and false-positive hits caused by misalignments or inaccurate bioinformatics processing results. Moreover, IGV is used to compute the read coverage in the viewed region and allele ratios for the observed genotypes. Sanger sequencing can be used as a secondary approach to further verify the shortlisted variants. Furthermore, this method should also be used to determine the variants’ segregation across the non-sequenced relatives in the pedigree. Ideally, the shortlisted causal variant should only be present in the affected relatives. However, the consideration of unaffected carriers should not be excluded due to factors such as incomplete penetrance or the late onset of the disease (Figure 1). Oligogenic inheritance, characterised by the segregation of multiple variants within a pedigree, is also a plausible scenario.
3.6 Replication and validation of the shortlisted variants
The shortlisted variants can be tested in other affected families to provide further proof of disease gene causation. A genetic variant within the same potentially causal gene should be identified in at least two independent, unrelated probands or families having the same disease (or phenotype). This criterion helps establish a more robust association between the gene and the phenotype, reducing the likelihood that the association is coincidental. GeneMatcher (111) was specifically set up to enable clinicians and researchers to make such connections. Additionally, the shortlisted variant(s) or others residing within the identified deleterious gene should be sought in large collections of unrelated individuals to determine whether they associate with BMD and other musculoskeletal phenotypes at the population level. Finally, functional assessment using in vitro cells and in vivo (e.g., mice or zebrafish) models (Figure 2), as well as expression studies, are warranted to understand the gene and/or variant’s biological role in bone and osteoporosis pathogenesis. The identification of a high impact, loss-of-function variant can be considered analogous to a “human knockout” for that gene. The shortlisted variant, the mode of inheritance of the disease, gene expression levels, and the functional characteristics of the associated protein should all be taken into consideration when designing a functional study, described further elsewhere (28, 112).
4 Discussion: limitations and future work
While family-based studies are a powerful tool in genetic research, there are several pitfalls that one must consider, including the presence of phenocopies and incomplete penetrance (Figure 1). Integrating additional methodologies, such as long-read sequencing (that is better suited to capture large SVs), multi-omics studies, and advanced computational models (e.g., machine-learning tools) (113), can help overcome some challenges leading to a more comprehensive understanding of the genetic and phenotypic relationships. In fact, the integration of genomic and metabolomic data provided further proof of the role of SGMS2 in osteoporosis and skeletal dysplasia (40). A multi-omics approach can provide a holistic integrated view from a system biology perspective, capturing the complexity of the underlying pathological mechanisms, and presenting opportunities for biomarker discovery. Furthermore, the importance of a multidisciplinary team involving clinical, basic and translational researchers, and bioinformaticians is becoming more evident for improved patient care with timely diagnosis and optimal treatment options. Active collaboration in international scientific consortia (e.g., GEFOS and GENOMOS), European Reference Networks (e.g., European Network for Rare Bone Conditions, ERN BOND), disease registries and patient organisations is the way forward.
5 Conclusion
As highlighted in this review, family-based studies have been instrumental in identifying genetic determinants governing bone metabolism and disease processes giving rise to osteoporosis and other bone mass disorders. To date, around 20% of the underlying genetic factors are known, emphasising the need for further research efforts in the field (114). Genes and variants uncovered in family studies may lead to the development of diagnostic biomarkers and drug targets based on an individual’s genetic make-up. This makes personalised medicine more of a reality, which is the ultimate goal of genomic studies.
Author contributions
MS: Conceptualization, Writing – original draft, Writing – review & editing. MF: Conceptualization, Funding acquisition, Supervision, Writing – original draft, Writing – review & editing.
Funding
The author(s) declare financial support was received for the research, authorship, and/or publication of this article. MS and MF received research funds from Project GRIT (R&I-2022-007L) financed by Xjenza Malta, for and behalf of the Foundation for Science and Technology, through the FUSION R&I Technology Development Programme Lite. MF is also supported by Projects ZeEBRA (R&I-2019-018T; a FUSION: R&I Technology Development Programme), DETERMINE (REP-2024-027; a FUSION: R&I Research Excellence Programme) and STRONG (R&I-2024-007L; a FUSION: R&I Technology Development Programme Lite) financed by Xjenza Malta, for and on behalf of the Foundation for Science and Technology, as well as BioGeMT (101086768), funded by the European Union.
Conflict of interest
The authors declare that the research was conducted in the absence of any commercial or financial relationships that could be construed as a potential conflict of interest.
Publisher’s note
All claims expressed in this article are solely those of the authors and do not necessarily represent those of their affiliated organizations, or those of the publisher, the editors and the reviewers. Any product that may be evaluated in this article, or claim that may be made by its manufacturer, is not guaranteed or endorsed by the publisher.
References
1. Ralston SH, Uitterlinden AG. Genetics of osteoporosis. Endocr Rev. (2010) 31:629–62. doi: 10.1210/er.2009-0044
2. Emkey GR, Epstein S. Secondary osteoporosis: pathophysiology & diagnosis. Best Pract Res Clin Endocrinol Metab. (2014) 28:911–35. doi: 10.1016/j.beem.2014.07.002
3. Mafi Golchin M, Heidari L, Ghaderian SM, Akhavan-Niaki H. Osteoporosis: A silent disease with complex genetic contribution. J Genet Genomics. (2016) 43:49–61. doi: 10.1016/j.jgg.2015.12.001
4. Urano T, Inoue S. Genetics of osteoporosis. Biochem Biophys Res Commun. (2014) 452:287–93. doi: 10.1016/j.bbrc.2014.07.141
5. Cooper C, Ferrari S. IOF Compendium of Osteoporosis, 2nd edition. (Nyon, Switzerland: International Osteoporosis Foundation (IOF)) (2019). pp. 1–90.
6. Kenkre JS, Bassett J. The bone remodelling cycle. Ann Clin Biochem. (2018) 55:308–27. doi: 10.1177/0004563218759371
7. Hendrickx G, Boudin E, Van Hul W. A look behind the scenes: the risk and pathogenesis of primary osteoporosis. Nat Rev Rheumatol. (2015) 11:462–74. doi: 10.1038/nrrheum.2015.48
8. Boudin E, Fijalkowski I, Hendrickx G, Van Hul W. Genetic control of bone mass. Mol Cell Endocrinol. (2016) 432:3–13. doi: 10.1016/j.mce.2015.12.021
9. Duncan EL, Brown MA. Clinical review 2: Genetic determinants of bone density and fracture risk–state of the art and future directions. J Clin Endocrinol Metab. (2010) 95:2576–87. doi: 10.1210/jc.2009-2406
10. Bez Batti Angulski A, Hosny N, Cohen H, Martin AA, Hahn D, Bauer J, et al. Duchenne muscular dystrophy: disease mechanism and therapeutic strategies. Front Physiol. (2023) 14:1183101. doi: 10.3389/fphys.2023.1183101
11. Lee T, Takeshima Y, Kusunoki N, Awano H, Yagi M, Matsuo M, et al. Differences in carrier frequency between mothers of Duchenne and Becker muscular dystrophy patients. J Hum Genet. (2014) 59:46–50. doi: 10.1038/jhg.2013.119
12. Sturchio A, Duker AP, Muñoz-Sanjuan I, Espay AJ. Subtyping monogenic disorders: Huntington disease. Handb Clin Neurol. (2023) 193:171–84. doi: 10.1016/b978-0-323-85555-6.00003-5
13. Knowles MR, Drumm M. The influence of genetics on cystic fibrosis phenotypes. Cold Spring Harb Perspect Med. (2012) 2:a009548. doi: 10.1101/cshperspect.a009548
14. Cleynen I, Halfvarsson J. How to approach understanding complex trait genetics - inflammatory bowel disease as a model complex trait. United Eur Gastroenterol J. (2019) 7:1426–30. doi: 10.1177/2050640619891120
15. Evangelou E, Trikalinos TA, Salanti G, Ioannidis JP. Family-based versus unrelated case-control designs for genetic associations. PLoS Genet. (2006) 2:e123. doi: 10.1371/journal.pgen.0020123
16. Almasy L. Family studies in the age of big data. Proc Natl Acad Sci USA. (2022) 119:e2200472119. doi: 10.1073/pnas.2200472119
17. Germain DP, Moiseev S, Suárez-Obando F, Al Ismaili F, Al Khawaja H, Altarescu G, et al. The benefits and challenges of family genetic testing in rare genetic diseases-lessons from Fabry disease. Mol Genet Genomic Med. (2021) 9:e1666. doi: 10.1002/mgg3.1666
18. Borecki IB, Province MA. Genetic and genomic discovery using family studies. Circulation. (2008) 118:1057–63. doi: 10.1161/circulationaha.107.714592
19. Cheung CL, Xiao SM, Kung AW. Genetic epidemiology of age-related osteoporosis and its clinical applications. Nat Rev Rheumatol. (2010) 6:507–17. doi: 10.1038/nrrheum.2010.106
20. Balemans W, Ebeling M, Patel N, Van Hul E, Olson P, Dioszegi M, et al. Increased bone density in sclerosteosis is due to the deficiency of a novel secreted protein (SOST). Hum Mol Genet. (2001) 10:537–43. doi: 10.1093/hmg/10.5.537
21. Balemans W, Patel N, Ebeling M, Van Hul E, Wuyts W, Lacza C, et al. Identification of a 52 kb deletion downstream of the SOST gene in patients with van Buchem disease. J Med Genet. (2002) 39:91–7. doi: 10.1136/jmg.39.2.91
22. Claeys L, Storoni S, Eekhoff M, Elting M, Wisse L, Pals G, et al. Collagen transport and related pathways in Osteogenesis Imperfecta. Hum Genet. (2021) 140:1121–41. doi: 10.1007/s00439-021-02302-2
23. Marini JC, Forlino A, Bächinger HP, Bishop NJ, Byers PH, Paepe A, et al. Osteogenesis imperfecta. Nat Rev Dis Primers. (2017) 3:17052. doi: 10.1038/nrdp.2017.52
24. Laine CM, Chung BD, Susic M, Prescott T, Semler O, Fiskerstrand T, et al. Novel mutations affecting LRP5 splicing in patients with osteoporosis-pseudoglioma syndrome (OPPG). Eur J Hum Genet. (2011) 19:875–81. doi: 10.1038/ejhg.2011.42
25. Lin WD, Wang CH, Wu KH, Chou IC, Tsai FJ. Identification and characterization of mutations in the CLCN7 gene in a Taiwanese patient with infantile Malignant osteopetrosis. Pediatr Neonatol. (2016) 57:155–7. doi: 10.1016/j.pedneo.2015.04.013
26. Cosman F, Crittenden DB, Adachi JD, Binkley N, Czerwinski E, Ferrari S, et al. Romosozumab treatment in postmenopausal women with osteoporosis. N Engl J Med. (2016) 375:1532–43. doi: 10.1056/NEJMoa1607948
27. Mäkitie RE, Costantini A, Kämpe A, Alm JJ, Mäkitie O. New insights into monogenic causes of osteoporosis. Front Endocrinol (Lausanne). (2019) 10:70. doi: 10.3389/fendo.2019.00070
28. Formosa MM, Bergen DJM, Gregson CL, Maurizi A, Kämpe A, Garcia-Giralt N, et al. A roadmap to gene discoveries and novel therapies in monogenic low and high bone mass disorders. Front Endocrinol (Lausanne). (2021) 12:709711. doi: 10.3389/fendo.2021.709711
29. Formosa MM, Christou MA, Mäkitie O. Bone fragility and osteoporosis in children and young adults. J Endocrinol Invest. (2024) 47:285–98. doi: 10.1007/s40618-023-02179-0
30. Keupp K, Beleggia F, Kayserili H, Barnes AM, Steiner M, Semler O, et al. Mutations in WNT1 cause different forms of bone fragility. Am J Hum Genet. (2013) 92:565–74. doi: 10.1016/j.ajhg.2013.02.010
31. Laine CM, Joeng KS, Campeau PM, Kiviranta R, Tarkkonen K, Grover M, et al. WNT1 mutations in early-onset osteoporosis and osteogenesis imperfecta. N Engl J Med. (2013) 368:1809–16. doi: 10.1056/NEJMoa1215458
32. van Dijk FS, Zillikens MC, Micha D, Riessland M, Marcelis CL, de Die-Smulders CE, et al. PLS3 mutations in X-linked osteoporosis with fractures. N Engl J Med. (2013) 369:1529–36. doi: 10.1056/NEJMoa1308223
33. Kämpe AJ, Costantini A, Levy-Shraga Y, Zeitlin L, Roschger P, Taylan F, et al. PLS3 deletions lead to severe spinal osteoporosis and disturbed bone matrix mineralization. J Bone Miner Res. (2017) 32:2394–404. doi: 10.1002/jbmr.3233
34. Mäkitie RE, Niinimäki T, Suo-Palosaari M, Kämpe A, Costantini A, Toiviainen-Salo S, et al. PLS3 mutations cause severe age and sex-related spinal pathology. Front Endocrinol (Lausanne). (2020) 11:393. doi: 10.3389/fendo.2020.00393
35. Wesseling-Perry K, Mäkitie RE, Välimäki VV, Laine T, Laine CM, Välimäki MJ, et al. Osteocyte protein expression is altered in low-turnover osteoporosis caused by mutations in WNT1 and PLS3. J Clin Endocrinol Metab. (2017) 102:2340–8. doi: 10.1210/jc.2017-00099
36. Korvala J, Jüppner H, Mäkitie O, Sochett E, Schnabel D, Mora S, et al. Mutations in LRP5 cause primary osteoporosis without features of OI by reducing Wnt signaling activity. BMC Med Genet. (2012) 13:26. doi: 10.1186/1471-2350-13-26
37. Ferrari SL, Deutsch S, Baudoin C, Cohen-Solal M, Ostertag A, Antonarakis SE, et al. LRP5 gene polymorphisms and idiopathic osteoporosis in men. Bone. (2005) 37:770–5. doi: 10.1016/j.bone.2005.06.017
38. Koay MA, Woon PY, Zhang Y, Miles LJ, Duncan EL, Ralston SH, et al. Influence of LRP5 polymorphisms on normal variation in BMD. J Bone Miner Res. (2004) 19:1619–27. doi: 10.1359/jbmr.040704
39. Lauretani F, Cepollaro C, Bandinelli S, Cherubini A, Gozzini A, Masi L, et al. LRP5 gene polymorphism and cortical bone. Aging Clin Exp Res. (2010) 22:281–8. doi: 10.1007/bf03324935
40. Pekkinen M, Terhal PA, Botto LD, Henning P, Mäkitie RE, Roschger P, et al. Osteoporosis and skeletal dysplasia caused by pathogenic variants in SGMS2. JCI Insight. (2019) 4:e126180. doi: 10.1172/jci.insight.126180
41. Mäkitie RE, Henning P, Jiu Y, Kämpe A, Kogan K, Costantini A, et al. An ARHGAP25 variant links aberrant Rac1 function to early-onset skeletal fragility. JBMR Plus. (2021) 5:e10509. doi: 10.1002/jbm4.10509
42. Caetano da Silva C, Edouard T, Fradin M, Aubert-Mucca M, Ricquebourg M, Raman R, et al. WNT11, a new gene associated with early onset osteoporosis, is required for osteoblastogenesis. Hum Mol Genet. (2022) 31:1622–34. doi: 10.1093/hmg/ddab349
43. Block TJ, Shore-Lorenti C, Zebaze R, Kerr PG, Kalff A, Perkins AC, et al. A novel RUNX1 genetic variant identified in a young male with severe osteoporosis. JBMR Plus. (2023) 7:e10791. doi: 10.1002/jbm4.10791
44. Dantsev IS, Parfenenko MA, Radzhabova GM, Nikolaeva EA. An FGFR2 mutation as the potential cause of a new phenotype including early-onset osteoporosis and bone fractures: a case report. BMC Med Genomics. (2023) 16:329. doi: 10.1186/s12920-023-01750-1
45. Kohli MA, Cukier HN, Hamilton-Nelson KL, Rolati S, Kunkle BW, Whitehead PL, et al. Segregation of a rare TTC3 variant in an extended family with late-onset Alzheimer disease. Neurol Genet. (2016) 2:e41. doi: 10.1212/nxg.0000000000000041
46. Deng HX, Shi Y, Yang Y, Ahmeti KB, Miller N, Huang C, et al. Identification of TMEM230 mutations in familial Parkinson's disease. Nat Genet. (2016) 48:733–9. doi: 10.1038/ng.3589
47. Prudente S, Jungtrakoon P, Marucci A, Ludovico O, Buranasupkajorn P, Mazza T, et al. Loss-of-function mutations in APPL1 in familial diabetes mellitus. Am J Hum Genet. (2015) 97:177–85. doi: 10.1016/j.ajhg.2015.05.011
48. Tanaka D, Nagashima K, Sasaki M, Funakoshi S, Kondo Y, Yasuda K, et al. Exome sequencing identifies a new candidate mutation for susceptibility to diabetes in a family with highly aggregated type 2 diabetes. Mol Genet Metab. (2013) 109:112–7. doi: 10.1016/j.ymgme.2013.02.010
49. Pace NP, Rizzo C, Abela A, Gruppetta M, Fava S, Felice A, et al. Identification of an HNF1A p.Gly292fs frameshift mutation presenting as diabetes during pregnancy in a maltese family. Clin Med Insights Case Rep. (2019) 12:1179547619831034. doi: 10.1177/1179547619831034
50. Johnson M, Løset M, Brennecke S, Peralta J, Dyer T, East C, et al. OS049. Exome sequencing identifies likely functional variants influencing preeclampsia and CVD risk. Pregnancy Hypertens. (2012) 2:203–4. doi: 10.1016/j.preghy.2012.04.050
51. Emrahi L, Hosseinzadeh H, Tabrizi MT. Two rare variants in the MYBPC3 gene associated with familial hypertrophic cardiomyopathy. Gene Rep. (2022) 26:101471. doi: 10.1016/j.genrep.2021.101471
52. Costantini A, Mäkitie RE, Hartmann MA, Fratzl-Zelman N, Zillikens MC, Kornak U, et al. Early-onset osteoporosis: rare monogenic forms elucidate the complexity of disease pathogenesis beyond type I collagen. J Bone Miner Res. (2022) 37:1623–41. doi: 10.1002/jbmr.4668
53. Mäkitie O, Zillikens MC. Early-onset osteoporosis. Calcif Tissue Int. (2022) 110:546–61. doi: 10.1007/s00223-021-00885-6
54. Ciancia S, van Rijn RR, Högler W, Appelman-Dijkstra NM, Boot AM, Sas TCJ, et al. Osteoporosis in children and adolescents: when to suspect and how to diagnose it. Eur J Pediatr. (2022) 181:2549–61. doi: 10.1007/s00431-022-04455-2
55. Kanis JA. Diagnosis of osteoporosis and assessment of fracture risk. Lancet. (2002) 359:1929–36. doi: 10.1016/s0140-6736(02)08761-5
56. Leib ES, Lewiecki EM, Binkley N, Hamdy RC. Official positions of the international society for clinical densitometry. J Clin Densitom. (2004) 7:1–6. doi: 10.1385/jcd:7:1:1
57. Dahn HA, Mountcastle J, Balacco J, Winkler S, Bista I, Schmitt AD, et al. Benchmarking ultra-high molecular weight DNA preservation methods for long-read and long-range sequencing. Gigascience. (2022) 11:1–13. doi: 10.1093/gigascience/giac068
58. Meynert AM, Ansari M, FitzPatrick DR, Taylor MS. Variant detection sensitivity and biases in whole genome and exome sequencing. BMC Bioinf. (2014) 15:247. doi: 10.1186/1471-2105-15-247
59. Erzurumluoglu AM, Rodriguez S, Shihab HA, Baird D, Richardson TG, Day IN, et al. Identifying highly penetrant disease causal mutations using next generation sequencing: guide to whole process. BioMed Res Int. (2015) 2015:923491. doi: 10.1155/2015/923491
60. Andrews S. FastQC: A Quality Control Tool for High Throughput Sequence Data. Available online at: http://www.bioinformatics.babraham.ac.uk/projects/fastqc/2010 (accessed date May 25, 2024).
61. Nassar LR, Barber GP, Benet-Pagès A, Casper J, Clawson H, Diekhans M, et al. The UCSC Genome Browser database: 2023 update. Nucleic Acids Res. (2023) 51:D1188–d95. doi: 10.1093/nar/gkac1072
62. O'Leary NA, Wright MW, Brister JR, Ciufo S, Haddad D, McVeigh R, et al. Reference sequence (RefSeq) database at NCBI: current status, taxonomic expansion, and functional annotation. Nucleic Acids Res. (2016) 44:D733–45. doi: 10.1093/nar/gkv1189
63. Li H, Durbin R. Fast and accurate short read alignment with Burrows-Wheeler transform. Bioinformatics. (2009) 25:1754–60. doi: 10.1093/bioinformatics/btp324
64. Lee WP, Stromberg MP, Ward A, Stewart C, Garrison EP, Marth GT. MOSAIK: a hash-based algorithm for accurate next-generation sequencing short-read mapping. PLoS One. (2014) 9:e90581. doi: 10.1371/journal.pone.0090581
65. Langmead B, Trapnell C, Pop M, Salzberg SL. Ultrafast and memory-efficient alignment of short DNA sequences to the human genome. Genome Biol. (2009) 10:R25. doi: 10.1186/gb-2009-10-3-r25
66. Chen S, Zhou Y, Chen Y, Gu J. fastp: an ultra-fast all-in-one FASTQ preprocessor. Bioinformatics. (2018) 34:i884–i90. doi: 10.1093/bioinformatics/bty560
67. McKenna A, Hanna M, Banks E, Sivachenko A, Cibulskis K, Kernytsky A, et al. The Genome Analysis Toolkit: a MapReduce framework for analyzing next-generation DNA sequencing data. Genome Res. (2010) 20:1297–303. doi: 10.1101/gr.107524.110
68. Li H, Handsaker B, Wysoker A, Fennell T, Ruan J, Homer N, et al. The sequence alignment/map format and SAMtools. Bioinformatics. (2009) 25:2078–9. doi: 10.1093/bioinformatics/btp352
69. Poplin R, Chang PC, Alexander D, Schwartz S, Colthurst T, Ku A, et al. A universal SNP and small-indel variant caller using deep neural networks. Nat Biotechnol. (2018) 36:983–7. doi: 10.1038/nbt.4235
70. Koboldt DC, Zhang Q, Larson DE, Shen D, McLellan MD, Lin L, et al. VarScan 2: somatic mutation and copy number alteration discovery in cancer by exome sequencing. Genome Res. (2012) 22:568–76. doi: 10.1101/gr.129684.111
71. Layer RM, Chiang C, Quinlan AR, Hall IM. LUMPY: a probabilistic framework for structural variant discovery. Genome Biol. (2014) 15:R84. doi: 10.1186/gb-2014-15-6-r84
72. Chen X, Schulz-Trieglaff O, Shaw R, Barnes B, Schlesinger F, Källberg M, et al. Manta: rapid detection of structural variants and indels for germline and cancer sequencing applications. Bioinformatics. (2016) 32:1220–2. doi: 10.1093/bioinformatics/btv710
73. Abyzov A, Urban AE, Snyder M, Gerstein M. CNVnator: an approach to discover, genotype, and characterize typical and atypical CNVs from family and population genome sequencing. Genome Res. (2011) 21:974–84. doi: 10.1101/gr.114876.110
74. Talevich E, Shain AH, Botton T, Bastian BC. CNVkit: genome-wide copy number detection and visualization from targeted DNA sequencing. PLoS Comput Biol. (2016) 12:e1004873. doi: 10.1371/journal.pcbi.1004873
75. McLaren W, Gil L, Hunt SE, Riat HS, Ritchie GR, Thormann A, et al. The ensembl variant effect predictor. Genome Biol. (2016) 17:122. doi: 10.1186/s13059-016-0974-4
76. Cingolani P, Platts A, Wang le L, Coon M, Nguyen T, Wang L, et al. A program for annotating and predicting the effects of single nucleotide polymorphisms, SnpEff: SNPs in the genome of Drosophila melanogaster strain w1118; iso-2; iso-3. Fly (Austin). (2012) 6:80–92. doi: 10.4161/fly.19695
77. Auton A, Abecasis GR, Altshuler DM, Durbin RM, Abecasis GR, Bentley DR, et al. A global reference for human genetic variation. Nature. (2015) 526:68–74. doi: 10.1038/nature15393
78. Bhagwat M. Searching NCBI's dbSNP database. Curr Protoc Bioinf. (2010) Chapter 1:Unit 1.19. doi: 10.1002/0471250953.bi0119s32
79. Karczewski KJ, Francioli LC, Tiao G, Cummings BB, Alföldi J, Wang Q, et al. The mutational constraint spectrum quantified from variation in 141,456 humans. Nature. (2020) 581:434–43. doi: 10.1038/s41586-020-2308-7
80. Pollard KS, Hubisz MJ, Rosenbloom KR, Siepel A. Detection of nonneutral substitution rates on mammalian phylogenies. Genome Res. (2010) 20:110–21. doi: 10.1101/gr.097857.109
81. Spies N, Weng Z, Bishara A, McDaniel J, Catoe D, Zook JM, et al. Genome-wide reconstruction of complex structural variants using read clouds. Nat Methods. (2017) 14:915–20. doi: 10.1038/nmeth.4366
82. Adzhubei IA, Schmidt S, Peshkin L, Ramensky VE, Gerasimova A, Bork P, et al. A method and server for predicting damaging missense mutations. Nat Methods. (2010) 7:248–9. doi: 10.1038/nmeth0410-248
83. Sim NL, Kumar P, Hu J, Henikoff S, Schneider G, Ng PC. SIFT web server: predicting effects of amino acid substitutions on proteins. Nucleic Acids Res. (2012) 40:W452–7. doi: 10.1093/nar/gks539
84. Chun S, Fay JC. Identification of deleterious mutations within three human genomes. Genome Res. (2009) 19:1553–61. doi: 10.1101/gr.092619.109
85. Douville C, Masica DL, Stenson PD, Cooper DN, Gygax DM, Kim R, et al. Assessing the pathogenicity of insertion and deletion variants with the variant effect scoring tool (VEST-indel). Hum Mutat. (2016) 37:28–35. doi: 10.1002/humu.22911
86. Schwarz JM, Rödelsperger C, Schuelke M, Seelow D. MutationTaster evaluates disease-causing potential of sequence alterations. Nat Methods. (2010) 7:575–6. doi: 10.1038/nmeth0810-575
87. Reva B, Antipin Y, Sander C. Predicting the functional impact of protein mutations: application to cancer genomics. Nucleic Acids Res. (2011) 39:e118. doi: 10.1093/nar/gkr407
88. Dong C, Wei P, Jian X, Gibbs R, Boerwinkle E, Wang K, et al. Comparison and integration of deleteriousness prediction methods for nonsynonymous SNVs in whole exome sequencing studies. Hum Mol Genet. (2015) 24:2125–37. doi: 10.1093/hmg/ddu733
89. Shihab HA, Gough J, Cooper DN, Stenson PD, Barker GL, Edwards KJ, et al. Predicting the functional, molecular, and phenotypic consequences of amino acid substitutions using hidden Markov models. Hum Mutat. (2013) 34:57–65. doi: 10.1002/humu.22225
90. Kim S, Jhong JH, Lee J, Koo JY. Meta-analytic support vector machine for integrating multiple omics data. BioData Min. (2017) 10:2. doi: 10.1186/s13040-017-0126-8
91. Fuentes Fajardo KV, Adams D, Mason CE, Sincan M, Tifft C, Toro C, et al. Detecting false-positive signals in exome sequencing. Hum Mutat. (2012) 33:609–13. doi: 10.1002/humu.22033
92. Shyr C, Tarailo-Graovac M, Gottlieb M, Lee JJ, van Karnebeek C, Wasserman WW. Correction to: FLAGS, frequently mutated genes in public exomes. BMC Med Genomics. (2017) 10:69. doi: 10.1186/s12920-017-0309-7
93. Rentzsch P, Witten D, Cooper GM, Shendure J, Kircher M. CADD: predicting the deleteriousness of variants throughout the human genome. Nucleic Acids Res. (2019) 47:D886–d94. doi: 10.1093/nar/gky1016
94. Richards S, Aziz N, Bale S, Bick D, Das S, Gastier-Foster J, et al. Standards and guidelines for the interpretation of sequence variants: a joint consensus recommendation of the American College of Medical Genetics and Genomics and the Association for Molecular Pathology. Genet Med. (2015) 17:405–24. doi: 10.1038/gim.2015.30
95. Ghosh R, Oak N, Plon SE. Evaluation of in silico algorithms for use with ACMG/AMP clinical variant interpretation guidelines. Genome Biol. (2017) 18:225. doi: 10.1186/s13059-017-1353-5
96. Kopanos C, Tsiolkas V, Kouris A, Chapple CE, Albarca Aguilera M, Meyer R, et al. VarSome: the human genomic variant search engine. Bioinformatics. (2019) 35:1978–80. doi: 10.1093/bioinformatics/bty897
97. Li C, Zhi D, Wang K, Liu X. MetaRNN: differentiating rare pathogenic and rare benign missense SNVs and InDels using deep learning. Genome Med. (2022) 14:115. doi: 10.1186/s13073-022-01120-z
98. Quang D, Chen Y, Xie X. DANN: a deep learning approach for annotating the pathogenicity of genetic variants. Bioinformatics. (2015) 31:761–3. doi: 10.1093/bioinformatics/btu703
99. UniProt Consortium T. UniProt: the universal protein knowledgebase. Nucleic Acids Res. (2018) 46:2699. doi: 10.1093/nar/gky092
100. Jian X, Boerwinkle E, Liu X. In silico prediction of splice-altering single nucleotide variants in the human genome. Nucleic Acids Res. (2014) 42:13534–44. doi: 10.1093/nar/gku1206
101. Landrum MJ, Lee JM, Riley GR, Jang W, Rubinstein WS, Church DM, et al. ClinVar: public archive of relationships among sequence variation and human phenotype. Nucleic Acids Res. (2014) 42:D980–5. doi: 10.1093/nar/gkt1113
102. Eppig JT, Richardson JE, Kadin JA, Ringwald M, Blake JA, Bult CJ. Mouse Genome Informatics (MGI): reflecting on 25 years. Mamm Genome. (2015) 26:272–84. doi: 10.1007/s00335-015-9589-4
103. Westendorf JJ, Bonewald LF, Kiel DP, Burtt NP. The Musculoskeletal Knowledge Portal: improving access to multi-omics data. Nat Rev Rheumatol. (2022) 18:1–2. doi: 10.1038/s41584-021-00711-1
104. Muñoz-Fuentes V, Cacheiro P, Meehan TF, Aguilar-Pimentel JA, Brown SDM, Flenniken AM, et al. The International Mouse Phenotyping Consortium (IMPC): a functional catalogue of the mammalian genome that informs conservation. Conserv Genet. (2018) 19:995–1005. doi: 10.1007/s10592-018-1072-9
105. Ashburner M, Ball CA, Blake JA, Botstein D, Butler H, Cherry JM, et al. Gene ontology: tool for the unification of biology. The Gene Ontology Consortium. Nat Genet. (2000) 25:25–9. doi: 10.1038/75556
106. Hamosh A, Scott AF, Amberger JS, Bocchini CA, McKusick VA. Online Mendelian Inheritance in Man (OMIM), a knowledgebase of human genes and genetic disorders. Nucleic Acids Res. (2005) 33:D514–7. doi: 10.1093/nar/gki033
107. Greene CS, Krishnan A, Wong AK, Ricciotti E, Zelaya RA, Himmelstein DS, et al. Understanding multicellular function and disease with human tissue-specific networks. Nat Genet. (2015) 47:569–76. doi: 10.1038/ng.3259
108. Kanehisa M, Sato Y, Kawashima M, Furumichi M, Tanabe M. KEGG as a reference resource for gene and protein annotation. Nucleic Acids Res. (2016) 44:D457–62. doi: 10.1093/nar/gkv1070
109. Krämer A, Green J, Pollard J Jr., Tugendreich S. Causal analysis approaches in Ingenuity Pathway Analysis. Bioinformatics. (2014) 30:523–30. doi: 10.1093/bioinformatics/btt703
110. Robinson JT, Thorvaldsdóttir H, Winckler W, Guttman M, Lander ES, Getz G, et al. Integrative genomics viewer. Nat Biotechnol. (2011) 29:24–6. doi: 10.1038/nbt.1754
111. Sobreira N, Schiettecatte F, Valle D, Hamosh A. GeneMatcher: a matching tool for connecting investigators with an interest in the same gene. Hum Mutat. (2015) 36:928–30. doi: 10.1002/humu.22844
112. Rauner M, Foessl I, Formosa MM, Kague E, Prijatelj V, Lopez NA, et al. Perspective of the GEMSTONE consortium on current and future approaches to functional validation for skeletal genetic disease using cellular, molecular and animal-modeling techniques. Front Endocrinol (Lausanne). (2021) 12:731217. doi: 10.3389/fendo.2021.731217
113. Lin J, Jia P, Wang S, Kosters W, Ye K. Comparison and benchmark of structural variants detected from long read and long-read assembly. Brief Bioinform. (2023) 24:1–12. doi: 10.1093/bib/bbad188
Keywords: genetics, osteoporosis, family studies, fragility fractures, high-throughput sequencing
Citation: Schembri M and Formosa MM (2024) Identification of osteoporosis genes using family studies. Front. Endocrinol. 15:1455689. doi: 10.3389/fendo.2024.1455689
Received: 27 June 2024; Accepted: 29 September 2024;
Published: 22 October 2024.
Edited by:
Gretl Hendrickx, KU Leuven, BelgiumReviewed by:
Patricia Canto, National Autonomous University of Mexico, MexicoCopyright © 2024 Schembri and Formosa. This is an open-access article distributed under the terms of the Creative Commons Attribution License (CC BY). The use, distribution or reproduction in other forums is permitted, provided the original author(s) and the copyright owner(s) are credited and that the original publication in this journal is cited, in accordance with accepted academic practice. No use, distribution or reproduction is permitted which does not comply with these terms.
*Correspondence: Melissa M. Formosa, bWVsaXNzYS5tLmZvcm1vc2FAdW0uZWR1Lm10